modelId
stringlengths 5
122
| author
stringlengths 2
42
| last_modified
unknown | downloads
int64 0
738M
| likes
int64 0
11k
| library_name
stringclasses 245
values | tags
sequencelengths 1
4.05k
| pipeline_tag
stringclasses 48
values | createdAt
unknown | card
stringlengths 1
901k
|
---|---|---|---|---|---|---|---|---|---|
dbmdz/bert-base-italian-xxl-cased | dbmdz | "2023-09-06T22:19:43Z" | 114,537 | 22 | transformers | [
"transformers",
"pytorch",
"tf",
"jax",
"safetensors",
"bert",
"fill-mask",
"it",
"dataset:wikipedia",
"license:mit",
"autotrain_compatible",
"endpoints_compatible",
"region:us"
] | fill-mask | "2022-03-02T23:29:05Z" | ---
language: it
license: mit
datasets:
- wikipedia
---
# 🤗 + 📚 dbmdz BERT and ELECTRA models
In this repository the MDZ Digital Library team (dbmdz) at the Bavarian State
Library open sources Italian BERT and ELECTRA models 🎉
# Italian BERT
The source data for the Italian BERT model consists of a recent Wikipedia dump and
various texts from the [OPUS corpora](http://opus.nlpl.eu/) collection. The final
training corpus has a size of 13GB and 2,050,057,573 tokens.
For sentence splitting, we use NLTK (faster compared to spacy).
Our cased and uncased models are training with an initial sequence length of 512
subwords for ~2-3M steps.
For the XXL Italian models, we use the same training data from OPUS and extend
it with data from the Italian part of the [OSCAR corpus](https://traces1.inria.fr/oscar/).
Thus, the final training corpus has a size of 81GB and 13,138,379,147 tokens.
Note: Unfortunately, a wrong vocab size was used when training the XXL models.
This explains the mismatch of the "real" vocab size of 31102, compared to the
vocab size specified in `config.json`. However, the model is working and all
evaluations were done under those circumstances.
See [this issue](https://github.com/dbmdz/berts/issues/7) for more information.
The Italian ELECTRA model was trained on the "XXL" corpus for 1M steps in total using a batch
size of 128. We pretty much following the ELECTRA training procedure as used for
[BERTurk](https://github.com/stefan-it/turkish-bert/tree/master/electra).
## Model weights
Currently only PyTorch-[Transformers](https://github.com/huggingface/transformers)
compatible weights are available. If you need access to TensorFlow checkpoints,
please raise an issue!
| Model | Downloads
| ---------------------------------------------------- | ---------------------------------------------------------------------------------------------------------------
| `dbmdz/bert-base-italian-cased` | [`config.json`](https://cdn.huggingface.co/dbmdz/bert-base-italian-cased/config.json) • [`pytorch_model.bin`](https://cdn.huggingface.co/dbmdz/bert-base-italian-cased/pytorch_model.bin) • [`vocab.txt`](https://cdn.huggingface.co/dbmdz/bert-base-italian-cased/vocab.txt)
| `dbmdz/bert-base-italian-uncased` | [`config.json`](https://cdn.huggingface.co/dbmdz/bert-base-italian-uncased/config.json) • [`pytorch_model.bin`](https://cdn.huggingface.co/dbmdz/bert-base-italian-uncased/pytorch_model.bin) • [`vocab.txt`](https://cdn.huggingface.co/dbmdz/bert-base-italian-uncased/vocab.txt)
| `dbmdz/bert-base-italian-xxl-cased` | [`config.json`](https://cdn.huggingface.co/dbmdz/bert-base-italian-xxl-cased/config.json) • [`pytorch_model.bin`](https://cdn.huggingface.co/dbmdz/bert-base-italian-xxl-cased/pytorch_model.bin) • [`vocab.txt`](https://cdn.huggingface.co/dbmdz/bert-base-italian-xxl-cased/vocab.txt)
| `dbmdz/bert-base-italian-xxl-uncased` | [`config.json`](https://cdn.huggingface.co/dbmdz/bert-base-italian-xxl-uncased/config.json) • [`pytorch_model.bin`](https://cdn.huggingface.co/dbmdz/bert-base-italian-xxl-uncased/pytorch_model.bin) • [`vocab.txt`](https://cdn.huggingface.co/dbmdz/bert-base-italian-xxl-uncased/vocab.txt)
| `dbmdz/electra-base-italian-xxl-cased-discriminator` | [`config.json`](https://s3.amazonaws.com/models.huggingface.co/bert/dbmdz/electra-base-italian-xxl-cased-discriminator/config.json) • [`pytorch_model.bin`](https://cdn.huggingface.co/dbmdz/electra-base-italian-xxl-cased-discriminator/pytorch_model.bin) • [`vocab.txt`](https://cdn.huggingface.co/dbmdz/electra-base-italian-xxl-cased-discriminator/vocab.txt)
| `dbmdz/electra-base-italian-xxl-cased-generator` | [`config.json`](https://s3.amazonaws.com/models.huggingface.co/bert/dbmdz/electra-base-italian-xxl-cased-generator/config.json) • [`pytorch_model.bin`](https://cdn.huggingface.co/dbmdz/electra-base-italian-xxl-cased-generator/pytorch_model.bin) • [`vocab.txt`](https://cdn.huggingface.co/dbmdz/electra-base-italian-xxl-cased-generator/vocab.txt)
## Results
For results on downstream tasks like NER or PoS tagging, please refer to
[this repository](https://github.com/stefan-it/italian-bertelectra).
## Usage
With Transformers >= 2.3 our Italian BERT models can be loaded like:
```python
from transformers import AutoModel, AutoTokenizer
model_name = "dbmdz/bert-base-italian-cased"
tokenizer = AutoTokenizer.from_pretrained(model_name)
model = AutoModel.from_pretrained(model_name)
```
To load the (recommended) Italian XXL BERT models, just use:
```python
from transformers import AutoModel, AutoTokenizer
model_name = "dbmdz/bert-base-italian-xxl-cased"
tokenizer = AutoTokenizer.from_pretrained(model_name)
model = AutoModel.from_pretrained(model_name)
```
To load the Italian XXL ELECTRA model (discriminator), just use:
```python
from transformers import AutoModel, AutoTokenizer
model_name = "dbmdz/electra-base-italian-xxl-cased-discriminator"
tokenizer = AutoTokenizer.from_pretrained(model_name)
model = AutoModelWithLMHead.from_pretrained(model_name)
```
# Huggingface model hub
All models are available on the [Huggingface model hub](https://huggingface.co/dbmdz).
# Contact (Bugs, Feedback, Contribution and more)
For questions about our BERT/ELECTRA models just open an issue
[here](https://github.com/dbmdz/berts/issues/new) 🤗
# Acknowledgments
Research supported with Cloud TPUs from Google's TensorFlow Research Cloud (TFRC).
Thanks for providing access to the TFRC ❤️
Thanks to the generous support from the [Hugging Face](https://huggingface.co/) team,
it is possible to download both cased and uncased models from their S3 storage 🤗
|
flair/ner-german | flair | "2023-04-05T09:42:58Z" | 114,268 | 14 | flair | [
"flair",
"pytorch",
"token-classification",
"sequence-tagger-model",
"de",
"dataset:conll2003",
"region:us"
] | token-classification | "2022-03-02T23:29:05Z" | ---
tags:
- flair
- token-classification
- sequence-tagger-model
language: de
datasets:
- conll2003
widget:
- text: "George Washington ging nach Washington"
---
## German NER in Flair (default model)
This is the standard 4-class NER model for German that ships with [Flair](https://github.com/flairNLP/flair/).
F1-Score: **87,94** (CoNLL-03 German revised)
Predicts 4 tags:
| **tag** | **meaning** |
|---------------------------------|-----------|
| PER | person name |
| LOC | location name |
| ORG | organization name |
| MISC | other name |
Based on [Flair embeddings](https://www.aclweb.org/anthology/C18-1139/) and LSTM-CRF.
---
### Demo: How to use in Flair
Requires: **[Flair](https://github.com/flairNLP/flair/)** (`pip install flair`)
```python
from flair.data import Sentence
from flair.models import SequenceTagger
# load tagger
tagger = SequenceTagger.load("flair/ner-german")
# make example sentence
sentence = Sentence("George Washington ging nach Washington")
# predict NER tags
tagger.predict(sentence)
# print sentence
print(sentence)
# print predicted NER spans
print('The following NER tags are found:')
# iterate over entities and print
for entity in sentence.get_spans('ner'):
print(entity)
```
This yields the following output:
```
Span [1,2]: "George Washington" [− Labels: PER (0.9977)]
Span [5]: "Washington" [− Labels: LOC (0.9895)]
```
So, the entities "*George Washington*" (labeled as a **person**) and "*Washington*" (labeled as a **location**) are found in the sentence "*George Washington ging nach Washington*".
---
### Training: Script to train this model
The following Flair script was used to train this model:
```python
from flair.data import Corpus
from flair.datasets import CONLL_03_GERMAN
from flair.embeddings import WordEmbeddings, StackedEmbeddings, FlairEmbeddings
# 1. get the corpus
corpus: Corpus = CONLL_03_GERMAN()
# 2. what tag do we want to predict?
tag_type = 'ner'
# 3. make the tag dictionary from the corpus
tag_dictionary = corpus.make_tag_dictionary(tag_type=tag_type)
# 4. initialize each embedding we use
embedding_types = [
# GloVe embeddings
WordEmbeddings('de'),
# contextual string embeddings, forward
FlairEmbeddings('de-forward'),
# contextual string embeddings, backward
FlairEmbeddings('de-backward'),
]
# embedding stack consists of Flair and GloVe embeddings
embeddings = StackedEmbeddings(embeddings=embedding_types)
# 5. initialize sequence tagger
from flair.models import SequenceTagger
tagger = SequenceTagger(hidden_size=256,
embeddings=embeddings,
tag_dictionary=tag_dictionary,
tag_type=tag_type)
# 6. initialize trainer
from flair.trainers import ModelTrainer
trainer = ModelTrainer(tagger, corpus)
# 7. run training
trainer.train('resources/taggers/ner-german',
train_with_dev=True,
max_epochs=150)
```
---
### Cite
Please cite the following paper when using this model.
```
@inproceedings{akbik2018coling,
title={Contextual String Embeddings for Sequence Labeling},
author={Akbik, Alan and Blythe, Duncan and Vollgraf, Roland},
booktitle = {{COLING} 2018, 27th International Conference on Computational Linguistics},
pages = {1638--1649},
year = {2018}
}
```
---
### Issues?
The Flair issue tracker is available [here](https://github.com/flairNLP/flair/issues/).
|
mradermacher/Athena-70B-L3-i1-GGUF | mradermacher | "2024-07-03T00:06:53Z" | 114,132 | 0 | transformers | [
"transformers",
"gguf",
"en",
"base_model:AiMavenAi/Athena-70B-L3",
"endpoints_compatible",
"region:us"
] | null | "2024-06-28T03:12:26Z" | ---
base_model: AiMavenAi/Athena-70B-L3
language:
- en
library_name: transformers
quantized_by: mradermacher
---
## About
<!-- ### quantize_version: 2 -->
<!-- ### output_tensor_quantised: 1 -->
<!-- ### convert_type: hf -->
<!-- ### vocab_type: -->
<!-- ### tags: nicoboss -->
weighted/imatrix quants of https://huggingface.co/AiMavenAi/Athena-70B-L3
<!-- provided-files -->
static quants are available at https://huggingface.co/mradermacher/Athena-70B-L3-GGUF
## Usage
If you are unsure how to use GGUF files, refer to one of [TheBloke's
READMEs](https://huggingface.co/TheBloke/KafkaLM-70B-German-V0.1-GGUF) for
more details, including on how to concatenate multi-part files.
## Provided Quants
(sorted by size, not necessarily quality. IQ-quants are often preferable over similar sized non-IQ quants)
| Link | Type | Size/GB | Notes |
|:-----|:-----|--------:|:------|
| [GGUF](https://huggingface.co/mradermacher/Athena-70B-L3-i1-GGUF/resolve/main/Athena-70B-L3.i1-IQ2_XXS.gguf) | i1-IQ2_XXS | 19.2 | |
| [GGUF](https://huggingface.co/mradermacher/Athena-70B-L3-i1-GGUF/resolve/main/Athena-70B-L3.i1-IQ2_XS.gguf) | i1-IQ2_XS | 21.2 | |
| [GGUF](https://huggingface.co/mradermacher/Athena-70B-L3-i1-GGUF/resolve/main/Athena-70B-L3.i1-IQ2_M.gguf) | i1-IQ2_M | 24.2 | |
| [GGUF](https://huggingface.co/mradermacher/Athena-70B-L3-i1-GGUF/resolve/main/Athena-70B-L3.i1-Q2_K.gguf) | i1-Q2_K | 26.5 | IQ3_XXS probably better |
| [GGUF](https://huggingface.co/mradermacher/Athena-70B-L3-i1-GGUF/resolve/main/Athena-70B-L3.i1-IQ3_XXS.gguf) | i1-IQ3_XXS | 27.6 | lower quality |
| [GGUF](https://huggingface.co/mradermacher/Athena-70B-L3-i1-GGUF/resolve/main/Athena-70B-L3.i1-IQ3_XS.gguf) | i1-IQ3_XS | 29.4 | |
| [GGUF](https://huggingface.co/mradermacher/Athena-70B-L3-i1-GGUF/resolve/main/Athena-70B-L3.i1-IQ3_S.gguf) | i1-IQ3_S | 31.0 | beats Q3_K* |
| [GGUF](https://huggingface.co/mradermacher/Athena-70B-L3-i1-GGUF/resolve/main/Athena-70B-L3.i1-Q3_K_S.gguf) | i1-Q3_K_S | 31.0 | IQ3_XS probably better |
| [GGUF](https://huggingface.co/mradermacher/Athena-70B-L3-i1-GGUF/resolve/main/Athena-70B-L3.i1-IQ3_M.gguf) | i1-IQ3_M | 32.0 | |
| [GGUF](https://huggingface.co/mradermacher/Athena-70B-L3-i1-GGUF/resolve/main/Athena-70B-L3.i1-Q3_K_M.gguf) | i1-Q3_K_M | 34.4 | IQ3_S probably better |
| [GGUF](https://huggingface.co/mradermacher/Athena-70B-L3-i1-GGUF/resolve/main/Athena-70B-L3.i1-Q3_K_L.gguf) | i1-Q3_K_L | 37.2 | IQ3_M probably better |
| [GGUF](https://huggingface.co/mradermacher/Athena-70B-L3-i1-GGUF/resolve/main/Athena-70B-L3.i1-IQ4_XS.gguf) | i1-IQ4_XS | 38.0 | |
| [GGUF](https://huggingface.co/mradermacher/Athena-70B-L3-i1-GGUF/resolve/main/Athena-70B-L3.i1-Q4_0.gguf) | i1-Q4_0 | 40.2 | fast, low quality |
| [GGUF](https://huggingface.co/mradermacher/Athena-70B-L3-i1-GGUF/resolve/main/Athena-70B-L3.i1-Q4_K_S.gguf) | i1-Q4_K_S | 40.4 | optimal size/speed/quality |
| [GGUF](https://huggingface.co/mradermacher/Athena-70B-L3-i1-GGUF/resolve/main/Athena-70B-L3.i1-Q4_K_M.gguf) | i1-Q4_K_M | 42.6 | fast, recommended |
| [GGUF](https://huggingface.co/mradermacher/Athena-70B-L3-i1-GGUF/resolve/main/Athena-70B-L3.i1-Q5_K_S.gguf) | i1-Q5_K_S | 48.8 | |
| [GGUF](https://huggingface.co/mradermacher/Athena-70B-L3-i1-GGUF/resolve/main/Athena-70B-L3.i1-Q5_K_M.gguf) | i1-Q5_K_M | 50.0 | |
| [PART 1](https://huggingface.co/mradermacher/Athena-70B-L3-i1-GGUF/resolve/main/Athena-70B-L3.i1-Q6_K.gguf.part1of2) [PART 2](https://huggingface.co/mradermacher/Athena-70B-L3-i1-GGUF/resolve/main/Athena-70B-L3.i1-Q6_K.gguf.part2of2) | i1-Q6_K | 58.0 | practically like static Q6_K |
Here is a handy graph by ikawrakow comparing some lower-quality quant
types (lower is better):

And here are Artefact2's thoughts on the matter:
https://gist.github.com/Artefact2/b5f810600771265fc1e39442288e8ec9
## FAQ / Model Request
See https://huggingface.co/mradermacher/model_requests for some answers to
questions you might have and/or if you want some other model quantized.
## Thanks
I thank my company, [nethype GmbH](https://www.nethype.de/), for letting
me use its servers and providing upgrades to my workstation to enable
this work in my free time. Additional thanks to [@nicoboss](https://huggingface.co/nicoboss) for giving me access to his hardware for calculating the imatrix for these quants.
<!-- end -->
|
fxmarty/tiny-doc-qa-vision-encoder-decoder | fxmarty | "2023-10-17T09:09:37Z" | 113,936 | 5 | transformers | [
"transformers",
"pytorch",
"vision-encoder-decoder",
"document-question-answering",
"license:mit",
"endpoints_compatible",
"region:us"
] | document-question-answering | "2023-06-14T09:03:48Z" | ---
license: mit
pipeline_tag: document-question-answering
---
For testing purposes only |
mradermacher/cerberus-v0.1-i1-GGUF | mradermacher | "2024-06-30T01:46:48Z" | 113,791 | 0 | transformers | [
"transformers",
"gguf",
"en",
"base_model:brahmairesearch/cerberus-v0.1",
"license:llama3",
"endpoints_compatible",
"region:us"
] | null | "2024-06-28T15:18:23Z" | ---
base_model: brahmairesearch/cerberus-v0.1
language:
- en
library_name: transformers
license: llama3
quantized_by: mradermacher
---
## About
<!-- ### quantize_version: 2 -->
<!-- ### output_tensor_quantised: 1 -->
<!-- ### convert_type: hf -->
<!-- ### vocab_type: -->
<!-- ### tags: nicoboss -->
weighted/imatrix quants of https://huggingface.co/brahmairesearch/cerberus-v0.1
<!-- provided-files -->
static quants are available at https://huggingface.co/mradermacher/cerberus-v0.1-GGUF
## Usage
If you are unsure how to use GGUF files, refer to one of [TheBloke's
READMEs](https://huggingface.co/TheBloke/KafkaLM-70B-German-V0.1-GGUF) for
more details, including on how to concatenate multi-part files.
## Provided Quants
(sorted by size, not necessarily quality. IQ-quants are often preferable over similar sized non-IQ quants)
| Link | Type | Size/GB | Notes |
|:-----|:-----|--------:|:------|
| [GGUF](https://huggingface.co/mradermacher/cerberus-v0.1-i1-GGUF/resolve/main/cerberus-v0.1.i1-IQ1_S.gguf) | i1-IQ1_S | 15.4 | for the desperate |
| [GGUF](https://huggingface.co/mradermacher/cerberus-v0.1-i1-GGUF/resolve/main/cerberus-v0.1.i1-IQ1_M.gguf) | i1-IQ1_M | 16.9 | mostly desperate |
| [GGUF](https://huggingface.co/mradermacher/cerberus-v0.1-i1-GGUF/resolve/main/cerberus-v0.1.i1-IQ2_XXS.gguf) | i1-IQ2_XXS | 19.2 | |
| [GGUF](https://huggingface.co/mradermacher/cerberus-v0.1-i1-GGUF/resolve/main/cerberus-v0.1.i1-IQ2_XS.gguf) | i1-IQ2_XS | 21.2 | |
| [GGUF](https://huggingface.co/mradermacher/cerberus-v0.1-i1-GGUF/resolve/main/cerberus-v0.1.i1-IQ2_S.gguf) | i1-IQ2_S | 22.3 | |
| [GGUF](https://huggingface.co/mradermacher/cerberus-v0.1-i1-GGUF/resolve/main/cerberus-v0.1.i1-IQ2_M.gguf) | i1-IQ2_M | 24.2 | |
| [GGUF](https://huggingface.co/mradermacher/cerberus-v0.1-i1-GGUF/resolve/main/cerberus-v0.1.i1-Q2_K.gguf) | i1-Q2_K | 26.5 | IQ3_XXS probably better |
| [GGUF](https://huggingface.co/mradermacher/cerberus-v0.1-i1-GGUF/resolve/main/cerberus-v0.1.i1-IQ3_XXS.gguf) | i1-IQ3_XXS | 27.6 | lower quality |
| [GGUF](https://huggingface.co/mradermacher/cerberus-v0.1-i1-GGUF/resolve/main/cerberus-v0.1.i1-IQ3_XS.gguf) | i1-IQ3_XS | 29.4 | |
| [GGUF](https://huggingface.co/mradermacher/cerberus-v0.1-i1-GGUF/resolve/main/cerberus-v0.1.i1-IQ3_S.gguf) | i1-IQ3_S | 31.0 | beats Q3_K* |
| [GGUF](https://huggingface.co/mradermacher/cerberus-v0.1-i1-GGUF/resolve/main/cerberus-v0.1.i1-Q3_K_S.gguf) | i1-Q3_K_S | 31.0 | IQ3_XS probably better |
| [GGUF](https://huggingface.co/mradermacher/cerberus-v0.1-i1-GGUF/resolve/main/cerberus-v0.1.i1-IQ3_M.gguf) | i1-IQ3_M | 32.0 | |
| [GGUF](https://huggingface.co/mradermacher/cerberus-v0.1-i1-GGUF/resolve/main/cerberus-v0.1.i1-Q3_K_M.gguf) | i1-Q3_K_M | 34.4 | IQ3_S probably better |
| [GGUF](https://huggingface.co/mradermacher/cerberus-v0.1-i1-GGUF/resolve/main/cerberus-v0.1.i1-Q3_K_L.gguf) | i1-Q3_K_L | 37.2 | IQ3_M probably better |
| [GGUF](https://huggingface.co/mradermacher/cerberus-v0.1-i1-GGUF/resolve/main/cerberus-v0.1.i1-IQ4_XS.gguf) | i1-IQ4_XS | 38.0 | |
| [GGUF](https://huggingface.co/mradermacher/cerberus-v0.1-i1-GGUF/resolve/main/cerberus-v0.1.i1-Q4_0.gguf) | i1-Q4_0 | 40.2 | fast, low quality |
| [GGUF](https://huggingface.co/mradermacher/cerberus-v0.1-i1-GGUF/resolve/main/cerberus-v0.1.i1-Q4_K_S.gguf) | i1-Q4_K_S | 40.4 | optimal size/speed/quality |
| [GGUF](https://huggingface.co/mradermacher/cerberus-v0.1-i1-GGUF/resolve/main/cerberus-v0.1.i1-Q4_K_M.gguf) | i1-Q4_K_M | 42.6 | fast, recommended |
| [GGUF](https://huggingface.co/mradermacher/cerberus-v0.1-i1-GGUF/resolve/main/cerberus-v0.1.i1-Q5_K_S.gguf) | i1-Q5_K_S | 48.8 | |
| [GGUF](https://huggingface.co/mradermacher/cerberus-v0.1-i1-GGUF/resolve/main/cerberus-v0.1.i1-Q5_K_M.gguf) | i1-Q5_K_M | 50.0 | |
| [PART 1](https://huggingface.co/mradermacher/cerberus-v0.1-i1-GGUF/resolve/main/cerberus-v0.1.i1-Q6_K.gguf.part1of2) [PART 2](https://huggingface.co/mradermacher/cerberus-v0.1-i1-GGUF/resolve/main/cerberus-v0.1.i1-Q6_K.gguf.part2of2) | i1-Q6_K | 58.0 | practically like static Q6_K |
Here is a handy graph by ikawrakow comparing some lower-quality quant
types (lower is better):

And here are Artefact2's thoughts on the matter:
https://gist.github.com/Artefact2/b5f810600771265fc1e39442288e8ec9
## FAQ / Model Request
See https://huggingface.co/mradermacher/model_requests for some answers to
questions you might have and/or if you want some other model quantized.
## Thanks
I thank my company, [nethype GmbH](https://www.nethype.de/), for letting
me use its servers and providing upgrades to my workstation to enable
this work in my free time. Additional thanks to [@nicoboss](https://huggingface.co/nicoboss) for giving me access to his hardware for calculating the imatrix for these quants.
<!-- end -->
|
facebook/dpr-question_encoder-multiset-base | facebook | "2022-12-21T15:20:05Z" | 113,103 | 4 | transformers | [
"transformers",
"pytorch",
"tf",
"dpr",
"feature-extraction",
"en",
"dataset:nq_open",
"dataset:trivia_qa",
"dataset:web_questions",
"dataset:trec",
"arxiv:2004.04906",
"arxiv:1702.08734",
"arxiv:1910.09700",
"license:cc-by-nc-4.0",
"region:us"
] | feature-extraction | "2022-03-02T23:29:05Z" | ---
language: en
license: cc-by-nc-4.0
tags:
- dpr
datasets:
- nq_open
- trivia_qa
- web_questions
- trec
inference: false
---
# `dpr-question_encoder-multiset-base`
## Table of Contents
- [Model Details](#model-details)
- [How To Get Started With the Model](#how-to-get-started-with-the-model)
- [Uses](#uses)
- [Risks, Limitations and Biases](#risks-limitations-and-biases)
- [Training](#training)
- [Evaluation](#evaluation-results)
- [Environmental Impact](#environmental-impact)
- [Technical Specifications](#technical-specifications)
- [Citation Information](#citation-information)
- [Model Card Authors](#model-card-authors)
## Model Details
**Model Description:** [Dense Passage Retrieval (DPR)](https://github.com/facebookresearch/DPR) is a set of tools and models for state-of-the-art open-domain Q&A research. `dpr-question_encoder-multiset-base` is the question encoder trained using the [Natural Questions (NQ) dataset](https://huggingface.co/datasets/nq_open), [TriviaQA](https://huggingface.co/datasets/trivia_qa), [WebQuestions (WQ)](https://huggingface.co/datasets/web_questions), and [CuratedTREC (TREC)](https://huggingface.co/datasets/trec).
- **Developed by:** See [GitHub repo](https://github.com/facebookresearch/DPR) for model developers
- **Model Type:** BERT-based encoder
- **Language(s):** [CC-BY-NC-4.0](https://github.com/facebookresearch/DPR/blob/main/LICENSE), also see [Code of Conduct](https://github.com/facebookresearch/DPR/blob/main/CODE_OF_CONDUCT.md)
- **License:** English
- **Related Models:**
- [`dpr-ctx_encoder-multiset-base`](https://huggingface.co/facebook/dpr-ctx_encoder-multiset-base)
- [`dpr-reader-multiset-base`](https://huggingface.co/facebook/dpr-reader-multiset-base)
- [`dpr-ctx_encoder-single-nq-base`](https://huggingface.co/facebook/dpr-ctx_encoder-single-nq-base)
- [`dpr-question_encoder-single-nq-base`](https://huggingface.co/facebook/dpr-question_encoder-single-nq-base)
- [`dpr-reader-single-nq-base`](https://huggingface.co/facebook/dpr-reader-single-nq-base)
- **Resources for more information:**
- [Research Paper](https://arxiv.org/abs/2004.04906)
- [GitHub Repo](https://github.com/facebookresearch/DPR)
- [Hugging Face DPR docs](https://huggingface.co/docs/transformers/main/en/model_doc/dpr)
- [BERT Base Uncased Model Card](https://huggingface.co/bert-base-uncased)
## How to Get Started with the Model
Use the code below to get started with the model.
```python
from transformers import DPRQuestionEncoder, DPRQuestionEncoderTokenizer
tokenizer = DPRQuestionEncoderTokenizer.from_pretrained("facebook/dpr-question_encoder-multiset-base")
model = DPRQuestionEncoder.from_pretrained("facebook/dpr-question_encoder-multiset-base")
input_ids = tokenizer("Hello, is my dog cute ?", return_tensors="pt")["input_ids"]
embeddings = model(input_ids).pooler_output
```
## Uses
#### Direct Use
`dpr-question_encoder-multiset-base`, [`dpr-ctx_encoder-multiset-base`](https://huggingface.co/facebook/dpr-ctx_encoder-multiset-base), and [`dpr-reader-multiset-base`](https://huggingface.co/facebook/dpr-reader-multiset-base) can be used for the task of open-domain question answering.
#### Misuse and Out-of-scope Use
The model should not be used to intentionally create hostile or alienating environments for people. In addition, the set of DPR models was not trained to be factual or true representations of people or events, and therefore using the models to generate such content is out-of-scope for the abilities of this model.
## Risks, Limitations and Biases
**CONTENT WARNING: Readers should be aware this section may contain content that is disturbing, offensive, and can propogate historical and current stereotypes.**
Significant research has explored bias and fairness issues with language models (see, e.g., [Sheng et al., 2021](https://aclanthology.org/2021.acl-long.330.pdf) and [Bender et al., 2021](https://dl.acm.org/doi/pdf/10.1145/3442188.3445922)). Predictions generated by the model can include disturbing and harmful stereotypes across protected classes; identity characteristics; and sensitive, social, and occupational groups.
## Training
#### Training Data
This model was trained using the following datasets:
- **[Natural Questions (NQ) dataset](https://huggingface.co/datasets/nq_open)** ([Lee et al., 2019](https://aclanthology.org/P19-1612/); [Kwiatkowski et al., 2019](https://aclanthology.org/Q19-1026/))
- **[TriviaQA](https://huggingface.co/datasets/trivia_qa)** ([Joshi et al., 2017](https://aclanthology.org/P17-1147/))
- **[WebQuestions (WQ)](https://huggingface.co/datasets/web_questions)** ([Berant et al., 2013](https://aclanthology.org/D13-1160/))
- **[CuratedTREC (TREC)](https://huggingface.co/datasets/trec)** ([Baudiš & Šedivý, 2015](https://www.aminer.cn/pub/599c7953601a182cd263079b/reading-wikipedia-to-answer-open-domain-questions))
#### Training Procedure
The training procedure is described in the [associated paper](https://arxiv.org/pdf/2004.04906.pdf):
> Given a collection of M text passages, the goal of our dense passage retriever (DPR) is to index all the passages in a low-dimensional and continuous space, such that it can retrieve efficiently the top k passages relevant to the input question for the reader at run-time.
> Our dense passage retriever (DPR) uses a dense encoder EP(·) which maps any text passage to a d- dimensional real-valued vectors and builds an index for all the M passages that we will use for retrieval. At run-time, DPR applies a different encoder EQ(·) that maps the input question to a d-dimensional vector, and retrieves k passages of which vectors are the closest to the question vector.
The authors report that for encoders, they used two independent BERT ([Devlin et al., 2019](https://aclanthology.org/N19-1423/)) networks (base, un-cased) and use FAISS ([Johnson et al., 2017](https://arxiv.org/abs/1702.08734)) during inference time to encode and index passages. See the paper for further details on training, including encoders, inference, positive and negative passages, and in-batch negatives.
## Evaluation
The following evaluation information is extracted from the [associated paper](https://arxiv.org/pdf/2004.04906.pdf).
#### Testing Data, Factors and Metrics
The model developers report the performance of the model on five QA datasets, using the top-k accuracy (k ∈ {20, 100}). The datasets were [NQ](https://huggingface.co/datasets/nq_open), [TriviaQA](https://huggingface.co/datasets/trivia_qa), [WebQuestions (WQ)](https://huggingface.co/datasets/web_questions), [CuratedTREC (TREC)](https://huggingface.co/datasets/trec), and [SQuAD v1.1](https://huggingface.co/datasets/squad).
#### Results
| | Top 20 | | | | | Top 100| | | | |
|:----:|:------:|:---------:|:--:|:----:|:-----:|:------:|:---------:|:--:|:----:|:-----:|
| | NQ | TriviaQA | WQ | TREC | SQuAD | NQ | TriviaQA | WQ | TREC | SQuAD |
| | 79.4 | 78.8 |75.0| 89.1 | 51.6 | 86.0 | 84.7 |82.9| 93.9 | 67.6 |
## Environmental Impact
Carbon emissions can be estimated using the [Machine Learning Impact calculator](https://mlco2.github.io/impact#compute) presented in [Lacoste et al. (2019)](https://arxiv.org/abs/1910.09700). We present the hardware type and based on the [associated paper](https://arxiv.org/abs/2004.04906).
- **Hardware Type:** 8 32GB GPUs
- **Hours used:** Unknown
- **Cloud Provider:** Unknown
- **Compute Region:** Unknown
- **Carbon Emitted:** Unknown
## Technical Specifications
See the [associated paper](https://arxiv.org/abs/2004.04906) for details on the modeling architecture, objective, compute infrastructure, and training details.
## Citation Information
```bibtex
@inproceedings{karpukhin-etal-2020-dense,
title = "Dense Passage Retrieval for Open-Domain Question Answering",
author = "Karpukhin, Vladimir and Oguz, Barlas and Min, Sewon and Lewis, Patrick and Wu, Ledell and Edunov, Sergey and Chen, Danqi and Yih, Wen-tau",
booktitle = "Proceedings of the 2020 Conference on Empirical Methods in Natural Language Processing (EMNLP)",
month = nov,
year = "2020",
address = "Online",
publisher = "Association for Computational Linguistics",
url = "https://www.aclweb.org/anthology/2020.emnlp-main.550",
doi = "10.18653/v1/2020.emnlp-main.550",
pages = "6769--6781",
}
```
## Model Card Authors
This model card was written by the team at Hugging Face. |
HooshvareLab/bert-base-parsbert-ner-uncased | HooshvareLab | "2021-05-18T20:43:54Z" | 113,056 | 4 | transformers | [
"transformers",
"pytorch",
"tf",
"jax",
"bert",
"token-classification",
"fa",
"arxiv:2005.12515",
"license:apache-2.0",
"autotrain_compatible",
"endpoints_compatible",
"region:us"
] | token-classification | "2022-03-02T23:29:04Z" | ---
language: fa
license: apache-2.0
---
## ParsBERT: Transformer-based Model for Persian Language Understanding
ParsBERT is a monolingual language model based on Google’s BERT architecture with the same configurations as BERT-Base.
