File size: 2,917 Bytes
b1c6cc2 81dd14b b1c6cc2 0f700a8 f856d73 b1c6cc2 1dec43e b1c6cc2 0f700a8 b1c6cc2 d1128de b1c6cc2 5c3c179 f856d73 ab80208 b1c6cc2 0f700a8 b1c6cc2 f8886c5 b1c6cc2 0f700a8 b1c6cc2 0f700a8 c423023 4ec18d4 c423023 0f700a8 b1c6cc2 f8886c5 b1c6cc2 0f700a8 b1c6cc2 c6ca8d2 b1c6cc2 0f700a8 |
1 2 3 4 5 6 7 8 9 10 11 12 13 14 15 16 17 18 19 20 21 22 23 24 25 26 27 28 29 30 31 32 33 34 35 36 37 38 39 40 41 42 43 44 45 46 47 48 49 50 51 52 53 54 55 56 57 58 59 60 61 62 63 64 65 66 67 68 69 70 71 72 73 74 75 76 |
---
license: llama2
model_type: llama
tags:
- facebook
- meta
- pytorch
- llama
- llama-2
- Storywriter
---
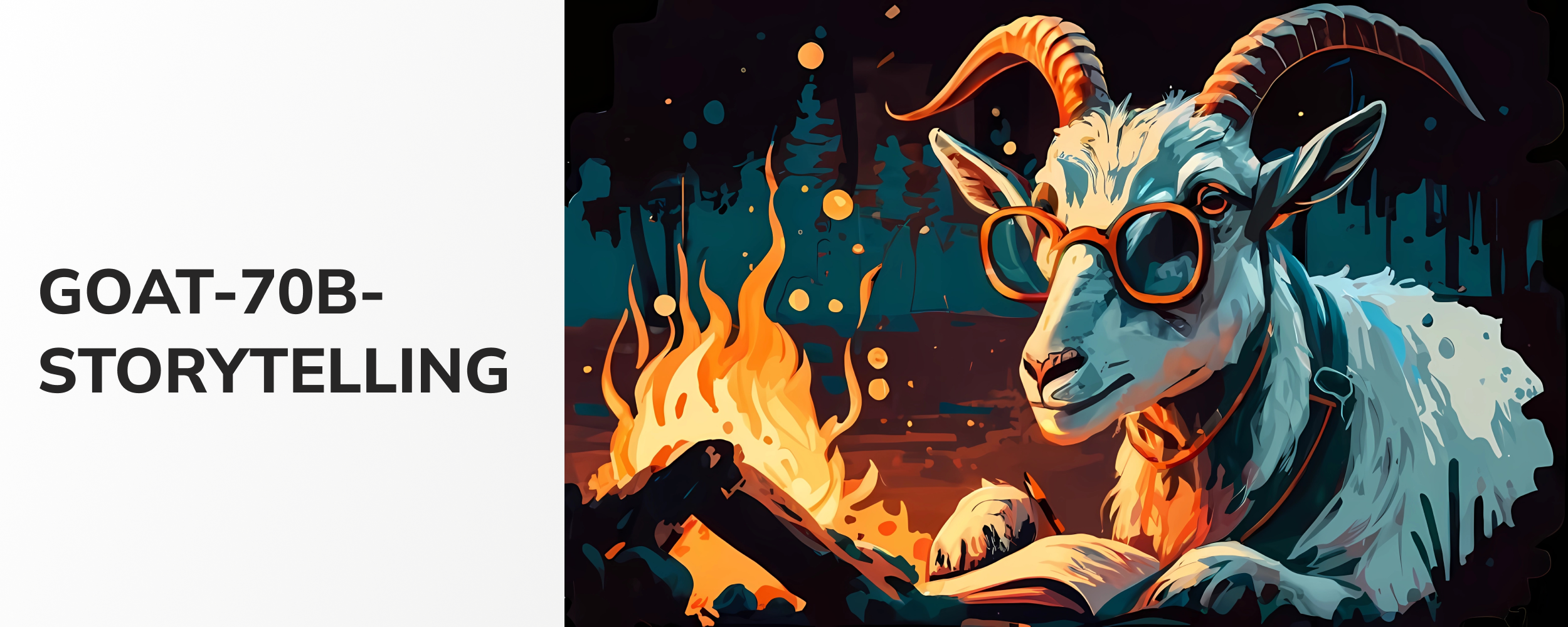
# GOAT-70B-Storytelling model
GOAT-70B-Storytelling model trained by GOAT.AI lab as a core model for autonomous story-writing agent.
# GOAT-Storytelling-Agent
The GOAT-70B-Storytelling model has been developed as an integral component within the GOAT-Storytelling-Agent. This agent facilitates the generation of high-quality, cohesive, and captivating narratives, including stories and books. It achieves this by utilizing inputs such as plot outlines, character profiles, their interrelationships, and other relevant details. Example is provided below.
# Model description
- **Base Architecture:** LLaMA 2 70B
- **License:** llama2
- **Context window length:** 4096 tokens
### Training details
For training, we apply the standard recipe with learning rate 1e-5, batch size per GPU 6, optimizer AdamW without weight decay and we train the model via ZeRO-3 on 64xH100 GPU cluster
### Learn more
- **Blogpost:** [GOAT-Storytelling: Arbitrarily Long Story Writing Agent](https://www.blog.goat.ai/goat-st/)
- **GitHub:** [here](github.com/GOAT-AI-lab/GOAT-Storytelling-Agent)
- **Generated examples:** [here](https://huggingface.co/datasets/GOAT-AI/generated-novels)
## Uses
The main purpose of GOAT-70B-Storytelling is to generate books, novels, movie scripts and etc. as an agent in coping with our GOAT-Storytelling-Agent. It is specifically designed for storywriters.
## Usage
Usage can be either self-hosted via `transformers` or used with Spaces
```python
import torch
from transformers import AutoTokenizer, AutoModelForCausalLM
model_name = "GOAT-AI/GOAT-70B-Storytelling"
tokenizer = AutoTokenizer.from_pretrained(model_name)
model = AutoModelForCausalLM.from_pretrained(
model_name,
torch_dtype=torch.bfloat16
)
```
Currently, we support LLM endpoint generation, where you need to send a post request to the generation endpoint (we recommend using Text Generation Inference by HuggingFace)
First, modify config.py and add your generation endpoint.
Then you can use it inside via GOAT-Storytelling-Agent:
```python
from goat_storytelling_agent.story_processor.prompt_manager import generate_story
novel_scenes = generate_story('never too much coffee', form='novel')
```
## License
GOAT-70B-Storytelling model is based on [Meta's LLaMA-2-70b-hf](https://huggingface.co/meta-llama/Llama-2-70b-hf), and using own datasets.
GOAT-70B-Storytelling model weights are available under LLAMA-2 license.
### Risks and Biases
GOAT-70B-Storytelling model can produce factually incorrect output and should not be relied on to deliver factually accurate information. Therefore, the GOAT-70B-Storytelling model could possibly generate wrong, biased, or otherwise offensive outputs. |