Datasets:
Upload 4 files
Browse files- .gitattributes +1 -0
- README.md +293 -0
- durhamtrees.py +238 -0
- merged.csv +3 -0
- plantsdataset.py +84 -0
.gitattributes
CHANGED
@@ -53,3 +53,4 @@ saved_model/**/* filter=lfs diff=lfs merge=lfs -text
|
|
53 |
*.jpg filter=lfs diff=lfs merge=lfs -text
|
54 |
*.jpeg filter=lfs diff=lfs merge=lfs -text
|
55 |
*.webp filter=lfs diff=lfs merge=lfs -text
|
|
|
|
53 |
*.jpg filter=lfs diff=lfs merge=lfs -text
|
54 |
*.jpeg filter=lfs diff=lfs merge=lfs -text
|
55 |
*.webp filter=lfs diff=lfs merge=lfs -text
|
56 |
+
merged.csv filter=lfs diff=lfs merge=lfs -text
|
README.md
ADDED
@@ -0,0 +1,293 @@
|
|
|
|
|
|
|
|
|
|
|
|
|
|
|
|
|
|
|
|
|
|
|
|
|
|
|
|
|
|
|
|
|
|
|
|
|
|
|
|
|
|
|
|
|
|
|
|
|
|
|
|
|
|
|
|
|
|
|
|
|
|
|
|
|
|
|
|
|
|
|
|
|
|
|
|
|
|
|
|
|
|
|
|
|
|
|
|
|
|
|
|
|
|
|
|
|
|
|
|
|
|
|
|
|
|
|
|
|
|
|
|
|
|
|
|
|
|
|
|
|
|
|
|
|
|
|
|
|
|
|
|
|
|
|
|
|
|
|
|
|
|
|
|
|
|
|
|
|
|
|
|
|
|
|
|
|
|
|
|
|
|
|
|
|
|
|
|
|
|
|
|
|
|
|
|
|
|
|
|
|
|
|
|
|
|
|
|
|
|
|
|
|
|
|
|
|
|
|
|
|
|
|
|
|
|
|
|
|
|
|
|
|
|
|
|
|
|
|
|
|
|
|
|
|
|
|
|
|
|
|
|
|
|
|
|
|
|
|
|
|
|
|
|
|
|
|
|
|
|
|
|
|
|
|
|
|
|
|
|
|
|
|
|
|
|
|
|
|
|
|
|
|
|
|
|
|
|
|
|
|
|
|
|
|
|
|
|
|
|
|
|
|
|
|
|
|
|
|
|
|
|
|
|
|
|
|
|
|
|
|
|
|
|
|
|
|
|
|
|
|
|
|
|
|
|
|
|
|
|
|
|
|
|
|
|
|
|
|
|
|
|
|
|
|
|
|
|
|
|
|
|
|
|
|
|
|
|
|
|
|
|
|
|
|
|
|
|
|
|
|
|
|
|
|
|
|
|
|
|
|
|
|
|
|
|
|
|
|
|
|
|
|
|
|
|
|
|
|
|
|
|
|
|
|
|
|
|
|
|
|
|
|
|
|
|
|
|
|
|
|
|
|
|
|
|
|
|
|
|
|
|
|
|
|
|
|
|
|
|
|
|
|
|
|
|
|
|
|
|
|
|
|
|
|
|
|
|
|
|
|
|
|
|
|
|
|
|
|
|
|
|
|
|
|
|
|
|
|
|
|
|
|
|
|
|
|
|
|
|
|
|
|
|
|
|
|
|
|
|
|
|
|
|
|
|
|
|
|
|
|
|
|
|
|
|
|
|
|
|
|
|
|
|
|
|
|
|
|
|
|
|
|
|
|
|
|
|
|
|
|
|
|
|
|
|
|
|
|
|
|
|
|
|
|
|
|
|
|
|
|
|
|
|
|
|
|
|
|
|
|
|
|
|
|
|
|
|
|
|
|
|
|
|
|
|
|
|
|
1 |
+
---
|
2 |
+
license: apache-2.0
|
3 |
+
language:
|
4 |
+
- en
|
5 |
+
size_categories:
|
6 |
+
- 1M<n<10M
|
7 |
+
task_categories:
|
8 |
+
- token-classification
|
9 |
+
- table-question-answering
|
10 |
+
---
|
11 |
+
# Durham Urban Canopy Analysis and Enhancement Initiative (DUCAEI)
|
12 |
+
The `Class` is a custom dataset class that brings together information from two distinct domains into a unified dataset.
|
13 |
+
This class is designed to streamline the process of working with data from different sources and enable users to seamlessly access and analyze combined datasets.