Paper presenting ParsBERT: [arXiv:2005.12515](https://arxiv.org/abs/2005.12515)
All the models (downstream tasks) are uncased and trained with whole word masking. (coming soon stay tuned)
## Persian NER [ARMAN, PEYMA, ARMAN+PEYMA]
This task aims to extract named entities in the text, such as names and label with appropriate `NER` classes such as locations, organizations, etc. The datasets used for this task contain sentences that are marked with `IOB` format. In this format, tokens that are not part of an entity are tagged as `”O”` the `”B”`tag corresponds to the first word of an object, and the `”I”` tag corresponds to the rest of the terms of the same entity. Both `”B”` and `”I”` tags are followed by a hyphen (or underscore), followed by the entity category. Therefore, the NER task is a multi-class token classification problem that labels the tokens upon being fed a raw text. There are two primary datasets used in Persian NER, `ARMAN`, and `PEYMA`. In ParsBERT, we prepared ner for both datasets as well as a combination of both datasets.
### PEYMA
PEYMA dataset includes 7,145 sentences with a total of 302,530 tokens from which 41,148 tokens are tagged with seven different classes.
1. Organization
2. Money
3. Location
4. Date
5. Time
6. Person
7. Percent
| Label | # |
|:------------:|:-----:|
| Organization | 16964 |
| Money | 2037 |
| Location | 8782 |
| Date | 4259 |
| Time | 732 |
| Person | 7675 |
| Percent | 699 |
**Download**
You can download the dataset from [here](http://nsurl.org/tasks/task-7-named-entity-recognition-ner-for-farsi/)
---
### ARMAN
ARMAN dataset holds 7,682 sentences with 250,015 sentences tagged over six different classes.
1. Organization
2. Location
3. Facility
4. Event
5. Product
6. Person
| Label | # |
|:------------:|:-----:|
| Organization | 30108 |
| Location | 12924 |
| Facility | 4458 |
| Event | 7557 |
| Product | 4389 |
| Person | 15645 |
**Download**
You can download the dataset from [here](https://github.com/HaniehP/PersianNER)
## Results
The following table summarizes the F1 score obtained by ParsBERT as compared to other models and architectures.
| Dataset | ParsBERT | MorphoBERT | Beheshti-NER | LSTM-CRF | Rule-Based CRF | BiLSTM-CRF |
|:---------------:|:--------:|:----------:|:--------------:|:----------:|:----------------:|:------------:|
| ARMAN + PEYMA | 95.13* | - | - | - | - | - |
| PEYMA | 98.79* | - | 90.59 | - | 84.00 | - |
| ARMAN | 93.10* | 89.9 | 84.03 | 86.55 | - | 77.45 |
## How to use :hugs:
| Notebook | Description | |
|:----------|:-------------|------:|
| [How to use Pipelines](https://github.com/hooshvare/parsbert-ner/blob/master/persian-ner-pipeline.ipynb) | Simple and efficient way to use State-of-the-Art models on downstream tasks through transformers | [](https://colab.research.google.com/github/hooshvare/parsbert-ner/blob/master/persian-ner-pipeline.ipynb) |
## Cite
Please cite the following paper in your publication if you are using [ParsBERT](https://arxiv.org/abs/2005.12515) in your research:
```markdown
@article{ParsBERT,
title={ParsBERT: Transformer-based Model for Persian Language Understanding},
author={Mehrdad Farahani, Mohammad Gharachorloo, Marzieh Farahani, Mohammad Manthouri},
journal={ArXiv},
year={2020},
volume={abs/2005.12515}
}
```
## Acknowledgments
We hereby, express our gratitude to the [Tensorflow Research Cloud (TFRC) program](https://tensorflow.org/tfrc) for providing us with the necessary computation resources. We also thank [Hooshvare](https://hooshvare.com) Research Group for facilitating dataset gathering and scraping online text resources.
## Contributors
- Mehrdad Farahani: [Linkedin](https://www.linkedin.com/in/m3hrdadfi/), [Twitter](https://twitter.com/m3hrdadfi), [Github](https://github.com/m3hrdadfi)
- Mohammad Gharachorloo: [Linkedin](https://www.linkedin.com/in/mohammad-gharachorloo/), [Twitter](https://twitter.com/MGharachorloo), [Github](https://github.com/baarsaam)
- Marzieh Farahani: [Linkedin](https://www.linkedin.com/in/marziehphi/), [Twitter](https://twitter.com/marziehphi), [Github](https://github.com/marziehphi)
- Mohammad Manthouri: [Linkedin](https://www.linkedin.com/in/mohammad-manthouri-aka-mansouri-07030766/), [Twitter](https://twitter.com/mmanthouri), [Github](https://github.com/mmanthouri)
- Hooshvare Team: [Official Website](https://hooshvare.com/), [Linkedin](https://www.linkedin.com/company/hooshvare), [Twitter](https://twitter.com/hooshvare), [Github](https://github.com/hooshvare), [Instagram](https://www.instagram.com/hooshvare/)
+ And a special thanks to Sara Tabrizi for her fantastic poster design. Follow her on: [Linkedin](https://www.linkedin.com/in/sara-tabrizi-64548b79/), [Behance](https://www.behance.net/saratabrizi), [Instagram](https://www.instagram.com/sara_b_tabrizi/)
## Releases
### Release v0.1 (May 29, 2019)
This is the first version of our ParsBERT NER!
|
yiyanghkust/finbert-esg-9-categories | yiyanghkust | "2022-10-17T00:34:01Z" | 112,858 | 35 | transformers | [
"transformers",
"pytorch",
"bert",
"text-classification",
"financial-text-analysis",
"esg",
"environmental-social-corporate-governance",
"en",
"autotrain_compatible",
"endpoints_compatible",
"region:us"
] | text-classification | "2022-10-14T01:16:21Z" | ---
language: "en"
tags:
- financial-text-analysis
- esg
- environmental-social-corporate-governance
widget:
- text: "For 2002, our total net emissions were approximately 60 million metric tons of CO2 equivalents for all businesses and operations we have financial interests in, based on its equity share in those businesses and operations. "
---
ESG analysis can help investors determine a business' long-term sustainability and identify associated risks. **FinBERT-esg-9-categories** is a FinBERT model fine-tuned on about 14,000 manually annotated sentences from firms' ESG reports and annual reports.
**finbert-esg-9-categories** classifies a text into nine fine-grained ESG topics: *Climate Change, Natural Capital, Pollution & Waste, Human Capital, Product Liability, Community Relations, Corporate Governance, Business Ethics & Values, and Non-ESG*. This model complements [**finbert-esg**](https://huggingface.co/yiyanghkust/finbert-esg) which classifies a text into four coarse-grained ESG themes (*E, S, G or None*).
Detailed description of the nine fine-grained ESG topic definition, some examples for each topic, training sample, and the model’s performance can be found [**here**](https://www.allenhuang.org/uploads/2/6/5/5/26555246/esg_9-class_descriptions.pdf).
**Input**: A text.
**Output**: Climate Change, Natural Capital, Pollution & Waste, Human Capital, Product Liability, Community Relations, Corporate Governance, Business Ethics & Values, or Non-ESG.
# How to use
You can use this model with Transformers pipeline for fine-grained ESG 9 categories classification.
```python
from transformers import BertTokenizer, BertForSequenceClassification, pipeline
finbert = BertForSequenceClassification.from_pretrained('yiyanghkust/finbert-esg-9-categories',num_labels=9)
tokenizer = BertTokenizer.from_pretrained('yiyanghkust/finbert-esg-9-categories')
nlp = pipeline("text-classification", model=finbert, tokenizer=tokenizer)
results = nlp('For 2002, our total net emissions were approximately 60 million metric tons of CO2 equivalents for all businesses
and operations we have financial interests in, based on its equity share in those businesses and operations.')
print(results) # [{'label': 'Climate Change', 'score': 0.9955655932426453}]
```
If you use the model in your academic work, please cite the following paper:
Huang, Allen H., Hui Wang, and Yi Yang. "FinBERT: A Large Language Model for Extracting Information from Financial Text." *Contemporary Accounting Research* (2022). |
urchade/gliner_base | urchade | "2024-04-10T10:10:19Z" | 112,642 | 66 | gliner | [
"gliner",
"pytorch",
"token-classification",
"en",
"dataset:Universal-NER/Pile-NER-type",
"arxiv:2311.08526",
"license:cc-by-nc-4.0",
"region:us"
] | token-classification | "2024-02-16T20:57:17Z" | ---
license: cc-by-nc-4.0
language:
- en
pipeline_tag: token-classification
datasets:
- Universal-NER/Pile-NER-type
library_name: gliner
---
# Model Card for GLiNER-base
GLiNER is a Named Entity Recognition (NER) model capable of identifying any entity type using a bidirectional transformer encoder (BERT-like). It provides a practical alternative to traditional NER models, which are limited to predefined entities, and Large Language Models (LLMs) that, despite their flexibility, are costly and large for resource-constrained scenarios.
## Links
* Paper: https://arxiv.org/abs/2311.08526
* Repository: https://github.com/urchade/GLiNER
## Available models
| Release | Model Name | # of Parameters | Language | License |
| - | - | - | - | - |
| v0 | [urchade/gliner_base](https://huggingface.co/urchade/gliner_base)<br>[urchade/gliner_multi](https://huggingface.co/urchade/gliner_multi) | 209M<br>209M | English<br>Multilingual | cc-by-nc-4.0 |
| v1 | [urchade/gliner_small-v1](https://huggingface.co/urchade/gliner_small-v1)<br>[urchade/gliner_medium-v1](https://huggingface.co/urchade/gliner_medium-v1)<br>[urchade/gliner_large-v1](https://huggingface.co/urchade/gliner_large-v1) | 166M<br>209M<br>459M | English <br> English <br> English | cc-by-nc-4.0 |
| v2 | [urchade/gliner_small-v2](https://huggingface.co/urchade/gliner_small-v2)<br>[urchade/gliner_medium-v2](https://huggingface.co/urchade/gliner_medium-v2)<br>[urchade/gliner_large-v2](https://huggingface.co/urchade/gliner_large-v2) | 166M<br>209M<br>459M | English <br> English <br> English | apache-2.0 |
| v2.1 | [urchade/gliner_small-v2.1](https://huggingface.co/urchade/gliner_small-v2.1)<br>[urchade/gliner_medium-v2.1](https://huggingface.co/urchade/gliner_medium-v2.1)<br>[urchade/gliner_large-v2.1](https://huggingface.co/urchade/gliner_large-v2.1) <br>[urchade/gliner_multi-v2.1](https://huggingface.co/urchade/gliner_multi-v2.1) | 166M<br>209M<br>459M<br>209M | English <br> English <br> English <br> Multilingual | apache-2.0 |
## Installation
To use this model, you must install the GLiNER Python library:
```
!pip install gliner
```
## Usage
Once you've downloaded the GLiNER library, you can import the GLiNER class. You can then load this model using `GLiNER.from_pretrained` and predict entities with `predict_entities`.
```python
from gliner import GLiNER
model = GLiNER.from_pretrained("urchade/gliner_base")
text = """
Cristiano Ronaldo dos Santos Aveiro (Portuguese pronunciation: [kɾiʃˈtjɐnu ʁɔˈnaldu]; born 5 February 1985) is a Portuguese professional footballer who plays as a forward for and captains both Saudi Pro League club Al Nassr and the Portugal national team. Widely regarded as one of the greatest players of all time, Ronaldo has won five Ballon d'Or awards,[note 3] a record three UEFA Men's Player of the Year Awards, and four European Golden Shoes, the most by a European player. He has won 33 trophies in his career, including seven league titles, five UEFA Champions Leagues, the UEFA European Championship and the UEFA Nations League. Ronaldo holds the records for most appearances (183), goals (140) and assists (42) in the Champions League, goals in the European Championship (14), international goals (128) and international appearances (205). He is one of the few players to have made over 1,200 professional career appearances, the most by an outfield player, and has scored over 850 official senior career goals for club and country, making him the top goalscorer of all time.
"""
labels = ["person", "award", "date", "competitions", "teams"]
entities = model.predict_entities(text, labels)
for entity in entities:
print(entity["text"], "=>", entity["label"])
```
```
Cristiano Ronaldo dos Santos Aveiro => person
5 February 1985 => date
Al Nassr => teams
Portugal national team => teams
Ballon d'Or => award
UEFA Men's Player of the Year Awards => award
European Golden Shoes => award
UEFA Champions Leagues => competitions
UEFA European Championship => competitions
UEFA Nations League => competitions
Champions League => competitions
European Championship => competitions
```
## Named Entity Recognition benchmark result

## Model Authors
The model authors are:
* [Urchade Zaratiana](https://huggingface.co/urchade)
* Nadi Tomeh
* Pierre Holat
* Thierry Charnois
## Citation
```bibtex
@misc{zaratiana2023gliner,
title={GLiNER: Generalist Model for Named Entity Recognition using Bidirectional Transformer},
author={Urchade Zaratiana and Nadi Tomeh and Pierre Holat and Thierry Charnois},
year={2023},
eprint={2311.08526},
archivePrefix={arXiv},
primaryClass={cs.CL}
}
``` |
RichardErkhov/abacusai_-_Smaug-Llama-3-70B-Instruct-gguf | RichardErkhov | "2024-06-27T06:41:36Z" | 112,624 | 0 | null | [
"gguf",
"region:us"
] | null | "2024-06-26T13:41:17Z" | Entry not found |
intfloat/e5-mistral-7b-instruct | intfloat | "2024-04-23T08:03:51Z" | 112,453 | 426 | sentence-transformers | [
"sentence-transformers",
"pytorch",
"safetensors",
"mistral",
"feature-extraction",
"mteb",
"transformers",
"en",
"arxiv:2401.00368",
"arxiv:2104.08663",
"arxiv:2210.07316",
"license:mit",
"model-index",
"autotrain_compatible",
"endpoints_compatible",
"region:us"
] | feature-extraction | "2023-12-20T10:17:02Z" | ---
tags:
- mteb
- sentence-transformers
- transformers
model-index:
- name: e5-mistral-7b-instruct
results:
- task:
type: STS
dataset:
type: C-MTEB/AFQMC
name: MTEB AFQMC
config: default
split: validation
revision: None
metrics:
- type: cos_sim_pearson
value: 37.863226091673866
- type: cos_sim_spearman
value: 38.98733013335281
- type: euclidean_pearson
value: 37.51783380497874
- type: euclidean_spearman
value: 38.98733012753365
- type: manhattan_pearson
value: 37.26706888081721
- type: manhattan_spearman
value: 38.709750161903834
- task:
type: STS
dataset:
type: C-MTEB/ATEC
name: MTEB ATEC
config: default
split: test
revision: None
metrics:
- type: cos_sim_pearson
value: 43.33924583134623
- type: cos_sim_spearman
value: 42.84316155158754
- type: euclidean_pearson
value: 45.62709879515238
- type: euclidean_spearman
value: 42.843155921732404
- type: manhattan_pearson
value: 45.4786950991229
- type: manhattan_spearman
value: 42.657334751855984
- task:
type: Classification
dataset:
type: mteb/amazon_counterfactual
name: MTEB AmazonCounterfactualClassification (en)
config: en
split: test
revision: e8379541af4e31359cca9fbcf4b00f2671dba205
metrics:
- type: accuracy
value: 78.68656716417911
- type: ap
value: 41.71522322900398
- type: f1
value: 72.37207703532552
- task:
type: Classification
dataset:
type: mteb/amazon_counterfactual
name: MTEB AmazonCounterfactualClassification (de)
config: de
split: test
revision: e8379541af4e31359cca9fbcf4b00f2671dba205
metrics:
- type: accuracy
value: 74.04710920770879
- type: ap
value: 83.42622221864045
- type: f1
value: 72.14388257905772
- task:
type: Classification
dataset:
type: mteb/amazon_counterfactual
name: MTEB AmazonCounterfactualClassification (en-ext)
config: en-ext
split: test
revision: e8379541af4e31359cca9fbcf4b00f2671dba205
metrics:
- type: accuracy
value: 77.93103448275862
- type: ap
value: 26.039284760509513
- type: f1
value: 64.81092954450712
- task:
type: Classification
dataset:
type: mteb/amazon_counterfactual
name: MTEB AmazonCounterfactualClassification (ja)
config: ja
split: test
revision: e8379541af4e31359cca9fbcf4b00f2671dba205
metrics:
- type: accuracy
value: 77.21627408993577
- type: ap
value: 24.876490553983036
- type: f1
value: 63.8773359684989
- task:
type: Classification
dataset:
type: mteb/amazon_polarity
name: MTEB AmazonPolarityClassification
config: default
split: test
revision: e2d317d38cd51312af73b3d32a06d1a08b442046
metrics:
- type: accuracy
value: 95.90679999999999
- type: ap
value: 94.32357863164454
- type: f1
value: 95.90485634708557
- task:
type: Classification
dataset:
type: mteb/amazon_reviews_multi
name: MTEB AmazonReviewsClassification (en)
config: en
split: test
revision: 1399c76144fd37290681b995c656ef9b2e06e26d
metrics:
- type: accuracy
value: 55.786
- type: f1
value: 55.31211995815146
- task:
type: Classification
dataset:
type: mteb/amazon_reviews_multi
name: MTEB AmazonReviewsClassification (de)
config: de
split: test
revision: 1399c76144fd37290681b995c656ef9b2e06e26d
metrics:
- type: accuracy
value: 53.26
- type: f1
value: 52.156230111544986
- task:
type: Classification
dataset:
type: mteb/amazon_reviews_multi
name: MTEB AmazonReviewsClassification (es)
config: es
split: test
revision: 1399c76144fd37290681b995c656ef9b2e06e26d
metrics:
- type: accuracy
value: 50.33
- type: f1
value: 49.195023008878145
- task:
type: Classification
dataset:
type: mteb/amazon_reviews_multi
name: MTEB AmazonReviewsClassification (fr)
config: fr
split: test
revision: 1399c76144fd37290681b995c656ef9b2e06e26d
metrics:
- type: accuracy
value: 49.3
- type: f1
value: 48.434470184108
- task:
type: Classification
dataset:
type: mteb/amazon_reviews_multi
name: MTEB AmazonReviewsClassification (ja)
config: ja
split: test
revision: 1399c76144fd37290681b995c656ef9b2e06e26d
metrics:
- type: accuracy
value: 48.68599999999999
- type: f1
value: 47.62681775202072
- task:
type: Classification
dataset:
type: mteb/amazon_reviews_multi
name: MTEB AmazonReviewsClassification (zh)
config: zh
split: test
revision: 1399c76144fd37290681b995c656ef9b2e06e26d
metrics:
- type: accuracy
value: 46.238
- type: f1
value: 45.014030559653705
- task:
type: Retrieval
dataset:
type: arguana
name: MTEB ArguAna
config: default
split: test
revision: None
metrics:
- type: map_at_1
value: 36.486000000000004
- type: map_at_10
value: 53.076
- type: map_at_100
value: 53.657999999999994
- type: map_at_1000
value: 53.659
- type: map_at_3
value: 48.234
- type: map_at_5
value: 51.121
- type: mrr_at_1
value: 37.269000000000005
- type: mrr_at_10
value: 53.335
- type: mrr_at_100
value: 53.916
- type: mrr_at_1000
value: 53.918
- type: mrr_at_3
value: 48.518
- type: mrr_at_5
value: 51.406
- type: ndcg_at_1
value: 36.486000000000004
- type: ndcg_at_10
value: 61.882000000000005
- type: ndcg_at_100
value: 64.165
- type: ndcg_at_1000
value: 64.203
- type: ndcg_at_3
value: 52.049
- type: ndcg_at_5
value: 57.199
- type: precision_at_1
value: 36.486000000000004
- type: precision_at_10
value: 8.982999999999999
- type: precision_at_100
value: 0.9939999999999999
- type: precision_at_1000
value: 0.1
- type: precision_at_3
value: 21.029
- type: precision_at_5
value: 15.092
- type: recall_at_1
value: 36.486000000000004
- type: recall_at_10
value: 89.82900000000001
- type: recall_at_100
value: 99.36
- type: recall_at_1000
value: 99.644
- type: recall_at_3
value: 63.087
- type: recall_at_5
value: 75.46199999999999
- task:
type: Clustering
dataset:
type: mteb/arxiv-clustering-p2p
name: MTEB ArxivClusteringP2P
config: default
split: test
revision: a122ad7f3f0291bf49cc6f4d32aa80929df69d5d
metrics:
- type: v_measure
value: 50.45119266859667
- task:
type: Clustering
dataset:
type: mteb/arxiv-clustering-s2s
name: MTEB ArxivClusteringS2S
config: default
split: test
revision: f910caf1a6075f7329cdf8c1a6135696f37dbd53
metrics:
- type: v_measure
value: 45.4958298992051
- task:
type: Reranking
dataset:
type: mteb/askubuntudupquestions-reranking
name: MTEB AskUbuntuDupQuestions
config: default
split: test
revision: 2000358ca161889fa9c082cb41daa8dcfb161a54
metrics:
- type: map
value: 66.98177472838887
- type: mrr
value: 79.91854636591478
- task:
type: STS
dataset:
type: mteb/biosses-sts
name: MTEB BIOSSES
config: default
split: test
revision: d3fb88f8f02e40887cd149695127462bbcf29b4a
metrics:
- type: cos_sim_pearson
value: 87.67086498650698
- type: cos_sim_spearman
value: 85.54773239564638
- type: euclidean_pearson
value: 86.48229161588425
- type: euclidean_spearman
value: 85.54773239564638
- type: manhattan_pearson
value: 86.67533327742343
- type: manhattan_spearman
value: 85.76099026691983
- task:
type: STS
dataset:
type: C-MTEB/BQ
name: MTEB BQ
config: default
split: test
revision: None
metrics:
- type: cos_sim_pearson
value: 50.31998888922809
- type: cos_sim_spearman
value: 50.6369940530675
- type: euclidean_pearson
value: 50.055544636296055
- type: euclidean_spearman
value: 50.63699405154838
- type: manhattan_pearson
value: 50.00739378036807
- type: manhattan_spearman
value: 50.607237418676945
- task:
type: BitextMining
dataset:
type: mteb/bucc-bitext-mining
name: MTEB BUCC (de-en)
config: de-en
split: test
revision: d51519689f32196a32af33b075a01d0e7c51e252
metrics:
- type: accuracy
value: 99.5615866388309
- type: f1
value: 99.49895615866389
- type: precision
value: 99.46764091858039
- type: recall
value: 99.5615866388309
- task:
type: BitextMining
dataset:
type: mteb/bucc-bitext-mining
name: MTEB BUCC (fr-en)
config: fr-en
split: test
revision: d51519689f32196a32af33b075a01d0e7c51e252
metrics:
- type: accuracy
value: 99.19656614571869
- type: f1
value: 99.08650671362535
- type: precision
value: 99.0314769975787
- type: recall
value: 99.19656614571869
- task:
type: BitextMining
dataset:
type: mteb/bucc-bitext-mining
name: MTEB BUCC (ru-en)
config: ru-en
split: test
revision: d51519689f32196a32af33b075a01d0e7c51e252
metrics:
- type: accuracy
value: 98.0256321440942
- type: f1
value: 97.83743216718624
- type: precision
value: 97.74390947927492
- type: recall
value: 98.0256321440942
- task:
type: BitextMining
dataset:
type: mteb/bucc-bitext-mining
name: MTEB BUCC (zh-en)
config: zh-en
split: test
revision: d51519689f32196a32af33b075a01d0e7c51e252
metrics:
- type: accuracy
value: 99.26276987888363
- type: f1
value: 99.22766368264
- type: precision
value: 99.21011058451816
- type: recall
value: 99.26276987888363
- task:
type: Classification
dataset:
type: mteb/banking77
name: MTEB Banking77Classification
config: default
split: test
revision: 0fd18e25b25c072e09e0d92ab615fda904d66300
metrics:
- type: accuracy
value: 88.22727272727272
- type: f1
value: 88.17411732496673
- task:
type: Clustering
dataset:
type: mteb/biorxiv-clustering-p2p
name: MTEB BiorxivClusteringP2P
config: default
split: test
revision: 65b79d1d13f80053f67aca9498d9402c2d9f1f40
metrics:
- type: v_measure
value: 43.530637846246975
- task:
type: Clustering
dataset:
type: mteb/biorxiv-clustering-s2s
name: MTEB BiorxivClusteringS2S
config: default
split: test
revision: 258694dd0231531bc1fd9de6ceb52a0853c6d908
metrics:
- type: v_measure
value: 40.23505728593893
- task:
type: Clustering
dataset:
type: C-MTEB/CLSClusteringP2P
name: MTEB CLSClusteringP2P
config: default
split: test
revision: None
metrics:
- type: v_measure
value: 44.419028279451275
- task:
type: Clustering
dataset:
type: C-MTEB/CLSClusteringS2S
name: MTEB CLSClusteringS2S
config: default
split: test
revision: None
metrics:
- type: v_measure
value: 42.5820277929776
- task:
type: Reranking
dataset:
type: C-MTEB/CMedQAv1-reranking
name: MTEB CMedQAv1
config: default
split: test
revision: None
metrics:
- type: map
value: 77.67811726152972
- type: mrr
value: 80.99003968253969
- task:
type: Reranking
dataset:
type: C-MTEB/CMedQAv2-reranking
name: MTEB CMedQAv2
config: default
split: test
revision: None
metrics:
- type: map
value: 78.66055354534922
- type: mrr
value: 81.66119047619047
- task:
type: Retrieval
dataset:
type: BeIR/cqadupstack
name: MTEB CQADupstackRetrieval
config: default
split: test
revision: None
metrics:
- type: map_at_1
value: 27.162333333333333
- type: map_at_10
value: 37.22291666666667
- type: map_at_100
value: 38.56733333333333
- type: map_at_1000
value: 38.684250000000006
- type: map_at_3
value: 34.22858333333333
- type: map_at_5
value: 35.852500000000006
- type: mrr_at_1
value: 32.459833333333336
- type: mrr_at_10
value: 41.65358333333333
- type: mrr_at_100
value: 42.566916666666664
- type: mrr_at_1000
value: 42.61766666666667
- type: mrr_at_3
value: 39.210499999999996
- type: mrr_at_5
value: 40.582166666666666
- type: ndcg_at_1
value: 32.459833333333336
- type: ndcg_at_10
value: 42.96758333333333
- type: ndcg_at_100
value: 48.5065
- type: ndcg_at_1000
value: 50.556583333333336
- type: ndcg_at_3
value: 38.004416666666664
- type: ndcg_at_5
value: 40.25916666666667
- type: precision_at_1
value: 32.459833333333336
- type: precision_at_10
value: 7.664583333333333
- type: precision_at_100
value: 1.2349999999999999
- type: precision_at_1000
value: 0.15966666666666668
- type: precision_at_3
value: 17.731166666666663
- type: precision_at_5
value: 12.575333333333335
- type: recall_at_1
value: 27.162333333333333
- type: recall_at_10
value: 55.44158333333334
- type: recall_at_100
value: 79.56966666666666
- type: recall_at_1000
value: 93.45224999999999
- type: recall_at_3
value: 41.433083333333336
- type: recall_at_5
value: 47.31108333333333
- task:
type: Retrieval
dataset:
type: climate-fever
name: MTEB ClimateFEVER
config: default
split: test
revision: None
metrics:
- type: map_at_1
value: 16.539
- type: map_at_10
value: 28.494999999999997
- type: map_at_100
value: 30.568
- type: map_at_1000
value: 30.741000000000003
- type: map_at_3
value: 23.846999999999998
- type: map_at_5
value: 26.275
- type: mrr_at_1
value: 37.394
- type: mrr_at_10
value: 50.068
- type: mrr_at_100
value: 50.727
- type: mrr_at_1000
value: 50.751000000000005
- type: mrr_at_3
value: 46.938
- type: mrr_at_5
value: 48.818
- type: ndcg_at_1
value: 37.394
- type: ndcg_at_10
value: 38.349
- type: ndcg_at_100
value: 45.512
- type: ndcg_at_1000
value: 48.321
- type: ndcg_at_3
value: 32.172
- type: ndcg_at_5
value: 34.265
- type: precision_at_1
value: 37.394
- type: precision_at_10
value: 11.927999999999999
- type: precision_at_100
value: 1.966
- type: precision_at_1000
value: 0.25
- type: precision_at_3
value: 24.126
- type: precision_at_5
value: 18.306
- type: recall_at_1
value: 16.539
- type: recall_at_10
value: 44.504
- type: recall_at_100
value: 68.605
- type: recall_at_1000
value: 84.1
- type: recall_at_3
value: 29.008
- type: recall_at_5
value: 35.58
- task:
type: Retrieval
dataset:
type: C-MTEB/CmedqaRetrieval
name: MTEB CmedqaRetrieval
config: default
split: dev
revision: None
metrics:
- type: map_at_1
value: 19.482
- type: map_at_10
value: 28.622999999999998
- type: map_at_100
value: 30.262
- type: map_at_1000
value: 30.432
- type: map_at_3
value: 25.647
- type: map_at_5
value: 27.128000000000004
- type: mrr_at_1
value: 30.408
- type: mrr_at_10
value: 37.188
- type: mrr_at_100
value: 38.196000000000005
- type: mrr_at_1000
value: 38.273
- type: mrr_at_3
value: 35.067
- type: mrr_at_5
value: 36.124
- type: ndcg_at_1
value: 30.408
- type: ndcg_at_10
value: 34.215
- type: ndcg_at_100
value: 41.349999999999994
- type: ndcg_at_1000
value: 44.689
- type: ndcg_at_3
value: 30.264999999999997
- type: ndcg_at_5
value: 31.572
- type: precision_at_1
value: 30.408
- type: precision_at_10
value: 7.6770000000000005
- type: precision_at_100
value: 1.352
- type: precision_at_1000
value: 0.178
- type: precision_at_3
value: 17.213
- type: precision_at_5
value: 12.198
- type: recall_at_1
value: 19.482
- type: recall_at_10
value: 42.368
- type: recall_at_100
value: 72.694
- type: recall_at_1000
value: 95.602
- type: recall_at_3
value: 30.101
- type: recall_at_5
value: 34.708
- task:
type: PairClassification
dataset:
type: C-MTEB/CMNLI
name: MTEB Cmnli
config: default
split: validation
revision: None
metrics:
- type: cos_sim_accuracy
value: 71.16055321707758
- type: cos_sim_ap
value: 80.21073839711723
- type: cos_sim_f1
value: 72.9740932642487
- type: cos_sim_precision
value: 65.53136050623488
- type: cos_sim_recall
value: 82.3240589198036
- type: dot_accuracy
value: 71.16055321707758
- type: dot_ap
value: 80.212299264122
- type: dot_f1
value: 72.9740932642487
- type: dot_precision
value: 65.53136050623488
- type: dot_recall
value: 82.3240589198036
- type: euclidean_accuracy
value: 71.16055321707758
- type: euclidean_ap
value: 80.21076298680417
- type: euclidean_f1
value: 72.9740932642487
- type: euclidean_precision
value: 65.53136050623488
- type: euclidean_recall
value: 82.3240589198036
- type: manhattan_accuracy
value: 70.71557426337944
- type: manhattan_ap
value: 79.93448977199749
- type: manhattan_f1
value: 72.83962726826877
- type: manhattan_precision
value: 62.7407908077053
- type: manhattan_recall
value: 86.81318681318682
- type: max_accuracy
value: 71.16055321707758
- type: max_ap
value: 80.212299264122
- type: max_f1
value: 72.9740932642487
- task:
type: Retrieval
dataset:
type: C-MTEB/CovidRetrieval
name: MTEB CovidRetrieval
config: default
split: dev
revision: None
metrics:
- type: map_at_1
value: 60.643
- type: map_at_10
value: 69.011
- type: map_at_100
value: 69.533
- type: map_at_1000
value: 69.545
- type: map_at_3
value: 67.167
- type: map_at_5
value: 68.12700000000001
- type: mrr_at_1
value: 60.801
- type: mrr_at_10
value: 69.111
- type: mrr_at_100
value: 69.6
- type: mrr_at_1000
value: 69.611
- type: mrr_at_3
value: 67.229
- type: mrr_at_5
value: 68.214
- type: ndcg_at_1
value: 60.801
- type: ndcg_at_10
value: 73.128
- type: ndcg_at_100
value: 75.614
- type: ndcg_at_1000
value: 75.92
- type: ndcg_at_3
value: 69.261
- type: ndcg_at_5
value: 70.973
- type: precision_at_1
value: 60.801
- type: precision_at_10
value: 8.662
- type: precision_at_100
value: 0.9860000000000001
- type: precision_at_1000
value: 0.101
- type: precision_at_3
value: 25.149
- type: precision_at_5
value: 15.953999999999999
- type: recall_at_1
value: 60.643
- type: recall_at_10
value: 85.959
- type: recall_at_100
value: 97.576
- type: recall_at_1000
value: 100.0
- type: recall_at_3
value: 75.184
- type: recall_at_5
value: 79.32000000000001
- task:
type: Retrieval
dataset:
type: dbpedia-entity
name: MTEB DBPedia
config: default
split: test
revision: None
metrics:
- type: map_at_1
value: 10.183
- type: map_at_10
value: 23.958
- type: map_at_100
value: 34.354
- type: map_at_1000
value: 36.442
- type: map_at_3
value: 16.345000000000002
- type: map_at_5
value: 19.647000000000002
- type: mrr_at_1
value: 74.25
- type: mrr_at_10
value: 80.976
- type: mrr_at_100
value: 81.256
- type: mrr_at_1000
value: 81.262
- type: mrr_at_3
value: 79.958
- type: mrr_at_5
value: 80.37100000000001
- type: ndcg_at_1
