Upload README.md with huggingface_hub
Browse files
README.md
CHANGED
@@ -1,199 +1,256 @@
|
|
|
|
1 |
---
|
2 |
-
|
3 |
-
|
|
|
|
|
|
|
|
|
|
|
|
|
|
|
|
|
|
|
4 |
---
|
5 |
|
6 |
-
# Model Card for Model ID
|
7 |
-
|
8 |
-
<!-- Provide a quick summary of what the model is/does. -->
|
9 |
-
|
10 |
-
|
11 |
-
|
12 |
-
## Model Details
|
13 |
-
|
14 |
-
### Model Description
|
15 |
-
|
16 |
-
<!-- Provide a longer summary of what this model is. -->
|
17 |
-
|
18 |
-
This is the model card of a 🤗 transformers model that has been pushed on the Hub. This model card has been automatically generated.
|
19 |
-
|
20 |
-
- **Developed by:** [More Information Needed]
|
21 |
-
- **Funded by [optional]:** [More Information Needed]
|
22 |
-
- **Shared by [optional]:** [More Information Needed]
|
23 |
-
- **Model type:** [More Information Needed]
|
24 |
-
- **Language(s) (NLP):** [More Information Needed]
|
25 |
-
- **License:** [More Information Needed]
|
26 |
-
- **Finetuned from model [optional]:** [More Information Needed]
|
27 |
-
|
28 |
-
### Model Sources [optional]
|
29 |
-
|
30 |
-
<!-- Provide the basic links for the model. -->
|
31 |
-
|
32 |
-
- **Repository:** [More Information Needed]
|
33 |
-
- **Paper [optional]:** [More Information Needed]
|
34 |
-
- **Demo [optional]:** [More Information Needed]
|
35 |
-
|
36 |
-
## Uses
|
37 |
-
|
38 |
-
<!-- Address questions around how the model is intended to be used, including the foreseeable users of the model and those affected by the model. -->
|
39 |
-
|
40 |
-
### Direct Use
|
41 |
-
|
42 |
-
<!-- This section is for the model use without fine-tuning or plugging into a larger ecosystem/app. -->
|
43 |
-
|
44 |
-
[More Information Needed]
|
45 |
-
|
46 |
-
### Downstream Use [optional]
|
47 |
-
|
48 |
-
<!-- This section is for the model use when fine-tuned for a task, or when plugged into a larger ecosystem/app -->
|
49 |
-
|
50 |
-
[More Information Needed]
|
51 |
-
|
52 |
-
### Out-of-Scope Use
|
53 |
-
|
54 |
-
<!-- This section addresses misuse, malicious use, and uses that the model will not work well for. -->
|
55 |
-
|
56 |
-
[More Information Needed]
|
57 |
-
|
58 |
-
## Bias, Risks, and Limitations
|
59 |
-
|
60 |
-
<!-- This section is meant to convey both technical and sociotechnical limitations. -->
|
61 |
-
|
62 |
-
[More Information Needed]
|
63 |
-
|
64 |
-
### Recommendations
|
65 |
-
|
66 |
-
<!-- This section is meant to convey recommendations with respect to the bias, risk, and technical limitations. -->
|
67 |
-
|
68 |
-
Users (both direct and downstream) should be made aware of the risks, biases and limitations of the model. More information needed for further recommendations.
|
69 |
-
|
70 |
-
## How to Get Started with the Model
|
71 |
-
|
72 |
-
Use the code below to get started with the model.