|
14 |
+
## Project Overview
|
15 |
+
|
16 |
+
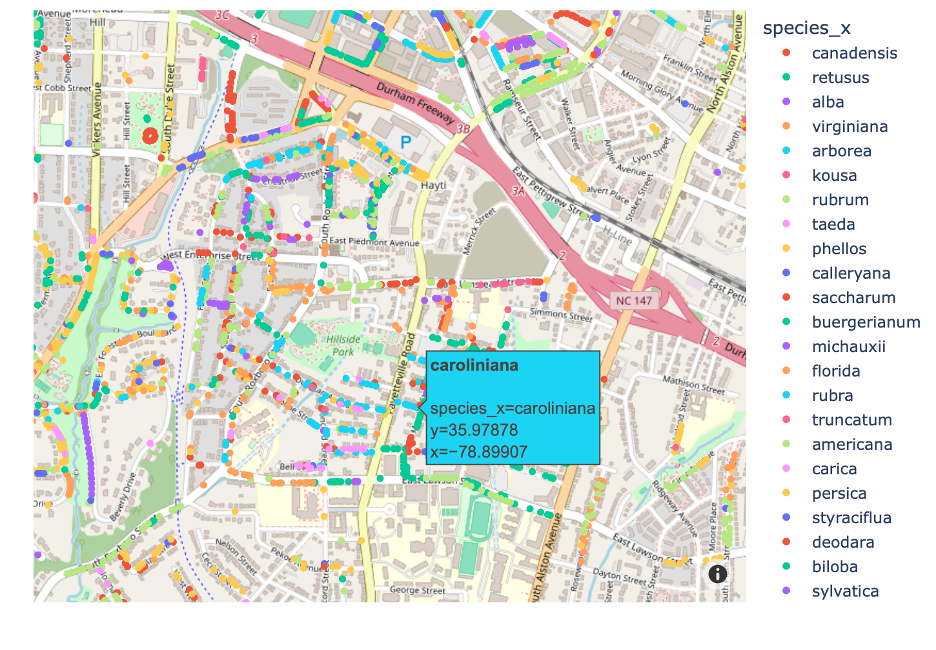
|
17 |
+
|
18 |
+
|
19 |
+
|
20 |
+
The Durham Urban Canopy Analysis and Enhancement Initiative (DUCAEI) is committed to utilizing the Trees & Planting Sites dataset for a comprehensive geospatial analysis of Durham's urban tree canopy. Through Python within Google Colab, our aim is to identify key locations for canopy expansion, evaluate the impact of urban development on green spaces, and deliver informed recommendations for the sustainable growth of urban tree coverage.
|
21 |
+
|
22 |
+
## Background and Rationale
|
23 |
+
|
24 |
+
Durham's urban tree canopy is a crucial component that contributes to environmental quality, public health, and overall city aesthetics. This canopy is under threat due to ongoing urban development and natural wear. A systematic, data-driven approach is critical for strategic planning and conservation of the urban forest to ensure its vitality for generations to come.
|
25 |
+
|
26 |
+
## Data Sources and Methodology
|
27 |
+
These data files are from durham open.
|
28 |
+
|
29 |
+
And for the .py file:
|
30 |
+
|
31 |
+
The provided Python script defines a dataset class named `DurhamTrees` using the `datasets` library. This class combines information from two different domains ("class1_domain1" and "class2_domain1") and includes features from both domains.
|
32 |
+
|
33 |
+
Trees & Planting Sites Dataset: Hosted on the Durham Open Data portal, this dataset includes location, species, size, and health of street trees, alongside designated future planting sites.
|
34 |
+
Data Source: Durham Trees & Planting Sites Dataset
|
35 |
+
https://live-durhamnc.opendata.arcgis.com/datasets/DurhamNC::trees-planting-sites/about
|
36 |
+
|
37 |
+
Key components of the script:
|
38 |
+
|
39 |
+
1. **Imported Libraries:**
|
40 |
+
- `datasets`: for building the dataset.
|
41 |
+
- `pandas`: for handling data in tabular form.
|
42 |
+
- `geopandas`: for working with geospatial data.
|
43 |
+
- Other standard libraries for various functionalities.
|
44 |
+
|
45 |
+
2. **URL Definitions:**
|
46 |
+
- Specifies URLs for CSV and GeoJSON files from two different domains.
|
47 |
+
|
48 |
+
3. **DurhamTrees Class:**
|
49 |
+
- Inherits from `datasets.GeneratorBasedBuilder`.
|
50 |
+
- Defines configurations for two classes ("class1_domain1" and "class2_domain1").
|
51 |
+
- Specifies features for the combined dataset, including features from both classes.
|
52 |
+
|
53 |
+
4. **Info Method:**
|
54 |
+
- Describes the combined dataset's features, supervised keys, homepage, and citation.
|
55 |
+
|
56 |
+
5. **Split Generators Method:**
|
57 |
+
- Downloads and extracts data from the provided URLs.
|
58 |
+
- Defines split generators for training data.
|
59 |
+
|
60 |
+
6. **Generate Examples Methods:**
|
61 |
+
- `_generate_examples`: Calls methods to generate examples for both classes.
|
62 |
+
- `_generate_examples_from_class1`: Reads CSV and GeoJSON data, merges them, and yields examples.
|
63 |
+
- `_generate_examples_from_class2`: Reads CSV data and yields examples.
|
64 |
+
|
65 |
+
7. **Column Extraction:**
|
66 |
+
- Defines columns to extract for both classes, indicating which features to include in the final dataset.
|
67 |
+
|
68 |
+
8. **Example Yielding:**
|
69 |
+
- Iterates over rows of the final dataframes, converting each row to a dictionary and yielding examples with unique identifiers.
|
70 |
+
|
71 |
+
The script is intended for creating a combined dataset from two different sources, and it uses the `datasets` library to facilitate data handling.
|
72 |
+
|
73 |
+
### Data Sources
|
74 |
+
|
75 |
+
We will leverage the following files from the Durham Trees & Planting Sites Dataset, as found on the Durham Open Data portal:
|
76 |
+
|
77 |
+
- `merge.csv`
|
78 |
+
- `Trees_&_Planting_Sites.csv`
|
79 |
+
- `Trees_%26_Planting_Sites.geojson`
|
80 |
+
|
81 |
+
# Dataset Card for Urban Tree Inventory
|
82 |
+
|
83 |
+
## Dataset Description
|
84 |
+
|
85 |
+
This dataset provides comprehensive information about urban trees within a specified area, including their physical characteristics, environmental benefits, and the economic value they add in terms of ecosystem services.
