Update README.md
Browse files
README.md
CHANGED
@@ -3,197 +3,167 @@ library_name: transformers
|
|
3 |
tags: []
|
4 |
---
|
5 |
|
6 |
-
|
7 |
-
|
8 |
-
|
9 |
-
|
10 |
-
|
11 |
-
|
12 |
-
|
13 |
-
|
14 |
-
|
15 |
-
|
16 |
-
|
17 |
-
|
18 |
-
|
19 |
-
|
20 |
-
- **
|
21 |
-
|
22 |
-
|
23 |
-
|
24 |
-
-
|
25 |
-
|
26 |
-
|
27 |
-
|
28 |
-
|
29 |
-
|
30 |
-
|
31 |
-
|
32 |
-
|
33 |
-
-
|
34 |
-
|
35 |
-
|
36 |
-
|
37 |
-
|
38 |
-
|
39 |
-
|
40 |
-
|
41 |
-
|
42 |
-
|
43 |
-
|
44 |
-
|
45 |
-
|
46 |
-
###
|
47 |
-
|
48 |
-
|
49 |
-
|
50 |
-
|
51 |
-
|
52 |
-
|
53 |
-
|
54 |
-
|
55 |
-
|
56 |
-
|
57 |
-
|
58 |
-
|
59 |
-
|
60 |
-
|
61 |
-
|
62 |
-
|
63 |
-
|
64 |
-
|
65 |
-
|
66 |
-
|
67 |
-
|
68 |
-
|
69 |
-
|
70 |
-
|
71 |
-
|
72 |
-
|
73 |
-
|
74 |
-
|
75 |
-
|
76 |
-
|
77 |
-
|
78 |
-
|
79 |
-
|
80 |
-
|
81 |
-
|
82 |
-
|
83 |
-
|
84 |
-
|
85 |
-
|
86 |
-
|
87 |
-
|
88 |
-
|
89 |
-
|
90 |
-
|
91 |
-
|
92 |
-
|
93 |
-
|
94 |
-
|
95 |
-
|
96 |
-
|
97 |
-
|
98 |
-
|
99 |
-
|
100 |
-
|
101 |
-
|
102 |
-
|
103 |
-
|
104 |
-
|
105 |
-
|
106 |
-
|
107 |
-
|
108 |
-
|
109 |
-
|
110 |
-
|
111 |
-
|
112 |
-
|
113 |
-
|
114 |
-
|
115 |
-
|
116 |
-
|
117 |
-
|
118 |
-
|
119 |
-
|
120 |
-
|
121 |
-
|
122 |
-
|
123 |
-
|
124 |
-
|
125 |
-
|
126 |
-
|
127 |
-
|
128 |
-
|
129 |
-
|
130 |
-
|
131 |
-
|
132 |
-
|
133 |
-
|
134 |
-
|
135 |
-
|
136 |
-
|
137 |
-
|
138 |
-
|
139 |
-
|
140 |
-
|
141 |
-
|
142 |
-
|
143 |
-
|
144 |
-
|
145 |
-
|
146 |
-
|
147 |
-
-
|
148 |
-
|
149 |
-
|
150 |
-
|
151 |
-
|
152 |
-
|
153 |
-
|
154 |
-
|
155 |
-
|
156 |
-
|
157 |
-
[
|
158 |
-
|
159 |
-
|
160 |
-
|
161 |
-
|
162 |
-
|
163 |
-
|
164 |
-
|
165 |
-
|
166 |
-
|
167 |
-
|
168 |
-
|
169 |
-
|
170 |
-
|
171 |
-
## Citation [optional]
|
172 |
-
|
173 |
-
<!-- If there is a paper or blog post introducing the model, the APA and Bibtex information for that should go in this section. -->