|
73 |
-
|
74 |
-
[More Information Needed]
|
75 |
-
|
76 |
-
## Training Details
|
77 |
-
|
78 |
-
### Training Data
|
79 |
-
|
80 |
-
<!-- This should link to a Dataset Card, perhaps with a short stub of information on what the training data is all about as well as documentation related to data pre-processing or additional filtering. -->
|
81 |
-
|
82 |
-
[More Information Needed]
|
83 |
-
|
84 |
-
### Training Procedure
|
85 |
-
|
86 |
-
<!-- This relates heavily to the Technical Specifications. Content here should link to that section when it is relevant to the training procedure. -->
|
87 |
-
|
88 |
-
#### Preprocessing [optional]
|
89 |
-
|
90 |
-
[More Information Needed]
|
91 |
-
|
92 |
-
|
93 |
-
#### Training Hyperparameters
|
94 |
-
|
95 |
-
- **Training regime:** [More Information Needed] <!--fp32, fp16 mixed precision, bf16 mixed precision, bf16 non-mixed precision, fp16 non-mixed precision, fp8 mixed precision -->
|
96 |
-
|
97 |
-
#### Speeds, Sizes, Times [optional]
|
98 |
-
|
99 |
-
<!-- This section provides information about throughput, start/end time, checkpoint size if relevant, etc. -->
|
100 |
-
|
101 |
-
[More Information Needed]
|
102 |
-
|
103 |
-
## Evaluation
|
104 |
-
|
105 |
-
<!-- This section describes the evaluation protocols and provides the results. -->
|
106 |
-
|
107 |
-
### Testing Data, Factors & Metrics
|
108 |
-
|
109 |
-
#### Testing Data
|
110 |
-
|
111 |
-
<!-- This should link to a Dataset Card if possible. -->
|
112 |
-
|
113 |
-
[More Information Needed]
|
114 |
-
|
115 |
-
#### Factors
|
116 |
-
|
117 |
-
<!-- These are the things the evaluation is disaggregating by, e.g., subpopulations or domains. -->
|
118 |
-
|
119 |
-
[More Information Needed]
|
120 |
-
|
121 |
-
#### Metrics
|
122 |
-
|
123 |
-
<!-- These are the evaluation metrics being used, ideally with a description of why. -->
|
124 |
-
|
125 |
-
[More Information Needed]
|
126 |
-
|
127 |
-
### Results
|
128 |
-
|
129 |
-
[More Information Needed]
|
130 |
-
|
131 |
-
#### Summary
|
132 |
-
|
133 |
-
|
134 |
-
|
135 |
-
## Model Examination [optional]
|
136 |
-
|
137 |
-
<!-- Relevant interpretability work for the model goes here -->
|
138 |
-
|
139 |
-
[More Information Needed]
|
140 |
-
|
141 |
-
## Environmental Impact
|
142 |
-
|
143 |
-
<!-- Total emissions (in grams of CO2eq) and additional considerations, such as electricity usage, go here. Edit the suggested text below accordingly -->
|
144 |
-
|
145 |
-
Carbon emissions can be estimated using the [Machine Learning Impact calculator](https://mlco2.github.io/impact#compute) presented in [Lacoste et al. (2019)](https://arxiv.org/abs/1910.09700).
|
146 |
-
|
147 |
-
- **Hardware Type:** [More Information Needed]
|
148 |
-
- **Hours used:** [More Information Needed]
|
149 |
-
- **Cloud Provider:** [More Information Needed]
|
150 |
-
- **Compute Region:** [More Information Needed]
|
151 |
-