|
86 |
+
|
87 |
+
### Spatial Data (GeoJSON)
|
88 |
+
|
89 |
+
**Format:** GeoJSON
|
90 |
+
|
91 |
+
**Content:**
|
92 |
+
|
93 |
+
- **Type:** `FeatureCollection` - A collection of feature objects.
|
94 |
+
- **Features:** Each feature object represents a tree and contains:
|
95 |
+
- **Type:** `Feature`
|
96 |
+
- **Geometry:** `Point` (includes longitude and latitude of the tree location).
|
97 |
+
- **Properties:** Detailed information about the tree (some fields may overlap with the CSV structure below).
|
98 |
+
|
99 |
+
**IMAGE DAYA**
|
100 |
+
dataset_info:
|
101 |
+
features:
|
102 |
+
- name: image
|
103 |
+
dtype: image
|
104 |
+
- name: label
|
105 |
+
dtype:
|
106 |
+
class_label:
|
107 |
+
names:
|
108 |
+
'0': aechmea_fasciata
|
109 |
+
'1': agave_americana
|
110 |
+
'2': agave_attenuata
|
111 |
+
'3': agave_tequilana
|
112 |
+
'4': aglaonema_commutatum
|
113 |
+
'5': albuca_spiralis
|
114 |
+
'6': allium_cepa
|
115 |
+
'7': allium_sativum
|
116 |
+
|
117 |
+
### Tabular Data (CSV)
|
118 |
+
|
119 |
+
**Format:** CSV
|
120 |
+
|
121 |
+
**Columns:**
|
122 |
+
|
123 |
+
- **X, Y:** Coordinates of the tree location.
|
124 |
+
- **OBJECTID:** Unique identifier for the tree.
|
125 |
+
- **streetaddress:** Street address nearest to the tree.
|
126 |
+
- **city:** City where the tree is located.
|
127 |
+
- **zipcode:** Zip code for the location of the tree.
|
128 |
+
- **facilityid:** Identifier for the facility associated with the tree, if any.
|
129 |
+
- **present:** Indication of whether the tree is currently present.
|
130 |
+
- **genus, species, commonname:** Botanical and common names of the tree.
|
131 |
+
- **plantingdate:** Date when the tree was planted.
|
132 |
+
- **diameterin:** Diameter of the tree trunk in inches.
|
133 |
+
- **heightft:** Height of the tree in feet.
|
134 |
+
- **condition:** Health condition of the tree.
|
135 |
+
- **contractwork:** Indicates if the tree has had any contract work done.
|
136 |
+
- **neighborhood:** Neighborhood where the tree is located.
|
137 |
+
- **program:** The program under which the tree was planted.
|
138 |
+
- **plantingw:** Width of the planting site.
|
139 |
+
- **plantingcond:** Condition of the planting site.
|
140 |
+
- **underpwerlins:** Whether the tree is under power lines.
|
141 |
+
- **matureheight:** The mature height of the tree.
|
142 |
+
- **GlobalID:** A global unique identifier for the tree.
|
143 |
+
- **created_user:** The user who created the record.
|
144 |
+
- **created_date:** The date the record was created.
|
145 |
+
- **last_edited_user:** The user who last edited the record.
|
146 |
+
- **last_edited_date:** The date the record was last edited.
|
147 |
+
|
148 |
+
#### Environmental and Economic Data:
|
149 |
+
|
150 |
+
- **isoprene, monoterpene, vocs:** Emissions and absorption data for various compounds.
|
151 |
+
- **coremoved_ozperyr, o3removed_ozperyr, etc.:** Annual pollutant removal metrics.
|
152 |
+
- **o2production_lbperyr:** Annual oxygen production.
|
153 |
+
- **carbonstorage_lb, carbonstorage_dol:** Carbon storage metrics.
|
154 |
+
- **grosscarseq_lbperyr, grosscarseq_dolperyr:** Gross carbon sequestration.
|
155 |
+
- **avoidrunoff_ft2peryr, avoidrunoff_dol2peryr:** Metrics related to stormwater runoff avoidance.
|
156 |
+
- **totannbenefits_dolperyr:** Total annual dollar benefits from the tree.
|
157 |
+
- **leafarea_sqft, potevapotran_cuftperyr, etc.:** Metrics related to the water cycle.
|
158 |
+
- **heating_mbtuperyr, cooling_kwhperyr, etc.:** Energy savings related to the tree's impact on building energy use.
