---
base_model: HuggingFaceM4/idefics2-8b
datasets:
- TIGER-Lab/Mantis-Instruct
language:
- en
license: apache-2.0
tags:
- multimodal
- lmm
- vlm
- llava
- siglip
- llama3
- mantis
model-index:
- name: mantis-8b-idefics2_8192
results: []
---
# 🔥 Mantis (TMLR 2024)
[Paper](https://arxiv.org/abs/2405.01483) |
[Website](https://tiger-ai-lab.github.io/Mantis/) |
[Github](https://github.com/TIGER-AI-Lab/Mantis) |
[Models](https://huggingface.co/collections/TIGER-Lab/mantis-6619b0834594c878cdb1d6e4) |
[Demo](https://huggingface.co/spaces/TIGER-Lab/Mantis) |
[Wandb](https://api.wandb.ai/links/dongfu/lnkrl3af)
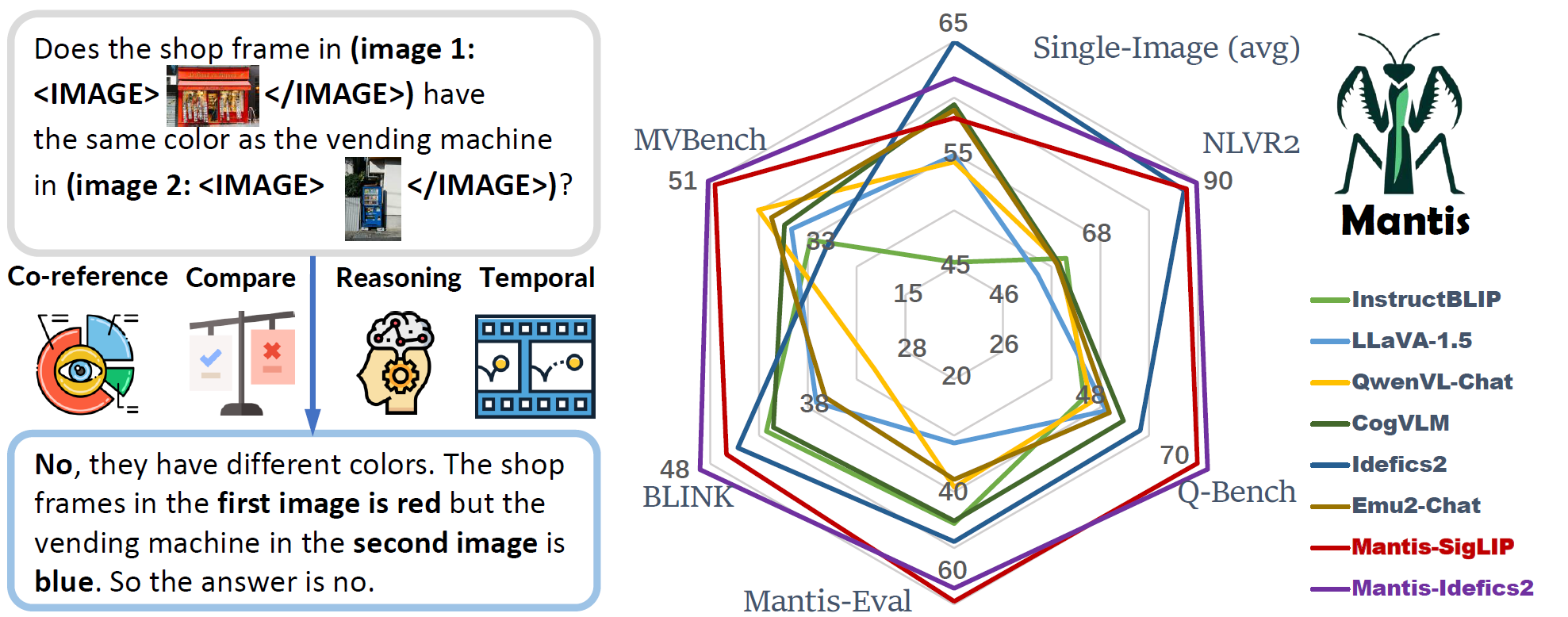
**Excited to announce Mantis-Idefics2, with enhanced ability in multi-image scenarios!**
It's fine-tuned on [Mantis-Instruct](https://huggingface.co/datasets/TIGER-Lab/Mantis-Instruct) from [Idefics2-8b](https://huggingface.co/HuggingFaceM4/idefics2-8b)
## Summary
- Mantis-Idefics2 is an LMM with **interleaved text and image as inputs**, trained on Mantis-Instruct under academic-level resources (i.e. 36 hours on 16xA100-40G).
- Mantis is trained to have multi-image skills including co-reference, reasoning, comparing, temporal understanding.
- Mantis reaches the state-of-the-art performance on five multi-image benchmarks (NLVR2, Q-Bench, BLINK, MVBench, Mantis-Eval), and also maintain a strong single-image performance on par with CogVLM and Emu2.