value: 62.0
- type: ndcg_at_10
value: 48.894999999999996
- type: ndcg_at_100
value: 53.867
- type: ndcg_at_1000
value: 61.304
- type: ndcg_at_3
value: 53.688
- type: ndcg_at_5
value: 50.900999999999996
- type: precision_at_1
value: 74.25
- type: precision_at_10
value: 39.525
- type: precision_at_100
value: 12.323
- type: precision_at_1000
value: 2.539
- type: precision_at_3
value: 57.49999999999999
- type: precision_at_5
value: 49.1
- type: recall_at_1
value: 10.183
- type: recall_at_10
value: 29.296
- type: recall_at_100
value: 60.394999999999996
- type: recall_at_1000
value: 83.12
- type: recall_at_3
value: 17.495
- type: recall_at_5
value: 22.235
- task:
type: Retrieval
dataset:
type: C-MTEB/DuRetrieval
name: MTEB DuRetrieval
config: default
split: dev
revision: None
metrics:
- type: map_at_1
value: 26.613999999999997
- type: map_at_10
value: 79.77300000000001
- type: map_at_100
value: 82.71
- type: map_at_1000
value: 82.75
- type: map_at_3
value: 55.92700000000001
- type: map_at_5
value: 70.085
- type: mrr_at_1
value: 90.7
- type: mrr_at_10
value: 93.438
- type: mrr_at_100
value: 93.504
- type: mrr_at_1000
value: 93.50699999999999
- type: mrr_at_3
value: 93.125
- type: mrr_at_5
value: 93.34
- type: ndcg_at_1
value: 90.7
- type: ndcg_at_10
value: 87.023
- type: ndcg_at_100
value: 90.068
- type: ndcg_at_1000
value: 90.43299999999999
- type: ndcg_at_3
value: 86.339
- type: ndcg_at_5
value: 85.013
- type: precision_at_1
value: 90.7
- type: precision_at_10
value: 41.339999999999996
- type: precision_at_100
value: 4.806
- type: precision_at_1000
value: 0.48900000000000005
- type: precision_at_3
value: 76.983
- type: precision_at_5
value: 64.69
- type: recall_at_1
value: 26.613999999999997
- type: recall_at_10
value: 87.681
- type: recall_at_100
value: 97.44699999999999
- type: recall_at_1000
value: 99.348
- type: recall_at_3
value: 57.809999999999995
- type: recall_at_5
value: 74.258
- task:
type: Retrieval
dataset:
type: C-MTEB/EcomRetrieval
name: MTEB EcomRetrieval
config: default
split: dev
revision: None
metrics:
- type: map_at_1
value: 30.9
- type: map_at_10
value: 40.467
- type: map_at_100
value: 41.423
- type: map_at_1000
value: 41.463
- type: map_at_3
value: 37.25
- type: map_at_5
value: 39.31
- type: mrr_at_1
value: 30.9
- type: mrr_at_10
value: 40.467
- type: mrr_at_100
value: 41.423
- type: mrr_at_1000
value: 41.463
- type: mrr_at_3
value: 37.25
- type: mrr_at_5
value: 39.31
- type: ndcg_at_1
value: 30.9
- type: ndcg_at_10
value: 45.957
- type: ndcg_at_100
value: 50.735
- type: ndcg_at_1000
value: 51.861999999999995
- type: ndcg_at_3
value: 39.437
- type: ndcg_at_5
value: 43.146
- type: precision_at_1
value: 30.9
- type: precision_at_10
value: 6.35
- type: precision_at_100
value: 0.861
- type: precision_at_1000
value: 0.095
- type: precision_at_3
value: 15.267
- type: precision_at_5
value: 10.96
- type: recall_at_1
value: 30.9
- type: recall_at_10
value: 63.5
- type: recall_at_100
value: 86.1
- type: recall_at_1000
value: 95.1
- type: recall_at_3
value: 45.800000000000004
- type: recall_at_5
value: 54.800000000000004
- task:
type: Classification
dataset:
type: mteb/emotion
name: MTEB EmotionClassification
config: default
split: test
revision: 4f58c6b202a23cf9a4da393831edf4f9183cad37
metrics:
- type: accuracy
value: 49.765
- type: f1
value: 45.93242203574485
- task:
type: Retrieval
dataset:
type: fever
name: MTEB FEVER
config: default
split: test
revision: None
metrics:
- type: map_at_1
value: 75.138
- type: map_at_10
value: 84.21300000000001
- type: map_at_100
value: 84.43
- type: map_at_1000
value: 84.441
- type: map_at_3
value: 83.071
- type: map_at_5
value: 83.853
- type: mrr_at_1
value: 80.948
- type: mrr_at_10
value: 88.175
- type: mrr_at_100
value: 88.24
- type: mrr_at_1000
value: 88.241
- type: mrr_at_3
value: 87.516
- type: mrr_at_5
value: 87.997
- type: ndcg_at_1
value: 80.948
- type: ndcg_at_10
value: 87.84100000000001
- type: ndcg_at_100
value: 88.576
- type: ndcg_at_1000
value: 88.75699999999999
- type: ndcg_at_3
value: 86.176
- type: ndcg_at_5
value: 87.214
- type: precision_at_1
value: 80.948
- type: precision_at_10
value: 10.632
- type: precision_at_100
value: 1.123
- type: precision_at_1000
value: 0.11499999999999999
- type: precision_at_3
value: 33.193
- type: precision_at_5
value: 20.663
- type: recall_at_1
value: 75.138
- type: recall_at_10
value: 94.89699999999999
- type: recall_at_100
value: 97.751
- type: recall_at_1000
value: 98.833
- type: recall_at_3
value: 90.455
- type: recall_at_5
value: 93.085
- task:
type: Retrieval
dataset:
type: fiqa
name: MTEB FiQA2018
config: default
split: test
revision: None
metrics:
- type: map_at_1
value: 29.45
- type: map_at_10
value: 48.596000000000004
- type: map_at_100
value: 50.70400000000001
- type: map_at_1000
value: 50.83800000000001
- type: map_at_3
value: 42.795
- type: map_at_5
value: 46.085
- type: mrr_at_1
value: 56.172999999999995
- type: mrr_at_10
value: 64.35300000000001
- type: mrr_at_100
value: 64.947
- type: mrr_at_1000
value: 64.967
- type: mrr_at_3
value: 62.653999999999996
- type: mrr_at_5
value: 63.534
- type: ndcg_at_1
value: 56.172999999999995
- type: ndcg_at_10
value: 56.593
- type: ndcg_at_100
value: 62.942
- type: ndcg_at_1000
value: 64.801
- type: ndcg_at_3
value: 53.024
- type: ndcg_at_5
value: 53.986999999999995
- type: precision_at_1
value: 56.172999999999995
- type: precision_at_10
value: 15.494
- type: precision_at_100
value: 2.222
- type: precision_at_1000
value: 0.254
- type: precision_at_3
value: 35.185
- type: precision_at_5
value: 25.556
- type: recall_at_1
value: 29.45
- type: recall_at_10
value: 62.882000000000005
- type: recall_at_100
value: 85.56099999999999
- type: recall_at_1000
value: 96.539
- type: recall_at_3
value: 47.911
- type: recall_at_5
value: 54.52
- task:
type: Retrieval
dataset:
type: hotpotqa
name: MTEB HotpotQA
config: default
split: test
revision: None
metrics:
- type: map_at_1
value: 39.581
- type: map_at_10
value: 68.401
- type: map_at_100
value: 69.207
- type: map_at_1000
value: 69.25200000000001
- type: map_at_3
value: 64.689
- type: map_at_5
value: 67.158
- type: mrr_at_1
value: 79.163
- type: mrr_at_10
value: 85.22999999999999
- type: mrr_at_100
value: 85.386
- type: mrr_at_1000
value: 85.39099999999999
- type: mrr_at_3
value: 84.432
- type: mrr_at_5
value: 84.952
- type: ndcg_at_1
value: 79.163
- type: ndcg_at_10
value: 75.721
- type: ndcg_at_100
value: 78.411
- type: ndcg_at_1000
value: 79.23599999999999
- type: ndcg_at_3
value: 70.68799999999999
- type: ndcg_at_5
value: 73.694
- type: precision_at_1
value: 79.163
- type: precision_at_10
value: 16.134
- type: precision_at_100
value: 1.821
- type: precision_at_1000
value: 0.193
- type: precision_at_3
value: 46.446
- type: precision_at_5
value: 30.242
- type: recall_at_1
value: 39.581
- type: recall_at_10
value: 80.66799999999999
- type: recall_at_100
value: 91.033
- type: recall_at_1000
value: 96.408
- type: recall_at_3
value: 69.669
- type: recall_at_5
value: 75.604
- task:
type: Classification
dataset:
type: C-MTEB/IFlyTek-classification
name: MTEB IFlyTek
config: default
split: validation
revision: None
metrics:
- type: accuracy
value: 45.04809542131589
- type: f1
value: 37.01181779071118
- task:
type: Classification
dataset:
type: mteb/imdb
name: MTEB ImdbClassification
config: default
split: test
revision: 3d86128a09e091d6018b6d26cad27f2739fc2db7
metrics:
- type: accuracy
value: 94.78120000000001
- type: ap
value: 92.52931921594387
- type: f1
value: 94.77902110732532
- task:
type: Classification
dataset:
type: C-MTEB/JDReview-classification
name: MTEB JDReview
config: default
split: test
revision: None
metrics:
- type: accuracy
value: 85.81613508442777
- type: ap
value: 52.430320593468394
- type: f1
value: 79.95467268178068
- task:
type: STS
dataset:
type: C-MTEB/LCQMC
name: MTEB LCQMC
config: default
split: test
revision: None
metrics:
- type: cos_sim_pearson
value: 71.05801751913393
- type: cos_sim_spearman
value: 75.47954644971965
- type: euclidean_pearson
value: 74.27472296759713
- type: euclidean_spearman
value: 75.47954201369866
- type: manhattan_pearson
value: 74.30508190186474
- type: manhattan_spearman
value: 75.51326518159436
- task:
type: Reranking
dataset:
type: C-MTEB/Mmarco-reranking
name: MTEB MMarcoReranking
config: default
split: dev
revision: None
metrics:
- type: map
value: 24.21110921666315
- type: mrr
value: 22.863492063492064
- task:
type: Retrieval
dataset:
type: C-MTEB/MMarcoRetrieval
name: MTEB MMarcoRetrieval
config: default
split: dev
revision: None
metrics:
- type: map_at_1
value: 61.38400000000001
- type: map_at_10
value: 70.895
- type: map_at_100
value: 71.314
- type: map_at_1000
value: 71.331
- type: map_at_3
value: 69.016
- type: map_at_5
value: 70.179
- type: mrr_at_1
value: 63.481
- type: mrr_at_10
value: 71.543
- type: mrr_at_100
value: 71.91300000000001
- type: mrr_at_1000
value: 71.928
- type: mrr_at_3
value: 69.90899999999999
- type: mrr_at_5
value: 70.907
- type: ndcg_at_1
value: 63.481
- type: ndcg_at_10
value: 74.833
- type: ndcg_at_100
value: 76.705
- type: ndcg_at_1000
value: 77.13600000000001
- type: ndcg_at_3
value: 71.236
- type: ndcg_at_5
value: 73.199
- type: precision_at_1
value: 63.481
- type: precision_at_10
value: 9.179
- type: precision_at_100
value: 1.011
- type: precision_at_1000
value: 0.105
- type: precision_at_3
value: 27.044
- type: precision_at_5
value: 17.272000000000002
- type: recall_at_1
value: 61.38400000000001
- type: recall_at_10
value: 86.318
- type: recall_at_100
value: 94.786
- type: recall_at_1000
value: 98.14500000000001
- type: recall_at_3
value: 76.717
- type: recall_at_5
value: 81.416
- task:
type: Retrieval
dataset:
type: msmarco
name: MTEB MSMARCO
config: default
split: dev
revision: None
metrics:
- type: map_at_1
value: 23.363999999999997
- type: map_at_10
value: 36.022
- type: map_at_100
value: 37.229
- type: map_at_1000
value: 37.274
- type: map_at_3
value: 32.131
- type: map_at_5
value: 34.391
- type: mrr_at_1
value: 24.069
- type: mrr_at_10
value: 36.620000000000005
- type: mrr_at_100
value: 37.769999999999996
- type: mrr_at_1000
value: 37.809
- type: mrr_at_3
value: 32.846
- type: mrr_at_5
value: 35.02
- type: ndcg_at_1
value: 24.069
- type: ndcg_at_10
value: 43.056
- type: ndcg_at_100
value: 48.754
- type: ndcg_at_1000
value: 49.829
- type: ndcg_at_3
value: 35.167
- type: ndcg_at_5
value: 39.168
- type: precision_at_1
value: 24.069
- type: precision_at_10
value: 6.762
- type: precision_at_100
value: 0.96
- type: precision_at_1000
value: 0.105
- type: precision_at_3
value: 14.957
- type: precision_at_5
value: 11.023
- type: recall_at_1
value: 23.363999999999997
- type: recall_at_10
value: 64.696
- type: recall_at_100
value: 90.795
- type: recall_at_1000
value: 98.892
- type: recall_at_3
value: 43.247
- type: recall_at_5
value: 52.86300000000001
- task:
type: Classification
dataset:
type: mteb/mtop_domain
name: MTEB MTOPDomainClassification (en)
config: en
split: test
revision: d80d48c1eb48d3562165c59d59d0034df9fff0bf
metrics:
- type: accuracy
value: 96.11947104423166
- type: f1
value: 95.89561841159332
- task:
type: Classification
dataset:
type: mteb/mtop_domain
name: MTEB MTOPDomainClassification (de)
config: de
split: test
revision: d80d48c1eb48d3562165c59d59d0034df9fff0bf
metrics:
- type: accuracy
value: 92.97548605240912
- type: f1
value: 92.17133696717212
- task:
type: Classification
dataset:
type: mteb/mtop_domain
name: MTEB MTOPDomainClassification (es)
config: es
split: test
revision: d80d48c1eb48d3562165c59d59d0034df9fff0bf
metrics:
- type: accuracy
value: 93.37224816544364
- type: f1
value: 93.19978829237863
- task:
type: Classification
dataset:
type: mteb/mtop_domain
name: MTEB MTOPDomainClassification (fr)
config: fr
split: test
revision: d80d48c1eb48d3562165c59d59d0034df9fff0bf
metrics:
- type: accuracy
value: 91.28719072972127
- type: f1
value: 91.28448045979604
- task:
type: Classification
dataset:
type: mteb/mtop_domain
name: MTEB MTOPDomainClassification (hi)
config: hi
split: test
revision: d80d48c1eb48d3562165c59d59d0034df9fff0bf
metrics:
- type: accuracy
value: 88.8131946934385
- type: f1
value: 88.27883019362747
- task:
type: Classification
dataset:
type: mteb/mtop_domain
name: MTEB MTOPDomainClassification (th)
config: th
split: test
revision: d80d48c1eb48d3562165c59d59d0034df9fff0bf
metrics:
- type: accuracy
value: 85.52260397830018
- type: f1
value: 85.15528226728568
- task:
type: Classification
dataset:
type: mteb/mtop_intent
name: MTEB MTOPIntentClassification (en)
config: en
split: test
revision: ae001d0e6b1228650b7bd1c2c65fb50ad11a8aba
metrics:
- type: accuracy
value: 86.10807113543093
- type: f1
value: 70.88498219072167
- task:
type: Classification
dataset:
type: mteb/mtop_intent
name: MTEB MTOPIntentClassification (de)
config: de
split: test
revision: ae001d0e6b1228650b7bd1c2c65fb50ad11a8aba
metrics:
- type: accuracy
value: 77.77120315581854
- type: f1
value: 57.97153920153224
- task:
type: Classification
dataset:
type: mteb/mtop_intent
name: MTEB MTOPIntentClassification (es)
config: es
split: test
revision: ae001d0e6b1228650b7bd1c2c65fb50ad11a8aba
metrics:
- type: accuracy
value: 79.93995997331554
- type: f1
value: 58.839203810064866
- task:
type: Classification
dataset:
type: mteb/mtop_intent
name: MTEB MTOPIntentClassification (fr)
config: fr
split: test
revision: ae001d0e6b1228650b7bd1c2c65fb50ad11a8aba
metrics:
- type: accuracy
value: 77.801440651425
- type: f1
value: 58.68009647839332
- task:
type: Classification
dataset:
type: mteb/mtop_intent
name: MTEB MTOPIntentClassification (hi)
config: hi
split: test
revision: ae001d0e6b1228650b7bd1c2c65fb50ad11a8aba
metrics:
- type: accuracy
value: 72.90785227680172
- type: f1
value: 49.83760954655788
- task:
type: Classification
dataset:
type: mteb/mtop_intent
name: MTEB MTOPIntentClassification (th)
config: th
split: test
revision: ae001d0e6b1228650b7bd1c2c65fb50ad11a8aba
metrics:
- type: accuracy
value: 73.24050632911391
- type: f1
value: 52.0562553541082
- task:
type: Classification
dataset:
type: mteb/amazon_massive_intent
name: MTEB MassiveIntentClassification (af)
config: af
split: test
revision: 31efe3c427b0bae9c22cbb560b8f15491cc6bed7
metrics:
- type: accuracy
value: 66.47948890383321
- type: f1
value: 63.334877563135485
- task:
type: Classification
dataset:
type: mteb/amazon_massive_intent
name: MTEB MassiveIntentClassification (am)
config: am
split: test
revision: 31efe3c427b0bae9c22cbb560b8f15491cc6bed7
metrics:
- type: accuracy
value: 44.2871553463349
- type: f1
value: 43.17658050605427
- task:
type: Classification
dataset:
type: mteb/amazon_massive_intent
name: MTEB MassiveIntentClassification (ar)
config: ar
split: test
revision: 31efe3c427b0bae9c22cbb560b8f15491cc6bed7
metrics:
- type: accuracy
value: 63.174176193678555
- type: f1
value: 59.236659587042425
- task:
type: Classification
dataset:
type: mteb/amazon_massive_intent
name: MTEB MassiveIntentClassification (az)
config: az
split: test
revision: 31efe3c427b0bae9c22cbb560b8f15491cc6bed7
metrics:
- type: accuracy
value: 64.226630800269
- type: f1
value: 60.951842696956184
- task:
type: Classification
dataset:
type: mteb/amazon_massive_intent
name: MTEB MassiveIntentClassification (bn)
config: bn
split: test
revision: 31efe3c427b0bae9c22cbb560b8f15491cc6bed7
metrics:
- type: accuracy
value: 64.94283792871555
- type: f1
value: 61.40057652844215
- task:
type: Classification
dataset:
type: mteb/amazon_massive_intent
name: MTEB MassiveIntentClassification (cy)
config: cy
split: test
revision: 31efe3c427b0bae9c22cbb560b8f15491cc6bed7
metrics:
- type: accuracy
value: 55.480833893745796
- type: f1
value: 52.5298332072816
- task:
type: Classification
dataset:
type: mteb/amazon_massive_intent
name: MTEB MassiveIntentClassification (da)
config: da
split: test
revision: 31efe3c427b0bae9c22cbb560b8f15491cc6bed7
metrics:
- type: accuracy
value: 72.52858103564223
- type: f1
value: 69.3770851919204
- task:
type: Classification
dataset:
type: mteb/amazon_massive_intent
name: MTEB MassiveIntentClassification (de)
config: de
split: test
revision: 31efe3c427b0bae9c22cbb560b8f15491cc6bed7
metrics:
- type: accuracy
value: 74.09213180901143
- type: f1
value: 71.13518469365879
- task:
type: Classification
dataset:
type: mteb/amazon_massive_intent
name: MTEB MassiveIntentClassification (el)
config: el
split: test
revision: 31efe3c427b0bae9c22cbb560b8f15491cc6bed7
metrics:
- type: accuracy
value: 68.31203765971756
- type: f1
value: 66.05906970865144
- task:
type: Classification
dataset:
type: mteb/amazon_massive_intent
name: MTEB MassiveIntentClassification (en)
config: en
split: test
revision: 31efe3c427b0bae9c22cbb560b8f15491cc6bed7
metrics:
- type: accuracy
value: 80.57162071284465
- type: f1
value: 77.7866172598823
- task:
type: Classification
dataset:
type: mteb/amazon_massive_intent
name: MTEB MassiveIntentClassification (es)
config: es
split: test
revision: 31efe3c427b0bae9c22cbb560b8f15491cc6bed7
metrics:
- type: accuracy
value: 75.09414929388029
- type: f1
value: 72.5712594833695
- task:
type: Classification
dataset:
type: mteb/amazon_massive_intent
name: MTEB MassiveIntentClassification (fa)
config: fa
split: test
revision: 31efe3c427b0bae9c22cbb560b8f15491cc6bed7
metrics:
- type: accuracy
value: 72.20914593140553
- type: f1
value: 68.90619124909186
- task:
type: Classification
dataset:
type: mteb/amazon_massive_intent
name: MTEB MassiveIntentClassification (fi)
config: fi
split: test
revision: 31efe3c427b0bae9c22cbb560b8f15491cc6bed7
metrics:
- type: accuracy
value: 68.74243443174176
- type: f1
value: 64.72743141749955
- task:
type: Classification
dataset:
type: mteb/amazon_massive_intent
name: MTEB MassiveIntentClassification (fr)
config: fr
split: test
revision: 31efe3c427b0bae9c22cbb560b8f15491cc6bed7
metrics:
- type: accuracy
value: 75.11096166778749
- type: f1
value: 72.61849933064694
- task:
type: Classification
dataset:
type: mteb/amazon_massive_intent
name: MTEB MassiveIntentClassification (he)
config: he
split: test
revision: 31efe3c427b0bae9c22cbb560b8f15491cc6bed7
metrics:
- type: accuracy
value: 66.22394082044384
- type: f1
value: 62.43648797607235
- task:
type: Classification
dataset:
type: mteb/amazon_massive_intent
name: MTEB MassiveIntentClassification (hi)
config: hi
split: test
revision: 31efe3c427b0bae9c22cbb560b8f15491cc6bed7
metrics:
- type: accuracy
value: 69.44855413584399
- type: f1
value: 66.56851670913659
- task:
type: Classification
dataset:
type: mteb/amazon_massive_intent
name: MTEB MassiveIntentClassification (hu)
config: hu
split: test
revision: 31efe3c427b0bae9c22cbb560b8f15491cc6bed7
metrics:
- type: accuracy
value: 69.4149293880296
- type: f1
value: 66.12960877904776
- task:
type: Classification
dataset:
type: mteb/amazon_massive_intent
name: MTEB MassiveIntentClassification (hy)
config: hy
split: test
revision: 31efe3c427b0bae9c22cbb560b8f15491cc6bed7
metrics:
- type: accuracy
value: 56.916610625420304
- type: f1
value: 54.02534600927991
- task:
type: Classification
dataset:
type: mteb/amazon_massive_intent
name: MTEB MassiveIntentClassification (id)
config: id
split: test
revision: 31efe3c427b0bae9c22cbb560b8f15491cc6bed7
metrics:
- type: accuracy
value: 72.71351714862138
- type: f1
value: 69.70227985126316
- task:
type: Classification
dataset:
type: mteb/amazon_massive_intent
name: MTEB MassiveIntentClassification (is)
config: is
split: test
revision: 31efe3c427b0bae9c22cbb560b8f15491cc6bed7
metrics:
- type: accuracy
value: 59.91257565568257
- type: f1
value: 57.06811572144974
- task:
type: Classification
dataset:
type: mteb/amazon_massive_intent
name: MTEB MassiveIntentClassification (it)
config: it
split: test
revision: 31efe3c427b0bae9c22cbb560b8f15491cc6bed7
metrics:
- type: accuracy
value: 75.25218560860793
- type: f1
value: 72.48057563104247
- task:
type: Classification
dataset:
type: mteb/amazon_massive_intent
name: MTEB MassiveIntentClassification (ja)
config: ja
split: test
revision: 31efe3c427b0bae9c22cbb560b8f15491cc6bed7
metrics:
- type: accuracy
value: 76.35507733691998
- type: f1
value: 73.03024649541128
- task:
type: Classification
dataset:
type: mteb/amazon_massive_intent
name: MTEB MassiveIntentClassification (jv)
config: jv
split: test
revision: 31efe3c427b0bae9c22cbb560b8f15491cc6bed7
metrics:
- type: accuracy
value: 57.918628110289184
- type: f1
value: 54.75590124456177
- task:
type: Classification
dataset:
type: mteb/amazon_massive_intent
name: MTEB MassiveIntentClassification (ka)
config: ka
split: test
revision: 31efe3c427b0bae9c22cbb560b8f15491cc6bed7
metrics:
- type: accuracy
value: 52.548755884330866
- type: f1
value: 51.5356975360209
- task:
type: Classification
dataset:
type: mteb/amazon_massive_intent
name: MTEB MassiveIntentClassification (km)
config: km
split: test
revision: 31efe3c427b0bae9c22cbb560b8f15491cc6bed7
metrics:
- type: accuracy
value: 46.44922663080027
- type: f1
value: 44.561114416830975
- task:
type: Classification
dataset:
type: mteb/amazon_massive_intent
name: MTEB MassiveIntentClassification (kn)
config: kn
split: test
revision: 31efe3c427b0bae9c22cbb560b8f15491cc6bed7
metrics:
- type: accuracy
value: 53.95763281775386
- type: f1
value: 50.68367245122476
- task:
type: Classification
dataset:
type: mteb/amazon_massive_intent
name: MTEB MassiveIntentClassification (ko)
config: ko
split: test
revision: 31efe3c427b0bae9c22cbb560b8f15491cc6bed7
metrics:
- type: accuracy
value: 74.20645595158035
- type: f1
value: 71.78450093258185
- task:
type: Classification
dataset:
type: mteb/amazon_massive_intent
name: MTEB MassiveIntentClassification (lv)
config: lv
split: test
revision: 31efe3c427b0bae9c22cbb560b8f15491cc6bed7
metrics:
- type: accuracy
value: 59.226630800269
- type: f1
value: 57.53988988993337
- task:
type: Classification
dataset:
type: mteb/amazon_massive_intent
name: MTEB MassiveIntentClassification (ml)
config: ml
split: test
revision: 31efe3c427b0bae9c22cbb560b8f15491cc6bed7
metrics:
- type: accuracy
value: 51.44922663080027
- type: f1
value: 48.58809018065056
- task:
type: Classification
dataset:
type: mteb/amazon_massive_intent
name: MTEB MassiveIntentClassification (mn)
config: mn
split: test
revision: 31efe3c427b0bae9c22cbb560b8f15491cc6bed7
metrics:
- type: accuracy
value: 51.3752521856086
- type: f1
value: 49.91373941436425
- task:
type: Classification
dataset:
type: mteb/amazon_massive_intent
name: MTEB MassiveIntentClassification (ms)
config: ms
split: test
revision: 31efe3c427b0bae9c22cbb560b8f15491cc6bed7
metrics:
- type: accuracy
value: 69.85205110961668
- type: f1
value: 67.05660019588582
- task:
type: Classification
dataset:
type: mteb/amazon_massive_intent
name: MTEB MassiveIntentClassification (my)
config: my
split: test
revision: 31efe3c427b0bae9c22cbb560b8f15491cc6bed7
metrics:
- type: accuracy
value: 49.1492938802959
- type: f1
value: 46.717578025393195
- task:
type: Classification
dataset:
type: mteb/amazon_massive_intent
name: MTEB MassiveIntentClassification (nb)
config: nb
split: test
revision: 31efe3c427b0bae9c22cbb560b8f15491cc6bed7
metrics:
- type: accuracy
value: 70.93140551445865
- type: f1
value: 67.45406609372205
- task:
type: Classification
dataset:
type: mteb/amazon_massive_intent
name: MTEB MassiveIntentClassification (nl)
config: nl
split: test
revision: 31efe3c427b0bae9c22cbb560b8f15491cc6bed7
metrics:
- type: accuracy
value: 74.82851378614662
- type: f1
value: 71.15951964393868
- task:
type: Classification
dataset:
type: mteb/amazon_massive_intent
name: MTEB MassiveIntentClassification (pl)
config: pl
split: test
revision: 31efe3c427b0bae9c22cbb560b8f15491cc6bed7
metrics:
- type: accuracy
value: 74.84868863483524
- type: f1
value: 71.76056802364877
- task:
type: Classification
dataset:
type: mteb/amazon_massive_intent
name: MTEB MassiveIntentClassification (pt)
config: pt
split: test
revision: 31efe3c427b0bae9c22cbb560b8f15491cc6bed7
metrics:
- type: accuracy
value: 75.27236045729657
- type: f1
value: 72.48733090101163
- task:
type: Classification
dataset:
type: mteb/amazon_massive_intent
name: MTEB MassiveIntentClassification (ro)
config: ro
split: test
revision: 31efe3c427b0bae9c22cbb560b8f15491cc6bed7
metrics:
- type: accuracy
value: 69.63012777404168
- type: f1
value: 66.56444015346203
- task:
type: Classification
dataset:
type: mteb/amazon_massive_intent
name: MTEB MassiveIntentClassification (ru)
config: ru
split: test
revision: 31efe3c427b0bae9c22cbb560b8f15491cc6bed7
metrics:
- type: accuracy
value: 76.62743779421655
- type: f1
value: 73.82720656992142
- task:
type: Classification
dataset:
type: mteb/amazon_massive_intent
name: MTEB MassiveIntentClassification (sl)
config: sl
split: test
revision: 31efe3c427b0bae9c22cbb560b8f15491cc6bed7
metrics:
- type: accuracy
value: 67.15198386012105
- type: f1
value: 64.41418309797744
- task:
type: Classification
dataset:
type: mteb/amazon_massive_intent
name: MTEB MassiveIntentClassification (sq)
config: sq
split: test
revision: 31efe3c427b0bae9c22cbb560b8f15491cc6bed7
metrics:
- type: accuracy
value: 58.8399462004035
- type: f1
value: 56.050989519693886
- task:
type: Classification
dataset:
type: mteb/amazon_massive_intent
name: MTEB MassiveIntentClassification (sv)
config: sv
split: test
revision: 31efe3c427b0bae9c22cbb560b8f15491cc6bed7
metrics:
- type: accuracy
value: 73.86684599865501
- type: f1
value: 70.80682480844303
- task:
type: Classification
dataset:
type: mteb/amazon_massive_intent
name: MTEB MassiveIntentClassification (sw)
config: sw
split: test
revision: 31efe3c427b0bae9c22cbb560b8f15491cc6bed7
metrics:
- type: accuracy
value: 57.36718224613316
- type: f1
value: 54.998746471013774
- task:
type: Classification
dataset:
type: mteb/amazon_massive_intent
name: MTEB MassiveIntentClassification (ta)
config: ta
split: test
revision: 31efe3c427b0bae9c22cbb560b8f15491cc6bed7
metrics:
- type: accuracy
value: 53.150638870208475
- type: f1
value: 49.79179342620099
- task:
type: Classification
dataset:
type: mteb/amazon_massive_intent
name: MTEB MassiveIntentClassification (te)
config: te
split: test
revision: 31efe3c427b0bae9c22cbb560b8f15491cc6bed7
metrics:
- type: accuracy
value: 51.50638870208473
- type: f1
value: 49.778960742003555
- task:
type: Classification
dataset:
type: mteb/amazon_massive_intent
name: MTEB MassiveIntentClassification (th)
config: th
split: test
revision: 31efe3c427b0bae9c22cbb560b8f15491cc6bed7
metrics:
- type: accuracy
value: 66.906523201076
- type: f1
value: 66.75784022138245
- task:
type: Classification
dataset:
type: mteb/amazon_massive_intent
name: MTEB MassiveIntentClassification (tl)
config: tl
split: test
revision: 31efe3c427b0bae9c22cbb560b8f15491cc6bed7
metrics:
- type: accuracy
value: 68.73234700739744
- type: f1
value: 65.75016141148413
- task:
type: Classification
dataset:
type: mteb/amazon_massive_intent
name: MTEB MassiveIntentClassification (tr)
config: tr
split: test
revision: 31efe3c427b0bae9c22cbb560b8f15491cc6bed7
metrics:
- type: accuracy
value: 72.06792199058508
- type: f1
value: 67.90334782594083
- task:
type: Classification
dataset:
type: mteb/amazon_massive_intent
name: MTEB MassiveIntentClassification (ur)
config: ur
split: test
revision: 31efe3c427b0bae9c22cbb560b8f15491cc6bed7
metrics:
- type: accuracy
value: 62.09145931405515
- type: f1
value: 58.88703095210731
- task:
type: Classification
dataset:
type: mteb/amazon_massive_intent
name: MTEB MassiveIntentClassification (vi)
config: vi
split: test
revision: 31efe3c427b0bae9c22cbb560b8f15491cc6bed7
metrics:
- type: accuracy
value: 71.17014122394083
- type: f1
value: 68.43676277921544
- task:
type: Classification
dataset:
type: mteb/amazon_massive_intent
name: MTEB MassiveIntentClassification (zh-CN)
config: zh-CN
split: test
revision: 31efe3c427b0bae9c22cbb560b8f15491cc6bed7
metrics:
- type: accuracy
value: 74.99327505043712
- type: f1
value: 72.26813373392943
- task:
type: Classification
dataset:
type: mteb/amazon_massive_intent
name: MTEB MassiveIntentClassification (zh-TW)
config: zh-TW
split: test
revision: 31efe3c427b0bae9c22cbb560b8f15491cc6bed7
metrics:
- type: accuracy
value: 71.13987895090787
- type: f1
value: 70.29309514467575
- task:
type: Classification
dataset:
type: mteb/amazon_massive_scenario
name: MTEB MassiveScenarioClassification (af)
config: af
split: test
revision: 7d571f92784cd94a019292a1f45445077d0ef634
metrics:
- type: accuracy
value: 73.37256220578345
- type: f1
value: 72.56456170538992
- task:
type: Classification
dataset:
type: mteb/amazon_massive_scenario
name: MTEB MassiveScenarioClassification (am)
config: am
split: test
revision: 7d571f92784cd94a019292a1f45445077d0ef634
metrics:
- type: accuracy
value: 47.205783456624076
- type: f1
value: 45.905999859074434
- task:
type: Classification
dataset:
type: mteb/amazon_massive_scenario
name: MTEB MassiveScenarioClassification (ar)
config: ar
split: test
revision: 7d571f92784cd94a019292a1f45445077d0ef634
metrics:
- type: accuracy
value: 69.8352387357095
- type: f1
value: 69.43553987525273
- task:
type: Classification
dataset:
type: mteb/amazon_massive_scenario
name: MTEB MassiveScenarioClassification (az)
config: az
split: test
revision: 7d571f92784cd94a019292a1f45445077d0ef634
metrics:
- type: accuracy
value: 67.00403496973773
- type: f1
value: 65.97477215779143
- task:
type: Classification
dataset:
type: mteb/amazon_massive_scenario
name: MTEB MassiveScenarioClassification (bn)
config: bn
split: test
revision: 7d571f92784cd94a019292a1f45445077d0ef634
metrics:
- type: accuracy
value: 68.04976462676531
- type: f1
value: 67.24581993778398
- task:
type: Classification
dataset:
type: mteb/amazon_massive_scenario
name: MTEB MassiveScenarioClassification (cy)
config: cy
split: test
revision: 7d571f92784cd94a019292a1f45445077d0ef634
metrics:
- type: accuracy
value: 61.882985877605925
- type: f1
value: 59.995293199988794
- task:
type: Classification
dataset:
type: mteb/amazon_massive_scenario
name: MTEB MassiveScenarioClassification (da)
config: da
split: test
revision: 7d571f92784cd94a019292a1f45445077d0ef634
metrics:
- type: accuracy
value: 76.75857431069267