|
159 |
+
|
160 |
+
### Example Record
|
161 |
+
|
162 |
+
**GeoJSON Feature:**
|
163 |
+
```json
|
164 |
+
{
|
165 |
+
"crs": {
|
166 |
+
"type": "name",
|
167 |
+
"properties": {
|
168 |
+
"name": "urn:ogc:def:crs:OGC:1.3:CRS84"
|
169 |
+
}
|
170 |
+
},
|
171 |
+
"features": [
|
172 |
+
{
|
173 |
+
"type": "Feature",
|
174 |
+
"properties": {
|
175 |
+
"OBJECTID": 2840940,
|
176 |
+
"streetaddress": "411 N GREGSON ST",
|
177 |
+
"city": "DURHAM",
|
178 |
+
"zipcode": 27701,
|
179 |
+
"facilityid": 2936423,
|
180 |
+
"present": "Planting Site",
|
181 |
+
"genus": null,
|
182 |
+
"species": "",
|
183 |
+
"commonname": null,
|
184 |
+
"plantingdate": null,
|
185 |
+
"diameterin": 0.0,
|
186 |
+
"heightft": null,
|
187 |
+
"condition": null,
|
188 |
+
"contractwork": null,
|
189 |
+
"neighborhood": "Walltown",
|
190 |
+
"program": null,
|
191 |
+
"plantingw": "Greater than 5 ft",
|
192 |
+
"plantingcond": "Fair",
|
193 |
+
"underpwerlins": "No",
|
194 |
+
"matureheight": "Large (over 60 feet tall)",
|
195 |
+
"GlobalID": "{8BA6662A-8777-473A-82BB-FD77FE6813BB}",
|
196 |
+
"created_user": "A1",
|
197 |
+
"created_date": "2024-02-03T10:17:12Z",
|
198 |
+
"last_edited_user": "A1",
|
199 |
+
"last_edited_date": "2024-02-03T10:17:12Z",
|
200 |
+
"isoprene": null,
|
201 |
+
"monoterpene": null,
|
202 |
+
"vocs": null,
|
203 |
+
"coremoved_ozperyr": null,
|
204 |
+
"coremoved_dolperyr": null,
|
205 |
+
"o3removed_ozperyr": null,
|
206 |
+
"o3removed_dolperyr": null,
|
207 |
+
"no2removed_ozperyr": null,
|
208 |
+
"no2removed_dolperyr": null,
|
209 |
+
"so2removed_ozperyr": null,
|
210 |
+
"so2removed_dolperyr": null,
|
211 |
+
"pm10removed_ozperyr": null,
|
212 |
+
"pm10removed_dolperyr": null,
|
213 |
+
"pm25removed_ozperyr": null,
|
214 |
+
"o2production_lbperyr": null,
|
215 |
+
"replacevalue_dol": null,
|
216 |
+
"carbonstorage_lb": null,
|
217 |
+
"carbonstorage_dol": null,
|
218 |
+
"grosscarseq_lbperyr": null,
|
219 |
+
"grosscarseq_dolperyr": null,
|
220 |
+
"avoidrunoff_ft2peryr": null,
|
221 |
+
"avoidrunoff_dol2peryr": null,
|
222 |
+
"polremoved_ozperyr": null,
|
223 |
+
"polremoved_dolperyr": null,
|
224 |
+
"totannbenefits_dolperyr": null,
|
225 |
+
"leafarea_sqft": null,
|
226 |
+
"potevapotran_cuftperyr": null,
|
227 |
+
"evaporation_cuftperyr": null,
|
228 |
+
"transpiration_cuftperyr": null,
|
229 |
+
"h2ointercept_cuftperyr": null,
|
230 |
+
"avoidrunval_cuftperyr": null,
|
231 |
+
"avoidrunval_dol2peryr": null,
|
232 |
+
"carbonavoid_lbperyr": null,
|
233 |
+
"carbonavoid_dolperyr": null,
|
234 |
+
"heating_mbtuperyr": null,
|
235 |
+
"heating_dolperyrmbtu": null,
|
236 |
+
"heating_kwhperyr": null,
|
237 |
+
"heating_dolperyrmwh": null,
|
238 |
+
"cooling_kwhperyr": null,
|
239 |
+
"cooling_dolperyr": null,
|
240 |
+
"totalenerg_dolperyr": null
|
241 |
+
},
|
242 |
+
"geometry": {
|
243 |
+
"type": "Point",
|
244 |
+
"coordinates": [-78.908630289999962, 36.00441249000005, 0.0]
|
245 |
+
}
|
246 |
+
}
|
247 |
+
]
|
248 |
+
}
|
249 |
+
|
250 |
+
```
|
251 |
+
The `Trees_&_Planting_Sites.csv` file encompasses a range of attributes for each record:
|
252 |
+
|
253 |
+
- **OBJECTID:** Unique identifier for each record.
|
254 |
+
- **streetaddr:** Street address where the tree or planting site is located.
|
255 |
+
- **city:** The city name, which is Durham.
|
256 |
+
- **zipcode:** Postal code for the location.
|
257 |
+
- **facilityid:** Identifier possibly linked to a facility or area associated with the tree.
|
258 |
+
- **present:** Type of feature present, such as a tree or a planting site.
|
259 |
+
- **genus:** Genus of the tree.
|
260 |
+
- **species:** Species of the tree.
|
261 |
+
- **commonname:** Common name of the tree.
|
262 |
+
- **plantingda:** Date or year range when the tree was planted or the planting site was established.
|
263 |
+
- ...
|
264 |
+
### Objectives
|
265 |
+
|
266 |
+
1. Combine Shapefile and CSV data into a comprehensive geospatial dataset using Python.
|
267 |
+
2. Apply Python libraries to uncover relationships between tree canopy data and urban development.
|
268 |
+
3. Provide practical insights and strategies for the expansion of Durham's urban tree canopy.
|
269 |
+
4. Produce analyses and visualizations with the GeoJSON file.
|
270 |
+
|
271 |
+
### Methodology
|
272 |
+
|
273 |
+
Our analytical process within Google Colab will encompass:
|
274 |
+
|
275 |
+
- **Data Preparation and Integration:** Using tools like Geopandas, Pandas, and PyShp to organize and combine spatial and tabular data.
|
276 |
+
- **Geospatial Analysis:** Applying Shapely and Rtree for spatial analysis, and using SciPy or Statsmodels for statistical correlations.
|
277 |
+
- **Visualization and Optimization:** Generating maps and graphs with Matplotlib, Seaborn, or Plotly, and utilizing optimization algorithms to suggest optimal planting locations.
|
278 |
+
|
279 |
+
## Deliverables
|
280 |
+
|
281 |
+
1. A collection of Google Colab Python notebooks that outline our analytical processes.
|
282 |
+
2. Interactive maps and visualizations that connect tree canopy coverage with urban development metrics.
|
283 |
+
3. An exhaustive report that contains our findings and recommendations for enhancing the urban canopy.
|
284 |
+
|
285 |
+
## Limitations
|
286 |
+
|
287 |
+
- **Computational Resources:** The limited computational offerings of Google Colab may pose a challenge to the size of the datasets or the complexity of models we can employ.