## Multi-Image Performance
| Models | Size | Format | NLVR2 | Q-Bench | Mantis-Eval | BLINK | MVBench | Avg |
|--------------------|:----:|:--------:|:-----:|:-------:|:-----------:|:-----:|:-------:|:----:|
| GPT-4V | - | sequence | 88.80 | 76.52 | 62.67 | 51.14 | 43.50 | 64.5 |
| Open Source Models | | | | | | | | |
| Random | - | - | 48.93 | 40.20 | 23.04 | 38.09 | 27.30 | 35.5 |
| Kosmos2 | 1.6B | merge | 49.00 | 35.10 | 30.41 | 37.50 | 21.62 | 34.7 |
| LLaVA-v1.5 | 7B | merge | 53.88 | 49.32 | 31.34 | 37.13 | 36.00 | 41.5 |
| LLava-V1.6 | 7B | merge | 58.88 | 54.80 | 45.62 | 39.55 | 40.90 | 48.0 |
| Qwen-VL-Chat | 7B | merge | 58.72 | 45.90 | 39.17 | 31.17 | 42.15 | 43.4 |
| Fuyu | 8B | merge | 51.10 | 49.15 | 27.19 | 36.59 | 30.20 | 38.8 |
| BLIP-2 | 13B | merge | 59.42 | 51.20 | 49.77 | 39.45 | 31.40 | 46.2 |
| InstructBLIP | 13B | merge | 60.26 | 44.30 | 45.62 | 42.24 | 32.50 | 45.0 |
| CogVLM | 17B | merge | 58.58 | 53.20 | 45.16 | 41.54 | 37.30 | 47.2 |
| OpenFlamingo | 9B | sequence | 36.41 | 19.60 | 12.44 | 39.18 | 7.90 | 23.1 |
| Otter-Image | 9B | sequence | 49.15 | 17.50 | 14.29 | 36.26 | 15.30 | 26.5 |
| Idefics1 | 9B | sequence | 54.63 | 30.60 | 28.11 | 24.69 | 26.42 | 32.9 |
| VideoLLaVA | 7B | sequence | 56.48 | 45.70 | 35.94 | 38.92 | 44.30 | 44.3 |
| Emu2-Chat | 37B | sequence | 58.16 | 50.05 | 37.79 | 36.20 | 39.72 | 44.4 |
| Vila | 8B | sequence | 76.45 | 45.70 | 51.15 | 39.30 | 49.40 | 52.4 |
| Idefics2 | 8B | sequence | 86.87 | 57.00 | 48.85 | 45.18 | 29.68 | 53.5 |
| Mantis-CLIP | 8B | sequence | 84.66 | 66.00 | 55.76 | 47.06 | 48.30 | 60.4 |
| Mantis-SIGLIP | 8B | sequence | 87.43 | 69.90 | **59.45** | 46.35 | 50.15 | 62.7 |
| Mantis-Flamingo | 9B | sequence | 52.96 | 46.80 | 32.72 | 38.00 | 40.83 | 42.3 |
| Mantis-Idefics2 | 8B | sequence | **89.71** | **75.20** | 57.14 | **49.05** | **51.38** | **64.5** |
| $\Delta$ over SOTA | - | - | +2.84 | +18.20 | +8.30 | +3.87 | +1.98 | +11.0 |
## Single-Image Performance
| Model | Size | TextVQA | VQA | MMB | MMMU | OKVQA | SQA | MathVista | Avg |
|-----------------|:----:|:-------:|:----:|:----:|:----:|:-----:|:----:|:---------:|:----:|
| OpenFlamingo | 9B | 46.3 | 58.0 | 32.4 | 28.7 | 51.4 | 45.7 | 18.6 | 40.2 |
| Idefics1 | 9B | 39.3 | 68.8 | 45.3 | 32.5 | 50.4 | 51.6 | 21.1 | 44.1 |
| InstructBLIP | 7B | 33.6 | 75.2 | 38.3 | 30.6 | 45.2 | 70.6 | 24.4 | 45.4 |
| Yi-VL | 6B | 44.8 | 72.5 | 68.4 | 39.1 | 51.3 | 71.7 | 29.7 | 53.9 |
| Qwen-VL-Chat | 7B | 63.8 | 78.2 | 61.8 | 35.9 | 56.6 | 68.2 | 15.5 | 54.3 |
| LLaVA-1.5 | 7B | 58.2 | 76.6 | 64.8 | 35.3 | 53.4 | 70.4 | 25.6 | 54.9 |
| Emu2-Chat | 37B | 66.6 | **84.9** | 63.6 | 36.3 | **64.8** | 65.3 | 30.7 | 58.9 |
| CogVLM | 17B | **70.4** | 82.3 | 65.8 | 32.1 | 64.8 | 65.6 | 35.0 | 59.4 |
| Idefics2 | 8B | 70.4 | 79.1 | 75.7 | **43.0** | 53.5 | **86.5** | **51.4** | **65.7** |
| Mantis-CLIP | 8B | 56.4 | 73.0 | 66.0 | 38.1 | 53.0 | 73.8 | 31.7 | 56.0 |
| Mantis-SigLIP | 8B | 59.2 | 74.9 | 68.7 | 40.1 | 55.4 | 74.9 | 34.4 | 58.2 |
| Mantis-Idefics2 | 8B | 63.5 | 77.6 | 75.7 | 41.1 | 52.6 | 81.3 | 40.4 | 61.7 |
## How to use
### Run example inference:
```python
import requests
import torch
from PIL import Image
from io import BytesIO
from transformers import AutoProcessor, AutoModelForVision2Seq
from transformers.image_utils import load_image
processor = AutoProcessor.from_pretrained("TIGER-Lab/Mantis-8B-Idefics2") # do_image_splitting is False by default
model = AutoModelForVision2Seq.from_pretrained(
"TIGER-Lab/Mantis-8B-Idefics2",
device_map="auto"
)
generation_kwargs = {
"max_new_tokens": 1024,
"num_beams": 1,