- type: f1
value: 76.52031675299841
- task:
type: Classification
dataset:
type: mteb/amazon_massive_scenario
name: MTEB MassiveScenarioClassification (de)
config: de
split: test
revision: 7d571f92784cd94a019292a1f45445077d0ef634
metrics:
- type: accuracy
value: 79.03496973772697
- type: f1
value: 79.25548063175344
- task:
type: Classification
dataset:
type: mteb/amazon_massive_scenario
name: MTEB MassiveScenarioClassification (el)
config: el
split: test
revision: 7d571f92784cd94a019292a1f45445077d0ef634
metrics:
- type: accuracy
value: 72.96570275722931
- type: f1
value: 72.19110435289122
- task:
type: Classification
dataset:
type: mteb/amazon_massive_scenario
name: MTEB MassiveScenarioClassification (en)
config: en
split: test
revision: 7d571f92784cd94a019292a1f45445077d0ef634
metrics:
- type: accuracy
value: 82.38735709482178
- type: f1
value: 82.34495627619785
- task:
type: Classification
dataset:
type: mteb/amazon_massive_scenario
name: MTEB MassiveScenarioClassification (es)
config: es
split: test
revision: 7d571f92784cd94a019292a1f45445077d0ef634
metrics:
- type: accuracy
value: 78.83994620040352
- type: f1
value: 78.91526355393667
- task:
type: Classification
dataset:
type: mteb/amazon_massive_scenario
name: MTEB MassiveScenarioClassification (fa)
config: fa
split: test
revision: 7d571f92784cd94a019292a1f45445077d0ef634
metrics:
- type: accuracy
value: 76.7350369872226
- type: f1
value: 75.919437344927
- task:
type: Classification
dataset:
type: mteb/amazon_massive_scenario
name: MTEB MassiveScenarioClassification (fi)
config: fi
split: test
revision: 7d571f92784cd94a019292a1f45445077d0ef634
metrics:
- type: accuracy
value: 71.21721587088096
- type: f1
value: 70.82973286243262
- task:
type: Classification
dataset:
type: mteb/amazon_massive_scenario
name: MTEB MassiveScenarioClassification (fr)
config: fr
split: test
revision: 7d571f92784cd94a019292a1f45445077d0ef634
metrics:
- type: accuracy
value: 78.59784801613988
- type: f1
value: 78.47383161087423
- task:
type: Classification
dataset:
type: mteb/amazon_massive_scenario
name: MTEB MassiveScenarioClassification (he)
config: he
split: test
revision: 7d571f92784cd94a019292a1f45445077d0ef634
metrics:
- type: accuracy
value: 69.64021519838602
- type: f1
value: 68.45118053027653
- task:
type: Classification
dataset:
type: mteb/amazon_massive_scenario
name: MTEB MassiveScenarioClassification (hi)
config: hi
split: test
revision: 7d571f92784cd94a019292a1f45445077d0ef634
metrics:
- type: accuracy
value: 73.51042367182245
- type: f1
value: 72.90013022879003
- task:
type: Classification
dataset:
type: mteb/amazon_massive_scenario
name: MTEB MassiveScenarioClassification (hu)
config: hu
split: test
revision: 7d571f92784cd94a019292a1f45445077d0ef634
metrics:
- type: accuracy
value: 74.0551445864156
- type: f1
value: 73.45871761713292
- task:
type: Classification
dataset:
type: mteb/amazon_massive_scenario
name: MTEB MassiveScenarioClassification (hy)
config: hy
split: test
revision: 7d571f92784cd94a019292a1f45445077d0ef634
metrics:
- type: accuracy
value: 59.54606590450571
- type: f1
value: 57.72711794953869
- task:
type: Classification
dataset:
type: mteb/amazon_massive_scenario
name: MTEB MassiveScenarioClassification (id)
config: id
split: test
revision: 7d571f92784cd94a019292a1f45445077d0ef634
metrics:
- type: accuracy
value: 77.40753194351042
- type: f1
value: 76.8157455506521
- task:
type: Classification
dataset:
type: mteb/amazon_massive_scenario
name: MTEB MassiveScenarioClassification (is)
config: is
split: test
revision: 7d571f92784cd94a019292a1f45445077d0ef634
metrics:
- type: accuracy
value: 66.58372562205783
- type: f1
value: 65.2654868709758
- task:
type: Classification
dataset:
type: mteb/amazon_massive_scenario
name: MTEB MassiveScenarioClassification (it)
config: it
split: test
revision: 7d571f92784cd94a019292a1f45445077d0ef634
metrics:
- type: accuracy
value: 78.39273705447208
- type: f1
value: 78.3592956594837
- task:
type: Classification
dataset:
type: mteb/amazon_massive_scenario
name: MTEB MassiveScenarioClassification (ja)
config: ja
split: test
revision: 7d571f92784cd94a019292a1f45445077d0ef634
metrics:
- type: accuracy
value: 79.62004034969739
- type: f1
value: 79.78673754501855
- task:
type: Classification
dataset:
type: mteb/amazon_massive_scenario
name: MTEB MassiveScenarioClassification (jv)
config: jv
split: test
revision: 7d571f92784cd94a019292a1f45445077d0ef634
metrics:
- type: accuracy
value: 64.29051782111634
- type: f1
value: 63.12502587609454
- task:
type: Classification
dataset:
type: mteb/amazon_massive_scenario
name: MTEB MassiveScenarioClassification (ka)
config: ka
split: test
revision: 7d571f92784cd94a019292a1f45445077d0ef634
metrics:
- type: accuracy
value: 57.51849361129791
- type: f1
value: 56.32320906403241
- task:
type: Classification
dataset:
type: mteb/amazon_massive_scenario
name: MTEB MassiveScenarioClassification (km)
config: km
split: test
revision: 7d571f92784cd94a019292a1f45445077d0ef634
metrics:
- type: accuracy
value: 52.41761936785474
- type: f1
value: 49.113762010098306
- task:
type: Classification
dataset:
type: mteb/amazon_massive_scenario
name: MTEB MassiveScenarioClassification (kn)
config: kn
split: test
revision: 7d571f92784cd94a019292a1f45445077d0ef634
metrics:
- type: accuracy
value: 58.547410894418284
- type: f1
value: 56.87580674198118
- task:
type: Classification
dataset:
type: mteb/amazon_massive_scenario
name: MTEB MassiveScenarioClassification (ko)
config: ko
split: test
revision: 7d571f92784cd94a019292a1f45445077d0ef634
metrics:
- type: accuracy
value: 78.89038332212507
- type: f1
value: 79.09210140529848
- task:
type: Classification
dataset:
type: mteb/amazon_massive_scenario
name: MTEB MassiveScenarioClassification (lv)
config: lv
split: test
revision: 7d571f92784cd94a019292a1f45445077d0ef634
metrics:
- type: accuracy
value: 63.503698722259585
- type: f1
value: 61.45718858568352
- task:
type: Classification
dataset:
type: mteb/amazon_massive_scenario
name: MTEB MassiveScenarioClassification (ml)
config: ml
split: test
revision: 7d571f92784cd94a019292a1f45445077d0ef634
metrics:
- type: accuracy
value: 54.02824478816408
- type: f1
value: 52.732738981386504
- task:
type: Classification
dataset:
type: mteb/amazon_massive_scenario
name: MTEB MassiveScenarioClassification (mn)
config: mn
split: test
revision: 7d571f92784cd94a019292a1f45445077d0ef634
metrics:
- type: accuracy
value: 54.23671822461331
- type: f1
value: 52.688080372545286
- task:
type: Classification
dataset:
type: mteb/amazon_massive_scenario
name: MTEB MassiveScenarioClassification (ms)
config: ms
split: test
revision: 7d571f92784cd94a019292a1f45445077d0ef634
metrics:
- type: accuracy
value: 75.5312710154674
- type: f1
value: 74.59368478550698
- task:
type: Classification
dataset:
type: mteb/amazon_massive_scenario
name: MTEB MassiveScenarioClassification (my)
config: my
split: test
revision: 7d571f92784cd94a019292a1f45445077d0ef634
metrics:
- type: accuracy
value: 52.192333557498316
- type: f1
value: 50.18302290152229
- task:
type: Classification
dataset:
type: mteb/amazon_massive_scenario
name: MTEB MassiveScenarioClassification (nb)
config: nb
split: test
revision: 7d571f92784cd94a019292a1f45445077d0ef634
metrics:
- type: accuracy
value: 75.6960322797579
- type: f1
value: 75.25331182714856
- task:
type: Classification
dataset:
type: mteb/amazon_massive_scenario
name: MTEB MassiveScenarioClassification (nl)
config: nl
split: test
revision: 7d571f92784cd94a019292a1f45445077d0ef634
metrics:
- type: accuracy
value: 78.47679892400808
- type: f1
value: 78.24044732352424
- task:
type: Classification
dataset:
type: mteb/amazon_massive_scenario
name: MTEB MassiveScenarioClassification (pl)
config: pl
split: test
revision: 7d571f92784cd94a019292a1f45445077d0ef634
metrics:
- type: accuracy
value: 77.36718224613315
- type: f1
value: 77.2714452985389
- task:
type: Classification
dataset:
type: mteb/amazon_massive_scenario
name: MTEB MassiveScenarioClassification (pt)
config: pt
split: test
revision: 7d571f92784cd94a019292a1f45445077d0ef634
metrics:
- type: accuracy
value: 77.96234028244788
- type: f1
value: 78.21282127011372
- task:
type: Classification
dataset:
type: mteb/amazon_massive_scenario
name: MTEB MassiveScenarioClassification (ro)
config: ro
split: test
revision: 7d571f92784cd94a019292a1f45445077d0ef634
metrics:
- type: accuracy
value: 73.19435104236717
- type: f1
value: 73.1963711292812
- task:
type: Classification
dataset:
type: mteb/amazon_massive_scenario
name: MTEB MassiveScenarioClassification (ru)
config: ru
split: test
revision: 7d571f92784cd94a019292a1f45445077d0ef634
metrics:
- type: accuracy
value: 80.52118359112306
- type: f1
value: 80.4179964390288
- task:
type: Classification
dataset:
type: mteb/amazon_massive_scenario
name: MTEB MassiveScenarioClassification (sl)
config: sl
split: test
revision: 7d571f92784cd94a019292a1f45445077d0ef634
metrics:
- type: accuracy
value: 73.65837256220577
- type: f1
value: 73.07156989634905
- task:
type: Classification
dataset:
type: mteb/amazon_massive_scenario
name: MTEB MassiveScenarioClassification (sq)
config: sq
split: test
revision: 7d571f92784cd94a019292a1f45445077d0ef634
metrics:
- type: accuracy
value: 64.02824478816409
- type: f1
value: 62.972399027713664
- task:
type: Classification
dataset:
type: mteb/amazon_massive_scenario
name: MTEB MassiveScenarioClassification (sv)
config: sv
split: test
revision: 7d571f92784cd94a019292a1f45445077d0ef634
metrics:
- type: accuracy
value: 78.87020847343645
- type: f1
value: 78.224240866849
- task:
type: Classification
dataset:
type: mteb/amazon_massive_scenario
name: MTEB MassiveScenarioClassification (sw)
config: sw
split: test
revision: 7d571f92784cd94a019292a1f45445077d0ef634
metrics:
- type: accuracy
value: 64.6570275722932
- type: f1
value: 63.274871811412545
- task:
type: Classification
dataset:
type: mteb/amazon_massive_scenario
name: MTEB MassiveScenarioClassification (ta)
config: ta
split: test
revision: 7d571f92784cd94a019292a1f45445077d0ef634
metrics:
- type: accuracy
value: 57.760591795561524
- type: f1
value: 56.73711528075771
- task:
type: Classification
dataset:
type: mteb/amazon_massive_scenario
name: MTEB MassiveScenarioClassification (te)
config: te
split: test
revision: 7d571f92784cd94a019292a1f45445077d0ef634
metrics:
- type: accuracy
value: 57.26967047747142
- type: f1
value: 55.74735330863165
- task:
type: Classification
dataset:
type: mteb/amazon_massive_scenario
name: MTEB MassiveScenarioClassification (th)
config: th
split: test
revision: 7d571f92784cd94a019292a1f45445077d0ef634
metrics:
- type: accuracy
value: 72.46133154001345
- type: f1
value: 71.9644168952811
- task:
type: Classification
dataset:
type: mteb/amazon_massive_scenario
name: MTEB MassiveScenarioClassification (tl)
config: tl
split: test
revision: 7d571f92784cd94a019292a1f45445077d0ef634
metrics:
- type: accuracy
value: 73.70880968392737
- type: f1
value: 73.61543141070884
- task:
type: Classification
dataset:
type: mteb/amazon_massive_scenario
name: MTEB MassiveScenarioClassification (tr)
config: tr
split: test
revision: 7d571f92784cd94a019292a1f45445077d0ef634
metrics:
- type: accuracy
value: 75.0437121721587
- type: f1
value: 74.83359868879921
- task:
type: Classification
dataset:
type: mteb/amazon_massive_scenario
name: MTEB MassiveScenarioClassification (ur)
config: ur
split: test
revision: 7d571f92784cd94a019292a1f45445077d0ef634
metrics:
- type: accuracy
value: 67.05110961667788
- type: f1
value: 66.25869819274315
- task:
type: Classification
dataset:
type: mteb/amazon_massive_scenario
name: MTEB MassiveScenarioClassification (vi)
config: vi
split: test
revision: 7d571f92784cd94a019292a1f45445077d0ef634
metrics:
- type: accuracy
value: 75.52118359112306
- type: f1
value: 75.92098546052303
- task:
type: Classification
dataset:
type: mteb/amazon_massive_scenario
name: MTEB MassiveScenarioClassification (zh-CN)
config: zh-CN
split: test
revision: 7d571f92784cd94a019292a1f45445077d0ef634
metrics:
- type: accuracy
value: 79.92938802958977
- type: f1
value: 79.79833572573796
- task:
type: Classification
dataset:
type: mteb/amazon_massive_scenario
name: MTEB MassiveScenarioClassification (zh-TW)
config: zh-TW
split: test
revision: 7d571f92784cd94a019292a1f45445077d0ef634
metrics:
- type: accuracy
value: 76.86617350369872
- type: f1
value: 77.42645654909516
- task:
type: Retrieval
dataset:
type: C-MTEB/MedicalRetrieval
name: MTEB MedicalRetrieval
config: default
split: dev
revision: None
metrics:
- type: map_at_1
value: 44.6
- type: map_at_10
value: 50.019000000000005
- type: map_at_100
value: 50.611
- type: map_at_1000
value: 50.67
- type: map_at_3
value: 48.699999999999996
- type: map_at_5
value: 49.455
- type: mrr_at_1
value: 44.800000000000004
- type: mrr_at_10
value: 50.119
- type: mrr_at_100
value: 50.711
- type: mrr_at_1000
value: 50.77
- type: mrr_at_3
value: 48.8
- type: mrr_at_5
value: 49.555
- type: ndcg_at_1
value: 44.6
- type: ndcg_at_10
value: 52.754
- type: ndcg_at_100
value: 55.935
- type: ndcg_at_1000
value: 57.607
- type: ndcg_at_3
value: 50.012
- type: ndcg_at_5
value: 51.393
- type: precision_at_1
value: 44.6
- type: precision_at_10
value: 6.140000000000001
- type: precision_at_100
value: 0.77
- type: precision_at_1000
value: 0.09
- type: precision_at_3
value: 17.933
- type: precision_at_5
value: 11.44
- type: recall_at_1
value: 44.6
- type: recall_at_10
value: 61.4
- type: recall_at_100
value: 77.0
- type: recall_at_1000
value: 90.4
- type: recall_at_3
value: 53.800000000000004
- type: recall_at_5
value: 57.199999999999996
- task:
type: Clustering
dataset:
type: mteb/medrxiv-clustering-p2p
name: MTEB MedrxivClusteringP2P
config: default
split: test
revision: e7a26af6f3ae46b30dde8737f02c07b1505bcc73
metrics:
- type: v_measure
value: 38.192667527616315
- task:
type: Clustering
dataset:
type: mteb/medrxiv-clustering-s2s
name: MTEB MedrxivClusteringS2S
config: default
split: test
revision: 35191c8c0dca72d8ff3efcd72aa802307d469663
metrics:
- type: v_measure
value: 37.44738902946689
- task:
type: Reranking
dataset:
type: mteb/mind_small
name: MTEB MindSmallReranking
config: default
split: test
revision: 3bdac13927fdc888b903db93b2ffdbd90b295a69
metrics:
- type: map
value: 32.59661273103955
- type: mrr
value: 33.82024242497473
- task:
type: Classification
dataset:
type: C-MTEB/MultilingualSentiment-classification
name: MTEB MultilingualSentiment
config: default
split: validation
revision: None
metrics:
- type: accuracy
value: 73.31333333333335
- type: f1
value: 73.0873466527602
- task:
type: Retrieval
dataset:
type: nfcorpus
name: MTEB NFCorpus
config: default
split: test
revision: None
metrics:
- type: map_at_1
value: 5.471
- type: map_at_10
value: 14.142
- type: map_at_100
value: 18.179000000000002
- type: map_at_1000
value: 19.772000000000002
- type: map_at_3
value: 9.716
- type: map_at_5
value: 11.763
- type: mrr_at_1
value: 51.393
- type: mrr_at_10
value: 58.814
- type: mrr_at_100
value: 59.330000000000005
- type: mrr_at_1000
value: 59.35
- type: mrr_at_3
value: 56.398
- type: mrr_at_5
value: 58.038999999999994
- type: ndcg_at_1
value: 49.69
- type: ndcg_at_10
value: 38.615
- type: ndcg_at_100
value: 35.268
- type: ndcg_at_1000
value: 43.745
- type: ndcg_at_3
value: 43.187
- type: ndcg_at_5
value: 41.528999999999996
- type: precision_at_1
value: 51.083999999999996
- type: precision_at_10
value: 29.474
- type: precision_at_100
value: 9.167
- type: precision_at_1000
value: 2.2089999999999996
- type: precision_at_3
value: 40.351
- type: precision_at_5
value: 36.285000000000004
- type: recall_at_1
value: 5.471
- type: recall_at_10
value: 19.242
- type: recall_at_100
value: 37.14
- type: recall_at_1000
value: 68.35900000000001
- type: recall_at_3
value: 10.896
- type: recall_at_5
value: 14.75
- task:
type: Retrieval
dataset:
type: nq
name: MTEB NQ
config: default
split: test
revision: None
metrics:
- type: map_at_1
value: 39.499
- type: map_at_10
value: 55.862
- type: map_at_100
value: 56.667
- type: map_at_1000
value: 56.684999999999995
- type: map_at_3
value: 51.534
- type: map_at_5
value: 54.2
- type: mrr_at_1
value: 44.351
- type: mrr_at_10
value: 58.567
- type: mrr_at_100
value: 59.099000000000004
- type: mrr_at_1000
value: 59.109
- type: mrr_at_3
value: 55.218999999999994
- type: mrr_at_5
value: 57.391999999999996
- type: ndcg_at_1
value: 44.322
- type: ndcg_at_10
value: 63.535
- type: ndcg_at_100
value: 66.654
- type: ndcg_at_1000
value: 66.991
- type: ndcg_at_3
value: 55.701
- type: ndcg_at_5
value: 60.06700000000001
- type: precision_at_1
value: 44.322
- type: precision_at_10
value: 10.026
- type: precision_at_100
value: 1.18
- type: precision_at_1000
value: 0.121
- type: precision_at_3
value: 24.865000000000002
- type: precision_at_5
value: 17.48
- type: recall_at_1
value: 39.499
- type: recall_at_10
value: 84.053
- type: recall_at_100
value: 97.11
- type: recall_at_1000
value: 99.493
- type: recall_at_3
value: 64.091
- type: recall_at_5
value: 74.063
- task:
type: PairClassification
dataset:
type: C-MTEB/OCNLI
name: MTEB Ocnli
config: default
split: validation
revision: None
metrics:
- type: cos_sim_accuracy
value: 61.18029236599891
- type: cos_sim_ap
value: 64.18398769398412
- type: cos_sim_f1
value: 67.96347757046446
- type: cos_sim_precision
value: 54.4529262086514
- type: cos_sim_recall
value: 90.3907074973601
- type: dot_accuracy
value: 61.18029236599891
- type: dot_ap
value: 64.18393484706077
- type: dot_f1
value: 67.96347757046446
- type: dot_precision
value: 54.4529262086514
- type: dot_recall
value: 90.3907074973601
- type: euclidean_accuracy
value: 61.18029236599891
- type: euclidean_ap
value: 64.18395024821486
- type: euclidean_f1
value: 67.96347757046446
- type: euclidean_precision
value: 54.4529262086514
- type: euclidean_recall
value: 90.3907074973601
- type: manhattan_accuracy
value: 61.451001624255554
- type: manhattan_ap
value: 64.38232708763513
- type: manhattan_f1
value: 68.05860805860804
- type: manhattan_precision
value: 52.10319685922602
- type: manhattan_recall
value: 98.09926082365365
- type: max_accuracy
value: 61.451001624255554
- type: max_ap
value: 64.38232708763513
- type: max_f1
value: 68.05860805860804
- task:
type: Classification
dataset:
type: C-MTEB/OnlineShopping-classification
name: MTEB OnlineShopping
config: default
split: test
revision: None
metrics:
- type: accuracy
value: 92.19000000000001
- type: ap
value: 89.73918431886767
- type: f1
value: 92.17175032574507
- task:
type: STS
dataset:
type: C-MTEB/PAWSX
name: MTEB PAWSX
config: default
split: test
revision: None
metrics:
- type: cos_sim_pearson
value: 15.079320253752224
- type: cos_sim_spearman
value: 16.813772504404263
- type: euclidean_pearson
value: 19.476541162041762
- type: euclidean_spearman
value: 16.813772498098782
- type: manhattan_pearson
value: 19.497429832915277
- type: manhattan_spearman
value: 16.869600674180607
- task:
type: STS
dataset:
type: C-MTEB/QBQTC
name: MTEB QBQTC
config: default
split: test
revision: None
metrics:
- type: cos_sim_pearson
value: 30.36139599797913
- type: cos_sim_spearman
value: 31.80296402851347
- type: euclidean_pearson
value: 30.10387888252793
- type: euclidean_spearman
value: 31.80297780103808
- type: manhattan_pearson
value: 30.86720382849436
- type: manhattan_spearman
value: 32.70491131366606
- task:
type: Retrieval
dataset:
type: quora
name: MTEB QuoraRetrieval
config: default
split: test
revision: None
metrics:
- type: map_at_1
value: 71.911
- type: map_at_10
value: 86.087
- type: map_at_100
value: 86.701
- type: map_at_1000
value: 86.715
- type: map_at_3
value: 83.231
- type: map_at_5
value: 85.051
- type: mrr_at_1
value: 82.75
- type: mrr_at_10
value: 88.759
- type: mrr_at_100
value: 88.844
- type: mrr_at_1000
value: 88.844
- type: mrr_at_3
value: 87.935
- type: mrr_at_5
value: 88.504
- type: ndcg_at_1
value: 82.75
- type: ndcg_at_10
value: 89.605
- type: ndcg_at_100
value: 90.664
- type: ndcg_at_1000
value: 90.733
- type: ndcg_at_3
value: 87.03
- type: ndcg_at_5
value: 88.473
- type: precision_at_1
value: 82.75
- type: precision_at_10
value: 13.575000000000001
- type: precision_at_100
value: 1.539
- type: precision_at_1000
value: 0.157
- type: precision_at_3
value: 38.153
- type: precision_at_5
value: 25.008000000000003
- type: recall_at_1
value: 71.911
- type: recall_at_10
value: 96.261
- type: recall_at_100
value: 99.72800000000001
- type: recall_at_1000
value: 99.993
- type: recall_at_3
value: 88.762
- type: recall_at_5
value: 92.949
- task:
type: Clustering
dataset:
type: mteb/reddit-clustering
name: MTEB RedditClustering
config: default
split: test
revision: 24640382cdbf8abc73003fb0fa6d111a705499eb
metrics:
- type: v_measure
value: 57.711581165572376
- task:
type: Clustering
dataset:
type: mteb/reddit-clustering-p2p
name: MTEB RedditClusteringP2P
config: default
split: test
revision: 282350215ef01743dc01b456c7f5241fa8937f16
metrics:
- type: v_measure
value: 66.48938885750297
- task:
type: Retrieval
dataset:
type: scidocs
name: MTEB SCIDOCS
config: default
split: test
revision: None
metrics:
- type: map_at_1
value: 3.7379999999999995
- type: map_at_10
value: 9.261
- type: map_at_100
value: 11.001
- type: map_at_1000
value: 11.262
- type: map_at_3
value: 6.816
- type: map_at_5
value: 8.0
- type: mrr_at_1
value: 18.4
- type: mrr_at_10
value: 28.755999999999997
- type: mrr_at_100
value: 29.892000000000003
- type: mrr_at_1000
value: 29.961
- type: mrr_at_3
value: 25.467000000000002
- type: mrr_at_5
value: 27.332
- type: ndcg_at_1
value: 18.4
- type: ndcg_at_10
value: 16.296
- type: ndcg_at_100
value: 23.52
- type: ndcg_at_1000
value: 28.504
- type: ndcg_at_3
value: 15.485
- type: ndcg_at_5
value: 13.471
- type: precision_at_1
value: 18.4
- type: precision_at_10
value: 8.469999999999999
- type: precision_at_100
value: 1.8950000000000002
- type: precision_at_1000
value: 0.309
- type: precision_at_3
value: 14.6
- type: precision_at_5
value: 11.84
- type: recall_at_1
value: 3.7379999999999995
- type: recall_at_10
value: 17.185
- type: recall_at_100
value: 38.397
- type: recall_at_1000
value: 62.798
- type: recall_at_3
value: 8.896999999999998
- type: recall_at_5
value: 12.021999999999998
- task:
type: STS
dataset:
type: mteb/sickr-sts
name: MTEB SICK-R
config: default
split: test
revision: a6ea5a8cab320b040a23452cc28066d9beae2cee
metrics:
- type: cos_sim_pearson
value: 86.43977757480083
- type: cos_sim_spearman
value: 82.64182475199533
- type: euclidean_pearson
value: 83.71756009999591
- type: euclidean_spearman
value: 82.64182331395057
- type: manhattan_pearson
value: 83.8028936913025
- type: manhattan_spearman
value: 82.71024597804252
- task:
type: STS
dataset:
type: mteb/sts12-sts
name: MTEB STS12
config: default
split: test
revision: a0d554a64d88156834ff5ae9920b964011b16384
metrics:
- type: cos_sim_pearson
value: 86.85653060698912
- type: cos_sim_spearman
value: 79.65598885228324
- type: euclidean_pearson
value: 83.1205137628455
- type: euclidean_spearman
value: 79.65629387709038
- type: manhattan_pearson
value: 83.71108853545837
- type: manhattan_spearman
value: 80.25617619716708
- task:
type: STS
dataset:
type: mteb/sts13-sts
name: MTEB STS13
config: default
split: test
revision: 7e90230a92c190f1bf69ae9002b8cea547a64cca
metrics:
- type: cos_sim_pearson
value: 88.22921688565664
- type: cos_sim_spearman
value: 88.42662103041957
- type: euclidean_pearson
value: 87.91679798473325
- type: euclidean_spearman
value: 88.42662103041957
- type: manhattan_pearson
value: 88.16927537961303
- type: manhattan_spearman
value: 88.81581680062541
- task:
type: STS
dataset:
type: mteb/sts14-sts
name: MTEB STS14
config: default
split: test
revision: 6031580fec1f6af667f0bd2da0a551cf4f0b2375
metrics:
- type: cos_sim_pearson
value: 86.77261424554293
- type: cos_sim_spearman
value: 84.53930146434155
- type: euclidean_pearson
value: 85.67420491389697
- type: euclidean_spearman
value: 84.53929771783851
- type: manhattan_pearson
value: 85.74306784515618
- type: manhattan_spearman
value: 84.7399304675314
- task:
type: STS
dataset:
type: mteb/sts15-sts
name: MTEB STS15
config: default
split: test
revision: ae752c7c21bf194d8b67fd573edf7ae58183cbe3
metrics:
- type: cos_sim_pearson
value: 89.86138395166455
- type: cos_sim_spearman
value: 90.42577823022054
- type: euclidean_pearson
value: 89.8787763797515
- type: euclidean_spearman
value: 90.42577823022054
- type: manhattan_pearson
value: 89.9592937492158
- type: manhattan_spearman
value: 90.63535505335524
- task:
type: STS
dataset:
type: mteb/sts16-sts
name: MTEB STS16
config: default
split: test
revision: 4d8694f8f0e0100860b497b999b3dbed754a0513
metrics:
- type: cos_sim_pearson
value: 86.5176674585941
- type: cos_sim_spearman
value: 87.6842917085397
- type: euclidean_pearson
value: 86.70213081520711
- type: euclidean_spearman
value: 87.6842917085397
- type: manhattan_pearson
value: 86.83702628983627
- type: manhattan_spearman
value: 87.87791000374443
- task:
type: STS
dataset:
type: mteb/sts17-crosslingual-sts
name: MTEB STS17 (ko-ko)
config: ko-ko
split: test
revision: af5e6fb845001ecf41f4c1e033ce921939a2a68d
metrics:
- type: cos_sim_pearson
value: 83.86395454805867
- type: cos_sim_spearman
value: 83.69454595252267
- type: euclidean_pearson
value: 83.04743892608313
- type: euclidean_spearman
value: 83.69454026433006
- type: manhattan_pearson
value: 83.4032095553322
- type: manhattan_spearman
value: 84.11527379013802
- task:
type: STS
dataset:
type: mteb/sts17-crosslingual-sts
name: MTEB STS17 (ar-ar)
config: ar-ar
split: test
revision: af5e6fb845001ecf41f4c1e033ce921939a2a68d
metrics:
- type: cos_sim_pearson
value: 81.80249894729546
- type: cos_sim_spearman
value: 81.87004960533409
- type: euclidean_pearson
value: 80.0392760044179
- type: euclidean_spearman
value: 81.87004960533409
- type: manhattan_pearson
value: 80.38096542355912
- type: manhattan_spearman
value: 82.40774679630341
- task:
type: STS
dataset:
type: mteb/sts17-crosslingual-sts
name: MTEB STS17 (en-ar)
config: en-ar
split: test
revision: af5e6fb845001ecf41f4c1e033ce921939a2a68d
metrics:
- type: cos_sim_pearson
value: 77.6158201787172
- type: cos_sim_spearman
value: 77.934651044009
- type: euclidean_pearson
value: 77.7874683895269
- type: euclidean_spearman
value: 77.934651044009
- type: manhattan_pearson
value: 78.36151849193052
- type: manhattan_spearman
value: 78.52439586349938
- task:
type: STS
dataset:
type: mteb/sts17-crosslingual-sts
name: MTEB STS17 (en-de)
config: en-de
split: test
revision: af5e6fb845001ecf41f4c1e033ce921939a2a68d
metrics:
- type: cos_sim_pearson
value: 87.04363311392207
- type: cos_sim_spearman
value: 87.30483659369973
- type: euclidean_pearson
value: 87.62634489502616
- type: euclidean_spearman
value: 87.30483659369973
- type: manhattan_pearson
value: 88.02340837141445
- type: manhattan_spearman
value: 87.55012003294
- task:
type: STS
dataset:
type: mteb/sts17-crosslingual-sts
name: MTEB STS17 (en-en)
config: en-en
split: test
revision: af5e6fb845001ecf41f4c1e033ce921939a2a68d
metrics:
- type: cos_sim_pearson
value: 91.69172851958248
- type: cos_sim_spearman
value: 91.7546879482416
- type: euclidean_pearson
value: 91.84843039183963
- type: euclidean_spearman
value: 91.7546879482416
- type: manhattan_pearson
value: 91.72325753804357
- type: manhattan_spearman
value: 91.55330259513397
- task:
type: STS
dataset:
type: mteb/sts17-crosslingual-sts
name: MTEB STS17 (en-tr)
config: en-tr
split: test
revision: af5e6fb845001ecf41f4c1e033ce921939a2a68d
metrics:
- type: cos_sim_pearson
value: 73.95572901084864
- type: cos_sim_spearman
value: 72.56217821552626
- type: euclidean_pearson
value: 74.24242980323574
- type: euclidean_spearman
value: 72.56217821552626
- type: manhattan_pearson
value: 74.57473362519922
- type: manhattan_spearman
value: 72.76048826648497
- task:
type: STS
dataset:
type: mteb/sts17-crosslingual-sts
name: MTEB STS17 (es-en)
config: es-en
split: test
revision: af5e6fb845001ecf41f4c1e033ce921939a2a68d
metrics:
- type: cos_sim_pearson
value: 86.93329396008296
- type: cos_sim_spearman
value: 88.2406635486219
- type: euclidean_pearson
value: 87.49687343908533
- type: euclidean_spearman
value: 88.2406635486219
- type: manhattan_pearson
value: 88.14088309231084
- type: manhattan_spearman
value: 88.93314020908534
- task:
type: STS
dataset:
type: mteb/sts17-crosslingual-sts
name: MTEB STS17 (es-es)
config: es-es
split: test
revision: af5e6fb845001ecf41f4c1e033ce921939a2a68d
metrics:
- type: cos_sim_pearson
value: 88.70124451546057
- type: cos_sim_spearman
value: 87.45988160052252
- type: euclidean_pearson
value: 88.44395505247728
- type: euclidean_spearman
value: 87.45988160052252
- type: manhattan_pearson
value: 88.69269783495425
- type: manhattan_spearman
value: 87.65383425621
- task:
type: STS
dataset:
type: mteb/sts17-crosslingual-sts
name: MTEB STS17 (fr-en)
config: fr-en
split: test
revision: af5e6fb845001ecf41f4c1e033ce921939a2a68d
metrics:
- type: cos_sim_pearson
value: 87.64109149761346
- type: cos_sim_spearman
value: 88.06459637689733
- type: euclidean_pearson
value: 88.02313315797703
- type: euclidean_spearman
value: 88.06459637689733
- type: manhattan_pearson
value: 88.28328539133253
- type: manhattan_spearman
value: 88.06605708379142
- task:
type: STS
dataset:
type: mteb/sts17-crosslingual-sts
name: MTEB STS17 (it-en)
config: it-en
split: test
revision: af5e6fb845001ecf41f4c1e033ce921939a2a68d
metrics:
- type: cos_sim_pearson
value: 88.9040028177525
- type: cos_sim_spearman
value: 89.68152202933464
- type: euclidean_pearson
value: 89.23684469601253
- type: euclidean_spearman
value: 89.68152202933464
- type: manhattan_pearson
value: 89.59504307277454
- type: manhattan_spearman
value: 89.88060100313582
- task:
type: STS
dataset:
type: mteb/sts17-crosslingual-sts
name: MTEB STS17 (nl-en)
config: nl-en
split: test
revision: af5e6fb845001ecf41f4c1e033ce921939a2a68d
metrics:
- type: cos_sim_pearson
value: 87.69891585325125
- type: cos_sim_spearman
value: 88.25252785071736
- type: euclidean_pearson
value: 87.99932873748662
- type: euclidean_spearman