|
288 |
+
- **Data Quality:** The accuracy and currency of the data ultimately affect the precision of our recommendations.
|
289 |
+
- **Sociopolitical Considerations:** Implementation of our data-driven suggestions must be reviewed within the context of local policy and community input.
|
290 |
+
|
291 |
+
## Conclusion
|
292 |
+
|
293 |
+
DUCAEI aims to create a more verdant and livable urban landscape in Durham through this Python-based analytical project. By laying a strong foundation for data-informed decision-making, we hope to cultivate a thriving, green, and sustainable urban environment.
|
durhamtrees.py
ADDED
@@ -0,0 +1,238 @@
|
|
|
|
|
|
|
|
|
|
|
|
|
|
|
|
|
|
|
|
|
|
|
|
|
|
|
|
|
|
|
|
|
|
|
|
|
|
|
|
|
|
|
|
|
|
|
|
|
|
|
|
|
|
|
|
|
|
|
|
|
|
|
|
|
|
|
|
|
|
|
|
|
|
|
|
|
|
|
|
|
|
|
|
|
|
|
|
|
|
|
|
|
|
|
|
|
|
|
|
|
|
|
|
|
|
|
|
|
|
|
|
|
|
|
|
|
|
|
|
|
|
|
|
|
|
|
|
|
|
|
|
|
|
|
|
|
|
|
|
|
|
|
|
|
|
|
|
|
|
|
|
|
|
|
|
|
|
|
|
|
|
|
|
|
|
|
|
|
|
|
|
|
|
|
|
|
|
|
|
|
|
|
|
|
|
|
|
|
|
|
|
|
|
|
|
|
|
|
|
|
|
|
|
|
|
|
|
|
|
|
|
|
|
|
|
|
|
|
|
|
|
|
|
|
|
|
|
|
|
|
|
|
|
|
|
|
|
|
|
|
|
|
|
|
|
|
|
|
|
|
|
|
|
|
|
|
|
|
|
|
|
|
|
|
|
|
|
|
|
|
|
|
|
|
|
|
|
|
|
|
|
|
|
|
|
|
|
|
|
|
|
|
|
|
|
|
|
|
|
|
|
|
|
|
|
|
|
|
|
|
|
|
|
|
|
|
|
|
|
|
|
|
|
|
|
|
|
|
|
|
|
|
|
|
|
|
|
|
|
|
|
|
|
|
|
|
|
|
|
|
|
|
|
|
|
|
|
|
|
|
|
|
|
|
|
|
|
|
|
|
|
|
|
|
|
|
|
|
|
|
|
|
|
|
|
|
|
|
|
|
|
|
|
|
|
|
|
|
|
|
|
|
|
|
|
|
|
|
|
|
|
|
|
|
|
|
|
|
|
|
|
|
|
|
|
|
|
|
|
|
|
|
|
|
|
|
|
|
|
|
|
|
|
|
|
|
|
|
|
|
|
|
|
|
|
|
|
|
|
|
|
|
|
|
|
|
|
|
|
|
|
|
|
|
|
|
|
|
1 |
+
# -*- coding: utf-8 -*-
|
2 |
+
"""DurhamTrees
|
3 |
+
|
4 |
+
Automatically generated by Colaboratory.
|
5 |
+
|
6 |
+
Original file is located at
|
7 |
+
https://colab.research.google.com/drive/1czig7JIbqTKp9wNUIRcdMEDF3pFgtxKv
|
8 |
+
"""
|
9 |
+
|
10 |
+
# -*- coding: utf-8 -*-
|
11 |
+
"""DurhamTrees
|
12 |
+
Automatically generated by Colaboratory.
|
13 |
+
Original file is located at
|
14 |
+
https://colab.research.google.com/drive/1czig7JIbqTKp9wNUIRcdMEDF3pFgtxKv
|
15 |
+
"""
|
16 |
+
import pyarrow.parquet as pq
|
17 |
+
import pandas as pd
|
18 |
+
import geopandas as gpd
|
19 |
+
from datasets import (
|
20 |
+
GeneratorBasedBuilder, Version, DownloadManager, SplitGenerator, Split,
|
21 |
+
Features, Value, BuilderConfig, DatasetInfo
|
22 |
+
)
|
23 |
+
import matplotlib.pyplot as plt
|
24 |
+
import seaborn as sns
|
25 |
+
import csv
|
26 |
+
import json
|
27 |
+
from shapely.geometry import Point
|
28 |
+
import base64
|
29 |
+
import matplotlib.pyplot as plt
|
30 |
+
import matplotlib.image as mpimg
|
31 |
+
import io
|
32 |
+
# URL definitions
|
33 |
+
_URLS = {
|
34 |
+
"first_domain1": {
|
35 |
+
"csv_file": "https://drive.google.com/uc?export=download&id=18HmgMbtbntWsvAySoZr4nV1KNu-i7GCy",
|
36 |
+
"geojson_file": "https://drive.google.com/uc?export=download&id=1cbn7EY7RofXN7c6Ph0eIGFIZowPZ5lKE",
|
37 |
+
|
38 |
+
},
|
39 |
+
"first_domain2": {
|
40 |
+
"csv_file2": "https://drive.google.com/uc?export=download&id=1RVdaI5dSTPStjhOHO40ypDv2cAQZpi_Y",
|
41 |
+
},
|
42 |
+
}
|
43 |
+
|
44 |
+
# Combined Dataset Class
|
45 |
+
class DurhamTrees(GeneratorBasedBuilder):
|
46 |
+
|
47 |
+
VERSION = Version("1.0.0")
|
48 |
+
|
49 |
+
|
50 |
+
class MyConfig(BuilderConfig):
|
51 |
+
def __init__(self, **kwargs):
|
52 |
+
super().__init__(**kwargs)
|
53 |
+
|
54 |
+
BUILDER_CONFIGS = [
|
55 |
+
MyConfig(name="durham_default", description="Default configuration for DurhamTrees"),
|
56 |
+
]
|
57 |
+
|
58 |
+
|
59 |
+
def _info(self):
|
60 |
+
return DatasetInfo(
|
61 |
+
description="This dataset combines information from both classes, with additional processing for csv_file2.",
|
62 |
+
features=Features({
|
63 |
+
"feature1_from_class1": Value("string"),
|
