File size: 8,888 Bytes
af4ba34 88e7d54 af4ba34 88e7d54 af4ba34 88e7d54 af4ba34 d7cb187 88e7d54 d7cb187 88e7d54 af4ba34 d7cb187 af4ba34 88e7d54 af4ba34 88e7d54 af4ba34 d7cb187 88e7d54 af4ba34 88e7d54 af4ba34 88e7d54 af4ba34 d7cb187 af4ba34 88e7d54 af4ba34 88e7d54 af4ba34 88e7d54 af4ba34 88e7d54 af4ba34 88e7d54 af4ba34 88e7d54 af4ba34 88e7d54 d7cb187 |
1 2 3 4 5 6 7 8 9 10 11 12 13 14 15 16 17 18 19 20 21 22 23 24 25 26 27 28 29 30 31 32 33 34 35 36 37 38 39 40 41 42 43 44 45 46 47 48 49 50 51 52 53 54 55 56 57 58 59 60 61 62 63 64 65 66 67 68 69 70 71 72 73 74 75 76 77 78 79 80 81 82 83 84 85 86 87 88 89 90 91 92 93 94 95 96 97 98 99 100 101 102 103 104 105 106 107 108 109 110 111 112 113 114 115 116 117 118 119 120 121 122 123 124 125 126 127 128 129 130 131 132 133 134 135 136 137 138 139 140 141 142 143 144 145 146 147 148 149 150 151 152 153 154 155 156 157 158 159 |
---
language:
- en
- zh
tags:
- GENIUS
- conditional text generation
- sketch-based text generation
- data augmentation
license: apache-2.0
datasets:
- c4
- beyond/chinese_clean_passages_80m
widget:
- text: "<mask> Conference on Empirical Methods <mask> submission of research papers <mask> Deep Learning <mask>"
example_title: "Example 1"
- text: "<mask> machine learning <mask> my research interest <mask> data science <mask>"
example_title: "Example 2"
- text: "<mask> play basketball <mask> a strong team <mask> Shanghai University of Finance and Economics <mask> last Sunday <mask>"
example_title: "Example 3"
- text: "Good news: <mask> the European Union <mask> month by EU <mask> Farm Commissioner Franz <mask>"
example_title: "Example with a prompt 1"
- text: "Bad news: <mask> the European Union <mask> month by EU <mask> Farm Commissioner Franz <mask>"
example_title: "Example with a prompt 2"
inference:
parameters:
max_length: 200
num_beams: 3
do_sample: True
---
# 💡GENIUS – generating text using sketches!
**基于草稿的文本生成模型**
- **Paper: [GENIUS: Sketch-based Language Model Pre-training via Extreme and Selective Masking for Text Generation and Augmentation](https://github.com/beyondguo/genius/blob/master/GENIUS_gby_arxiv.pdf)**
💡**GENIUS** is a powerful conditional text generation model using sketches as input, which can fill in the missing contexts for a given **sketch** (key information consisting of textual spans, phrases, or words, concatenated by mask tokens). GENIUS is pre-trained on a large- scale textual corpus with a novel *reconstruction from sketch* objective using an *extreme and selective masking* strategy, enabling it to generate diverse and high-quality texts given sketches.
**Example 1:**
- sketch: `__ machine learning __ my research interest __ data science __`
- **GENIUS**: `I am a Ph.D. student in machine learning, and my research interest is in data science. I am interested in understanding how humans and machines interact and how we can improve the quality of life for people around the world.`
**Example 2:**
- sketch: `自然语言处理__谷歌__通用人工智能__`
- **GENIUS**: `自然语言处理是谷歌在通用人工智能领域的一个重要研究方向,其目的是为了促进人类智能的发展。 `
**GENIUS** can also be used as a general textual **data augmentation tool** for **various NLP tasks** (including sentiment analysis, topic classification, NER, and QA).