|
174 |
-
|
175 |
-
**BibTeX:**
|
176 |
-
|
177 |
-
[More Information Needed]
|
178 |
-
|
179 |
-
**APA:**
|
180 |
-
|
181 |
-
[More Information Needed]
|
182 |
-
|
183 |
-
## Glossary [optional]
|
184 |
-
|
185 |
-
<!-- If relevant, include terms and calculations in this section that can help readers understand the model or model card. -->
|
186 |
-
|
187 |
-
[More Information Needed]
|
188 |
-
|
189 |
-
## More Information [optional]
|
190 |
-
|
191 |
-
[More Information Needed]
|
192 |
-
|
193 |
-
## Model Card Authors [optional]
|
194 |
-
|
195 |
-
[More Information Needed]
|
196 |
-
|
197 |
-
## Model Card Contact
|
198 |
-
|
199 |
-
[More Information Needed]
|
|
|
3 |
tags: []
|
4 |
---
|
5 |
|
6 |
+
[📃Paper](https://arxiv.org/abs/2406.15252) | [🌐Website](https://tiger-ai-lab.github.io/VideoScore/) | [💻Github](https://github.com/TIGER-AI-Lab/VideoScore) | [🛢️Datasets](https://huggingface.co/datasets/TIGER-Lab/VideoFeedback) | [🤗Model](https://huggingface.co/TIGER-Lab/VideoScore) | [🤗Demo](https://huggingface.co/spaces/TIGER-Lab/VideoScore) | [📉Wandb](https://api.wandb.ai/links/xuanhe/ptohlfcx)
|
7 |
+
|
8 |
+
|
9 |
+
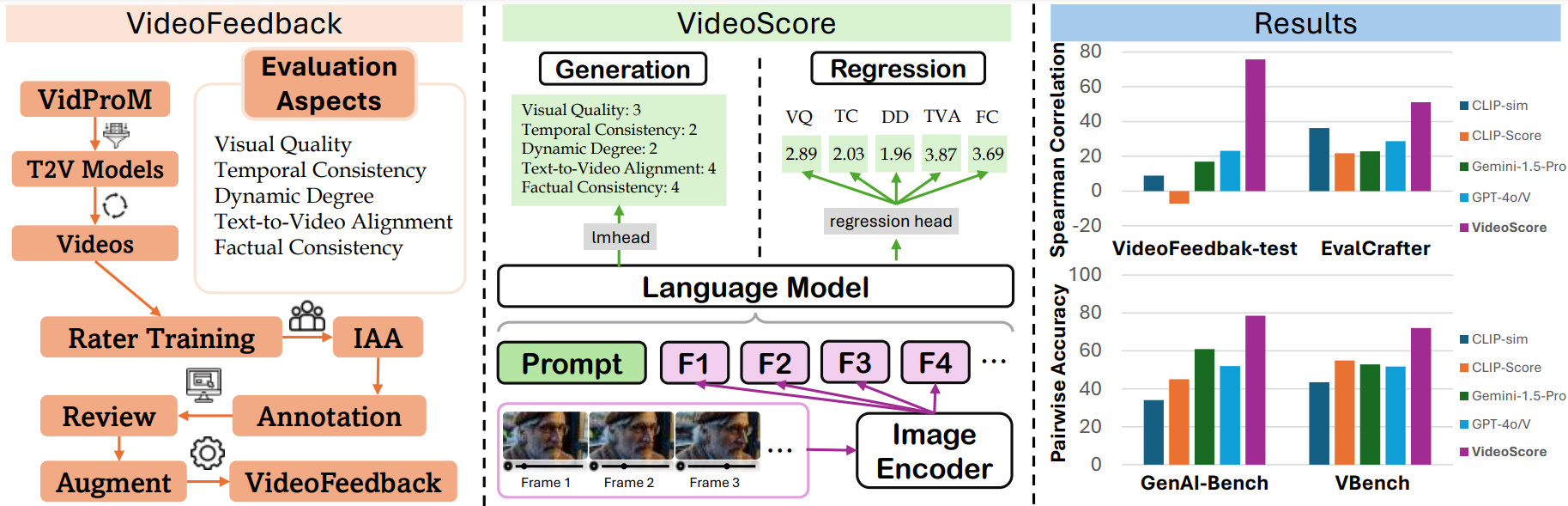
|
10 |
+
|
11 |
+
## Introduction
|
12 |
+
- VideoScore is a video quality evaluation model, taking [Mantis-8B-Idefics2](https://huggingface.co/TIGER-Lab/Mantis-8B-Idefics2) as base-model
|
13 |
+
and trained on [VideoFeedback](https://huggingface.co/datasets/TIGER-Lab/VideoFeedback),
|
14 |
+
a large video evaluation dataset with multi-aspect human scores.
|
15 |
+
|
16 |
+
- VideoScore can reach 75+ Spearman correlation with humans on VideoEval-test, surpassing all the MLLM-prompting methods and feature-based metrics.
|
17 |
+
|
18 |
+
- VideoScore also beat the best baselines on other three benchmarks EvalCrafter, GenAI-Bench and VBench, showing high alignment with human evaluations.