- **Carbon Emitted:** [More Information Needed]
|
152 |
|
153 |
-
## Technical Specifications [optional]
|
154 |
|
155 |
-
|
|
|
|
|
|
|
|
|
|
|
|
|
|
|
|
|
|
|
|
|
|
|
|
|
|
|
|
|
|
|
|
|
|
|
|
|
|
|
|
|
|
|
|
|
|
|
|
|
|
|
|
|
|
|
|
|
|
|
|
|
|
|
|
|
|
|
|
|
|
|
|
|
|
|
|
|
|
|
|
|
|
|
|
|
|
|
|
|
|
|
|
|
|
|
|
|
|
|
|
|
|
|
|
|
|
|
|
|
|
|
|
|
|
|
|
|
|
|
|
|
|
|
|
|
|
|
|
|
|
|
|
|
|
|
|
|
|
|
|
|
|
|
|
|
|
|
|
|
|
|
|
|
|
|
|
|
|
|
|
|
|
|
|
|
|
|
|
|
|
|
|
|
|
|
|
|
|
|
|
|
|
|
|
|
|
|
|
|
|
|
|
|
|
|
|
|
|
|
|
|
|
|
|
|
|
|
|
|
|
|
|
|
|
|
|
|
|
|
|
|
|
|
|
|
|
|
|
|
|
|
|
|
|
|
|
|
|
|
|
|
|
|
|
|
|
|
|
|
|
|
|
|
|
|
|
|
|
|
|
|
|
|
|
|
|
|
|
|
|
|
|
|
|
|
|
|
|
|
|
|
|
|
|
|
|
|
|
|
|
|
|
|
|
|
|
|
|
|
|
|
|
|
|
|
|
|
|
|
|
|
|
|
|
|
|
|
|
|
|
|
|
|
|
|
|
|
|
|
|
|
|
|
|
|
|
|
|
|
|
|
|
|
156 |
|
157 |
-
|
|
|
|
|
|
|
158 |
|
159 |
-
|
160 |
|
161 |
-
|
162 |
|
163 |
-
|
|
|
164 |
|
165 |
-
|
|
|
|
|
166 |
|
167 |
-
|
168 |
|
169 |
-
|
|
|
170 |
|
171 |
-
|
|
|
|
|
|
|
172 |
|
173 |
-
|
174 |
|
175 |
-
|
|
|
176 |
|
177 |
-
|
178 |
|
179 |
-
**
|
180 |
|
181 |
-
|
182 |
|
183 |
-
##
|
184 |
|
185 |
-
|
|
|
|
|
|
|
186 |
|
187 |
-
|
188 |
|
189 |
-
|
190 |
|
191 |
-
|
192 |
|
193 |
-
|
|
|
194 |
|
195 |
-
|
196 |
|
197 |
-
|
198 |
|
199 |
-
|
|
|
|
|
|
|
|
|
|
|
|
|
|
|
|
|
|
1 |
+
|
2 |
---
|
3 |
+
datasets:
|
4 |
+
- bigscience/xP3
|
5 |
+
- mc4
|
6 |
+
- Leipzig_corpora_collection
|
7 |
+
license: apache-2.0
|
8 |
+
language:
|
9 |
+
- en
|
10 |
+
- de
|
11 |
+
tags:
|
12 |
+
- text2text-generation
|
13 |
+
pipeline_tag: text2text-generation
|
14 |
---
|
15 |
|
|
|
|
|
|
|
|
|
|
|
|
|
|
|
|
|
|
|
|
|
|
|
|
|
|
|
|
|
|
|
|
|
|
|
|
|
|
|
|
|
|
|
|
|
|
|
|
|
|
|
|
|
|
|
|
|
|
|
|
|
|
|
|
|
|
|
|
|
|
|
|
|
|
|
|
|
|
|
|
|
|
|
|
|
|
|
|
|
|
|
|
|
|
|
|
|
|
|
|
|
|
|
|
|
|
|
|
|
|
|
|
|
|
|
|
|
|
|
|
|
|
|
|
|
|
|
|
|
|
|
|
|
|
|
|
|
|
|
|
|
|
|
|
|
|
|
|
|
|
|
|
|
|
|
|
|
|
|
|
|
|
|
|
|
|
|
|
|
|
|
|
|
|
|
|
|
|
|
|
|
|
|
|
|
|
|
|
|
|
|
|
|
|
|
|
|
|
|
|
|
|
|
|
|
|
|
|
|
|
|
|
|
|
|
|
|
|
|
|
|
|
|
|
|
|
|
|
|
|
|
|
|
|
|
|
|
|
|
|
|
|
|
|
|
|
|
|
|
|
|
|
|
|
|
|
|
|
|
|
|
|
|
|
|
|
|
|
|
|
|
|
|
|
|
|
|
|
|
|
|
|
|
|
|
|
|
|
|
|
|
|
|
16 |
|
|
|
17 |
|
18 |
+
# Language Adaption of mT0
|
19 |
+
This model is an adaption of the mT0 variant (see below) for languages en, de based on the methodology in [Load What You Need: Smaller Versions of Multilingual BERT](https://arxiv.org/ftp/arxiv/papers/2010/2010.05609.pdf).
|
20 |
+
|
21 |
+
The vocabulary has been adjusted to contain the top 2000 tokens overall as well as
|
22 |
+
- the top 15000 tokens from en
|
23 |
+
- the top 30000 tokens from de
|
24 |
+
and 100 special tokens.
|
25 |
+
|
26 |
+
The new vocabulary has been computed using
|
27 |
+
- deu_news_2023_1M for de
|
28 |
+
- eng_news_2023_1M for en
|
29 |
+
|
30 |
+
from the [Leipzig language corpora collection](https://wortschatz.uni-leipzig.de/en/download/).