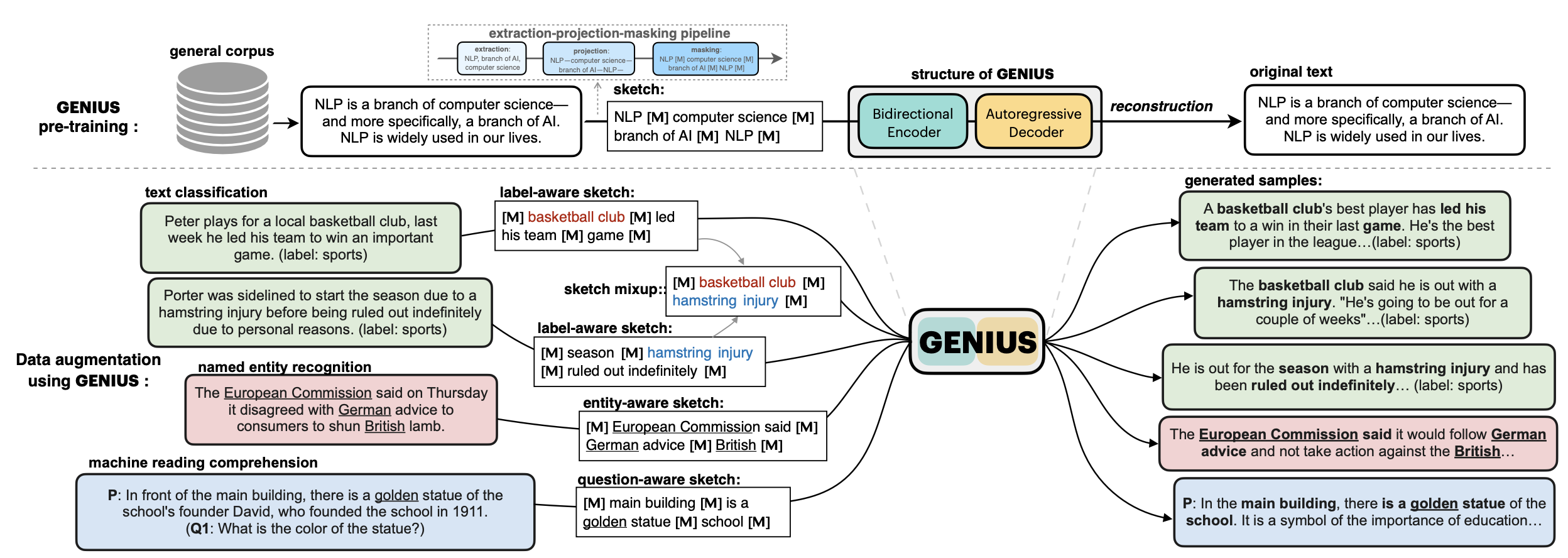
- Models hosted in 🤗 Huggingface:
**Model variations:**
| Model | #params | Language | comment|
|------------------------|--------------------------------|-------|---------|
| [`genius-large`](https://huggingface.co/beyond/genius-large) | 406M | English | The version used in **paper** (recommend) |
| [`genius-large-k2t`](https://huggingface.co/beyond/genius-large-k2t) | 406M | English | keywords-to-text |
| [`genius-base`](https://huggingface.co/beyond/genius-base) | 139M | English | smaller version |
| [`genius-base-ps`](https://huggingface.co/beyond/genius-base) | 139M | English | pre-trained both in paragraphs and short sentences |
| [`genius-base-chinese`](https://huggingface.co/beyond/genius-base-chinese) | 116M | 中文 | 在一千万纯净中文段落上预训练|