value: 88.25252785071736
- type: manhattan_pearson
value: 88.26959683009446
- type: manhattan_spearman
value: 88.32583227300715
- task:
type: STS
dataset:
type: mteb/sts22-crosslingual-sts
name: MTEB STS22 (en)
config: en
split: test
revision: 6d1ba47164174a496b7fa5d3569dae26a6813b80
metrics:
- type: cos_sim_pearson
value: 67.53235909794135
- type: cos_sim_spearman
value: 66.97521740529574
- type: euclidean_pearson
value: 68.19502223613912
- type: euclidean_spearman
value: 66.97521740529574
- type: manhattan_pearson
value: 68.39070714774539
- type: manhattan_spearman
value: 67.1072812364868
- task:
type: STS
dataset:
type: mteb/sts22-crosslingual-sts
name: MTEB STS22 (de)
config: de
split: test
revision: 6d1ba47164174a496b7fa5d3569dae26a6813b80
metrics:
- type: cos_sim_pearson
value: 43.715742021204775
- type: cos_sim_spearman
value: 49.12255971271453
- type: euclidean_pearson
value: 40.76848562610837
- type: euclidean_spearman
value: 49.12255971271453
- type: manhattan_pearson
value: 40.92204625614112
- type: manhattan_spearman
value: 49.23333793661129
- task:
type: STS
dataset:
type: mteb/sts22-crosslingual-sts
name: MTEB STS22 (es)
config: es
split: test
revision: 6d1ba47164174a496b7fa5d3569dae26a6813b80
metrics:
- type: cos_sim_pearson
value: 63.35268345563588
- type: cos_sim_spearman
value: 66.99661626042061
- type: euclidean_pearson
value: 65.85589122857066
- type: euclidean_spearman
value: 66.99661626042061
- type: manhattan_pearson
value: 66.78454301512294
- type: manhattan_spearman
value: 67.17570330149233
- task:
type: STS
dataset:
type: mteb/sts22-crosslingual-sts
name: MTEB STS22 (pl)
config: pl
split: test
revision: 6d1ba47164174a496b7fa5d3569dae26a6813b80
metrics:
- type: cos_sim_pearson
value: 33.36599908204445
- type: cos_sim_spearman
value: 39.20768331939503
- type: euclidean_pearson
value: 22.16066769530468
- type: euclidean_spearman
value: 39.20768331939503
- type: manhattan_pearson
value: 22.386053195546022
- type: manhattan_spearman
value: 39.70172817465986
- task:
type: STS
dataset:
type: mteb/sts22-crosslingual-sts
name: MTEB STS22 (tr)
config: tr
split: test
revision: 6d1ba47164174a496b7fa5d3569dae26a6813b80
metrics:
- type: cos_sim_pearson
value: 63.06813956986753
- type: cos_sim_spearman
value: 68.72065117995668
- type: euclidean_pearson
value: 66.97373456344194
- type: euclidean_spearman
value: 68.72065117995668
- type: manhattan_pearson
value: 67.34907265771595
- type: manhattan_spearman
value: 68.73705769957843
- task:
type: STS
dataset:
type: mteb/sts22-crosslingual-sts
name: MTEB STS22 (ar)
config: ar
split: test
revision: 6d1ba47164174a496b7fa5d3569dae26a6813b80
metrics:
- type: cos_sim_pearson
value: 47.17664865207108
- type: cos_sim_spearman
value: 54.115568323148864
- type: euclidean_pearson
value: 48.56418162879182
- type: euclidean_spearman
value: 54.115568323148864
- type: manhattan_pearson
value: 48.85951643453165
- type: manhattan_spearman
value: 54.13599784169052
- task:
type: STS
dataset:
type: mteb/sts22-crosslingual-sts
name: MTEB STS22 (ru)
config: ru
split: test
revision: 6d1ba47164174a496b7fa5d3569dae26a6813b80
metrics:
- type: cos_sim_pearson
value: 55.87514136275987
- type: cos_sim_spearman
value: 60.82923573674973
- type: euclidean_pearson
value: 53.724183308215615
- type: euclidean_spearman
value: 60.82923573674973
- type: manhattan_pearson
value: 53.954305573102445
- type: manhattan_spearman
value: 60.957483900644526
- task:
type: STS
dataset:
type: mteb/sts22-crosslingual-sts
name: MTEB STS22 (zh)
config: zh
split: test
revision: 6d1ba47164174a496b7fa5d3569dae26a6813b80
metrics:
- type: cos_sim_pearson
value: 59.55001413648593
- type: cos_sim_spearman
value: 63.395777040381276
- type: euclidean_pearson
value: 59.869972550293305
- type: euclidean_spearman
value: 63.395777040381276
- type: manhattan_pearson
value: 61.16195496847885
- type: manhattan_spearman
value: 63.41968682525581
- task:
type: STS
dataset:
type: mteb/sts22-crosslingual-sts
name: MTEB STS22 (fr)
config: fr
split: test
revision: 6d1ba47164174a496b7fa5d3569dae26a6813b80
metrics:
- type: cos_sim_pearson
value: 79.13334972675852
- type: cos_sim_spearman
value: 79.86263136371802
- type: euclidean_pearson
value: 78.2433603592541
- type: euclidean_spearman
value: 79.86263136371802
- type: manhattan_pearson
value: 78.87337106318412
- type: manhattan_spearman
value: 80.31230584758441
- task:
type: STS
dataset:
type: mteb/sts22-crosslingual-sts
name: MTEB STS22 (de-en)
config: de-en
split: test
revision: 6d1ba47164174a496b7fa5d3569dae26a6813b80
metrics:
- type: cos_sim_pearson
value: 63.559700748242356
- type: cos_sim_spearman
value: 60.92342109509558
- type: euclidean_pearson
value: 66.07256437521119
- type: euclidean_spearman
value: 60.92342109509558
- type: manhattan_pearson
value: 67.72769744612663
- type: manhattan_spearman
value: 59.64714507774168
- task:
type: STS
dataset:
type: mteb/sts22-crosslingual-sts
name: MTEB STS22 (es-en)
config: es-en
split: test
revision: 6d1ba47164174a496b7fa5d3569dae26a6813b80
metrics:
- type: cos_sim_pearson
value: 73.93491616145891
- type: cos_sim_spearman
value: 75.84242594400156
- type: euclidean_pearson
value: 74.87279745626121
- type: euclidean_spearman
value: 75.84242594400156
- type: manhattan_pearson
value: 76.47764144677505
- type: manhattan_spearman
value: 77.08411157845183
- task:
type: STS
dataset:
type: mteb/sts22-crosslingual-sts
name: MTEB STS22 (it)
config: it
split: test
revision: 6d1ba47164174a496b7fa5d3569dae26a6813b80
metrics:
- type: cos_sim_pearson
value: 72.75624124540954
- type: cos_sim_spearman
value: 75.8667941654703
- type: euclidean_pearson
value: 73.74314588451925
- type: euclidean_spearman
value: 75.8667941654703
- type: manhattan_pearson
value: 73.99641425871518
- type: manhattan_spearman
value: 76.1982840205817
- task:
type: STS
dataset:
type: mteb/sts22-crosslingual-sts
name: MTEB STS22 (pl-en)
config: pl-en
split: test
revision: 6d1ba47164174a496b7fa5d3569dae26a6813b80
metrics:
- type: cos_sim_pearson
value: 75.20898141298767
- type: cos_sim_spearman
value: 73.18060375331436
- type: euclidean_pearson
value: 75.44489280944619
- type: euclidean_spearman
value: 73.18060375331436
- type: manhattan_pearson
value: 75.65451039552286
- type: manhattan_spearman
value: 72.97744006123156
- task:
type: STS
dataset:
type: mteb/sts22-crosslingual-sts
name: MTEB STS22 (zh-en)
config: zh-en
split: test
revision: 6d1ba47164174a496b7fa5d3569dae26a6813b80
metrics:
- type: cos_sim_pearson
value: 72.04278252247816
- type: cos_sim_spearman
value: 71.8846446821539
- type: euclidean_pearson
value: 73.16043307050612
- type: euclidean_spearman
value: 71.8846446821539
- type: manhattan_pearson
value: 74.76905116839777
- type: manhattan_spearman
value: 72.66237093518471
- task:
type: STS
dataset:
type: mteb/sts22-crosslingual-sts
name: MTEB STS22 (es-it)
config: es-it
split: test
revision: 6d1ba47164174a496b7fa5d3569dae26a6813b80
metrics:
- type: cos_sim_pearson
value: 71.71033173838558
- type: cos_sim_spearman
value: 75.043122881885
- type: euclidean_pearson
value: 72.77579680345087
- type: euclidean_spearman
value: 75.043122881885
- type: manhattan_pearson
value: 72.99901534854922
- type: manhattan_spearman
value: 75.15418335015957
- task:
type: STS
dataset:
type: mteb/sts22-crosslingual-sts
name: MTEB STS22 (de-fr)
config: de-fr
split: test
revision: 6d1ba47164174a496b7fa5d3569dae26a6813b80
metrics:
- type: cos_sim_pearson
value: 55.75733447190482
- type: cos_sim_spearman
value: 61.38968334176681
- type: euclidean_pearson
value: 55.479231520643744
- type: euclidean_spearman
value: 61.38968334176681
- type: manhattan_pearson
value: 56.05230571465244
- type: manhattan_spearman
value: 62.69383054007398
- task:
type: STS
dataset:
type: mteb/sts22-crosslingual-sts
name: MTEB STS22 (de-pl)
config: de-pl
split: test
revision: 6d1ba47164174a496b7fa5d3569dae26a6813b80
metrics:
- type: cos_sim_pearson
value: 41.72244325050302
- type: cos_sim_spearman
value: 54.47476909084119
- type: euclidean_pearson
value: 43.94629756436873
- type: euclidean_spearman
value: 54.47476909084119
- type: manhattan_pearson
value: 46.36533046394657
- type: manhattan_spearman
value: 54.87509243633636
- task:
type: STS
dataset:
type: mteb/sts22-crosslingual-sts
name: MTEB STS22 (fr-pl)
config: fr-pl
split: test
revision: 6d1ba47164174a496b7fa5d3569dae26a6813b80
metrics:
- type: cos_sim_pearson
value: 70.75183711835146
- type: cos_sim_spearman
value: 84.51542547285167
- type: euclidean_pearson
value: 71.84188960126669
- type: euclidean_spearman
value: 84.51542547285167
- type: manhattan_pearson
value: 73.94847166379994
- type: manhattan_spearman
value: 84.51542547285167
- task:
type: STS
dataset:
type: C-MTEB/STSB
name: MTEB STSB
config: default
split: test
revision: None
metrics:
- type: cos_sim_pearson
value: 81.78690149086131
- type: cos_sim_spearman
value: 81.81202616916873
- type: euclidean_pearson
value: 80.98792254251062
- type: euclidean_spearman
value: 81.81202616916873
- type: manhattan_pearson
value: 81.46953021346732
- type: manhattan_spearman
value: 82.34259562492315
- task:
type: STS
dataset:
type: mteb/stsbenchmark-sts
name: MTEB STSBenchmark
config: default
split: test
revision: b0fddb56ed78048fa8b90373c8a3cfc37b684831
metrics:
- type: cos_sim_pearson
value: 87.68273341294419
- type: cos_sim_spearman
value: 88.59927164210958
- type: euclidean_pearson
value: 88.10745681818025
- type: euclidean_spearman
value: 88.59927164210958
- type: manhattan_pearson
value: 88.25166703784649
- type: manhattan_spearman
value: 88.85343247873482
- task:
type: Reranking
dataset:
type: mteb/scidocs-reranking
name: MTEB SciDocsRR
config: default
split: test
revision: d3c5e1fc0b855ab6097bf1cda04dd73947d7caab
metrics:
- type: map
value: 86.3340463345719
- type: mrr
value: 96.5182611506141
- task:
type: Retrieval
dataset:
type: scifact
name: MTEB SciFact
config: default
split: test
revision: None
metrics:
- type: map_at_1
value: 60.967000000000006
- type: map_at_10
value: 71.873
- type: map_at_100
value: 72.271
- type: map_at_1000
value: 72.292
- type: map_at_3
value: 69.006
- type: map_at_5
value: 70.856
- type: mrr_at_1
value: 63.666999999999994
- type: mrr_at_10
value: 72.929
- type: mrr_at_100
value: 73.26
- type: mrr_at_1000
value: 73.282
- type: mrr_at_3
value: 71.111
- type: mrr_at_5
value: 72.328
- type: ndcg_at_1
value: 63.666999999999994
- type: ndcg_at_10
value: 76.414
- type: ndcg_at_100
value: 78.152
- type: ndcg_at_1000
value: 78.604
- type: ndcg_at_3
value: 71.841
- type: ndcg_at_5
value: 74.435
- type: precision_at_1
value: 63.666999999999994
- type: precision_at_10
value: 10.067
- type: precision_at_100
value: 1.097
- type: precision_at_1000
value: 0.11299999999999999
- type: precision_at_3
value: 27.667
- type: precision_at_5
value: 18.467
- type: recall_at_1
value: 60.967000000000006
- type: recall_at_10
value: 88.922
- type: recall_at_100
value: 96.667
- type: recall_at_1000
value: 100.0
- type: recall_at_3
value: 77.228
- type: recall_at_5
value: 83.428
- task:
type: PairClassification
dataset:
type: mteb/sprintduplicatequestions-pairclassification
name: MTEB SprintDuplicateQuestions
config: default
split: test
revision: d66bd1f72af766a5cc4b0ca5e00c162f89e8cc46
metrics:
- type: cos_sim_accuracy
value: 99.82277227722773
- type: cos_sim_ap
value: 95.66279851444406
- type: cos_sim_f1
value: 90.9367088607595
- type: cos_sim_precision
value: 92.1025641025641
- type: cos_sim_recall
value: 89.8
- type: dot_accuracy
value: 99.82277227722773
- type: dot_ap
value: 95.66279851444406
- type: dot_f1
value: 90.9367088607595
- type: dot_precision
value: 92.1025641025641
- type: dot_recall
value: 89.8
- type: euclidean_accuracy
value: 99.82277227722773
- type: euclidean_ap
value: 95.66279851444406
- type: euclidean_f1
value: 90.9367088607595
- type: euclidean_precision
value: 92.1025641025641
- type: euclidean_recall
value: 89.8
- type: manhattan_accuracy
value: 99.82673267326733
- type: manhattan_ap
value: 95.86094873177069
- type: manhattan_f1
value: 91.26788357178096
- type: manhattan_precision
value: 90.06815968841285
- type: manhattan_recall
value: 92.5
- type: max_accuracy
value: 99.82673267326733
- type: max_ap
value: 95.86094873177069
- type: max_f1
value: 91.26788357178096
- task:
type: Clustering
dataset:
type: mteb/stackexchange-clustering
name: MTEB StackExchangeClustering
config: default
split: test
revision: 6cbc1f7b2bc0622f2e39d2c77fa502909748c259
metrics:
- type: v_measure
value: 73.09533925852372
- task:
type: Clustering
dataset:
type: mteb/stackexchange-clustering-p2p
name: MTEB StackExchangeClusteringP2P
config: default
split: test
revision: 815ca46b2622cec33ccafc3735d572c266efdb44
metrics:
- type: v_measure
value: 45.90745648090035
- task:
type: Reranking
dataset:
type: mteb/stackoverflowdupquestions-reranking
name: MTEB StackOverflowDupQuestions
config: default
split: test
revision: e185fbe320c72810689fc5848eb6114e1ef5ec69
metrics:
- type: map
value: 54.91147686504404
- type: mrr
value: 56.03900082760377
- task:
type: Summarization
dataset:
type: mteb/summeval
name: MTEB SummEval
config: default
split: test
revision: cda12ad7615edc362dbf25a00fdd61d3b1eaf93c
metrics:
- type: cos_sim_pearson
value: 31.46908662038217
- type: cos_sim_spearman
value: 31.40325730367437
- type: dot_pearson
value: 31.469083969291894
- type: dot_spearman
value: 31.40325730367437
- task:
type: Reranking
dataset:
type: C-MTEB/T2Reranking
name: MTEB T2Reranking
config: default
split: dev
revision: None
metrics:
- type: map
value: 66.90300783402137
- type: mrr
value: 77.06451972574179
- task:
type: Retrieval
dataset:
type: C-MTEB/T2Retrieval
name: MTEB T2Retrieval
config: default
split: dev
revision: None
metrics:
- type: map_at_1
value: 25.82
- type: map_at_10
value: 72.32300000000001
- type: map_at_100
value: 76.198
- type: map_at_1000
value: 76.281
- type: map_at_3
value: 50.719
- type: map_at_5
value: 62.326
- type: mrr_at_1
value: 86.599
- type: mrr_at_10
value: 89.751
- type: mrr_at_100
value: 89.876
- type: mrr_at_1000
value: 89.88000000000001
- type: mrr_at_3
value: 89.151
- type: mrr_at_5
value: 89.519
- type: ndcg_at_1
value: 86.599
- type: ndcg_at_10
value: 80.676
- type: ndcg_at_100
value: 85.03
- type: ndcg_at_1000
value: 85.854
- type: ndcg_at_3
value: 82.057
- type: ndcg_at_5
value: 80.537
- type: precision_at_1
value: 86.599
- type: precision_at_10
value: 40.373
- type: precision_at_100
value: 4.95
- type: precision_at_1000
value: 0.514
- type: precision_at_3
value: 71.918
- type: precision_at_5
value: 60.246
- type: recall_at_1
value: 25.82
- type: recall_at_10
value: 79.905
- type: recall_at_100
value: 93.88499999999999
- type: recall_at_1000
value: 98.073
- type: recall_at_3
value: 52.623
- type: recall_at_5
value: 66.233
- task:
type: Classification
dataset:
type: C-MTEB/TNews-classification
name: MTEB TNews
config: default
split: validation
revision: None
metrics:
- type: accuracy
value: 47.050000000000004
- type: f1
value: 45.704071498353294
- task:
type: Retrieval
dataset:
type: trec-covid
name: MTEB TRECCOVID
config: default
split: test
revision: None
metrics:
- type: map_at_1
value: 0.243
- type: map_at_10
value: 2.278
- type: map_at_100
value: 14.221
- type: map_at_1000
value: 33.474
- type: map_at_3
value: 0.7270000000000001
- type: map_at_5
value: 1.183
- type: mrr_at_1
value: 94.0
- type: mrr_at_10
value: 97.0
- type: mrr_at_100
value: 97.0
- type: mrr_at_1000
value: 97.0
- type: mrr_at_3
value: 97.0
- type: mrr_at_5
value: 97.0
- type: ndcg_at_1
value: 90.0
- type: ndcg_at_10
value: 87.249
- type: ndcg_at_100
value: 67.876
- type: ndcg_at_1000
value: 59.205
- type: ndcg_at_3
value: 90.12299999999999
- type: ndcg_at_5
value: 89.126
- type: precision_at_1
value: 94.0
- type: precision_at_10
value: 90.8
- type: precision_at_100
value: 69.28
- type: precision_at_1000
value: 25.85
- type: precision_at_3
value: 94.667
- type: precision_at_5
value: 92.80000000000001
- type: recall_at_1
value: 0.243
- type: recall_at_10
value: 2.392
- type: recall_at_100
value: 16.982
- type: recall_at_1000
value: 55.214
- type: recall_at_3
value: 0.745
- type: recall_at_5
value: 1.2229999999999999
- task:
type: BitextMining
dataset:
type: mteb/tatoeba-bitext-mining
name: MTEB Tatoeba (sqi-eng)
config: sqi-eng
split: test
revision: 9080400076fbadbb4c4dcb136ff4eddc40b42553
metrics:
- type: accuracy
value: 70.5
- type: f1
value: 67.05501804646966
- type: precision
value: 65.73261904761904
- type: recall
value: 70.5
- task:
type: BitextMining
dataset:
type: mteb/tatoeba-bitext-mining
name: MTEB Tatoeba (fry-eng)
config: fry-eng
split: test
revision: 9080400076fbadbb4c4dcb136ff4eddc40b42553
metrics:
- type: accuracy
value: 75.14450867052022
- type: f1
value: 70.98265895953759
- type: precision
value: 69.26782273603082
- type: recall
value: 75.14450867052022
- task:
type: BitextMining
dataset:
type: mteb/tatoeba-bitext-mining
name: MTEB Tatoeba (kur-eng)
config: kur-eng
split: test
revision: 9080400076fbadbb4c4dcb136ff4eddc40b42553
metrics:
- type: accuracy
value: 33.170731707317074
- type: f1
value: 29.92876500193573
- type: precision
value: 28.669145894755648
- type: recall
value: 33.170731707317074
- task:
type: BitextMining
dataset:
type: mteb/tatoeba-bitext-mining
name: MTEB Tatoeba (tur-eng)
config: tur-eng
split: test
revision: 9080400076fbadbb4c4dcb136ff4eddc40b42553
metrics:
- type: accuracy
value: 95.5
- type: f1
value: 94.13333333333333
- type: precision
value: 93.46666666666667
- type: recall
value: 95.5
- task:
type: BitextMining
dataset:
type: mteb/tatoeba-bitext-mining
name: MTEB Tatoeba (deu-eng)
config: deu-eng
split: test
revision: 9080400076fbadbb4c4dcb136ff4eddc40b42553
metrics:
- type: accuracy
value: 99.6
- type: f1
value: 99.46666666666665
- type: precision
value: 99.4
- type: recall
value: 99.6
- task:
type: BitextMining
dataset:
type: mteb/tatoeba-bitext-mining
name: MTEB Tatoeba (nld-eng)
config: nld-eng
split: test
revision: 9080400076fbadbb4c4dcb136ff4eddc40b42553
metrics:
- type: accuracy
value: 97.2
- type: f1
value: 96.39999999999999
- type: precision
value: 96.0
- type: recall
value: 97.2
- task:
type: BitextMining
dataset:
type: mteb/tatoeba-bitext-mining
name: MTEB Tatoeba (ron-eng)
config: ron-eng
split: test
revision: 9080400076fbadbb4c4dcb136ff4eddc40b42553
metrics:
- type: accuracy
value: 94.5
- type: f1
value: 92.99666666666667
- type: precision
value: 92.31666666666666
- type: recall
value: 94.5
- task:
type: BitextMining
dataset:
type: mteb/tatoeba-bitext-mining
name: MTEB Tatoeba (ang-eng)
config: ang-eng
split: test
revision: 9080400076fbadbb4c4dcb136ff4eddc40b42553
metrics:
- type: accuracy
value: 85.82089552238806
- type: f1
value: 81.59203980099502
- type: precision
value: 79.60199004975124
- type: recall
value: 85.82089552238806
- task:
type: BitextMining
dataset:
type: mteb/tatoeba-bitext-mining
name: MTEB Tatoeba (ido-eng)
config: ido-eng
split: test
revision: 9080400076fbadbb4c4dcb136ff4eddc40b42553
metrics:
- type: accuracy
value: 79.5
- type: f1
value: 75.11246031746032
- type: precision
value: 73.38734126984127
- type: recall
value: 79.5
- task:
type: BitextMining
dataset:
type: mteb/tatoeba-bitext-mining
name: MTEB Tatoeba (jav-eng)
config: jav-eng
split: test
revision: 9080400076fbadbb4c4dcb136ff4eddc40b42553
metrics:
- type: accuracy
value: 44.390243902439025
- type: f1
value: 38.48896631823461
- type: precision
value: 36.57220286488579
- type: recall
value: 44.390243902439025
- task:
type: BitextMining
dataset:
type: mteb/tatoeba-bitext-mining
name: MTEB Tatoeba (isl-eng)
config: isl-eng
split: test
revision: 9080400076fbadbb4c4dcb136ff4eddc40b42553
metrics:
- type: accuracy
value: 90.2
- type: f1
value: 87.57333333333334
- type: precision
value: 86.34166666666665
- type: recall
value: 90.2
- task:
type: BitextMining
dataset:
type: mteb/tatoeba-bitext-mining
name: MTEB Tatoeba (slv-eng)
config: slv-eng
split: test
revision: 9080400076fbadbb4c4dcb136ff4eddc40b42553
metrics:
- type: accuracy
value: 88.82138517618469
- type: f1
value: 85.98651854423423
- type: precision
value: 84.79257073424753
- type: recall
value: 88.82138517618469
- task:
type: BitextMining
dataset:
type: mteb/tatoeba-bitext-mining
name: MTEB Tatoeba (cym-eng)
config: cym-eng
split: test
revision: 9080400076fbadbb4c4dcb136ff4eddc40b42553
metrics:
- type: accuracy
value: 77.04347826086956
- type: f1
value: 72.32108147606868
- type: precision
value: 70.37207357859532
- type: recall
value: 77.04347826086956
- task:
type: BitextMining
dataset:
type: mteb/tatoeba-bitext-mining
name: MTEB Tatoeba (kaz-eng)
config: kaz-eng
split: test
revision: 9080400076fbadbb4c4dcb136ff4eddc40b42553
metrics:
- type: accuracy
value: 53.04347826086957
- type: f1
value: 46.88868184955141
- type: precision
value: 44.71730105643149
- type: recall
value: 53.04347826086957
- task:
type: BitextMining
dataset:
type: mteb/tatoeba-bitext-mining
name: MTEB Tatoeba (est-eng)
config: est-eng
split: test
revision: 9080400076fbadbb4c4dcb136ff4eddc40b42553
metrics:
- type: accuracy
value: 68.0
- type: f1
value: 62.891813186813195
- type: precision
value: 61.037906162464985
- type: recall
value: 68.0
- task:
type: BitextMining
dataset:
type: mteb/tatoeba-bitext-mining
name: MTEB Tatoeba (heb-eng)
config: heb-eng
split: test
revision: 9080400076fbadbb4c4dcb136ff4eddc40b42553
metrics:
- type: accuracy
value: 86.3
- type: f1
value: 82.82000000000001
- type: precision
value: 81.25690476190475
- type: recall
value: 86.3
- task:
type: BitextMining
dataset:
type: mteb/tatoeba-bitext-mining
name: MTEB Tatoeba (gla-eng)
config: gla-eng
split: test
revision: 9080400076fbadbb4c4dcb136ff4eddc40b42553
metrics:
- type: accuracy
value: 68.87816646562122
- type: f1
value: 63.53054933272062
- type: precision
value: 61.47807816331196
- type: recall
value: 68.87816646562122
- task:
type: BitextMining
dataset:
type: mteb/tatoeba-bitext-mining
name: MTEB Tatoeba (mar-eng)
config: mar-eng
split: test
revision: 9080400076fbadbb4c4dcb136ff4eddc40b42553
metrics:
- type: accuracy
value: 74.4
- type: f1
value: 68.99388888888889
- type: precision
value: 66.81035714285713
- type: recall
value: 74.4
- task:
type: BitextMining
dataset:
type: mteb/tatoeba-bitext-mining
name: MTEB Tatoeba (lat-eng)
config: lat-eng
split: test
revision: 9080400076fbadbb4c4dcb136ff4eddc40b42553
metrics:
- type: accuracy
value: 90.5
- type: f1
value: 87.93666666666667
- type: precision
value: 86.825
- type: recall
value: 90.5
- task:
type: BitextMining
dataset:
type: mteb/tatoeba-bitext-mining
name: MTEB Tatoeba (bel-eng)
config: bel-eng
split: test
revision: 9080400076fbadbb4c4dcb136ff4eddc40b42553
metrics:
- type: accuracy
value: 90.7
- type: f1
value: 88.09
- type: precision
value: 86.85833333333333
- type: recall
value: 90.7
- task:
type: BitextMining
dataset:
type: mteb/tatoeba-bitext-mining
name: MTEB Tatoeba (pms-eng)
config: pms-eng
split: test
revision: 9080400076fbadbb4c4dcb136ff4eddc40b42553
metrics:
- type: accuracy
value: 67.61904761904762
- type: f1
value: 62.30239247214037
- type: precision
value: 60.340702947845806
- type: recall
value: 67.61904761904762
- task:
type: BitextMining
dataset:
type: mteb/tatoeba-bitext-mining
name: MTEB Tatoeba (gle-eng)
config: gle-eng
split: test
revision: 9080400076fbadbb4c4dcb136ff4eddc40b42553
metrics:
- type: accuracy
value: 77.9
- type: f1
value: 73.81285714285714
- type: precision
value: 72.21570818070818
- type: recall
value: 77.9
- task:
type: BitextMining
dataset:
type: mteb/tatoeba-bitext-mining
name: MTEB Tatoeba (pes-eng)
config: pes-eng
split: test
revision: 9080400076fbadbb4c4dcb136ff4eddc40b42553
metrics:
- type: accuracy
value: 91.8
- type: f1
value: 89.66666666666667
- type: precision
value: 88.66666666666666
- type: recall
value: 91.8
- task:
type: BitextMining
dataset:
type: mteb/tatoeba-bitext-mining
name: MTEB Tatoeba (nob-eng)
config: nob-eng
split: test
revision: 9080400076fbadbb4c4dcb136ff4eddc40b42553
metrics:
- type: accuracy
value: 97.6
- type: f1
value: 96.85666666666665
- type: precision
value: 96.50833333333333
- type: recall
value: 97.6
- task:
type: BitextMining
dataset:
type: mteb/tatoeba-bitext-mining
name: MTEB Tatoeba (bul-eng)
config: bul-eng
split: test
revision: 9080400076fbadbb4c4dcb136ff4eddc40b42553
metrics:
- type: accuracy
value: 95.39999999999999
- type: f1
value: 93.98333333333333
- type: precision
value: 93.30000000000001
- type: recall
value: 95.39999999999999
- task:
type: BitextMining
dataset:
type: mteb/tatoeba-bitext-mining
name: MTEB Tatoeba (cbk-eng)
config: cbk-eng
split: test
revision: 9080400076fbadbb4c4dcb136ff4eddc40b42553
metrics:
- type: accuracy
value: 85.0
- type: f1
value: 81.31538461538462
- type: precision
value: 79.70666666666666
- type: recall
value: 85.0
- task:
type: BitextMining
dataset:
type: mteb/tatoeba-bitext-mining
name: MTEB Tatoeba (hun-eng)
config: hun-eng
split: test
revision: 9080400076fbadbb4c4dcb136ff4eddc40b42553
metrics:
- type: accuracy
value: 91.60000000000001
- type: f1
value: 89.81888888888888
- type: precision
value: 89.08583333333333
- type: recall
value: 91.60000000000001
- task:
type: BitextMining
dataset:
type: mteb/tatoeba-bitext-mining
name: MTEB Tatoeba (uig-eng)
config: uig-eng
split: test
revision: 9080400076fbadbb4c4dcb136ff4eddc40b42553
metrics:
- type: accuracy
value: 44.3
- type: f1
value: 38.8623088023088
- type: precision
value: 37.03755623461505
- type: recall
value: 44.3
- task:
type: BitextMining
dataset:
type: mteb/tatoeba-bitext-mining
name: MTEB Tatoeba (rus-eng)
config: rus-eng
split: test
revision: 9080400076fbadbb4c4dcb136ff4eddc40b42553
metrics:
- type: accuracy
value: 95.19999999999999
- type: f1
value: 93.75
- type: precision
value: 93.05
- type: recall
value: 95.19999999999999
- task:
type: BitextMining
dataset:
type: mteb/tatoeba-bitext-mining
name: MTEB Tatoeba (spa-eng)
config: spa-eng
split: test
revision: 9080400076fbadbb4c4dcb136ff4eddc40b42553
metrics:
- type: accuracy
value: 99.1
- type: f1
value: 98.8
- type: precision
value: 98.65
- type: recall
value: 99.1
- task:
type: BitextMining
dataset:
type: mteb/tatoeba-bitext-mining
name: MTEB Tatoeba (hye-eng)
config: hye-eng
split: test
revision: 9080400076fbadbb4c4dcb136ff4eddc40b42553
metrics:
- type: accuracy
value: 69.6765498652291
- type: f1
value: 63.991785393402644
- type: precision
value: 61.7343729944808
- type: recall
value: 69.6765498652291
- task:
type: BitextMining
dataset:
type: mteb/tatoeba-bitext-mining
name: MTEB Tatoeba (tel-eng)
config: tel-eng
split: test
revision: 9080400076fbadbb4c4dcb136ff4eddc40b42553
metrics:
- type: accuracy
value: 50.0
- type: f1
value: 42.79341029341029
- type: precision
value: 40.25098358431692
- type: recall
value: 50.0
- task:
type: BitextMining
dataset:
type: mteb/tatoeba-bitext-mining
name: MTEB Tatoeba (afr-eng)
config: afr-eng
split: test
revision: 9080400076fbadbb4c4dcb136ff4eddc40b42553
metrics:
- type: accuracy
value: 89.7
- type: f1
value: 87.19023809523809
- type: precision
value: 86.12595238095237
- type: recall
value: 89.7
- task:
type: BitextMining
dataset:
type: mteb/tatoeba-bitext-mining
name: MTEB Tatoeba (mon-eng)
config: mon-eng
split: test
revision: 9080400076fbadbb4c4dcb136ff4eddc40b42553
metrics:
- type: accuracy
value: 42.72727272727273
- type: f1
value: 37.78789518562245
- type: precision
value: 36.24208471267295
- type: recall
value: 42.72727272727273
- task:
type: BitextMining
dataset:
type: mteb/tatoeba-bitext-mining
name: MTEB Tatoeba (arz-eng)
config: arz-eng
split: test
revision: 9080400076fbadbb4c4dcb136ff4eddc40b42553
metrics:
- type: accuracy
value: 75.26205450733752
- type: f1
value: 70.72842833849123
- type: precision
value: 68.93256464011182
- type: recall
value: 75.26205450733752
- task:
type: BitextMining
dataset:
type: mteb/tatoeba-bitext-mining
name: MTEB Tatoeba (hrv-eng)
config: hrv-eng
split: test
revision: 9080400076fbadbb4c4dcb136ff4eddc40b42553
metrics:
- type: accuracy
value: 95.19999999999999
- type: f1
value: 93.96666666666668
- type: precision
value: 93.42
- type: recall
value: 95.19999999999999
- task:
type: BitextMining
dataset:
type: mteb/tatoeba-bitext-mining
name: MTEB Tatoeba (nov-eng)
config: nov-eng
split: test
revision: 9080400076fbadbb4c4dcb136ff4eddc40b42553
metrics:
- type: accuracy
value: 76.26459143968872
- type: f1
value: 72.40190419178747
- type: precision
value: 70.84954604409856
- type: recall
value: 76.26459143968872
- task:
type: BitextMining
dataset:
type: mteb/tatoeba-bitext-mining
name: MTEB Tatoeba (gsw-eng)
config: gsw-eng
split: test
revision: 9080400076fbadbb4c4dcb136ff4eddc40b42553
metrics:
- type: accuracy
value: 59.82905982905983
- type: f1
value: 52.2100122100122
- type: precision
value: 49.52516619183286
- type: recall
value: 59.82905982905983
- task:
type: BitextMining
dataset:
type: mteb/tatoeba-bitext-mining
name: MTEB Tatoeba (nds-eng)
config: nds-eng
split: test
revision: 9080400076fbadbb4c4dcb136ff4eddc40b42553
metrics:
- type: accuracy
value: 81.69999999999999
- type: f1
value: 77.41714285714286
- type: precision
value: 75.64833333333334
- type: recall
value: 81.69999999999999
- task:
type: BitextMining
dataset:
type: mteb/tatoeba-bitext-mining
name: MTEB Tatoeba (ukr-eng)
config: ukr-eng
split: test
revision: 9080400076fbadbb4c4dcb136ff4eddc40b42553
metrics:
- type: accuracy
value: 95.5
- type: f1
value: 94.45
- type: precision
value: 93.93333333333334
- type: recall
value: 95.5
- task:
type: BitextMining
dataset:
type: mteb/tatoeba-bitext-mining
name: MTEB Tatoeba (uzb-eng)
config: uzb-eng
split: test
revision: 9080400076fbadbb4c4dcb136ff4eddc40b42553
metrics:
- type: accuracy
value: 58.41121495327103
- type: f1
value: 52.73495974430554
- type: precision
value: 50.717067200712066
- type: recall
value: 58.41121495327103
- task:
type: BitextMining
dataset:
type: mteb/tatoeba-bitext-mining
name: MTEB Tatoeba (lit-eng)
config: lit-eng
split: test
revision: 9080400076fbadbb4c4dcb136ff4eddc40b42553
metrics:
- type: accuracy
value: 73.3
- type: f1
value: 69.20371794871795
- type: precision
value: 67.6597557997558
- type: recall