|
31 |
+
|
32 |
+
According to the mentioned article the perfarmance degradation can be expected to be small (but no evaluation was done).
|
33 |
+
|
34 |
+
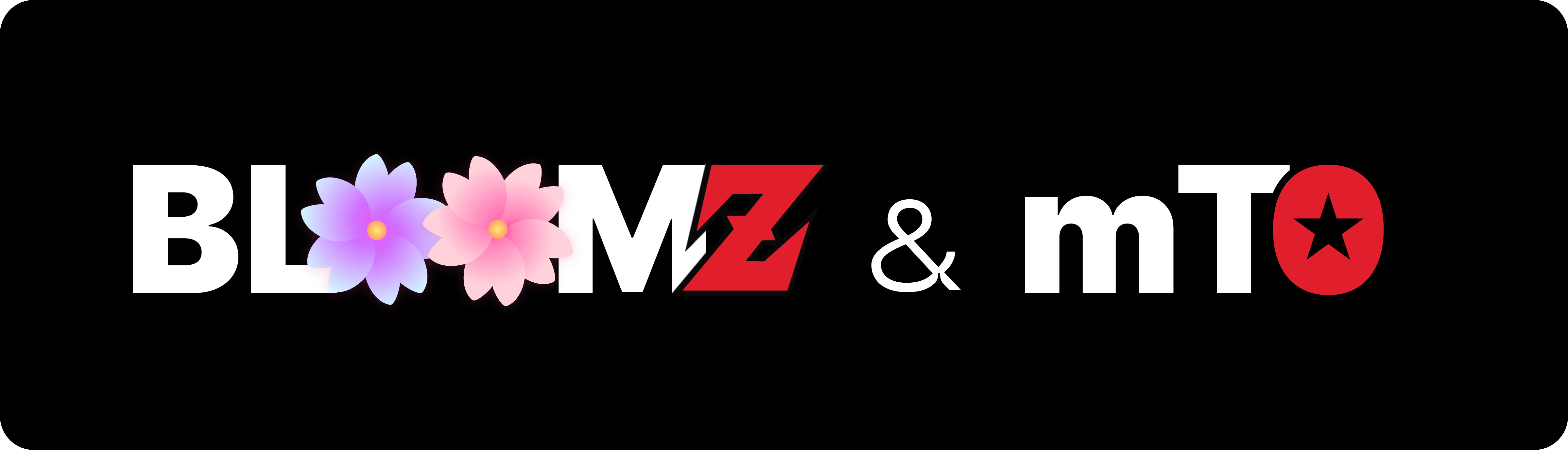
|
35 |
+
|
36 |
+
# Table of Contents
|
37 |
+
|
38 |
+
1. [Model Summary](#model-summary)
|
39 |
+
2. [Use](#use)
|
40 |
+
3. [Limitations](#limitations)
|
41 |
+
4. [Training](#training)
|
42 |
+
5. [Evaluation](#evaluation)
|
43 |
+
7. [Citation](#citation)
|
44 |
+
|
45 |
+
# Model Summary
|
46 |
+
|
47 |
+
> We present BLOOMZ & mT0, a family of models capable of following human instructions in dozens of languages zero-shot. We finetune BLOOM & mT5 pretrained multilingual language models on our crosslingual task mixture (xP3) and find our resulting models capable of crosslingual generalization to unseen tasks & languages.
|
48 |
+
|
49 |
+
- **Repository:** [bigscience-workshop/xmtf](https://github.com/bigscience-workshop/xmtf)
|
50 |
+
- **Paper:** [Crosslingual Generalization through Multitask Finetuning](https://arxiv.org/abs/2211.01786)
|
51 |
+
- **Point of Contact:** [Niklas Muennighoff](mailto:niklas@hf.co)
|
52 |
+
- **Languages:** Refer to [mc4](https://huggingface.co/datasets/mc4) for pretraining & [xP3](https://huggingface.co/bigscience/xP3) for finetuning language proportions. It understands both pretraining & finetuning languages.
|
53 |
+
- **BLOOMZ & mT0 Model Family:**
|
54 |
+
|
55 |
+
<div class="max-w-full overflow-auto">
|
56 |
+
<table>
|
57 |
+
<tr>
|
58 |
+
<th colspan="12">Multitask finetuned on <a style="font-weight:bold" href=https://huggingface.co/datasets/bigscience/xP3>xP3</a>. Recommended for prompting in English.