64 |
+
"geometry":Value("string"),
|
65 |
+
"OBJECTID": Value("int64"),
|
66 |
+
"X": Value("float64"),
|
67 |
+
"Y": Value("float64"),
|
68 |
+
"feature1_from_class2": Value("string"),
|
69 |
+
"streetaddress": Value("string"),
|
70 |
+
"city": Value("string"),
|
71 |
+
"facilityid": Value("int64"),
|
72 |
+
"present": Value("string"),
|
73 |
+
"genus": Value("string"),
|
74 |
+
"species": Value("string"),
|
75 |
+
"commonname": Value("string"),
|
76 |
+
"diameterin": Value("float64"),
|
77 |
+
"condition": Value("string"),
|
78 |
+
"neighborhood": Value("string"),
|
79 |
+
"program": Value("string"),
|
80 |
+
"plantingw": Value("string"),
|
81 |
+
"plantingcond": Value("string"),
|
82 |
+
"underpwerlins": Value("string"),
|
83 |
+
"GlobalID": Value("string"),
|
84 |
+
"created_user": Value("string"),
|
85 |
+
"last_edited_user": Value("string"),
|
86 |
+
"isoprene": Value("float64"),
|
87 |
+
"monoterpene": Value("float64"),
|
88 |
+
"monoterpene_class2": Value("float64"),
|
89 |
+
"vocs": Value("float64"),
|
90 |
+
"coremoved_ozperyr": Value("float64"),
|
91 |
+
"coremoved_dolperyr": Value("float64"),
|
92 |
+
"o3removed_ozperyr": Value("float64"),
|
93 |
+
"o3removed_dolperyr": Value("float64"),
|
94 |
+
"no2removed_ozperyr": Value("float64"),
|
95 |
+
"no2removed_dolperyr": Value("float64"),
|
96 |
+
"so2removed_ozperyr": Value("float64"),
|
97 |
+
"so2removed_dolperyr": Value("float64"),
|
98 |
+
"pm10removed_ozperyr": Value("float64"),
|
99 |
+
"pm10removed_dolperyr": Value("float64"),
|
100 |
+
"pm25removed_ozperyr": Value("float64"),
|
101 |
+
"o2production_lbperyr": Value("float64"),
|
102 |
+
"replacevalue_dol": Value("float64"),
|
103 |
+
"carbonstorage_lb": Value("float64"),
|
104 |
+
"carbonstorage_dol": Value("float64"),
|
105 |
+
"grosscarseq_lbperyr": Value("float64"),
|
106 |
+
"grosscarseq_dolperyr": Value("float64"),
|
107 |
+
"avoidrunoff_ft2peryr": Value("float64"),
|
108 |
+
"avoidrunoff_dol2peryr": Value("float64"),
|
109 |
+
"polremoved_ozperyr": Value("float64"),
|
110 |
+
"polremoved_dolperyr": Value("float64"),
|
111 |
+
"totannbenefits_dolperyr": Value("float64"),
|
112 |
+
"leafarea_sqft": Value("float64"),
|
113 |
+
"potevapotran_cuftperyr": Value("float64"),
|
114 |
+
"evaporation_cuftperyr": Value("float64"),
|
115 |
+
"transpiration_cuftperyr": Value("float64"),
|
116 |
+
"h2ointercept_cuftperyr": Value("float64"),
|
117 |
+
"carbonavoid_lbperyr": Value("float64"),
|
118 |
+
"carbonavoid_dolperyr": Value("float64"),
|
119 |
+
"heating_mbtuperyr": Value("float64"),
|
120 |
+
"heating_dolperyrmbtu": Value("float64"),
|
121 |
+
"heating_kwhperyr": Value("float64"),
|
122 |
+
"heating_dolperyrmwh": Value("float64"),
|
123 |
+
"cooling_kwhperyr": Value("float64"),
|
124 |
+
"cooling_dolperyr": Value("float64"),
|
125 |
+
"totalenerg_dolperyr": Value("float64"),
|
126 |
+
}),
|
127 |
+
supervised_keys=("image", "label"),
|
128 |
+
homepage="https://github.com/AuraMa111?tab=repositories",
|
129 |
+
citation="Citation for the combined dataset",
|
130 |
+
)
|
131 |
+
|
132 |
+
def _split_generators(self, dl_manager):
|
133 |
+
downloaded_files = dl_manager.download_and_extract(_URLS)
|
134 |
+
|
135 |
+
return [
|
136 |
+
SplitGenerator(
|
137 |
+
name=Split.TRAIN,
|
138 |
+
gen_kwargs={
|
139 |
+
"class1_data_file": downloaded_files["first_domain1"]["csv_file"],
|
140 |
+
"class1_geojson_file": downloaded_files["first_domain1"]["geojson_file"],
|
141 |
+
"class2_data_file": downloaded_files["first_domain2"]["csv_file2"],
|
142 |
+
"split": Split.TRAIN,
|
143 |
+
},
|
144 |
+
),
|
145 |
+
]
|
146 |
+
|
147 |
+
|
148 |
+
|
149 |
+
|
150 |
+
def _generate_examples(self, class1_data_file, class1_geojson_file, class2_data_file, parquet_file, split):
|
151 |
+
class1_examples = list(self._generate_examples_from_class1(class1_data_file, class1_geojson_file))