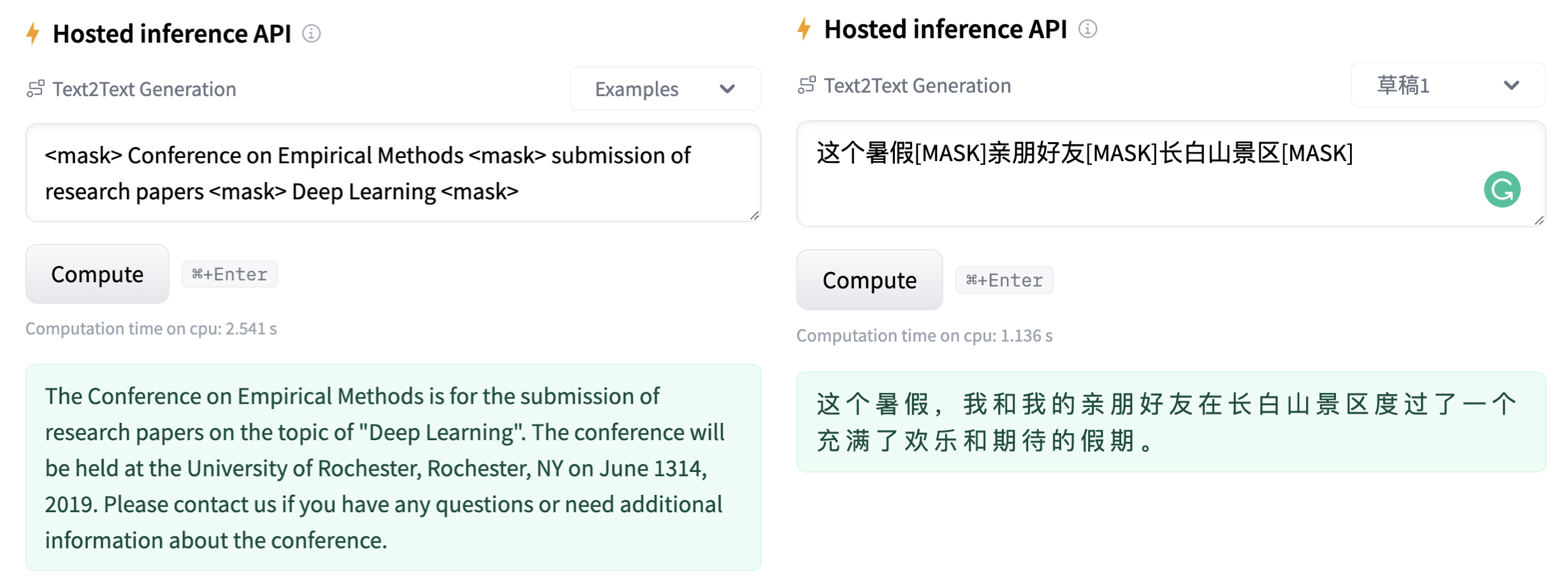
More Examples:
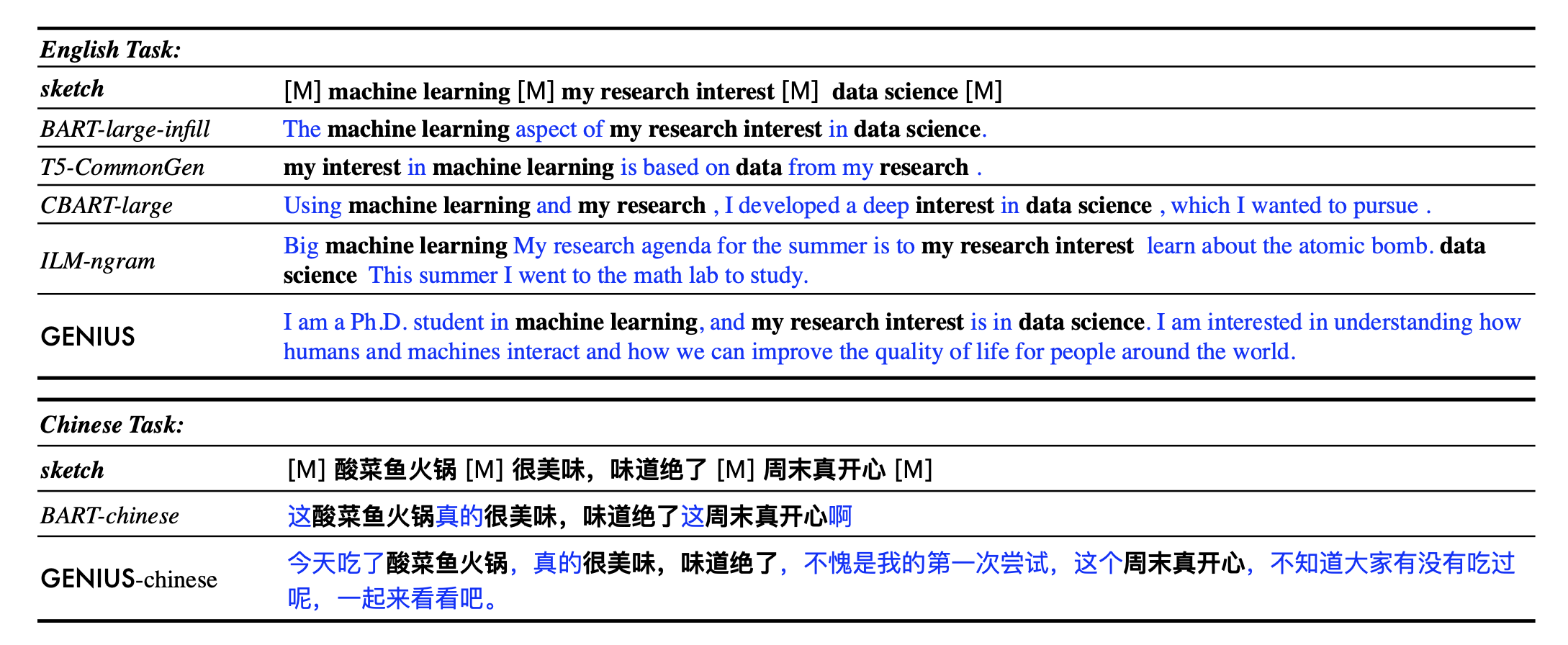
## Usage
### What is a sketch?
First, what is a **sketch**? As defined in our paper, a sketch is "key information consisting of textual spans, phrases, or words, concatenated by mask tokens". It's like a draft or framework when you begin to write an article. With GENIUS model, you can input some key elements you want to mention in your wrinting, then the GENIUS model can generate cohrent text based on your sketch.
The sketch which can be composed of:
- keywords /key-phrases, like `__NLP__AI__computer__science__`
- spans, like `Conference on Empirical Methods__submission of research papers__`
- sentences, like `I really like machine learning__I work at Google since last year__`
- or a mixup!