value: 73.3
- task:
type: BitextMining
dataset:
type: mteb/tatoeba-bitext-mining
name: MTEB Tatoeba (ina-eng)
config: ina-eng
split: test
revision: 9080400076fbadbb4c4dcb136ff4eddc40b42553
metrics:
- type: accuracy
value: 96.5
- type: f1
value: 95.51666666666667
- type: precision
value: 95.05
- type: recall
value: 96.5
- task:
type: BitextMining
dataset:
type: mteb/tatoeba-bitext-mining
name: MTEB Tatoeba (lfn-eng)
config: lfn-eng
split: test
revision: 9080400076fbadbb4c4dcb136ff4eddc40b42553
metrics:
- type: accuracy
value: 78.4
- type: f1
value: 73.88856643356644
- type: precision
value: 72.01373015873016
- type: recall
value: 78.4
- task:
type: BitextMining
dataset:
type: mteb/tatoeba-bitext-mining
name: MTEB Tatoeba (zsm-eng)
config: zsm-eng
split: test
revision: 9080400076fbadbb4c4dcb136ff4eddc40b42553
metrics:
- type: accuracy
value: 95.3
- type: f1
value: 94.09666666666668
- type: precision
value: 93.53333333333332
- type: recall
value: 95.3
- task:
type: BitextMining
dataset:
type: mteb/tatoeba-bitext-mining
name: MTEB Tatoeba (ita-eng)
config: ita-eng
split: test
revision: 9080400076fbadbb4c4dcb136ff4eddc40b42553
metrics:
- type: accuracy
value: 93.7
- type: f1
value: 91.94
- type: precision
value: 91.10833333333333
- type: recall
value: 93.7
- task:
type: BitextMining
dataset:
type: mteb/tatoeba-bitext-mining
name: MTEB Tatoeba (cmn-eng)
config: cmn-eng
split: test
revision: 9080400076fbadbb4c4dcb136ff4eddc40b42553
metrics:
- type: accuracy
value: 96.8
- type: f1
value: 95.89999999999999
- type: precision
value: 95.46666666666668
- type: recall
value: 96.8
- task:
type: BitextMining
dataset:
type: mteb/tatoeba-bitext-mining
name: MTEB Tatoeba (lvs-eng)
config: lvs-eng
split: test
revision: 9080400076fbadbb4c4dcb136ff4eddc40b42553
metrics:
- type: accuracy
value: 70.5
- type: f1
value: 66.00635642135641
- type: precision
value: 64.36345238095238
- type: recall
value: 70.5
- task:
type: BitextMining
dataset:
type: mteb/tatoeba-bitext-mining
name: MTEB Tatoeba (glg-eng)
config: glg-eng
split: test
revision: 9080400076fbadbb4c4dcb136ff4eddc40b42553
metrics:
- type: accuracy
value: 92.4
- type: f1
value: 90.44388888888889
- type: precision
value: 89.5767857142857
- type: recall
value: 92.4
- task:
type: BitextMining
dataset:
type: mteb/tatoeba-bitext-mining
name: MTEB Tatoeba (ceb-eng)
config: ceb-eng
split: test
revision: 9080400076fbadbb4c4dcb136ff4eddc40b42553
metrics:
- type: accuracy
value: 48.0
- type: f1
value: 43.15372775372776
- type: precision
value: 41.53152510162313
- type: recall
value: 48.0
- task:
type: BitextMining
dataset:
type: mteb/tatoeba-bitext-mining
name: MTEB Tatoeba (bre-eng)
config: bre-eng
split: test
revision: 9080400076fbadbb4c4dcb136ff4eddc40b42553
metrics:
- type: accuracy
value: 16.7
- type: f1
value: 14.198431372549017
- type: precision
value: 13.411765873015872
- type: recall
value: 16.7
- task:
type: BitextMining
dataset:
type: mteb/tatoeba-bitext-mining
name: MTEB Tatoeba (ben-eng)
config: ben-eng
split: test
revision: 9080400076fbadbb4c4dcb136ff4eddc40b42553
metrics:
- type: accuracy
value: 85.7
- type: f1
value: 81.81666666666666
- type: precision
value: 80.10833333333332
- type: recall
value: 85.7
- task:
type: BitextMining
dataset:
type: mteb/tatoeba-bitext-mining
name: MTEB Tatoeba (swg-eng)
config: swg-eng
split: test
revision: 9080400076fbadbb4c4dcb136ff4eddc40b42553
metrics:
- type: accuracy
value: 69.64285714285714
- type: f1
value: 64.745670995671
- type: precision
value: 62.916666666666664
- type: recall
value: 69.64285714285714
- task:
type: BitextMining
dataset:
type: mteb/tatoeba-bitext-mining
name: MTEB Tatoeba (arq-eng)
config: arq-eng
split: test
revision: 9080400076fbadbb4c4dcb136ff4eddc40b42553
metrics:
- type: accuracy
value: 54.665203073545555
- type: f1
value: 48.55366630916923
- type: precision
value: 46.35683318998357
- type: recall
value: 54.665203073545555
- task:
type: BitextMining
dataset:
type: mteb/tatoeba-bitext-mining
name: MTEB Tatoeba (kab-eng)
config: kab-eng
split: test
revision: 9080400076fbadbb4c4dcb136ff4eddc40b42553
metrics:
- type: accuracy
value: 4.8
- type: f1
value: 3.808587223587223
- type: precision
value: 3.5653174603174604
- type: recall
value: 4.8
- task:
type: BitextMining
dataset:
type: mteb/tatoeba-bitext-mining
name: MTEB Tatoeba (fra-eng)
config: fra-eng
split: test
revision: 9080400076fbadbb4c4dcb136ff4eddc40b42553
metrics:
- type: accuracy
value: 96.6
- type: f1
value: 95.77333333333333
- type: precision
value: 95.39166666666667
- type: recall
value: 96.6
- task:
type: BitextMining
dataset:
type: mteb/tatoeba-bitext-mining
name: MTEB Tatoeba (por-eng)
config: por-eng
split: test
revision: 9080400076fbadbb4c4dcb136ff4eddc40b42553
metrics:
- type: accuracy
value: 95.39999999999999
- type: f1
value: 94.44
- type: precision
value: 93.975
- type: recall
value: 95.39999999999999
- task:
type: BitextMining
dataset:
type: mteb/tatoeba-bitext-mining
name: MTEB Tatoeba (tat-eng)
config: tat-eng
split: test
revision: 9080400076fbadbb4c4dcb136ff4eddc40b42553
metrics:
- type: accuracy
value: 42.0
- type: f1
value: 37.024908424908425
- type: precision
value: 35.365992063492065
- type: recall
value: 42.0
- task:
type: BitextMining
dataset:
type: mteb/tatoeba-bitext-mining
name: MTEB Tatoeba (oci-eng)
config: oci-eng
split: test
revision: 9080400076fbadbb4c4dcb136ff4eddc40b42553
metrics:
- type: accuracy
value: 66.7
- type: f1
value: 62.20460835058661
- type: precision
value: 60.590134587634594
- type: recall
value: 66.7
- task:
type: BitextMining
dataset:
type: mteb/tatoeba-bitext-mining
name: MTEB Tatoeba (pol-eng)
config: pol-eng
split: test
revision: 9080400076fbadbb4c4dcb136ff4eddc40b42553
metrics:
- type: accuracy
value: 97.3
- type: f1
value: 96.46666666666667
- type: precision
value: 96.06666666666668
- type: recall
value: 97.3
- task:
type: BitextMining
dataset:
type: mteb/tatoeba-bitext-mining
name: MTEB Tatoeba (war-eng)
config: war-eng
split: test
revision: 9080400076fbadbb4c4dcb136ff4eddc40b42553
metrics:
- type: accuracy
value: 47.3
- type: f1
value: 41.96905408317173
- type: precision
value: 40.18741402116402
- type: recall
value: 47.3
- task:
type: BitextMining
dataset:
type: mteb/tatoeba-bitext-mining
name: MTEB Tatoeba (aze-eng)
config: aze-eng
split: test
revision: 9080400076fbadbb4c4dcb136ff4eddc40b42553
metrics:
- type: accuracy
value: 80.2
- type: f1
value: 76.22690476190476
- type: precision
value: 74.63539682539682
- type: recall
value: 80.2
- task:
type: BitextMining
dataset:
type: mteb/tatoeba-bitext-mining
name: MTEB Tatoeba (vie-eng)
config: vie-eng
split: test
revision: 9080400076fbadbb4c4dcb136ff4eddc40b42553
metrics:
- type: accuracy
value: 96.0
- type: f1
value: 94.83333333333333
- type: precision
value: 94.26666666666668
- type: recall
value: 96.0
- task:
type: BitextMining
dataset:
type: mteb/tatoeba-bitext-mining
name: MTEB Tatoeba (nno-eng)
config: nno-eng
split: test
revision: 9080400076fbadbb4c4dcb136ff4eddc40b42553
metrics:
- type: accuracy
value: 89.7
- type: f1
value: 87.24333333333334
- type: precision
value: 86.17
- type: recall
value: 89.7
- task:
type: BitextMining
dataset:
type: mteb/tatoeba-bitext-mining
name: MTEB Tatoeba (cha-eng)
config: cha-eng
split: test
revision: 9080400076fbadbb4c4dcb136ff4eddc40b42553
metrics:
- type: accuracy
value: 50.36496350364964
- type: f1
value: 44.795520780922246
- type: precision
value: 43.09002433090024
- type: recall
value: 50.36496350364964
- task:
type: BitextMining
dataset:
type: mteb/tatoeba-bitext-mining
name: MTEB Tatoeba (mhr-eng)
config: mhr-eng
split: test
revision: 9080400076fbadbb4c4dcb136ff4eddc40b42553
metrics:
- type: accuracy
value: 18.8
- type: f1
value: 16.242864357864356
- type: precision
value: 15.466596638655464
- type: recall
value: 18.8
- task:
type: BitextMining
dataset:
type: mteb/tatoeba-bitext-mining
name: MTEB Tatoeba (dan-eng)
config: dan-eng
split: test
revision: 9080400076fbadbb4c4dcb136ff4eddc40b42553
metrics:
- type: accuracy
value: 95.19999999999999
- type: f1
value: 93.92333333333333
- type: precision
value: 93.30833333333332
- type: recall
value: 95.19999999999999
- task:
type: BitextMining
dataset:
type: mteb/tatoeba-bitext-mining
name: MTEB Tatoeba (ell-eng)
config: ell-eng
split: test
revision: 9080400076fbadbb4c4dcb136ff4eddc40b42553
metrics:
- type: accuracy
value: 93.4
- type: f1
value: 91.42333333333333
- type: precision
value: 90.50833333333334
- type: recall
value: 93.4
- task:
type: BitextMining
dataset:
type: mteb/tatoeba-bitext-mining
name: MTEB Tatoeba (amh-eng)
config: amh-eng
split: test
revision: 9080400076fbadbb4c4dcb136ff4eddc40b42553
metrics:
- type: accuracy
value: 26.190476190476193
- type: f1
value: 22.05208151636723
- type: precision
value: 21.09292328042328
- type: recall
value: 26.190476190476193
- task:
type: BitextMining
dataset:
type: mteb/tatoeba-bitext-mining
name: MTEB Tatoeba (pam-eng)
config: pam-eng
split: test
revision: 9080400076fbadbb4c4dcb136ff4eddc40b42553
metrics:
- type: accuracy
value: 17.2
- type: f1
value: 14.021009731460952
- type: precision
value: 13.1389886698243
- type: recall
value: 17.2
- task:
type: BitextMining
dataset:
type: mteb/tatoeba-bitext-mining
name: MTEB Tatoeba (hsb-eng)
config: hsb-eng
split: test
revision: 9080400076fbadbb4c4dcb136ff4eddc40b42553
metrics:
- type: accuracy
value: 78.67494824016563
- type: f1
value: 74.24430641821947
- type: precision
value: 72.50747642051991
- type: recall
value: 78.67494824016563
- task:
type: BitextMining
dataset:
type: mteb/tatoeba-bitext-mining
name: MTEB Tatoeba (srp-eng)
config: srp-eng
split: test
revision: 9080400076fbadbb4c4dcb136ff4eddc40b42553
metrics:
- type: accuracy
value: 94.19999999999999
- type: f1
value: 92.54
- type: precision
value: 91.75833333333334
- type: recall
value: 94.19999999999999
- task:
type: BitextMining
dataset:
type: mteb/tatoeba-bitext-mining
name: MTEB Tatoeba (epo-eng)
config: epo-eng
split: test
revision: 9080400076fbadbb4c4dcb136ff4eddc40b42553
metrics:
- type: accuracy
value: 90.2
- type: f1
value: 87.78666666666666
- type: precision
value: 86.69833333333334
- type: recall
value: 90.2
- task:
type: BitextMining
dataset:
type: mteb/tatoeba-bitext-mining
name: MTEB Tatoeba (kzj-eng)
config: kzj-eng
split: test
revision: 9080400076fbadbb4c4dcb136ff4eddc40b42553
metrics:
- type: accuracy
value: 14.7
- type: f1
value: 12.19206214842218
- type: precision
value: 11.526261904761904
- type: recall
value: 14.7
- task:
type: BitextMining
dataset:
type: mteb/tatoeba-bitext-mining
name: MTEB Tatoeba (awa-eng)
config: awa-eng
split: test
revision: 9080400076fbadbb4c4dcb136ff4eddc40b42553
metrics:
- type: accuracy
value: 73.16017316017316
- type: f1
value: 67.44858316286889
- type: precision
value: 65.23809523809523
- type: recall
value: 73.16017316017316
- task:
type: BitextMining
dataset:
type: mteb/tatoeba-bitext-mining
name: MTEB Tatoeba (fao-eng)
config: fao-eng
split: test
revision: 9080400076fbadbb4c4dcb136ff4eddc40b42553
metrics:
- type: accuracy
value: 75.19083969465649
- type: f1
value: 70.33078880407125
- type: precision
value: 68.3969465648855
- type: recall
value: 75.19083969465649
- task:
type: BitextMining
dataset:
type: mteb/tatoeba-bitext-mining
name: MTEB Tatoeba (mal-eng)
config: mal-eng
split: test
revision: 9080400076fbadbb4c4dcb136ff4eddc40b42553
metrics:
- type: accuracy
value: 62.154294032023294
- type: f1
value: 55.86030821838681
- type: precision
value: 53.53509623160277
- type: recall
value: 62.154294032023294
- task:
type: BitextMining
dataset:
type: mteb/tatoeba-bitext-mining
name: MTEB Tatoeba (ile-eng)
config: ile-eng
split: test
revision: 9080400076fbadbb4c4dcb136ff4eddc40b42553
metrics:
- type: accuracy
value: 86.8
- type: f1
value: 83.9652380952381
- type: precision
value: 82.84242424242424
- type: recall
value: 86.8
- task:
type: BitextMining
dataset:
type: mteb/tatoeba-bitext-mining
name: MTEB Tatoeba (bos-eng)
config: bos-eng
split: test
revision: 9080400076fbadbb4c4dcb136ff4eddc40b42553
metrics:
- type: accuracy
value: 93.50282485875707
- type: f1
value: 91.54425612052731
- type: precision
value: 90.65442561205272
- type: recall
value: 93.50282485875707
- task:
type: BitextMining
dataset:
type: mteb/tatoeba-bitext-mining
name: MTEB Tatoeba (cor-eng)
config: cor-eng
split: test
revision: 9080400076fbadbb4c4dcb136ff4eddc40b42553
metrics:
- type: accuracy
value: 11.4
- type: f1
value: 9.189775870222714
- type: precision
value: 8.66189886502811
- type: recall
value: 11.4
- task:
type: BitextMining
dataset:
type: mteb/tatoeba-bitext-mining
name: MTEB Tatoeba (cat-eng)
config: cat-eng
split: test
revision: 9080400076fbadbb4c4dcb136ff4eddc40b42553
metrics:
- type: accuracy
value: 93.4
- type: f1
value: 91.88666666666666
- type: precision
value: 91.21444444444444
- type: recall
value: 93.4
- task:
type: BitextMining
dataset:
type: mteb/tatoeba-bitext-mining
name: MTEB Tatoeba (eus-eng)
config: eus-eng
split: test
revision: 9080400076fbadbb4c4dcb136ff4eddc40b42553
metrics:
- type: accuracy
value: 46.0
- type: f1
value: 40.51069226095542
- type: precision
value: 38.57804926010808
- type: recall
value: 46.0
- task:
type: BitextMining
dataset:
type: mteb/tatoeba-bitext-mining
name: MTEB Tatoeba (yue-eng)
config: yue-eng
split: test
revision: 9080400076fbadbb4c4dcb136ff4eddc40b42553
metrics:
- type: accuracy
value: 91.0
- type: f1
value: 89.11333333333333
- type: precision
value: 88.27000000000001
- type: recall
value: 91.0
- task:
type: BitextMining
dataset:
type: mteb/tatoeba-bitext-mining
name: MTEB Tatoeba (swe-eng)
config: swe-eng
split: test
revision: 9080400076fbadbb4c4dcb136ff4eddc40b42553
metrics:
- type: accuracy
value: 94.39999999999999
- type: f1
value: 92.95
- type: precision
value: 92.27000000000001
- type: recall
value: 94.39999999999999
- task:
type: BitextMining
dataset:
type: mteb/tatoeba-bitext-mining
name: MTEB Tatoeba (dtp-eng)
config: dtp-eng
split: test
revision: 9080400076fbadbb4c4dcb136ff4eddc40b42553
metrics:
- type: accuracy
value: 14.2
- type: f1
value: 11.73701698770113
- type: precision
value: 11.079207014736676
- type: recall
value: 14.2
- task:
type: BitextMining
dataset:
type: mteb/tatoeba-bitext-mining
name: MTEB Tatoeba (kat-eng)
config: kat-eng
split: test
revision: 9080400076fbadbb4c4dcb136ff4eddc40b42553
metrics:
- type: accuracy
value: 65.14745308310992
- type: f1
value: 59.665707393589415
- type: precision
value: 57.560853653346946
- type: recall
value: 65.14745308310992
- task:
type: BitextMining
dataset:
type: mteb/tatoeba-bitext-mining
name: MTEB Tatoeba (jpn-eng)
config: jpn-eng
split: test
revision: 9080400076fbadbb4c4dcb136ff4eddc40b42553
metrics:
- type: accuracy
value: 95.39999999999999
- type: f1
value: 94.0
- type: precision
value: 93.33333333333333
- type: recall
value: 95.39999999999999
- task:
type: BitextMining
dataset:
type: mteb/tatoeba-bitext-mining
name: MTEB Tatoeba (csb-eng)
config: csb-eng
split: test
revision: 9080400076fbadbb4c4dcb136ff4eddc40b42553
metrics:
- type: accuracy
value: 69.56521739130434
- type: f1
value: 62.92490118577074
- type: precision
value: 60.27009222661397
- type: recall
value: 69.56521739130434
- task:
type: BitextMining
dataset:
type: mteb/tatoeba-bitext-mining
name: MTEB Tatoeba (xho-eng)
config: xho-eng
split: test
revision: 9080400076fbadbb4c4dcb136ff4eddc40b42553
metrics:
- type: accuracy
value: 40.140845070422536
- type: f1
value: 35.96411804158283
- type: precision
value: 34.89075869357559
- type: recall
value: 40.140845070422536
- task:
type: BitextMining
dataset:
type: mteb/tatoeba-bitext-mining
name: MTEB Tatoeba (orv-eng)
config: orv-eng
split: test
revision: 9080400076fbadbb4c4dcb136ff4eddc40b42553
metrics:
- type: accuracy
value: 65.86826347305389
- type: f1
value: 59.646248628284546
- type: precision
value: 57.22982606216139
- type: recall
value: 65.86826347305389
- task:
type: BitextMining
dataset:
type: mteb/tatoeba-bitext-mining
name: MTEB Tatoeba (ind-eng)
config: ind-eng
split: test
revision: 9080400076fbadbb4c4dcb136ff4eddc40b42553
metrics:
- type: accuracy
value: 94.89999999999999
- type: f1
value: 93.48333333333333
- type: precision
value: 92.83666666666667
- type: recall
value: 94.89999999999999
- task:
type: BitextMining
dataset:
type: mteb/tatoeba-bitext-mining
name: MTEB Tatoeba (tuk-eng)
config: tuk-eng
split: test
revision: 9080400076fbadbb4c4dcb136ff4eddc40b42553
metrics:
- type: accuracy
value: 47.783251231527096
- type: f1
value: 42.006447302013804
- type: precision
value: 40.12747105111637
- type: recall
value: 47.783251231527096
- task:
type: BitextMining
dataset:
type: mteb/tatoeba-bitext-mining
name: MTEB Tatoeba (max-eng)
config: max-eng
split: test
revision: 9080400076fbadbb4c4dcb136ff4eddc40b42553
metrics:
- type: accuracy
value: 69.71830985915493
- type: f1
value: 64.80266212660578
- type: precision
value: 63.08098591549296
- type: recall
value: 69.71830985915493
- task:
type: BitextMining
dataset:
type: mteb/tatoeba-bitext-mining
name: MTEB Tatoeba (swh-eng)
config: swh-eng
split: test
revision: 9080400076fbadbb4c4dcb136ff4eddc40b42553
metrics:
- type: accuracy
value: 67.94871794871796
- type: f1
value: 61.59912309912309
- type: precision
value: 59.17338217338218
- type: recall
value: 67.94871794871796
- task:
type: BitextMining
dataset:
type: mteb/tatoeba-bitext-mining
name: MTEB Tatoeba (hin-eng)
config: hin-eng
split: test
revision: 9080400076fbadbb4c4dcb136ff4eddc40b42553
metrics:
- type: accuracy
value: 96.39999999999999
- type: f1
value: 95.28333333333335
- type: precision
value: 94.75
- type: recall
value: 96.39999999999999
- task:
type: BitextMining
dataset:
type: mteb/tatoeba-bitext-mining
name: MTEB Tatoeba (dsb-eng)
config: dsb-eng
split: test
revision: 9080400076fbadbb4c4dcb136ff4eddc40b42553
metrics:
- type: accuracy
value: 70.14613778705638
- type: f1
value: 65.4349338900487
- type: precision
value: 63.57599255302805
- type: recall
value: 70.14613778705638
- task:
type: BitextMining
dataset:
type: mteb/tatoeba-bitext-mining
name: MTEB Tatoeba (ber-eng)
config: ber-eng
split: test
revision: 9080400076fbadbb4c4dcb136ff4eddc40b42553
metrics:
- type: accuracy
value: 9.2
- type: f1
value: 7.622184434339607
- type: precision
value: 7.287048159682417
- type: recall
value: 9.2
- task:
type: BitextMining
dataset:
type: mteb/tatoeba-bitext-mining
name: MTEB Tatoeba (tam-eng)
config: tam-eng
split: test
revision: 9080400076fbadbb4c4dcb136ff4eddc40b42553
metrics:
- type: accuracy
value: 77.85016286644951
- type: f1
value: 72.83387622149837
- type: precision
value: 70.58450959102424
- type: recall
value: 77.85016286644951
- task:
type: BitextMining
dataset:
type: mteb/tatoeba-bitext-mining
name: MTEB Tatoeba (slk-eng)
config: slk-eng
split: test
revision: 9080400076fbadbb4c4dcb136ff4eddc40b42553
metrics:
- type: accuracy
value: 90.8
- type: f1
value: 88.84333333333333
- type: precision
value: 87.96666666666665
- type: recall
value: 90.8
- task:
type: BitextMining
dataset:
type: mteb/tatoeba-bitext-mining
name: MTEB Tatoeba (tgl-eng)
config: tgl-eng
split: test
revision: 9080400076fbadbb4c4dcb136ff4eddc40b42553
metrics:
- type: accuracy
value: 94.6
- type: f1
value: 93.14
- type: precision
value: 92.49833333333333
- type: recall
value: 94.6
- task:
type: BitextMining
dataset:
type: mteb/tatoeba-bitext-mining
name: MTEB Tatoeba (ast-eng)
config: ast-eng
split: test
revision: 9080400076fbadbb4c4dcb136ff4eddc40b42553
metrics:
- type: accuracy
value: 84.25196850393701
- type: f1
value: 80.94488188976378
- type: precision
value: 79.65879265091863
- type: recall
value: 84.25196850393701
- task:
type: BitextMining
dataset:
type: mteb/tatoeba-bitext-mining
name: MTEB Tatoeba (mkd-eng)
config: mkd-eng
split: test
revision: 9080400076fbadbb4c4dcb136ff4eddc40b42553
metrics:
- type: accuracy
value: 89.5
- type: f1
value: 86.89666666666666
- type: precision
value: 85.7
- type: recall
value: 89.5
- task:
type: BitextMining
dataset:
type: mteb/tatoeba-bitext-mining
name: MTEB Tatoeba (khm-eng)
config: khm-eng
split: test
revision: 9080400076fbadbb4c4dcb136ff4eddc40b42553
metrics:
- type: accuracy
value: 42.797783933518005
- type: f1
value: 37.30617360155193
- type: precision
value: 35.34933825792552
- type: recall
value: 42.797783933518005
- task:
type: BitextMining
dataset:
type: mteb/tatoeba-bitext-mining
name: MTEB Tatoeba (ces-eng)
config: ces-eng
split: test
revision: 9080400076fbadbb4c4dcb136ff4eddc40b42553
metrics:
- type: accuracy
value: 96.1
- type: f1
value: 94.93333333333332
- type: precision
value: 94.38333333333333
- type: recall
value: 96.1
- task:
type: BitextMining
dataset:
type: mteb/tatoeba-bitext-mining
name: MTEB Tatoeba (tzl-eng)
config: tzl-eng
split: test
revision: 9080400076fbadbb4c4dcb136ff4eddc40b42553
metrics:
- type: accuracy
value: 54.807692307692314
- type: f1
value: 49.506903353057204
- type: precision
value: 47.54807692307693
- type: recall
value: 54.807692307692314
- task:
type: BitextMining
dataset:
type: mteb/tatoeba-bitext-mining
name: MTEB Tatoeba (urd-eng)
config: urd-eng
split: test
revision: 9080400076fbadbb4c4dcb136ff4eddc40b42553
metrics:
- type: accuracy
value: 87.1
- type: f1
value: 83.61857142857143
- type: precision
value: 81.975
- type: recall
value: 87.1
- task:
type: BitextMining
dataset:
type: mteb/tatoeba-bitext-mining
name: MTEB Tatoeba (ara-eng)
config: ara-eng
split: test
revision: 9080400076fbadbb4c4dcb136ff4eddc40b42553
metrics:
- type: accuracy
value: 91.10000000000001
- type: f1
value: 88.76333333333332
- type: precision
value: 87.67
- type: recall
value: 91.10000000000001
- task:
type: BitextMining
dataset:
type: mteb/tatoeba-bitext-mining
name: MTEB Tatoeba (kor-eng)
config: kor-eng
split: test
revision: 9080400076fbadbb4c4dcb136ff4eddc40b42553
metrics:
- type: accuracy
value: 93.10000000000001
- type: f1
value: 91.28999999999999
- type: precision
value: 90.44500000000001
- type: recall
value: 93.10000000000001
- task:
type: BitextMining
dataset:
type: mteb/tatoeba-bitext-mining
name: MTEB Tatoeba (yid-eng)
config: yid-eng
split: test
revision: 9080400076fbadbb4c4dcb136ff4eddc40b42553
metrics:
- type: accuracy
value: 39.97641509433962
- type: f1
value: 33.12271889998028
- type: precision
value: 30.95185381542554
- type: recall
value: 39.97641509433962
- task:
type: BitextMining
dataset:
type: mteb/tatoeba-bitext-mining
name: MTEB Tatoeba (fin-eng)
config: fin-eng
split: test
revision: 9080400076fbadbb4c4dcb136ff4eddc40b42553
metrics:
- type: accuracy
value: 92.60000000000001
- type: f1
value: 90.69
- type: precision
value: 89.84500000000001
- type: recall
value: 92.60000000000001
- task:
type: BitextMining
dataset:
type: mteb/tatoeba-bitext-mining
name: MTEB Tatoeba (tha-eng)
config: tha-eng
split: test
revision: 9080400076fbadbb4c4dcb136ff4eddc40b42553
metrics:
- type: accuracy
value: 95.07299270072993
- type: f1
value: 93.64355231143554
- type: precision
value: 92.94403892944038
- type: recall
value: 95.07299270072993
- task:
type: BitextMining
dataset:
type: mteb/tatoeba-bitext-mining
name: MTEB Tatoeba (wuu-eng)
config: wuu-eng
split: test
revision: 9080400076fbadbb4c4dcb136ff4eddc40b42553
metrics:
- type: accuracy
value: 91.9
- type: f1
value: 89.61333333333333
- type: precision
value: 88.53333333333333
- type: recall
value: 91.9
- task:
type: Clustering
dataset:
type: C-MTEB/ThuNewsClusteringP2P
name: MTEB ThuNewsClusteringP2P
config: default
split: test
revision: None
metrics:
- type: v_measure
value: 64.68478289806511
- task:
type: Clustering
dataset:
type: C-MTEB/ThuNewsClusteringS2S
name: MTEB ThuNewsClusteringS2S
config: default
split: test
revision: None
metrics:
- type: v_measure
value: 57.53010296184097
- task:
type: Retrieval
dataset:
type: webis-touche2020
name: MTEB Touche2020
config: default
split: test
revision: None
metrics:
- type: map_at_1
value: 2.519
- type: map_at_10
value: 10.31
- type: map_at_100
value: 16.027
- type: map_at_1000
value: 17.827
- type: map_at_3
value: 5.721
- type: map_at_5
value: 7.7829999999999995
- type: mrr_at_1
value: 34.694
- type: mrr_at_10
value: 52.642999999999994
- type: mrr_at_100
value: 53.366
- type: mrr_at_1000
value: 53.366
- type: mrr_at_3
value: 48.638999999999996
- type: mrr_at_5
value: 50.578
- type: ndcg_at_1
value: 31.633
- type: ndcg_at_10
value: 26.394000000000002
- type: ndcg_at_100
value: 36.41
- type: ndcg_at_1000
value: 49.206
- type: ndcg_at_3
value: 31.694
- type: ndcg_at_5
value: 29.529
- type: precision_at_1
value: 34.694
- type: precision_at_10
value: 23.469
- type: precision_at_100
value: 7.286
- type: precision_at_1000
value: 1.5610000000000002
- type: precision_at_3
value: 34.014
- type: precision_at_5
value: 29.796
- type: recall_at_1
value: 2.519
- type: recall_at_10
value: 17.091
- type: recall_at_100
value: 45.429
- type: recall_at_1000
value: 84.621
- type: recall_at_3
value: 7.208
- type: recall_at_5
value: 10.523
- task:
type: Classification
dataset:
type: mteb/toxic_conversations_50k
name: MTEB ToxicConversationsClassification
config: default
split: test
revision: d7c0de2777da35d6aae2200a62c6e0e5af397c4c
metrics:
- type: accuracy
value: 69.58659999999999
- type: ap
value: 14.735696532619
- type: f1
value: 54.23517220069903
- task:
type: Classification
dataset:
type: mteb/tweet_sentiment_extraction
name: MTEB TweetSentimentExtractionClassification
config: default
split: test
revision: d604517c81ca91fe16a244d1248fc021f9ecee7a
metrics:
- type: accuracy
value: 63.723825693265425
- type: f1
value: 64.02405729449103
- task:
type: Clustering
dataset:
type: mteb/twentynewsgroups-clustering
name: MTEB TwentyNewsgroupsClustering
config: default
split: test
revision: 6125ec4e24fa026cec8a478383ee943acfbd5449
metrics:
- type: v_measure
value: 54.310161547491006
- task:
type: PairClassification
dataset:
type: mteb/twittersemeval2015-pairclassification
name: MTEB TwitterSemEval2015
config: default
split: test
revision: 70970daeab8776df92f5ea462b6173c0b46fd2d1
metrics:
- type: cos_sim_accuracy
value: 88.77630088812064
- type: cos_sim_ap
value: 81.61725457333809
- type: cos_sim_f1
value: 74.91373801916932
- type: cos_sim_precision
value: 72.63940520446097
- type: cos_sim_recall
value: 77.33509234828496
- type: dot_accuracy
value: 88.77630088812064
- type: dot_ap
value: 81.61725317476251
- type: dot_f1
value: 74.91373801916932
- type: dot_precision
value: 72.63940520446097
- type: dot_recall
value: 77.33509234828496
- type: euclidean_accuracy
value: 88.77630088812064
- type: euclidean_ap
value: 81.61724596869566
- type: euclidean_f1
value: 74.91373801916932
- type: euclidean_precision
value: 72.63940520446097
- type: euclidean_recall
value: 77.33509234828496
- type: manhattan_accuracy
value: 88.67497168742922
- type: manhattan_ap
value: 81.430251048948
- type: manhattan_f1
value: 74.79593118171543
- type: manhattan_precision
value: 71.3635274382938
- type: manhattan_recall
value: 78.57519788918206
- type: max_accuracy
value: 88.77630088812064
- type: max_ap
value: 81.61725457333809
- type: max_f1
value: 74.91373801916932
- task:
type: PairClassification
dataset:
type: mteb/twitterurlcorpus-pairclassification
name: MTEB TwitterURLCorpus
config: default
split: test
revision: 8b6510b0b1fa4e4c4f879467980e9be563ec1cdf
metrics:
- type: cos_sim_accuracy
value: 89.85136026700819
- type: cos_sim_ap
value: 87.74656687446567
- type: cos_sim_f1
value: 80.3221673073403
- type: cos_sim_precision
value: 76.56871640957633
- type: cos_sim_recall
value: 84.46258084385587
- type: dot_accuracy
value: 89.85136026700819
- type: dot_ap
value: 87.74656471395072
- type: dot_f1
value: 80.3221673073403
- type: dot_precision
value: 76.56871640957633
- type: dot_recall
value: 84.46258084385587
- type: euclidean_accuracy
value: 89.85136026700819
- type: euclidean_ap
value: 87.74656885754466
- type: euclidean_f1
value: 80.3221673073403
- type: euclidean_precision
value: 76.56871640957633
- type: euclidean_recall
value: 84.46258084385587
- type: manhattan_accuracy
value: 89.86300306593705
- type: manhattan_ap
value: 87.78807479093082
- type: manhattan_f1
value: 80.31663429471911
- type: manhattan_precision
value: 76.63472970137772
- type: manhattan_recall
value: 84.3701878657222
- type: max_accuracy
value: 89.86300306593705
- type: max_ap
value: 87.78807479093082
- type: max_f1
value: 80.3221673073403
- task:
type: Retrieval
dataset:
type: C-MTEB/VideoRetrieval
name: MTEB VideoRetrieval
config: default
split: dev
revision: None
metrics:
- type: map_at_1
value: 32.4
- type: map_at_10
value: 40.961999999999996
- type: map_at_100
value: 41.660000000000004
- type: map_at_1000
value: 41.721000000000004
- type: map_at_3
value: 38.550000000000004
- type: map_at_5
value: 40.06
- type: mrr_at_1
value: 32.4
- type: mrr_at_10
value: 40.961999999999996
- type: mrr_at_100
value: 41.660000000000004
- type: mrr_at_1000
value: 41.721000000000004
- type: mrr_at_3
value: 38.550000000000004
- type: mrr_at_5
value: 40.06
- type: ndcg_at_1
value: 32.4
- type: ndcg_at_10
value: 45.388
- type: ndcg_at_100
value: 49.012
- type: ndcg_at_1000
value: 50.659
- type: ndcg_at_3
value: 40.47
- type: ndcg_at_5
value: 43.232
- type: precision_at_1
value: 32.4
- type: precision_at_10
value: 5.94
- type: precision_at_100
value: 0.769
- type: precision_at_1000
value: 0.09
- type: precision_at_3
value: 15.333
- type: precision_at_5
value: 10.56
- type: recall_at_1
value: 32.4
- type: recall_at_10
value: 59.4
- type: recall_at_100
value: 76.9
- type: recall_at_1000
value: 90.0
- type: recall_at_3
value: 46.0
- type: recall_at_5
value: 52.800000000000004
- task:
type: Classification
dataset:
type: C-MTEB/waimai-classification
name: MTEB Waimai
config: default
split: test
revision: None
metrics:
- type: accuracy
value: 86.94000000000001
- type: ap
value: 70.57373468481975
- type: f1
value: 85.26264784928323
language:
- en
license: mit
---
## E5-mistral-7b-instruct
[Improving Text Embeddings with Large Language Models](https://arxiv.org/pdf/2401.00368.pdf). Liang Wang, Nan Yang, Xiaolong Huang, Linjun Yang, Rangan Majumder, Furu Wei, arXiv 2024
This model has 32 layers and the embedding size is 4096.