|
59 |
+
</tr>
|
60 |
+
<tr>
|
61 |
+
<td>Parameters</td>
|
62 |
+
<td>300M</td>
|
63 |
+
<td>580M</td>
|
64 |
+
<td>1.2B</td>
|
65 |
+
<td>3.7B</td>
|
66 |
+
<td>13B</td>
|
67 |
+
<td>560M</td>
|
68 |
+
<td>1.1B</td>
|
69 |
+
<td>1.7B</td>
|
70 |
+
<td>3B</td>
|
71 |
+
<td>7.1B</td>
|
72 |
+
<td>176B</td>
|
73 |
+
</tr>
|
74 |
+
<tr>
|
75 |
+
<td>Finetuned Model</td>
|
76 |
+
<td><a href=https://huggingface.co/bigscience/mt0-small>mt0-small</a></td>
|
77 |
+
<td><a href=https://huggingface.co/bigscience/mt0-base>mt0-base</a></td>
|
78 |
+
<td><a href=https://huggingface.co/bigscience/mt0-large>mt0-large</a></td>
|
79 |
+
<td><a href=https://huggingface.co/bigscience/mt0-xl>mt0-xl</a></td>
|
80 |
+
<td><a href=https://huggingface.co/bigscience/mt0-xxl>mt0-xxl</a></td>
|
81 |
+
<td><a href=https://huggingface.co/bigscience/bloomz-560m>bloomz-560m</a></td>
|
82 |
+
<td><a href=https://huggingface.co/bigscience/bloomz-1b1>bloomz-1b1</a></td>
|
83 |
+
<td><a href=https://huggingface.co/bigscience/bloomz-1b7>bloomz-1b7</a></td>
|
84 |
+
<td><a href=https://huggingface.co/bigscience/bloomz-3b>bloomz-3b</a></td>
|
85 |
+
<td><a href=https://huggingface.co/bigscience/bloomz-7b1>bloomz-7b1</a></td>
|
86 |
+
<td><a href=https://huggingface.co/bigscience/bloomz>bloomz</a></td>
|
87 |
+
</tr>
|
88 |
+
</tr>
|
89 |
+
<tr>
|
90 |
+
<th colspan="12">Multitask finetuned on <a style="font-weight:bold" href=https://huggingface.co/datasets/bigscience/xP3mt>xP3mt</a>. Recommended for prompting in non-English.</th>
|
91 |
+
</tr>
|
92 |
+
<tr>
|
93 |
+
<td>Finetuned Model</td>
|
94 |
+
<td></td>
|
95 |
+
<td></td>
|
96 |
+
<td></td>
|
97 |
+
<td></td>
|
98 |
+
<td><a href=https://huggingface.co/bigscience/mt0-xxl-mt>mt0-xxl-mt</a></td>
|
99 |
+
<td></td>
|
100 |
+
<td></td>
|
101 |
+
<td></td>
|
102 |
+
<td></td>
|
103 |
+
<td><a href=https://huggingface.co/bigscience/bloomz-7b1-mt>bloomz-7b1-mt</a></td>
|
104 |
+
<td><a href=https://huggingface.co/bigscience/bloomz-mt>bloomz-mt</a></td>
|
105 |
+
</tr>
|
106 |
+
<th colspan="12">Multitask finetuned on <a style="font-weight:bold" href=https://huggingface.co/datasets/Muennighoff/P3>P3</a>. Released for research purposes only. Strictly inferior to above models!</th>
|
107 |
+
</tr>
|
108 |
+
<tr>
|
109 |
+
<td>Finetuned Model</td>
|
110 |
+
<td></td>
|
111 |
+
<td></td>
|
112 |
+
<td></td>
|
113 |
+
<td></td>
|
114 |
+
<td><a href=https://huggingface.co/bigscience/mt0-xxl-p3>mt0-xxl-p3</a></td>
|
115 |
+
<td></td>
|
116 |
+
<td></td>
|
117 |
+
<td></td>
|
118 |
+
<td></td>
|
119 |
+
<td><a href=https://huggingface.co/bigscience/bloomz-7b1-p3>bloomz-7b1-p3</a></td>
|
120 |
+
<td><a href=https://huggingface.co/bigscience/bloomz-p3>bloomz-p3</a></td>
|
121 |
+
</tr>
|
122 |
+
<th colspan="12">Original pretrained checkpoints. Not recommended.</th>
|
123 |
+
<tr>
|
124 |
+
<td>Pretrained Model</td>
|
125 |
+
<td><a href=https://huggingface.co/google/mt5-small>mt5-small</a></td>
|
126 |
+
<td><a href=https://huggingface.co/google/mt5-base>mt5-base</a></td>
|
127 |
+