|
19 |
+
|
20 |
+
- **This is the regression version of VideoScore**
|
21 |
+
|
22 |
+
## Evaluation Results
|
23 |
+
|
24 |
+
We test our video evaluation model VideoScore on VideoEval-test, EvalCrafter, GenAI-Bench and VBench.
|
25 |
+
For the first two benchmarks, we take Spearman corrleation between model's output and human ratings
|
26 |
+
averaged among all the evaluation aspects as indicator.
|
27 |
+
For GenAI-Bench and VBench, which include human preference data among two or more videos,
|
28 |
+
we employ the model's output to predict preferences and use pairwise accuracy as the performance indicator.
|
29 |
+
|
30 |
+
- We use [VideoScore](https://huggingface.co/TIGER-Lab/VideoScore) trained on the entire VideoFeedback dataset
|
31 |
+
for VideoFeedback-test set, while for other three benchmarks.
|
32 |
+
|
33 |
+
- We use [VideoScore-anno-only](https://huggingface.co/TIGER-Lab/VideoScore-anno-only) trained on VideoFeedback dataset
|
34 |
+
excluding the real videos.
|
35 |
+
|
36 |
+
The evaluation results are coming soon
|
37 |
+
|
38 |
+
## Usage
|
39 |
+
### Installation
|
40 |
+
```
|
41 |
+
pip install git+https://github.com/TIGER-AI-Lab/VideoScore.git
|
42 |
+
# or
|
43 |
+
# pip install mantis-vl
|
44 |
+
```
|
45 |
+
|
46 |
+
### Inference
|
47 |
+
```
|
48 |
+
cd VideoScore/examples
|
49 |
+
```
|
50 |
+
|
51 |
+
```python
|
52 |
+
"""
|
53 |
+
pip install qwen_vl_utils mantis-vl
|
54 |
+
"""
|
55 |
+
import torch
|
56 |
+
from mantis.models.qwen2_vl import Qwen2VLForSequenceClassification
|
57 |
+
from transformers import Qwen2VLProcessor
|
58 |
+
from qwen_vl_utils import process_vision_info
|
59 |
+
|
60 |
+
MAX_NUM_FRAMES=16
|
61 |
+
ROUND_DIGIT=3
|
62 |
+
REGRESSION_QUERY_PROMPT = """
|
63 |
+
Suppose you are an expert in judging and evaluating the quality of AI-generated videos,
|
64 |
+
please watch the following frames of a given video and see the text prompt for generating the video,
|
65 |
+
then give scores from 5 different dimensions:
|
66 |
+
(1) visual quality: the quality of the video in terms of clearness, resolution, brightness, and color
|
67 |
+
(2) temporal consistency, both the consistency of objects or humans and the smoothness of motion or movements
|
68 |
+
(3) dynamic degree, the degree of dynamic changes
|
69 |
+
(4) text-to-video alignment, the alignment between the text prompt and the video content
|
70 |
+
(5) factual consistency, the consistency of the video content with the common-sense and factual knowledge
|
71 |
+
|
72 |
+
for each dimension, output a float number from 1.0 to 4.0,
|
73 |
+
the higher the number is, the better the video performs in that sub-score,
|
74 |
+
the lowest 1.0 means Bad, the highest 4.0 means Perfect/Real (the video is like a real video)
|
75 |
+
Here is an output example:
|
76 |
+
visual quality: 3.2
|
77 |
+
temporal consistency: 2.7
|
78 |
+
dynamic degree: 4.0
|
79 |
+
text-to-video alignment: 2.3
|
80 |
+
factual consistency: 1.8
|
81 |
+
|
82 |
+
For this video, the text prompt is "{text_prompt}",
|
83 |
+
all the frames of video are as follows:
|
84 |
+
"""
|
85 |
+
|
86 |
+
model_name="Mantis-VL/qwen2-vl-video-eval-debug_12288_regression"
|
87 |
+
video_path="video1.mp4"
|
88 |
+
video_prompt="Near the Elephant Gate village, they approach the haunted house at night. Rajiv feels anxious, but Bhavesh encourages him. As they reach the house, a mysterious sound in the air adds to the suspense."