## Usage
Below is an example to encode queries and passages from the MS-MARCO passage ranking dataset.
### Sentence Transformers
```python
from sentence_transformers import SentenceTransformer
model = SentenceTransformer("intfloat/e5-mistral-7b-instruct")
# In case you want to reduce the maximum sequence length:
model.max_seq_length = 4096
queries = [
"how much protein should a female eat",
"summit define",
]
documents = [
"As a general guideline, the CDC's average requirement of protein for women ages 19 to 70 is 46 grams per day. But, as you can see from this chart, you'll need to increase that if you're expecting or training for a marathon. Check out the chart below to see how much protein you should be eating each day.",
"Definition of summit for English Language Learners. : 1 the highest point of a mountain : the top of a mountain. : 2 the highest level. : 3 a meeting or series of meetings between the leaders of two or more governments."
]
query_embeddings = model.encode(queries, prompt_name="web_search_query")
document_embeddings = model.encode(documents)
scores = (query_embeddings @ document_embeddings.T) * 100
print(scores.tolist())
```
Have a look at [config_sentence_transformers.json](config_sentence_transformers.json) for the prompts that are pre-configured, such as `web_search_query`, `sts_query`, and `summarization_query`. Additionally, check out [unilm/e5/utils.py](https://github.com/microsoft/unilm/blob/9c0f1ff7ca53431fe47d2637dfe253643d94185b/e5/utils.py#L106) for prompts we used for evaluation. You can use these via e.g. `model.encode(queries, prompt="Instruct: Given a claim, find documents that refute the claim\nQuery: ")`.
### Transformers
```python
import torch
import torch.nn.functional as F
from torch import Tensor
from transformers import AutoTokenizer, AutoModel
def last_token_pool(last_hidden_states: Tensor,
attention_mask: Tensor) -> Tensor:
left_padding = (attention_mask[:, -1].sum() == attention_mask.shape[0])
if left_padding:
return last_hidden_states[:, -1]
else:
sequence_lengths = attention_mask.sum(dim=1) - 1
batch_size = last_hidden_states.shape[0]
return last_hidden_states[torch.arange(batch_size, device=last_hidden_states.device), sequence_lengths]
def get_detailed_instruct(task_description: str, query: str) -> str:
return f'Instruct: {task_description}\nQuery: {query}'
# Each query must come with a one-sentence instruction that describes the task
task = 'Given a web search query, retrieve relevant passages that answer the query'
queries = [
get_detailed_instruct(task, 'how much protein should a female eat'),
get_detailed_instruct(task, 'summit define')
]
# No need to add instruction for retrieval documents
documents = [
"As a general guideline, the CDC's average requirement of protein for women ages 19 to 70 is 46 grams per day. But, as you can see from this chart, you'll need to increase that if you're expecting or training for a marathon. Check out the chart below to see how much protein you should be eating each day.",
"Definition of summit for English Language Learners. : 1 the highest point of a mountain : the top of a mountain. : 2 the highest level. : 3 a meeting or series of meetings between the leaders of two or more governments."
]
input_texts = queries + documents
tokenizer = AutoTokenizer.from_pretrained('intfloat/e5-mistral-7b-instruct')
model = AutoModel.from_pretrained('intfloat/e5-mistral-7b-instruct')
max_length = 4096
# Tokenize the input texts
batch_dict = tokenizer(input_texts, max_length=max_length, padding=True, truncation=True, return_tensors='pt')
outputs = model(**batch_dict)
embeddings = last_token_pool(outputs.last_hidden_state, batch_dict['attention_mask'])
# normalize embeddings
embeddings = F.normalize(embeddings, p=2, dim=1)
scores = (embeddings[:2] @ embeddings[2:].T) * 100
print(scores.tolist())
```
## Supported Languages
This model is initialized from [Mistral-7B-v0.1](https://huggingface.co/mistralai/Mistral-7B-v0.1)
and fine-tuned on a mixture of multilingual datasets.
As a result, it has some multilingual capability.
However, since Mistral-7B-v0.1 is mainly trained on English data, we recommend using this model for English only.
For multilingual use cases, please refer to [multilingual-e5-large](https://huggingface.co/intfloat/multilingual-e5-large).
## MTEB Benchmark Evaluation
Check out [unilm/e5](https://github.com/microsoft/unilm/tree/master/e5) to reproduce evaluation results
on the [BEIR](https://arxiv.org/abs/2104.08663) and [MTEB benchmark](https://arxiv.org/abs/2210.07316).
## FAQ
**1. Do I need to add instructions to the query?**
Yes, this is how the model is trained, otherwise you will see a performance degradation.
The task definition should be a one-sentence instruction that describes the task.
This is a way to customize text embeddings for different scenarios through natural language instructions.
Please check out [unilm/e5/utils.py](https://github.com/microsoft/unilm/blob/9c0f1ff7ca53431fe47d2637dfe253643d94185b/e5/utils.py#L106) for instructions we used for evaluation.
On the other hand, there is no need to add instructions to the document side.
**2. Why are my reproduced results slightly different from reported in the model card?**
Different versions of `transformers` and `pytorch` could cause negligible but non-zero performance differences.
**3. Where are the LoRA-only weights?**
You can find the LoRA-only weights at [https://huggingface.co/intfloat/e5-mistral-7b-instruct/tree/main/lora](https://huggingface.co/intfloat/e5-mistral-7b-instruct/tree/main/lora).
## Citation
If you find our paper or models helpful, please consider cite as follows:
```bibtex
@article{wang2023improving,
title={Improving Text Embeddings with Large Language Models},
author={Wang, Liang and Yang, Nan and Huang, Xiaolong and Yang, Linjun and Majumder, Rangan and Wei, Furu},
journal={arXiv preprint arXiv:2401.00368},
year={2023}
}
@article{wang2022text,
title={Text Embeddings by Weakly-Supervised Contrastive Pre-training},
author={Wang, Liang and Yang, Nan and Huang, Xiaolong and Jiao, Binxing and Yang, Linjun and Jiang, Daxin and Majumder, Rangan and Wei, Furu},
journal={arXiv preprint arXiv:2212.03533},
year={2022}
}
```
## Limitations
Using this model for inputs longer than 4096 tokens is not recommended.
This model's multilingual capability is still inferior to [multilingual-e5-large](https://huggingface.co/intfloat/multilingual-e5-large) for some cases.
|
LanguageBind/LanguageBind_Video_FT | LanguageBind | "2024-02-01T06:57:50Z" | 112,075 | 3 | transformers | [
"transformers",
"pytorch",
"LanguageBindVideo",
"zero-shot-image-classification",
"arxiv:2310.01852",
"license:mit",
"endpoints_compatible",
"region:us"
] | zero-shot-image-classification | "2023-11-26T07:37:18Z" | ---
license: mit
---
<p align="center">
<img src="https://s11.ax1x.com/2024/02/01/pFMDAm9.png" width="250" style="margin-bottom: 0.2;"/>
<p>
<h2 align="center"> <a href="https://arxiv.org/pdf/2310.01852.pdf">【ICLR 2024 🔥】LanguageBind: Extending Video-Language Pretraining to N-modality by Language-based Semantic Alignment</a></h2>
<h5 align="center"> If you like our project, please give us a star ⭐ on GitHub for latest update. </h2>
## 📰 News
* **[2024.01.27]** 👀👀👀 Our [MoE-LLaVA](https://github.com/PKU-YuanGroup/MoE-LLaVA) is released! A sparse model with 3B parameters outperformed the dense model with 7B parameters.
* **[2024.01.16]** 🔥🔥🔥 Our LanguageBind has been accepted at ICLR 2024! We earn the score of 6(3)8(6)6(6)6(6) [here](https://openreview.net/forum?id=QmZKc7UZCy¬eId=OgsxQxAleA).
* **[2023.12.15]** 💪💪💪 We expand the 💥💥💥 VIDAL dataset and now have **10M video-text data**. We launch **LanguageBind_Video 1.5**, checking our [model zoo](#-model-zoo).
* **[2023.12.10]** We expand the 💥💥💥 VIDAL dataset and now have **10M depth and 10M thermal data**. We are in the process of uploading thermal and depth data on [Hugging Face](https://huggingface.co/datasets/LanguageBind/VIDAL-Depth-Thermal) and expect the whole process to last 1-2 months.
* **[2023.11.27]** 🔥🔥🔥 We have updated our [paper](https://arxiv.org/abs/2310.01852) with emergency zero-shot results., checking our ✨ [results](#emergency-results).
* **[2023.11.26]** 💥💥💥 We have open-sourced all textual sources and corresponding YouTube IDs [here](DATASETS.md).
* **[2023.11.26]** 📣📣📣 We have open-sourced fully fine-tuned **Video & Audio**, achieving improved performance once again, checking our [model zoo](#-model-zoo).
* **[2023.11.22]** We are about to release a fully fine-tuned version, and the **HUGE** version is currently undergoing training.
* **[2023.11.21]** 💥 We are releasing sample data in [DATASETS.md](DATASETS.md) so that individuals who are interested can further modify the code to train it on their own data.
* **[2023.11.20]** 🚀🚀🚀 [Video-LLaVA](https://github.com/PKU-YuanGroup/Video-LLaVA) builds a large visual-language model to achieve 🎉SOTA performances based on LanguageBind encoders.
* **[2023.10.23]** 🎶 LanguageBind-Audio achieves 🎉🎉🎉**state-of-the-art (SOTA) performance on 5 datasets**, checking our ✨ [results](#multiple-modalities)!
* **[2023.10.14]** 😱 Released a stronger LanguageBind-Video, checking our ✨ [results](#video-language)! The video checkpoint **have updated** on Huggingface Model Hub!
* **[2023.10.10]** We provide sample data, which can be found in [assets](assets), and [emergency zero-shot usage](#emergency-zero-shot) is described.
* **[2023.10.07]** The checkpoints are available on 🤗 [Huggingface Model](https://huggingface.co/LanguageBind).
* **[2023.10.04]** Code and [demo](https://huggingface.co/spaces/LanguageBind/LanguageBind) are available now! Welcome to **watch** 👀 this repository for the latest updates.
## 😮 Highlights
### 💡 High performance, but NO intermediate modality required
LanguageBind is a **language-centric** multimodal pretraining approach, **taking the language as the bind across different modalities** because the language modality is well-explored and contains rich semantics.
* The following first figure shows the architecture of LanguageBind. LanguageBind can be easily extended to segmentation, detection tasks, and potentially to unlimited modalities.
### ⚡️ A multimodal, fully aligned and voluminous dataset
We propose **VIDAL-10M**, **10 Million data** with **V**ideo, **I**nfrared, **D**epth, **A**udio and their corresponding **L**anguage, which greatly expands the data beyond visual modalities.
* The second figure shows our proposed VIDAL-10M dataset, which includes five modalities: video, infrared, depth, audio, and language.
### 🔥 Multi-view enhanced description for training
We make multi-view enhancements to language. We produce multi-view description that combines **meta-data**, **spatial**, and **temporal** to greatly enhance the semantic information of the language. In addition we further **enhance the language with ChatGPT** to create a good semantic space for each modality aligned language.
## 🤗 Demo
* **Local demo.** Highly recommend trying out our web demo, which incorporates all features currently supported by LanguageBind.
```bash
python gradio_app.py
```
* **Online demo.** We provide the [online demo](https://huggingface.co/spaces/LanguageBind/LanguageBind) in Huggingface Spaces. In this demo, you can calculate the similarity of modalities to language, such as audio-to-language, video-to-language, and depth-to-image.
## 🛠️ Requirements and Installation
* Python >= 3.8
* Pytorch >= 1.13.1
* CUDA Version >= 11.6
* Install required packages:
```bash
git clone https://github.com/PKU-YuanGroup/LanguageBind
cd LanguageBind
pip install torch==1.13.1+cu116 torchvision==0.14.1+cu116 torchaudio==0.13.1 --extra-index-url https://download.pytorch.org/whl/cu116
pip install -r requirements.txt
```
## 🐳 Model Zoo
The names in the table represent different encoder models. For example, `LanguageBind/LanguageBind_Video_FT` represents the fully fine-tuned version, while `LanguageBind/LanguageBind_Video` represents the LoRA-tuned version.
You can freely replace them in the recommended [API usage](#-api). We recommend using the fully fine-tuned version, as it offers stronger performance.
<div align="center">
<table border="1" width="100%">
<tr align="center">
<th>Modality</th><th>LoRA tuning</th><th>Fine-tuning</th>
</tr>
<tr align="center">
<td>Video</td><td><a href="https://huggingface.co/LanguageBind/LanguageBind_Video">LanguageBind_Video</a></td><td><a href="https://huggingface.co/LanguageBind/LanguageBind_Video_FT">LanguageBind_Video_FT</a></td>
</tr>
<tr align="center">
<td>Audio</td><td><a href="https://huggingface.co/LanguageBind/LanguageBind_Audio">LanguageBind_Audio</a></td><td><a href="https://huggingface.co/LanguageBind/LanguageBind_Audio_FT">LanguageBind_Audio_FT</a></td>
</tr>
<tr align="center">
<td>Depth</td><td><a href="https://huggingface.co/LanguageBind/LanguageBind_Depth">LanguageBind_Depth</a></td><td>-</td>
</tr>
<tr align="center">
<td>Thermal</td><td><a href="https://huggingface.co/LanguageBind/LanguageBind_Thermal">LanguageBind_Thermal</a></td><td>-</td>
</tr>
</table>
</div>
<div align="center">
<table border="1" width="100%">
<tr align="center">
<th>Version</th><th>Tuning</th><th>Model size</th><th>Num_frames</th><th>HF Link</th><th>MSR-VTT</th><th>DiDeMo</th><th>ActivityNet</th><th>MSVD</th>
</tr>
<tr align="center">
<td>LanguageBind_Video</td><td>LoRA</td><td>Large</td><td>8</td><td><a href="https://huggingface.co/LanguageBind/LanguageBind_Video">Link</a></td><td>42.6</td><td>37.8</td><td>35.1</td><td>52.2</td>
</tr>
<tr align="center">
<td>LanguageBind_Video_FT</td><td>Full-tuning</td><td>Large</td><td>8</td><td><a href="https://huggingface.co/LanguageBind/LanguageBind_Video_FT">Link</a></td><td>42.7</td><td>38.1</td><td>36.9</td><td>53.5</td>
</tr>
<tr align="center">
<td>LanguageBind_Video_V1.5_FT</td><td>Full-tuning</td><td>Large</td><td>8</td><td><a href="https://huggingface.co/LanguageBind/LanguageBind_Video_V1.5_FT">Link</a></td><td>42.8</td><td>39.7</td><td>38.4</td><td>54.1</td>
</tr>
<tr align="center">
<td>LanguageBind_Video_V1.5_FT</td><td>Full-tuning</td><td>Large</td><td>12</td><td>Coming soon</td>
</tr>
<tr align="center">
<td>LanguageBind_Video_Huge_V1.5_FT</td><td>Full-tuning</td><td>Huge</td><td>8</td><td><a href="https://huggingface.co/LanguageBind/LanguageBind_Video_Huge_V1.5_FT">Link</a></td><td>44.8</td><td>39.9</td><td>41.0</td><td>53.7</td>
</tr>
<tr align="center">
<td>LanguageBind_Video_Huge_V1.5_FT</td><td>Full-tuning</td><td>Huge</td><td>12</td><td>Coming soon</td>
</tr>
</table>
</div>
## 🤖 API
**We open source all modalities preprocessing code.** If you want to load the model (e.g. ```LanguageBind/LanguageBind_Thermal```) from the model hub on Huggingface or on local, you can use the following code snippets!
### Inference for Multi-modal Binding
We have provided some sample datasets in [assets](assets) to quickly see how languagebind works.
```python
import torch
from languagebind import LanguageBind, to_device, transform_dict, LanguageBindImageTokenizer
if __name__ == '__main__':
device = 'cuda:0'
device = torch.device(device)
clip_type = {
'video': 'LanguageBind_Video_FT', # also LanguageBind_Video
'audio': 'LanguageBind_Audio_FT', # also LanguageBind_Audio
'thermal': 'LanguageBind_Thermal',
'image': 'LanguageBind_Image',
'depth': 'LanguageBind_Depth',
}
model = LanguageBind(clip_type=clip_type, cache_dir='./cache_dir')
model = model.to(device)
model.eval()
pretrained_ckpt = f'lb203/LanguageBind_Image'
tokenizer = LanguageBindImageTokenizer.from_pretrained(pretrained_ckpt, cache_dir='./cache_dir/tokenizer_cache_dir')
modality_transform = {c: transform_dict[c](model.modality_config[c]) for c in clip_type.keys()}
image = ['assets/image/0.jpg', 'assets/image/1.jpg']
audio = ['assets/audio/0.wav', 'assets/audio/1.wav']
video = ['assets/video/0.mp4', 'assets/video/1.mp4']
depth = ['assets/depth/0.png', 'assets/depth/1.png']
thermal = ['assets/thermal/0.jpg', 'assets/thermal/1.jpg']
language = ["Training a parakeet to climb up a ladder.", 'A lion climbing a tree to catch a monkey.']
inputs = {
'image': to_device(modality_transform['image'](image), device),
'video': to_device(modality_transform['video'](video), device),
'audio': to_device(modality_transform['audio'](audio), device),
'depth': to_device(modality_transform['depth'](depth), device),
'thermal': to_device(modality_transform['thermal'](thermal), device),
}
inputs['language'] = to_device(tokenizer(language, max_length=77, padding='max_length',
truncation=True, return_tensors='pt'), device)
with torch.no_grad():
embeddings = model(inputs)
print("Video x Text: \n",
torch.softmax(embeddings['video'] @ embeddings['language'].T, dim=-1).detach().cpu().numpy())
print("Image x Text: \n",
torch.softmax(embeddings['image'] @ embeddings['language'].T, dim=-1).detach().cpu().numpy())
print("Depth x Text: \n",
torch.softmax(embeddings['depth'] @ embeddings['language'].T, dim=-1).detach().cpu().numpy())
print("Audio x Text: \n",
torch.softmax(embeddings['audio'] @ embeddings['language'].T, dim=-1).detach().cpu().numpy())
print("Thermal x Text: \n",
torch.softmax(embeddings['thermal'] @ embeddings['language'].T, dim=-1).detach().cpu().numpy())
```
Then returns the following result.
```bash
Video x Text:
[[9.9989331e-01 1.0667283e-04]
[1.3255903e-03 9.9867439e-01]]
Image x Text:
[[9.9990666e-01 9.3292067e-05]
[4.6132666e-08 1.0000000e+00]]
Depth x Text:
[[0.9954276 0.00457235]
[0.12042473 0.8795753 ]]
Audio x Text:
[[0.97634876 0.02365119]
[0.02917843 0.97082156]]
Thermal x Text:
[[0.9482511 0.0517489 ]
[0.48746133 0.5125386 ]]
```
### Emergency zero-shot
Since languagebind binds each modality together, we also found the **emergency zero-shot**. It's very simple to use.
```python
print("Video x Audio: \n", torch.softmax(embeddings['video'] @ embeddings['audio'].T, dim=-1).detach().cpu().numpy())
print("Image x Depth: \n", torch.softmax(embeddings['image'] @ embeddings['depth'].T, dim=-1).detach().cpu().numpy())
print("Image x Thermal: \n", torch.softmax(embeddings['image'] @ embeddings['thermal'].T, dim=-1).detach().cpu().numpy())
```
Then, you will get:
```
Video x Audio:
[[1.0000000e+00 0.0000000e+00]
[3.1150486e-32 1.0000000e+00]]
Image x Depth:
[[1. 0.]
[0. 1.]]
Image x Thermal:
[[1. 0.]
[0. 1.]]
```
### Different branches for X-Language task
Additionally, LanguageBind can be **disassembled into different branches** to handle different tasks. Note that we do not train Image, which just initialize from OpenCLIP.
#### Thermal
```python
import torch
from languagebind import LanguageBindThermal, LanguageBindThermalTokenizer, LanguageBindThermalProcessor
pretrained_ckpt = 'LanguageBind/LanguageBind_Thermal'
model = LanguageBindThermal.from_pretrained(pretrained_ckpt, cache_dir='./cache_dir')
tokenizer = LanguageBindThermalTokenizer.from_pretrained(pretrained_ckpt, cache_dir='./cache_dir')
thermal_process = LanguageBindThermalProcessor(model.config, tokenizer)
model.eval()
data = thermal_process([r"your/thermal.jpg"], ['your text'], return_tensors='pt')
with torch.no_grad():
out = model(**data)
print(out.text_embeds @ out.image_embeds.T)
```
#### Depth
```python
import torch
from languagebind import LanguageBindDepth, LanguageBindDepthTokenizer, LanguageBindDepthProcessor
pretrained_ckpt = 'LanguageBind/LanguageBind_Depth'
model = LanguageBindDepth.from_pretrained(pretrained_ckpt, cache_dir='./cache_dir')
tokenizer = LanguageBindDepthTokenizer.from_pretrained(pretrained_ckpt, cache_dir='./cache_dir')
depth_process = LanguageBindDepthProcessor(model.config, tokenizer)
model.eval()
data = depth_process([r"your/depth.png"], ['your text.'], return_tensors='pt')
with torch.no_grad():
out = model(**data)
print(out.text_embeds @ out.image_embeds.T)
```
#### Video
```python
import torch
from languagebind import LanguageBindVideo, LanguageBindVideoTokenizer, LanguageBindVideoProcessor
pretrained_ckpt = 'LanguageBind/LanguageBind_Video_FT' # also 'LanguageBind/LanguageBind_Video'
model = LanguageBindVideo.from_pretrained(pretrained_ckpt, cache_dir='./cache_dir')
tokenizer = LanguageBindVideoTokenizer.from_pretrained(pretrained_ckpt, cache_dir='./cache_dir')
video_process = LanguageBindVideoProcessor(model.config, tokenizer)
model.eval()
data = video_process(["your/video.mp4"], ['your text.'], return_tensors='pt')
with torch.no_grad():
out = model(**data)
print(out.text_embeds @ out.image_embeds.T)
```
#### Audio
```python
import torch
from languagebind import LanguageBindAudio, LanguageBindAudioTokenizer, LanguageBindAudioProcessor
pretrained_ckpt = 'LanguageBind/LanguageBind_Audio_FT' # also 'LanguageBind/LanguageBind_Audio'
model = LanguageBindAudio.from_pretrained(pretrained_ckpt, cache_dir='./cache_dir')
tokenizer = LanguageBindAudioTokenizer.from_pretrained(pretrained_ckpt, cache_dir='./cache_dir')
audio_process = LanguageBindAudioProcessor(model.config, tokenizer)
model.eval()
data = audio_process([r"your/audio.wav"], ['your audio.'], return_tensors='pt')
with torch.no_grad():
out = model(**data)
print(out.text_embeds @ out.image_embeds.T)
```
#### Image
Note that our image encoder is the same as OpenCLIP. **Not** as fine-tuned as other modalities.
```python
import torch
from languagebind import LanguageBindImage, LanguageBindImageTokenizer, LanguageBindImageProcessor
pretrained_ckpt = 'LanguageBind/LanguageBind_Image'
model = LanguageBindImage.from_pretrained(pretrained_ckpt, cache_dir='./cache_dir')
tokenizer = LanguageBindImageTokenizer.from_pretrained(pretrained_ckpt, cache_dir='./cache_dir')
image_process = LanguageBindImageProcessor(model.config, tokenizer)
model.eval()
data = image_process([r"your/image.jpg"], ['your text.'], return_tensors='pt')
with torch.no_grad():
out = model(**data)
print(out.text_embeds @ out.image_embeds.T)
```
## 💥 VIDAL-10M
The datasets is in [DATASETS.md](DATASETS.md).
## 🗝️ Training & Validating
The training & validating instruction is in [TRAIN_AND_VALIDATE.md](TRAIN_AND_VALIDATE.md).
## 👍 Acknowledgement
* [OpenCLIP](https://github.com/mlfoundations/open_clip) An open source pretraining framework.
* [CLIP4Clip](https://github.com/ArrowLuo/CLIP4Clip) An open source Video-Text retrieval framework.
* [sRGB-TIR](https://github.com/rpmsnu/sRGB-TIR) An open source framework to generate infrared (thermal) images.
* [GLPN](https://github.com/vinvino02/GLPDepth) An open source framework to generate depth images.
## 🔒 License
* The majority of this project is released under the MIT license as found in the [LICENSE](https://github.com/PKU-YuanGroup/LanguageBind/blob/main/LICENSE) file.
* The dataset of this project is released under the CC-BY-NC 4.0 license as found in the [DATASET_LICENSE](https://github.com/PKU-YuanGroup/LanguageBind/blob/main/DATASET_LICENSE) file.
## ✏️ Citation
If you find our paper and code useful in your research, please consider giving a star :star: and citation :pencil:.
```BibTeX
@misc{zhu2023languagebind,
title={LanguageBind: Extending Video-Language Pretraining to N-modality by Language-based Semantic Alignment},
author={Bin Zhu and Bin Lin and Munan Ning and Yang Yan and Jiaxi Cui and Wang HongFa and Yatian Pang and Wenhao Jiang and Junwu Zhang and Zongwei Li and Cai Wan Zhang and Zhifeng Li and Wei Liu and Li Yuan},
year={2023},
eprint={2310.01852},
archivePrefix={arXiv},
primaryClass={cs.CV}
}
```
## ✨ Star History
[](https://star-history.com/#PKU-YuanGroup/LanguageBind&Date)
## 🤝 Contributors
<a href="https://github.com/PKU-YuanGroup/LanguageBind/graphs/contributors">
<img src="https://contrib.rocks/image?repo=PKU-YuanGroup/LanguageBind" />
</a>
|
saattrupdan/wav2vec2-xls-r-300m-ftspeech | saattrupdan | "2023-09-11T13:27:55Z" | 111,638 | 0 | transformers | [
"transformers",
"pytorch",
"safetensors",
"wav2vec2",
"automatic-speech-recognition",
"da",
"dataset:ftspeech",
"base_model:facebook/wav2vec2-xls-r-300m",
"license:other",
"model-index",
"endpoints_compatible",
"region:us"
] | automatic-speech-recognition | "2022-03-04T14:53:05Z" | ---
language:
- da
license: other
datasets:
- ftspeech
metrics:
- wer
tasks:
- automatic-speech-recognition
base_model: facebook/wav2vec2-xls-r-300m
model-index:
- name: wav2vec2-xls-r-300m-ftspeech
results:
- task:
type: automatic-speech-recognition
dataset:
name: Danish Common Voice 8.0
type: mozilla-foundation/common_voice_8_0
args: da
metrics:
- type: wer
value: 17.91
- task:
type: automatic-speech-recognition
dataset:
name: Alvenir ASR test dataset
type: Alvenir/alvenir_asr_da_eval
metrics:
- type: wer
value: 13.84
---
# XLS-R-300m-FTSpeech
## Model description
This model is a fine-tuned version of [facebook/wav2vec2-xls-r-300m](https://huggingface.co/facebook/wav2vec2-xls-r-300m) on the [FTSpeech dataset](https://ftspeech.github.io/), being a dataset of 1,800 hours of transcribed speeches from the Danish parliament.