<td><a href=https://huggingface.co/google/mt5-large>mt5-large</a></td>
|
128 |
+
<td><a href=https://huggingface.co/google/mt5-xl>mt5-xl</a></td>
|
129 |
+
<td><a href=https://huggingface.co/google/mt5-xxl>mt5-xxl</a></td>
|
130 |
+
<td><a href=https://huggingface.co/bigscience/bloom-560m>bloom-560m</a></td>
|
131 |
+
<td><a href=https://huggingface.co/bigscience/bloom-1b1>bloom-1b1</a></td>
|
132 |
+
<td><a href=https://huggingface.co/bigscience/bloom-1b7>bloom-1b7</a></td>
|
133 |
+
<td><a href=https://huggingface.co/bigscience/bloom-3b>bloom-3b</a></td>
|
134 |
+
<td><a href=https://huggingface.co/bigscience/bloom-7b1>bloom-7b1</a></td>
|
135 |
+
<td><a href=https://huggingface.co/bigscience/bloom>bloom</a></td>
|
136 |
+
</tr>
|
137 |
+
</table>
|
138 |
+
</div>
|
139 |
+
|
140 |
+
|
141 |
+
|
142 |
+
# Use
|
143 |
+
|
144 |
+
## Intended use
|
145 |
+
|
146 |
+
We recommend using the model to perform tasks expressed in natural language. For example, given the prompt "*Translate to English: Je t’aime.*", the model will most likely answer "*I love you.*". Some prompt ideas from our paper:
|
147 |
+
- 一个传奇的开端,一个不灭的神话,这不仅仅是一部电影,而是作为一个走进新时代的标签,永远彪炳史册。你认为这句话的立场是赞扬、中立还是批评?
|
148 |
+
- Suggest at least five related search terms to "Mạng neural nhân tạo".
|
149 |
+
- Write a fairy tale about a troll saving a princess from a dangerous dragon. The fairy tale is a masterpiece that has achieved praise worldwide and its moral is "Heroes Come in All Shapes and Sizes". Story (in Spanish):
|
150 |
+
- Explain in a sentence in Telugu what is backpropagation in neural networks.
|
151 |
+
|
152 |
+
**Feel free to share your generations in the Community tab!**
|
153 |
+
|
154 |
+
## How to use
|
155 |
+
|
156 |
+
### CPU
|
157 |
+
|
158 |
+
<details>
|
159 |
+
<summary> Click to expand </summary>
|
160 |
+
|
161 |
+
```python
|
162 |
+
# pip install -q transformers
|
163 |
+
from transformers import AutoModelForSeq2SeqLM, AutoTokenizer
|
164 |
+
|
165 |
+
checkpoint = "bigscience/mt0-xl"
|
166 |
+
|
167 |
+
tokenizer = AutoTokenizer.from_pretrained(checkpoint)
|
168 |
+
model = AutoModelForSeq2SeqLM.from_pretrained(checkpoint)
|
169 |
+
|
170 |
+
inputs = tokenizer.encode("Translate to English: Je t’aime.", return_tensors="pt")
|
171 |
+
outputs = model.generate(inputs)
|
172 |
+
print(tokenizer.decode(outputs[0]))
|
173 |
+
```
|
174 |
+
|
175 |
+
</details>
|
176 |
+
|
177 |
+
### GPU
|
178 |
+
|
179 |
+
<details>
|
180 |
+
<summary> Click to expand </summary>
|
181 |
+
|
182 |
+
```python
|
183 |
+
# pip install -q transformers accelerate
|
184 |
+
from transformers import AutoModelForSeq2SeqLM, AutoTokenizer
|
185 |
+
|
186 |
+
checkpoint = "bigscience/mt0-xl"
|
187 |
+
|
188 |
+
tokenizer = AutoTokenizer.from_pretrained(checkpoint)
|
189 |
+
model = AutoModelForSeq2SeqLM.from_pretrained(checkpoint, torch_dtype="auto", device_map="auto")
|
190 |
|
191 |
+
inputs = tokenizer.encode("Translate to English: Je t’aime.", return_tensors="pt").to("cuda")
|
192 |
+
outputs = model.generate(inputs)
|
193 |
+
print(tokenizer.decode(outputs[0]))
|
194 |
+
```
|
195 |