|
152 |
+
class2_examples = list(self._generate_examples_from_class2(class2_data_file))
|
153 |
+
|
154 |
+
|
155 |
+
examples = class1_examples + class2_examples
|
156 |
+
df = pd.DataFrame(examples)
|
157 |
+
|
158 |
+
for id_, example in enumerate(examples):
|
159 |
+
if not isinstance(example, dict):
|
160 |
+
# Convert the example to a dictionary if it's not
|
161 |
+
example = {"example": example}
|
162 |
+
yield id_, example
|
163 |
+
|
164 |
+
def _generate_examples_from_class1(self, csv_filepath, geojson_filepath):
|
165 |
+
columns_to_extract = ["OBJECTID", "X", "Y"] # Remove "geometry" from columns_to_extract
|
166 |
+
csv_data = pd.read_csv(csv_filepath)
|
167 |
+
|
168 |
+
with open(geojson_filepath, 'r') as file:
|
169 |
+
geojson_dict = json.load(file)
|
170 |
+
gdf = gpd.GeoDataFrame.from_features(geojson_dict['features'], crs="EPSG:4326") # Specify the CRS if known
|
171 |
+
merged_data = gdf.merge(csv_data, on='OBJECTID')
|
172 |
+
final_data = merged_data[columns_to_extract + ['geometry']] # Include 'geometry' in the final_data
|
173 |
+
for id_, row in final_data.iterrows():
|
174 |
+
example = row.to_dict()
|
175 |
+
yield id_, example
|
176 |
+
|
177 |
+
|
178 |
+
|
179 |
+
|
180 |
+
|
181 |
+
def _generate_examples_from_class2(self, csv_filepath2):
|
182 |
+
csv_data2 = pd.read_csv(csv_filepath2)
|
183 |
+
|
184 |
+
|
185 |
+
columns_to_extract = [
|
186 |
+
"streetaddress", "city", "facilityid", "present", "genus", "species",
|
187 |
+
"commonname", "diameterin", "condition", "neighborhood", "program", "plantingw",
|
188 |
+
"plantingcond", "underpwerlins", "GlobalID", "created_user", "last_edited_user", "isoprene", "monoterpene",
|
189 |
+
"monoterpene", "vocs", "coremoved_ozperyr", "coremoved_dolperyr",
|
190 |
+
"o3removed_ozperyr", "o3removed_dolperyr", "no2removed_ozperyr", "no2removed_dolperyr",
|
191 |
+
"so2removed_ozperyr", "so2removed_dolperyr", "pm10removed_ozperyr", "pm10removed_dolperyr",
|
192 |
+
"pm25removed_ozperyr", "o2production_lbperyr", "replacevalue_dol", "carbonstorage_lb",
|
193 |
+
"carbonstorage_dol", "grosscarseq_lbperyr", "grosscarseq_dolperyr", "polremoved_ozperyr", "polremoved_dolperyr",
|
194 |
+
"totannbenefits_dolperyr", "leafarea_sqft", "potevapotran_cuftperyr", "evaporation_cuftperyr",
|
195 |
+
"transpiration_cuftperyr", "h2ointercept_cuftperyr",
|
196 |
+
"carbonavoid_lbperyr", "carbonavoid_dolperyr", "heating_mbtuperyr",
|
197 |
+
"heating_dolperyrmbtu", "heating_kwhperyr", "heating_dolperyrmwh", "cooling_kwhperyr",
|
198 |
+
"cooling_dolperyr", "totalenerg_dolperyr",
|
199 |
+
]
|
200 |
+
|
201 |
+
final_data = csv_data2[columns_to_extract]
|
202 |
+
for id_, row in final_data.iterrows():
|
203 |
+
example = row.to_dict()
|
204 |
+
non_empty_example = {key: value for key, value in example.items() if pd.notna(value)}
|
205 |
+
yield id_, example
|
206 |
+
|
207 |
+
|
208 |
+
|
209 |
+
|
210 |
+
def _correlation_analysis(self, df):
|
211 |
+
correlation_matrix = df.corr()
|
212 |
+
sns.heatmap(correlation_matrix, annot=True, cmap='coolwarm', linewidths=.5)
|
213 |
+
plt.title("Correlation Analysis")
|
214 |
+
plt.show()
|
215 |
+
|
216 |
+
|
217 |
+
|
218 |
+
|
219 |
+
|
220 |
+
|
221 |
+
# Create an instance of the DurhamTrees class
|
222 |
+
durham_trees_dataset = DurhamTrees(name='class1_domain1')
|
223 |
+
|
224 |
+
# Build the dataset
|
225 |
+
durham_trees_dataset.download_and_prepare()
|
226 |
+
|
227 |
+
# Access the dataset
|
228 |
+
dataset = durham_trees_dataset.as_dataset()
|
229 |
+
|
230 |
+
|
231 |
+
# Create an instance of the DurhamTrees class for another configuration
|
232 |
+
durham_trees_dataset_another = DurhamTrees(name='class2_domain1')
|
233 |
+
|
234 |
+
# Build the dataset for the new instance
|
235 |
+
durham_trees_dataset_another.download_and_prepare()