"do_sample": False
}
# Note that passing the image urls (instead of the actual pil images) to the processor is also possible
image1 = load_image("https://cdn.britannica.com/59/94459-050-DBA42467/Skyline-Chicago.jpg")
image2 = load_image("https://cdn.britannica.com/61/93061-050-99147DCE/Statue-of-Liberty-Island-New-York-Bay.jpg")
image3 = load_image("https://cdn.britannica.com/68/170868-050-8DDE8263/Golden-Gate-Bridge-San-Francisco.jpg")
images = [image1, image2, image3]
query1 = "What cities image 1, image 2, and image 3 belong to respectively? Answer me in order."
query2 = "Which one do you recommend for a visit? and why?"
query3 = "Which picture has most cars in it?"
### Chat
### Round 1
messages = [
{
"role": "user",
"content": [
{"type": "image"},
{"type": "image"},
{"type": "image"},
{"type": "text", "text": query1},
]
}
]
prompt = processor.apply_chat_template(messages, add_generation_prompt=True)
inputs = processor(text=prompt, images=images, return_tensors="pt")
inputs = {k: v.to(model.device) for k, v in inputs.items()}
# Generate
generated_ids = model.generate(**inputs, **generation_kwargs)
response = processor.batch_decode(generated_ids[:, inputs["input_ids"].shape[1]:], skip_special_tokens=True)
print("User: ", query1)
print("ASSISTANT: ", response[0])
### Round 2
messages.append(
{
"role": "assistant",
"content": [
{"type": "text", "text": response[0]},
]
}
)
messages.append(
{
"role": "user",
"content": [
{"type": "text", "text": query2},
]
}
)
prompt = processor.apply_chat_template(messages, add_generation_prompt=True)
inputs = processor(text=prompt, images=images, return_tensors="pt")
inputs = {k: v.to(model.device) for k, v in inputs.items()}
generated_ids = model.generate(**inputs, **generation_kwargs)
response = processor.batch_decode(generated_ids[:, inputs["input_ids"].shape[1]:], skip_special_tokens=True)
print("User: ", query2)
print("ASSISTANT: ", response[0])
### Round 3
messages.append(
{
"role": "assistant",
"content": [
{"type": "text", "text": response[0]},
]
}
)
messages.append(
{
"role": "user",
"content": [
{"type": "text", "text": query3},
]
}
)
prompt = processor.apply_chat_template(messages, add_generation_prompt=True)
inputs = processor(text=prompt, images=images, return_tensors="pt")
inputs = {k: v.to(model.device) for k, v in inputs.items()}
generated_ids = model.generate(**inputs, **generation_kwargs)
response = processor.batch_decode(generated_ids[:, inputs["input_ids"].shape[1]:], skip_special_tokens=True)
print("User: ", query3)
print("ASSISTANT: ", response[0])
"""
User: What cities image 1, image 2, and image 3 belong to respectively? Answer me in order.
ASSISTANT: Chicago, New York, San Francisco
User: Which one do you recommend for a visit? and why?
ASSISTANT: New York - because it's a bustling metropolis with iconic landmarks like the Statue of Liberty and the Empire State Building.
User: Which picture has most cars in it?
ASSISTANT: Image 3
"""
```
### Training
See [mantis/train](https://github.com/TIGER-AI-Lab/Mantis/tree/main/mantis/train) for details
### Evaluation
See [mantis/benchmark](https://github.com/TIGER-AI-Lab/Mantis/tree/main/mantis/benchmark) for details
**Please cite our paper or give a star to out Github repo if you find this model useful**
## Citation
```
@article{Jiang2024MANTISIM,
title={MANTIS: Interleaved Multi-Image Instruction Tuning},
author={Dongfu Jiang and Xuan He and Huaye Zeng and Cong Wei and Max W.F. Ku and Qian Liu and Wenhu Chen},
journal={Trans. Mach. Learn. Res.},
year={2024},
volume={2024},
url={Transactions on Machine Learning Research}
}
```