### How to use the model
#### 1. If you already have a sketch in mind, and want to get a paragraph based on it...
```python
from transformers import pipeline
# 1. load the model with the huggingface `pipeline`
genius = pipeline("text2text-generation", model='beyond/genius-large', device=0)
# 2. provide a sketch (joint by <mask> tokens)
sketch = "<mask> Conference on Empirical Methods <mask> submission of research papers <mask> Deep Learning <mask>"
# 3. here we go!
generated_text = genius(sketch, num_beams=3, do_sample=True, max_length=200)[0]['generated_text']
print(generated_text)
```
Output:
```shell
'The Conference on Empirical Methods welcomes the submission of research papers. Abstracts should be in the form of a paper or presentation. Please submit abstracts to the following email address: eemml.stanford.edu. The conference will be held at Stanford University on April 1618, 2019. The theme of the conference is Deep Learning.'
```
If you have a lot of sketches, you can batch-up your sketches to a Huggingface `Dataset` object, which can be much faster.
TODO: we are also building a python package for more convenient use of GENIUS, which will be released in few weeks.
#### 2. If you have an NLP dataset (e.g. classification) and want to do data augmentation to enlarge your dataset...
Please check [genius/augmentation_clf](https://github.com/beyondguo/genius/tree/master/augmentation_clf) and [genius/augmentation_ner_qa](https://github.com/beyondguo/genius/tree/master/augmentation_ner_qa), where we provide ready-to-run scripts for data augmentation for text classification/NER/MRC tasks.
## Augmentation Experiments:
Data augmentation is an important application for natural language generation (NLG) models, which is also a valuable evaluation of whether the generated text can be used in real applications.
- Setting: Low-resource setting, where only n={50,100,200,500,1000} labeled samples are available for training. The below results are the average of all training sizes.
- Text Classification Datasets: [HuffPost](https://huggingface.co/datasets/khalidalt/HuffPost), [BBC](https://huggingface.co/datasets/SetFit/bbc-news), [SST2](https://huggingface.co/datasets/glue), [IMDB](https://huggingface.co/datasets/imdb), [Yahoo](https://huggingface.co/datasets/yahoo_answers_topics), [20NG](https://huggingface.co/datasets/newsgroup).
- Base classifier: [DistilBERT](https://huggingface.co/distilbert-base-cased)
In-distribution (ID) evaluations:
| Method | Huff | BBC | Yahoo | 20NG | IMDB | SST2 | avg. |
|:----------:|:----------:|:----------:|:----------:|:----------:|:----------:|:----------:|:----------:|
| none | 79.17 | **96.16** | 45.77 | 46.67 | 77.87 | 76.67 | 70.39 |
| EDA | 79.20 | 95.11 | 45.10 | 46.15 | 77.88 | 75.52 | 69.83 |
| BackT | 80.48 | 95.28 | 46.10 | 46.61 | 78.35 | 76.96 | 70.63 |
| MLM | 80.04 | 96.07 | 45.35 | 46.53 | 75.73 | 76.61 | 70.06 |
| C-MLM | 80.60 | 96.13 | 45.40 | 46.36 | 77.31 | 76.91 | 70.45 |
| LAMBADA | 81.46 | 93.74 | 50.49 | 47.72 | 78.22 | 78.31 | 71.66 |
| STA | 80.74 | 95.64 | 46.96 | 47.27 | 77.88 | 77.80 | 71.05 |
| **GeniusAug** | 81.43 | 95.74 | 49.60 | 50.38 | **80.16** | 78.82 | 72.68 |
| **GeniusAug-f** | **81.82** | 95.99 | **50.42** | **50.81** | 79.40 | **80.57** | **73.17** |
Out-of-distribution (OOD) evaluations:
| | Huff->BBC | BBC->Huff | IMDB->SST2 | SST2->IMDB | avg. |
|------------|:----------:|:----------:|:----------:|:----------:|:----------:|
| none | 62.32 | 62.00 | 74.37 | 73.11 | 67.95 |
| EDA | 67.48 | 58.92 | 75.83 | 69.42 | 67.91 |
| BackT | 67.75 | 63.10 | 75.91 | 72.19 | 69.74 |
| MLM | 66.80 | 65.39 | 73.66 | 73.06 | 69.73 |
| C-MLM | 64.94 | **67.80** | 74.98 | 71.78 | 69.87 |
| LAMBADA | 68.57 | 52.79 | 75.24 | 76.04 | 68.16 |
| STA | 69.31 | 64.82 | 74.72 | 73.62 | 70.61 |
| **GeniusAug** | 74.87 | 66.85 | 76.02 | 74.76 | 73.13 |
| **GeniusAug-f** | **76.18** | 66.89 | **77.45** | **80.36** | **75.22** |
### BibTeX entry and citation info
TBD
|