|
196 |
+
</details>
|
197 |
|
198 |
+
### GPU in 8bit
|
199 |
|
200 |
+
<details>
|
201 |
+
<summary> Click to expand </summary>
|
202 |
|
203 |
+
```python
|
204 |
+
# pip install -q transformers accelerate bitsandbytes
|
205 |
+
from transformers import AutoModelForSeq2SeqLM, AutoTokenizer
|
206 |
|
207 |
+
checkpoint = "bigscience/mt0-xl"
|
208 |
|
209 |
+
tokenizer = AutoTokenizer.from_pretrained(checkpoint)
|
210 |
+
model = AutoModelForSeq2SeqLM.from_pretrained(checkpoint, device_map="auto", load_in_8bit=True)
|
211 |
|
212 |
+
inputs = tokenizer.encode("Translate to English: Je t’aime.", return_tensors="pt").to("cuda")
|
213 |
+
outputs = model.generate(inputs)
|
214 |
+
print(tokenizer.decode(outputs[0]))
|
215 |
+
```
|
216 |
|
217 |
+
</details>
|
218 |
|
219 |
+
<!-- Necessary for whitespace -->
|
220 |
+
###
|
221 |
|
222 |
+
# Limitations
|
223 |
|
224 |
+
**Prompt Engineering:** The performance may vary depending on the prompt. For BLOOMZ models, we recommend making it very clear when the input stops to avoid the model trying to continue it. For example, the prompt "*Translate to English: Je t'aime*" without the full stop (.) at the end, may result in the model trying to continue the French sentence. Better prompts are e.g. "*Translate to English: Je t'aime.*", "*Translate to English: Je t'aime. Translation:*" "*What is "Je t'aime." in English?*", where it is clear for the model when it should answer. Further, we recommend providing the model as much context as possible. For example, if you want it to answer in Telugu, then tell the model, e.g. "*Explain in a sentence in Telugu what is backpropagation in neural networks.*".
|
225 |
|
226 |
+
# Training
|
227 |
|
228 |
+
## Model
|
229 |
|
230 |
+
- **Architecture:** Same as [mt5-xl](https://huggingface.co/google/mt5-xl), also refer to the `config.json` file
|
231 |
+
- **Finetuning steps:** 10000
|
232 |
+
- **Finetuning tokens:** 1.85 billion
|
233 |
+
- **Precision:** bfloat16
|
234 |
|
235 |
+
## Hardware
|
236 |
|
237 |
+
- **TPUs:** TPUv4-128
|
238 |
|
239 |
+
## Software
|
240 |
|
241 |
+
- **Orchestration:** [T5X](https://github.com/google-research/t5x)
|
242 |
+
- **Neural networks:** [Jax](https://github.com/google/jax)
|
243 |
|
244 |
+
# Evaluation
|
245 |
|
246 |
+
We refer to Table 7 from our [paper](https://arxiv.org/abs/2211.01786) & [bigscience/evaluation-results](https://huggingface.co/datasets/bigscience/evaluation-results) for zero-shot results on unseen tasks. The sidebar reports zero-shot performance of the best prompt per dataset config.
|
247 |
|
248 |
+
# Citation
|
249 |
+
```bibtex
|
250 |
+
@article{muennighoff2022crosslingual,
|
251 |
+
title={Crosslingual generalization through multitask finetuning},
|
252 |
+
author={Muennighoff, Niklas and Wang, Thomas and Sutawika, Lintang and Roberts, Adam and Biderman, Stella and Scao, Teven Le and Bari, M Saiful and Shen, Sheng and Yong, Zheng-Xin and Schoelkopf, Hailey and others},
|
253 |
+
journal={arXiv preprint arXiv:2211.01786},
|
254 |
+
year={2022}
|
255 |
+
}
|
256 |
+
```
|