|
236 |
+
|
237 |
+
# Access the dataset for the new instance
|
238 |
+
dataset_another = durham_trees_dataset_another.as_dataset()
|
merged.csv
ADDED
@@ -0,0 +1,3 @@
|
|
|
|
|
|
|
|
|
1 |
+
version https://git-lfs.github.com/spec/v1
|
2 |
+
oid sha256:1c641de2bfbe65205fb17a842455e14a65d7be2f9dafe4a86972152f1d877f9a
|
3 |
+
size 11188246
|
plantsdataset.py
ADDED
@@ -0,0 +1,84 @@
|
|
|
|
|
|
|
|
|
|
|
|
|
|
|
|
|
|
|
|
|
|
|
|
|
|
|
|
|
|
|
|
|
|
|
|
|
|
|
|
|
|
|
|
|
|
|
|
|
|
|
|
|
|
|
|
|
|
|
|
|
|
|
|
|
|
|
|
|
|
|
|
|
|
|
|
|
|
|
|
|
|
|
|
|
|
|
|
|
|
|
|
|
|
|
|
|
|
|
|
|
|
|
|
|
|
|
|
|
|
|
|
|
|
|
|
|
|
|
|
|
|
|
|
|
|
|
|
|
|
|
|
|
|
|
|
|
|
|
|
|
|
|
|
|
|
|
|
|
|
|
|
|
|
|
|
|
|
|
|
|
|
|
|
|
|
|
|
|
|
|
1 |
+
from datasets import DatasetInfo, Features, Value, ClassLabel, Split, SplitGenerator, GeneratorBasedBuilder
|
2 |
+
import os
|
3 |
+
from PIL import Image
|
4 |
+
import matplotlib.pyplot as plt
|
5 |
+
import numpy as np
|
6 |
+
# Google Drive ID for your ZIP file
|
7 |
+
_DRIVE_ID = "1fXgVwhdU5YGj0SPIcHxSpxkhvRh54oEH"
|
8 |
+
_URL = f"https://drive.google.com/uc?export=download&id={_DRIVE_ID}"
|
9 |
+
|
10 |
+
class PlantsDataset(GeneratorBasedBuilder):
|
11 |
+
class MyConfig(BuilderConfig):
|
12 |
+
def __init__(self, **kwargs):
|
13 |
+
super().__init__(**kwargs)
|
14 |
+
|
15 |
+
BUILDER_CONFIGS = [
|
16 |
+
MyConfig(name="plants_default", description="Default configuration for PlantsDataset"),
|
17 |
+
]
|
18 |
+
|
19 |
+
BUILDER_CONFIGS = [
|
20 |
+
MyConfig(name="default", description="Default configuration"),
|
21 |
+
]
|
22 |
+
|
23 |
+
def _info(self):
|
24 |
+
features = Features({
|
25 |
+
"image": Value("string"),
|
26 |
+
"label": ClassLabel(names=["aleo vera", "calotropis gigantea"]),
|
27 |
+
})
|
28 |
+
return DatasetInfo(
|
29 |
+
description="Your dataset description",
|
30 |
+
features=features,
|
31 |
+
supervised_keys=("image", "label"),
|
32 |
+
homepage="Your dataset homepage",
|
33 |
+
citation="Citation for your dataset",
|
34 |
+
)
|
35 |
+
|
36 |
+
def _split_generators(self, dl_manager):
|
37 |
+
downloaded_file = dl_manager.download_and_extract(_URL)
|
38 |
+
|
39 |
+
return [
|
40 |
+
SplitGenerator(
|
41 |
+
name=Split.TRAIN,
|
42 |
+
gen_kwargs={
|
43 |
+
"data_folder": os.path.join(downloaded_file, "train"),
|
44 |
+
},
|
45 |
+
),
|
46 |
+
SplitGenerator(
|
47 |
+
name=Split.TEST,
|
48 |
+
gen_kwargs={
|
49 |
+
"data_folder": os.path.join(downloaded_file, "test"),
|
50 |
+
},
|
51 |
+
),
|
52 |
+
]
|
53 |
+
|
54 |
+
def _generate_examples(self, data_folder):
|
55 |
+
"""Yields examples as (key, example) tuples."""
|
56 |
+
label_names = self.info.features['label'].names
|
57 |
+
for label, subfolder in enumerate(label_names):
|
58 |
+
subfolder_path = os.path.join(data_folder, subfolder)
|
59 |
+
for root, _, files in os.walk(subfolder_path):
|
60 |
+
for file_name in files:
|
61 |
+
file_path = os.path.join(root, file_name)
|
62 |
+
if os.path.isfile(file_path):
|
63 |
+
# Open the image using Pillow and convert it to an array
|
64 |
+
with Image.open(file_path) as image:
|
65 |
+
image_array = np.array(image)
|
66 |
+
|
67 |
+
# Yield the example with the image data and label
|
68 |
+
yield file_path, {
|
69 |
+
"image": image_array.tolist(), # Convert array to list
|
70 |
+
"label": label,
|
71 |
+
}
|
72 |
+
|
73 |
+
def _display_image(self, image_path, label):
|
74 |
+
with Image.open(image_path) as img:
|
75 |
+
plt.imshow(img)
|
76 |
+
plt.title(f"Label: {self.info.features['label'].int2str(label)}")
|
77 |
+
plt.axis('off') # Hide the axis
|
78 |
+
plt.show()
|
79 |
+
|
80 |
+
# Create an instance of the PlantsDataset class
|
81 |
+
plants_dataset = PlantsDataset()
|
82 |
+
|
83 |
+
# Build and upload the dataset
|
84 |
+
plants_dataset.download_and_prepare()
|