|
89 |
+
|
90 |
+
# default: Load the model on the available device(s)
|
91 |
+
model = Qwen2VLForSequenceClassification.from_pretrained(
|
92 |
+
model_name, torch_dtype="auto", device_map="auto", attn_implementation="flash_attention_2"
|
93 |
+
)
|
94 |
+
|
95 |
+
# default processer
|
96 |
+
processor = Qwen2VLProcessor.from_pretrained(model_name)
|
97 |
+
|
98 |
+
|
99 |
+
model.push_to_hub("TIGER-Lab/VideoScore-Qwen2-VL")
|
100 |
+
processor.push_to_hub("TIGER-Lab/VideoScore-Qwen2-VL")
|
101 |
+
exit(1)
|
102 |
+
|
103 |
+
# Messages containing a images list as a video and a text query
|
104 |
+
messages = [
|
105 |
+
{
|
106 |
+
"role": "user",
|
107 |
+
"content": [
|
108 |
+
{
|
109 |
+
"type": "video",
|
110 |
+
"video": video_path,
|
111 |
+
"fps": 8.0,
|
112 |
+
},
|
113 |
+
{"type": "text", "text": REGRESSION_QUERY_PROMPT.format(text_prompt=video_prompt)},
|
114 |
+
],
|
115 |
+
}
|
116 |
+
]
|
117 |
+
|
118 |
+
# Preparation for inference
|
119 |
+
text = processor.apply_chat_template(
|
120 |
+
messages, tokenize=False, add_generation_prompt=True
|
121 |
+
)
|
122 |
+
image_inputs, video_inputs = process_vision_info(messages)
|
123 |
+
inputs = processor(
|
124 |
+
text=[text],
|
125 |
+
images=image_inputs,
|
126 |
+
videos=video_inputs,
|
127 |
+
padding=True,
|
128 |
+
return_tensors="pt",
|
129 |
+
)
|
130 |
+
inputs = inputs.to("cuda")
|
131 |
+
print(inputs['input_ids'].shape)
|
132 |
+
|
133 |
+
# Inference
|
134 |
+
with torch.no_grad():
|
135 |
+
outputs = model(**inputs)
|
136 |
+
|
137 |
+
logits = outputs.logits
|
138 |
+
num_aspects = logits.shape[-1]
|
139 |
+
|
140 |
+
aspect_scores = []
|
141 |
+
for i in range(num_aspects):
|
142 |
+
aspect_scores.append(round(logits[0, i].item(),ROUND_DIGIT))
|
143 |
+
print(aspect_scores)
|
144 |
+
|
145 |
+
"""
|
146 |
+
model output on visual quality, temporal consistency, dynamic degree,
|
147 |
+
text-to-video alignment, factual consistency, respectively
|
148 |
+
|
149 |
+
[3.578, 3.594, 3.703, 3.156, 3.688]
|
150 |
+
"""
|
151 |
+
```
|
152 |
+
|
153 |
+
### Training
|
154 |
+
see [VideoScore/training](https://github.com/TIGER-AI-Lab/VideoScore/tree/main/training) for details
|
155 |
+
|
156 |
+
### Evaluation
|
157 |
+
see [VideoScore/benchmark](https://github.com/TIGER-AI-Lab/VideoScore/tree/main/benchmark) for details
|
158 |
+
|
159 |
+
## Citation
|
160 |
+
```bibtex
|
161 |
+
@article{he2024videoscore,
|
162 |
+
title = {VideoScore: Building Automatic Metrics to Simulate Fine-grained Human Feedback for Video Generation},
|
163 |
+
author = {He, Xuan and Jiang, Dongfu and Zhang, Ge and Ku, Max and Soni, Achint and Siu, Sherman and Chen, Haonan and Chandra, Abhranil and Jiang, Ziyan and Arulraj, Aaran and Wang, Kai and Do, Quy Duc and Ni, Yuansheng and Lyu, Bohan and Narsupalli, Yaswanth and Fan, Rongqi and Lyu, Zhiheng and Lin, Yuchen and Chen, Wenhu},
|
164 |
+
journal = {ArXiv},
|
165 |
+
year = {2024},
|
166 |
+
volume={abs/2406.15252},
|
167 |
+
url = {https://arxiv.org/abs/2406.15252},
|
168 |
+
}
|
169 |
+
```
|
|
|
|
|
|
|
|
|
|
|
|
|
|
|
|
|
|
|
|
|
|
|
|
|
|
|
|
|
|
|
|
|
|
|
|
|
|
|
|
|
|
|
|
|
|
|
|
|
|
|
|
|
|
|
|
|
|
|
|