## Performance
The model achieves the following WER scores (lower is better):
| **Dataset** | **WER without LM** | **WER with 5-gram LM** |
| :---: | ---: | ---: |
| [Danish part of Common Voice 8.0](https://huggingface.co/datasets/mozilla-foundation/common_voice_8_0/viewer/da/train) | 20.48 | 17.91 |
| [Alvenir test set](https://huggingface.co/datasets/Alvenir/alvenir_asr_da_eval) | 15.46 | 13.84 |
## License
The use of this model needs to adhere to [this license from the Danish Parliament](https://www.ft.dk/da/aktuelt/tv-fra-folketinget/deling-og-rettigheder). |
mradermacher/New-Dawn-Llama-3-70B-32K-v1.0-GGUF | mradermacher | "2024-06-23T11:44:31Z" | 111,419 | 0 | transformers | [
"transformers",
"gguf",
"mergekit",
"merge",
"Not-for-all-Audiences",
"en",
"base_model:sophosympatheia/New-Dawn-Llama-3-70B-32K-v1.0",
"license:llama3",
"endpoints_compatible",
"region:us"
] | null | "2024-06-23T01:22:40Z" | ---
base_model: sophosympatheia/New-Dawn-Llama-3-70B-32K-v1.0
language:
- en
library_name: transformers
license: llama3
quantized_by: mradermacher
tags:
- mergekit
- merge
- Not-for-all-Audiences
---
## About
<!-- ### quantize_version: 2 -->
<!-- ### output_tensor_quantised: 1 -->
<!-- ### convert_type: hf -->
<!-- ### vocab_type: -->
<!-- ### tags: -->
static quants of https://huggingface.co/sophosympatheia/New-Dawn-Llama-3-70B-32K-v1.0
<!-- provided-files -->
weighted/imatrix quants are available at https://huggingface.co/mradermacher/New-Dawn-Llama-3-70B-32K-v1.0-i1-GGUF
## Usage
If you are unsure how to use GGUF files, refer to one of [TheBloke's
READMEs](https://huggingface.co/TheBloke/KafkaLM-70B-German-V0.1-GGUF) for
more details, including on how to concatenate multi-part files.
## Provided Quants
(sorted by size, not necessarily quality. IQ-quants are often preferable over similar sized non-IQ quants)
| Link | Type | Size/GB | Notes |
|:-----|:-----|--------:|:------|
| [GGUF](https://huggingface.co/mradermacher/New-Dawn-Llama-3-70B-32K-v1.0-GGUF/resolve/main/New-Dawn-Llama-3-70B-32K-v1.0.Q2_K.gguf) | Q2_K | 26.5 | |
| [GGUF](https://huggingface.co/mradermacher/New-Dawn-Llama-3-70B-32K-v1.0-GGUF/resolve/main/New-Dawn-Llama-3-70B-32K-v1.0.IQ3_XS.gguf) | IQ3_XS | 29.4 | |
| [GGUF](https://huggingface.co/mradermacher/New-Dawn-Llama-3-70B-32K-v1.0-GGUF/resolve/main/New-Dawn-Llama-3-70B-32K-v1.0.IQ3_S.gguf) | IQ3_S | 31.0 | beats Q3_K* |
| [GGUF](https://huggingface.co/mradermacher/New-Dawn-Llama-3-70B-32K-v1.0-GGUF/resolve/main/New-Dawn-Llama-3-70B-32K-v1.0.Q3_K_S.gguf) | Q3_K_S | 31.0 | |
| [GGUF](https://huggingface.co/mradermacher/New-Dawn-Llama-3-70B-32K-v1.0-GGUF/resolve/main/New-Dawn-Llama-3-70B-32K-v1.0.IQ3_M.gguf) | IQ3_M | 32.0 | |
| [GGUF](https://huggingface.co/mradermacher/New-Dawn-Llama-3-70B-32K-v1.0-GGUF/resolve/main/New-Dawn-Llama-3-70B-32K-v1.0.Q3_K_M.gguf) | Q3_K_M | 34.4 | lower quality |
| [GGUF](https://huggingface.co/mradermacher/New-Dawn-Llama-3-70B-32K-v1.0-GGUF/resolve/main/New-Dawn-Llama-3-70B-32K-v1.0.Q3_K_L.gguf) | Q3_K_L | 37.2 | |
| [GGUF](https://huggingface.co/mradermacher/New-Dawn-Llama-3-70B-32K-v1.0-GGUF/resolve/main/New-Dawn-Llama-3-70B-32K-v1.0.IQ4_XS.gguf) | IQ4_XS | 38.4 | |
| [GGUF](https://huggingface.co/mradermacher/New-Dawn-Llama-3-70B-32K-v1.0-GGUF/resolve/main/New-Dawn-Llama-3-70B-32K-v1.0.Q4_K_S.gguf) | Q4_K_S | 40.4 | fast, recommended |
| [GGUF](https://huggingface.co/mradermacher/New-Dawn-Llama-3-70B-32K-v1.0-GGUF/resolve/main/New-Dawn-Llama-3-70B-32K-v1.0.Q4_K_M.gguf) | Q4_K_M | 42.6 | fast, recommended |
| [GGUF](https://huggingface.co/mradermacher/New-Dawn-Llama-3-70B-32K-v1.0-GGUF/resolve/main/New-Dawn-Llama-3-70B-32K-v1.0.Q5_K_S.gguf) | Q5_K_S | 48.8 | |
| [GGUF](https://huggingface.co/mradermacher/New-Dawn-Llama-3-70B-32K-v1.0-GGUF/resolve/main/New-Dawn-Llama-3-70B-32K-v1.0.Q5_K_M.gguf) | Q5_K_M | 50.0 | |
| [PART 1](https://huggingface.co/mradermacher/New-Dawn-Llama-3-70B-32K-v1.0-GGUF/resolve/main/New-Dawn-Llama-3-70B-32K-v1.0.Q6_K.gguf.part1of2) [PART 2](https://huggingface.co/mradermacher/New-Dawn-Llama-3-70B-32K-v1.0-GGUF/resolve/main/New-Dawn-Llama-3-70B-32K-v1.0.Q6_K.gguf.part2of2) | Q6_K | 58.0 | very good quality |
| [PART 1](https://huggingface.co/mradermacher/New-Dawn-Llama-3-70B-32K-v1.0-GGUF/resolve/main/New-Dawn-Llama-3-70B-32K-v1.0.Q8_0.gguf.part1of2) [PART 2](https://huggingface.co/mradermacher/New-Dawn-Llama-3-70B-32K-v1.0-GGUF/resolve/main/New-Dawn-Llama-3-70B-32K-v1.0.Q8_0.gguf.part2of2) | Q8_0 | 75.1 | fast, best quality |
Here is a handy graph by ikawrakow comparing some lower-quality quant
types (lower is better):

And here are Artefact2's thoughts on the matter:
https://gist.github.com/Artefact2/b5f810600771265fc1e39442288e8ec9
## FAQ / Model Request
See https://huggingface.co/mradermacher/model_requests for some answers to
questions you might have and/or if you want some other model quantized.
## Thanks
I thank my company, [nethype GmbH](https://www.nethype.de/), for letting
me use its servers and providing upgrades to my workstation to enable
this work in my free time.
<!-- end -->
|
maidalun1020/bce-embedding-base_v1 | maidalun1020 | "2024-04-16T06:57:59Z" | 111,272 | 275 | sentence-transformers | [
"sentence-transformers",
"pytorch",
"xlm-roberta",
"feature-extraction",
"sentence-similarity",
"transformers",
"en",
"zh",
"license:apache-2.0",
"endpoints_compatible",
"region:us"
] | feature-extraction | "2023-12-29T07:38:08Z" | ---
license: apache-2.0
pipeline_tag: feature-extraction
tags:
- sentence-transformers
- feature-extraction
- sentence-similarity
- transformers
language:
- en
- zh
---
<!--
* @Description:
* @Author: shenlei
* @Date: 2023-12-19 10:31:41
* @LastEditTime: 2024-01-09 23:52:00
* @LastEditors: shenlei
-->
<h1 align="center">BCEmbedding: Bilingual and Crosslingual Embedding for RAG</h1>
<p align="center">
<a href="https://github.com/netease-youdao/BCEmbedding/blob/master/LICENSE">
<img src="https://img.shields.io/badge/license-Apache--2.0-yellow">
</a>
<a href="https://twitter.com/YDopensource">
<img src="https://img.shields.io/badge/follow-%40YDOpenSource-1DA1F2?logo=twitter&style={style}">
</a>
</p>
最新、最详细的bce-embedding-base_v1相关信息,请移步(The latest "Updates" should be checked in):
<p align="left">
<a href="https://github.com/netease-youdao/BCEmbedding">GitHub</a>
</p>
## 主要特点(Key Features):
- 中英双语,以及中英跨语种能力(Bilingual and Crosslingual capability in English and Chinese);
- RAG优化,适配更多真实业务场景(RAG adaptation for more domains, including Education, Law, Finance, Medical, Literature, FAQ, Textbook, Wikipedia, etc.);
- 方便集成进langchain和llamaindex(Easy integrations for langchain and llamaindex in <a href="https://github.com/netease-youdao/BCEmbedding">BCEmbedding</a>)。
- `EmbeddingModel`不需要“精心设计”instruction,尽可能召回有用片段。 (No need for "instruction")
- **最佳实践(Best practice)** :embedding召回top50-100片段,reranker对这50-100片段精排,最后取top5-10片段。(1. Get top 50-100 passages with [bce-embedding-base_v1](https://huggingface.co/maidalun1020/bce-embedding-base_v1) for "`recall`"; 2. Rerank passages with [bce-reranker-base_v1](https://huggingface.co/maidalun1020/bce-reranker-base_v1) and get top 5-10 for "`precision`" finally. )
## News:
- `BCEmbedding`技术博客( **Technical Blog** ): [为RAG而生-BCEmbedding技术报告](https://zhuanlan.zhihu.com/p/681370855)
- Related link for **RerankerModel** : [bce-reranker-base_v1](https://huggingface.co/maidalun1020/bce-reranker-base_v1)
## Third-party Examples:
- RAG applications: [QAnything](https://github.com/netease-youdao/qanything), [HuixiangDou](https://github.com/InternLM/HuixiangDou), [ChatPDF](https://github.com/shibing624/ChatPDF).
- Efficient inference framework: [ChatLLM.cpp](https://github.com/foldl/chatllm.cpp), [Xinference](https://github.com/xorbitsai/inference), [mindnlp (Huawei GPU, 华为GPU)](https://github.com/mindspore-lab/mindnlp/tree/master/llm/inference/bce).


-----------------------------------------
<details open="open">
<summary>Click to Open Contents</summary>
- <a href="#-bilingual-and-crosslingual-superiority" target="_Self">🌐 Bilingual and Crosslingual Superiority</a>
- <a href="#-key-features" target="_Self">💡 Key Features</a>
- <a href="#-latest-updates" target="_Self">🚀 Latest Updates</a>
- <a href="#-model-list" target="_Self">🍎 Model List</a>
- <a href="#-manual" target="_Self">📖 Manual</a>
- <a href="#installation" target="_Self">Installation</a>
- <a href="#quick-start" target="_Self">Quick Start (`transformers`, `sentence-transformers`)</a>
- <a href="#integrations-for-rag-frameworks" target="_Self">Integrations for RAG Frameworks (`langchain`, `llama_index`)</a>
- <a href="#%EF%B8%8F-evaluation" target="_Self">⚙️ Evaluation</a>
- <a href="#evaluate-semantic-representation-by-mteb" target="_Self">Evaluate Semantic Representation by MTEB</a>
- <a href="#evaluate-rag-by-llamaindex" target="_Self">Evaluate RAG by LlamaIndex</a>
- <a href="#-leaderboard" target="_Self">📈 Leaderboard</a>
- <a href="#semantic-representation-evaluations-in-mteb" target="_Self">Semantic Representation Evaluations in MTEB</a>
- <a href="#rag-evaluations-in-llamaindex" target="_Self">RAG Evaluations in LlamaIndex</a>
- <a href="#-youdaos-bcembedding-api" target="_Self">🛠 Youdao's BCEmbedding API</a>
- <a href="#-wechat-group" target="_Self">🧲 WeChat Group</a>
- <a href="#%EF%B8%8F-citation" target="_Self">✏️ Citation</a>
- <a href="#-license" target="_Self">🔐 License</a>
- <a href="#-related-links" target="_Self">🔗 Related Links</a>
</details>
<br>
**B**ilingual and **C**rosslingual **Embedding** (`BCEmbedding`), developed by NetEase Youdao, encompasses `EmbeddingModel` and `RerankerModel`. The `EmbeddingModel` specializes in generating semantic vectors, playing a crucial role in semantic search and question-answering, and the `RerankerModel` excels at refining search results and ranking tasks.
`BCEmbedding` serves as the cornerstone of Youdao's Retrieval Augmented Generation (RAG) implmentation, notably [QAnything](http://qanything.ai) [[github](https://github.com/netease-youdao/qanything)], an open-source implementation widely integrated in various Youdao products like [Youdao Speed Reading](https://read.youdao.com/#/home) and [Youdao Translation](https://fanyi.youdao.com/download-Mac?keyfrom=fanyiweb_navigation).
Distinguished for its bilingual and crosslingual proficiency, `BCEmbedding` excels in bridging Chinese and English linguistic gaps, which achieves
- **A high performence on <a href="#semantic-representation-evaluations-in-mteb">Semantic Representation Evaluations in MTEB</a>**;
- **A new benchmark in the realm of <a href="#rag-evaluations-in-llamaindex">RAG Evaluations in LlamaIndex</a>**.
`BCEmbedding`是由网易有道开发的双语和跨语种语义表征算法模型库,其中包含`EmbeddingModel`和`RerankerModel`两类基础模型。`EmbeddingModel`专门用于生成语义向量,在语义搜索和问答中起着关键作用,而`RerankerModel`擅长优化语义搜索结果和语义相关顺序精排。
`BCEmbedding`作为有道的检索增强生成式应用(RAG)的基石,特别是在[QAnything](http://qanything.ai) [[github](https://github.com/netease-youdao/qanything)]中发挥着重要作用。QAnything作为一个网易有道开源项目,在有道许多产品中有很好的应用实践,比如[有道速读](https://read.youdao.com/#/home)和[有道翻译](https://fanyi.youdao.com/download-Mac?keyfrom=fanyiweb_navigation)
`BCEmbedding`以其出色的双语和跨语种能力而著称,在语义检索中消除中英语言之间的差异,从而实现:
- **强大的双语和跨语种语义表征能力【<a href="#semantic-representation-evaluations-in-mteb">基于MTEB的语义表征评测指标</a>】。**
- **基于LlamaIndex的RAG评测,表现SOTA【<a href="#rag-evaluations-in-llamaindex">基于LlamaIndex的RAG评测指标</a>】。**
## 🌐 Bilingual and Crosslingual Superiority
Existing embedding models often encounter performance challenges in bilingual and crosslingual scenarios, particularly in Chinese, English and their crosslingual tasks. `BCEmbedding`, leveraging the strength of Youdao's translation engine, excels in delivering superior performance across monolingual, bilingual, and crosslingual settings.
`EmbeddingModel` supports ***Chinese (ch) and English (en)*** (more languages support will come soon), while `RerankerModel` supports ***Chinese (ch), English (en), Japanese (ja) and Korean (ko)***.
现有的单个语义表征模型在双语和跨语种场景中常常表现不佳,特别是在中文、英文及其跨语种任务中。`BCEmbedding`充分利用有道翻译引擎的优势,实现只需一个模型就可以在单语、双语和跨语种场景中表现出卓越的性能。
`EmbeddingModel`支持***中文和英文***(之后会支持更多语种);`RerankerModel`支持***中文,英文,日文和韩文***。
## 💡 Key Features
- **Bilingual and Crosslingual Proficiency**: Powered by Youdao's translation engine, excelling in Chinese, English and their crosslingual retrieval task, with upcoming support for additional languages.
- **RAG-Optimized**: Tailored for diverse RAG tasks including **translation, summarization, and question answering**, ensuring accurate **query understanding**. See <a href=#rag-evaluations-in-llamaindex>RAG Evaluations in LlamaIndex</a>.
- **Efficient and Precise Retrieval**: Dual-encoder for efficient retrieval of `EmbeddingModel` in first stage, and cross-encoder of `RerankerModel` for enhanced precision and deeper semantic analysis in second stage.
- **Broad Domain Adaptability**: Trained on diverse datasets for superior performance across various fields.
- **User-Friendly Design**: Instruction-free, versatile use for multiple tasks without specifying query instruction for each task.
- **Meaningful Reranking Scores**: `RerankerModel` provides relevant scores to improve result quality and optimize large language model performance.
- **Proven in Production**: Successfully implemented and validated in Youdao's products.
- **双语和跨语种能力**:基于有道翻译引擎的强大能力,我们的`BCEmbedding`具备强大的中英双语和跨语种语义表征能力。
- **RAG适配**:面向RAG做了针对性优化,可以适配大多数相关任务,比如**翻译,摘要,问答**等。此外,针对**问题理解**(query understanding)也做了针对优化,详见 <a href="#rag-evaluations-in-llamaindex">基于LlamaIndex的RAG评测指标</a>。
- **高效且精确的语义检索**:`EmbeddingModel`采用双编码器,可以在第一阶段实现高效的语义检索。`RerankerModel`采用交叉编码器,可以在第二阶段实现更高精度的语义顺序精排。
- **更好的领域泛化性**:为了在更多场景实现更好的效果,我们收集了多种多样的领域数据。
- **用户友好**:语义检索时不需要特殊指令前缀。也就是,你不需要为各种任务绞尽脑汁设计指令前缀。
- **有意义的重排序分数**:`RerankerModel`可以提供有意义的语义相关性分数(不仅仅是排序),可以用于过滤无意义文本片段,提高大模型生成效果。
- **产品化检验**:`BCEmbedding`已经被有道众多真实产品检验。
## 🚀 Latest Updates
- ***2024-01-03***: **Model Releases** - [bce-embedding-base_v1](https://huggingface.co/maidalun1020/bce-embedding-base_v1) and [bce-reranker-base_v1](https://huggingface.co/maidalun1020/bce-reranker-base_v1) are available.
- ***2024-01-03***: **Eval Datasets** [[CrosslingualMultiDomainsDataset](https://huggingface.co/datasets/maidalun1020/CrosslingualMultiDomainsDataset)] - Evaluate the performence of RAG, using [LlamaIndex](https://github.com/run-llama/llama_index).
- ***2024-01-03***: **Eval Datasets** [[Details](https://github.com/netease-youdao/BCEmbedding/blob/master/BCEmbedding/evaluation/c_mteb/Retrieval.py)] - Evaluate the performence of crosslingual semantic representation, using [MTEB](https://github.com/embeddings-benchmark/mteb).
- ***2024-01-03***: **模型发布** - [bce-embedding-base_v1](https://huggingface.co/maidalun1020/bce-embedding-base_v1)和[bce-reranker-base_v1](https://huggingface.co/maidalun1020/bce-reranker-base_v1)已发布.
- ***2024-01-03***: **RAG评测数据** [[CrosslingualMultiDomainsDataset](https://huggingface.co/datasets/maidalun1020/CrosslingualMultiDomainsDataset)] - 基于[LlamaIndex](https://github.com/run-llama/llama_index)的RAG评测数据已发布。
- ***2024-01-03***: **跨语种语义表征评测数据** [[详情](https://github.com/netease-youdao/BCEmbedding/blob/master/BCEmbedding/evaluation/c_mteb/Retrieval.py)] - 基于[MTEB](https://github.com/embeddings-benchmark/mteb)的跨语种评测数据已发布.
## 🍎 Model List
| Model Name | Model Type | Languages | Parameters | Weights |
|:-------------------------------|:--------:|:--------:|:--------:|:--------:|
| bce-embedding-base_v1 | `EmbeddingModel` | ch, en | 279M | [download](https://huggingface.co/maidalun1020/bce-embedding-base_v1) |
| bce-reranker-base_v1 | `RerankerModel` | ch, en, ja, ko | 279M | [download](https://huggingface.co/maidalun1020/bce-reranker-base_v1) |
## 📖 Manual
### Installation
First, create a conda environment and activate it.
```bash
conda create --name bce python=3.10 -y
conda activate bce
```
Then install `BCEmbedding` for minimal installation:
```bash
pip install BCEmbedding==0.1.1
```
Or install from source:
```bash
git clone git@github.com:netease-youdao/BCEmbedding.git
cd BCEmbedding
pip install -v -e .
```
### Quick Start
#### 1. Based on `BCEmbedding`
Use `EmbeddingModel`, and `cls` [pooler](./BCEmbedding/models/embedding.py#L24) is default.
```python
from BCEmbedding import EmbeddingModel
# list of sentences
sentences = ['sentence_0', 'sentence_1', ...]
# init embedding model
model = EmbeddingModel(model_name_or_path="maidalun1020/bce-embedding-base_v1")
# extract embeddings
embeddings = model.encode(sentences)
```
Use `RerankerModel` to calculate relevant scores and rerank:
```python
from BCEmbedding import RerankerModel
# your query and corresponding passages
query = 'input_query'
passages = ['passage_0', 'passage_1', ...]
# construct sentence pairs
sentence_pairs = [[query, passage] for passage in passages]
# init reranker model
model = RerankerModel(model_name_or_path="maidalun1020/bce-reranker-base_v1")
# method 0: calculate scores of sentence pairs
scores = model.compute_score(sentence_pairs)
# method 1: rerank passages
rerank_results = model.rerank(query, passages)
```
NOTE:
- In [`RerankerModel.rerank`](./BCEmbedding/models/reranker.py#L137) method, we provide an advanced preproccess that we use in production for making `sentence_pairs`, when "passages" are very long.
#### 2. Based on `transformers`
For `EmbeddingModel`:
```python
from transformers import AutoModel, AutoTokenizer
# list of sentences
sentences = ['sentence_0', 'sentence_1', ...]
# init model and tokenizer
tokenizer = AutoTokenizer.from_pretrained('maidalun1020/bce-embedding-base_v1')
model = AutoModel.from_pretrained('maidalun1020/bce-embedding-base_v1')
device = 'cuda' # if no GPU, set "cpu"
model.to(device)
# get inputs
inputs = tokenizer(sentences, padding=True, truncation=True, max_length=512, return_tensors="pt")
inputs_on_device = {k: v.to(self.device) for k, v in inputs.items()}
# get embeddings
outputs = model(**inputs_on_device, return_dict=True)
embeddings = outputs.last_hidden_state[:, 0] # cls pooler
embeddings = embeddings / embeddings.norm(dim=1, keepdim=True) # normalize
```
For `RerankerModel`:
```python
import torch
from transformers import AutoTokenizer, AutoModelForSequenceClassification
# init model and tokenizer
tokenizer = AutoTokenizer.from_pretrained('maidalun1020/bce-reranker-base_v1')
model = AutoModelForSequenceClassification.from_pretrained('maidalun1020/bce-reranker-base_v1')
device = 'cuda' # if no GPU, set "cpu"
model.to(device)
# get inputs
inputs = tokenizer(sentence_pairs, padding=True, truncation=True, max_length=512, return_tensors="pt")
inputs_on_device = {k: v.to(device) for k, v in inputs.items()}
# calculate scores
scores = model(**inputs_on_device, return_dict=True).logits.view(-1,).float()
scores = torch.sigmoid(scores)
```
#### 3. Based on `sentence_transformers`
For `EmbeddingModel`:
```python
from sentence_transformers import SentenceTransformer
# list of sentences
sentences = ['sentence_0', 'sentence_1', ...]
# init embedding model
## New update for sentence-trnasformers. So clean up your "`SENTENCE_TRANSFORMERS_HOME`/maidalun1020_bce-embedding-base_v1" or "~/.cache/torch/sentence_transformers/maidalun1020_bce-embedding-base_v1" first for downloading new version.
model = SentenceTransformer("maidalun1020/bce-embedding-base_v1")
# extract embeddings
embeddings = model.encode(sentences, normalize_embeddings=True)
```
For `RerankerModel`:
```python
from sentence_transformers import CrossEncoder
# init reranker model
model = CrossEncoder('maidalun1020/bce-reranker-base_v1', max_length=512)
# calculate scores of sentence pairs
scores = model.predict(sentence_pairs)
```
### Integrations for RAG Frameworks
#### 1. Used in `langchain`
```python
from langchain.embeddings import HuggingFaceEmbeddings
from langchain_community.vectorstores import FAISS
from langchain_community.vectorstores.utils import DistanceStrategy
query = 'apples'
passages = [
'I like apples',
'I like oranges',
'Apples and oranges are fruits'
]
# init embedding model
model_name = 'maidalun1020/bce-embedding-base_v1'
model_kwargs = {'device': 'cuda'}
encode_kwargs = {'batch_size': 64, 'normalize_embeddings': True, 'show_progress_bar': False}
embed_model = HuggingFaceEmbeddings(
model_name=model_name,
model_kwargs=model_kwargs,
encode_kwargs=encode_kwargs
)
# example #1. extract embeddings
query_embedding = embed_model.embed_query(query)
passages_embeddings = embed_model.embed_documents(passages)
# example #2. langchain retriever example
faiss_vectorstore = FAISS.from_texts(passages, embed_model, distance_strategy=DistanceStrategy.MAX_INNER_PRODUCT)
retriever = faiss_vectorstore.as_retriever(search_type="similarity", search_kwargs={"score_threshold": 0.5, "k": 3})
related_passages = retriever.get_relevant_documents(query)
```
#### 2. Used in `llama_index`
```python
from llama_index.embeddings import HuggingFaceEmbedding
from llama_index import VectorStoreIndex, ServiceContext, SimpleDirectoryReader
from llama_index.node_parser import SimpleNodeParser
from llama_index.llms import OpenAI
query = 'apples'
passages = [
'I like apples',
'I like oranges',
'Apples and oranges are fruits'
]
# init embedding model
model_args = {'model_name': 'maidalun1020/bce-embedding-base_v1', 'max_length': 512, 'embed_batch_size': 64, 'device': 'cuda'}
embed_model = HuggingFaceEmbedding(**model_args)
# example #1. extract embeddings
query_embedding = embed_model.get_query_embedding(query)
passages_embeddings = embed_model.get_text_embedding_batch(passages)
# example #2. rag example
llm = OpenAI(model='gpt-3.5-turbo-0613', api_key=os.environ.get('OPENAI_API_KEY'), api_base=os.environ.get('OPENAI_BASE_URL'))
service_context = ServiceContext.from_defaults(llm=llm, embed_model=embed_model)
documents = SimpleDirectoryReader(input_files=["BCEmbedding/tools/eval_rag/eval_pdfs/Comp_en_llama2.pdf"]).load_data()
node_parser = SimpleNodeParser.from_defaults(chunk_size=512)
nodes = node_parser.get_nodes_from_documents(documents[0:36])
index = VectorStoreIndex(nodes, service_context=service_context)
query_engine = index.as_query_engine()
response = query_engine.query("What is llama?")
```
## ⚙️ Evaluation
### Evaluate Semantic Representation by MTEB
We provide evaluateion tools for `embedding` and `reranker` models, based on [MTEB](https://github.com/embeddings-benchmark/mteb) and [C_MTEB](https://github.com/FlagOpen/FlagEmbedding/tree/master/C_MTEB).
我们基于[MTEB](https://github.com/embeddings-benchmark/mteb)和[C_MTEB](https://github.com/FlagOpen/FlagEmbedding/tree/master/C_MTEB),提供`embedding`和`reranker`模型的语义表征评测工具。
#### 1. Embedding Models
Just run following cmd to evaluate `your_embedding_model` (e.g. `maidalun1020/bce-embedding-base_v1`) in **bilingual and crosslingual settings** (e.g. `["en", "zh", "en-zh", "zh-en"]`).
运行下面命令评测`your_embedding_model`(比如,`maidalun1020/bce-embedding-base_v1`)。评测任务将会在**双语和跨语种**(比如,`["en", "zh", "en-zh", "zh-en"]`)模式下评测:
```bash
python BCEmbedding/tools/eval_mteb/eval_embedding_mteb.py --model_name_or_path maidalun1020/bce-embedding-base_v1 --pooler cls
```
The total evaluation tasks contain ***114 datastes*** of **"Retrieval", "STS", "PairClassification", "Classification", "Reranking" and "Clustering"**.
评测包含 **"Retrieval", "STS", "PairClassification", "Classification", "Reranking"和"Clustering"** 这六大类任务的 ***114个数据集***。
***NOTE:***
- **All models are evaluated in their recommended pooling method (`pooler`)**.
- `mean` pooler: "jina-embeddings-v2-base-en", "m3e-base", "m3e-large", "e5-large-v2", "multilingual-e5-base", "multilingual-e5-large" and "gte-large".
- `cls` pooler: Other models.
- "jina-embeddings-v2-base-en" model should be loaded with `trust_remote_code`.
```bash
python BCEmbedding/tools/eval_mteb/eval_embedding_mteb.py --model_name_or_path {moka-ai/m3e-base | moka-ai/m3e-large} --pooler mean
python BCEmbedding/tools/eval_mteb/eval_embedding_mteb.py --model_name_or_path jinaai/jina-embeddings-v2-base-en --pooler mean --trust_remote_code
```
***注意:***
- 所有模型的评测采用各自推荐的`pooler`。"jina-embeddings-v2-base-en", "m3e-base", "m3e-large", "e5-large-v2", "multilingual-e5-base", "multilingual-e5-large"和"gte-large"的 `pooler`采用`mean`,其他模型的`pooler`采用`cls`.
- "jina-embeddings-v2-base-en"模型在载入时需要`trust_remote_code`。
#### 2. Reranker Models
Run following cmd to evaluate `your_reranker_model` (e.g. "maidalun1020/bce-reranker-base_v1") in **bilingual and crosslingual settings** (e.g. `["en", "zh", "en-zh", "zh-en"]`).
运行下面命令评测`your_reranker_model`(比如,`maidalun1020/bce-reranker-base_v1`)。评测任务将会在 **双语种和跨语种**(比如,`["en", "zh", "en-zh", "zh-en"]`)模式下评测:
```bash
python BCEmbedding/tools/eval_mteb/eval_reranker_mteb.py --model_name_or_path maidalun1020/bce-reranker-base_v1
```
The evaluation tasks contain ***12 datastes*** of **"Reranking"**.
评测包含 **"Reranking"** 任务的 ***12个数据集***。
#### 3. Metrics Visualization Tool
We proveide a one-click script to sumarize evaluation results of `embedding` and `reranker` models as [Embedding Models Evaluation Summary](https://github.com/netease-youdao/BCEmbedding/blob/master/Docs/EvaluationSummary/embedding_eval_summary.md) and [Reranker Models Evaluation Summary](https://github.com/netease-youdao/BCEmbedding/blob/master/Docs/EvaluationSummary/reranker_eval_summary.md).
我们提供了`embedding`和`reranker`模型的指标可视化一键脚本,输出一个markdown文件,详见[Embedding模型指标汇总](https://github.com/netease-youdao/BCEmbedding/blob/master/Docs/EvaluationSummary/embedding_eval_summary.md)和[Reranker模型指标汇总](https://github.com/netease-youdao/BCEmbedding/blob/master/Docs/EvaluationSummary/reranker_eval_summary.md)。
```bash
python BCEmbedding/evaluation/mteb/summarize_eval_results.py --results_dir {your_embedding_results_dir | your_reranker_results_dir}
```
### Evaluate RAG by LlamaIndex
[LlamaIndex](https://github.com/run-llama/llama_index) is a famous data framework for LLM-based applications, particularly in RAG. Recently, the [LlamaIndex Blog](https://blog.llamaindex.ai/boosting-rag-picking-the-best-embedding-reranker-models-42d079022e83) has evaluated the popular embedding and reranker models in RAG pipeline and attract great attention. Now, we follow its pipeline to evaluate our `BCEmbedding`.
[LlamaIndex](https://github.com/run-llama/llama_index)是一个著名的大模型应用的开源工具,在RAG中很受欢迎。最近,[LlamaIndex博客](https://blog.llamaindex.ai/boosting-rag-picking-the-best-embedding-reranker-models-42d079022e83)对市面上常用的embedding和reranker模型进行RAG流程的评测,吸引广泛关注。下面我们按照该评测流程验证`BCEmbedding`在RAG中的效果。
First, install LlamaIndex:
```bash
pip install llama-index==0.9.22
```
#### 1. Metrics Definition
- Hit Rate:
Hit rate calculates the fraction of queries where the correct answer is found within the top-k retrieved documents. In simpler terms, it's about how often our system gets it right within the top few guesses. ***The larger, the better.***
- Mean Reciprocal Rank (MRR):
For each query, MRR evaluates the system's accuracy by looking at the rank of the highest-placed relevant document. Specifically, it's the average of the reciprocals of these ranks across all the queries. So, if the first relevant document is the top result, the reciprocal rank is 1; if it's second, the reciprocal rank is 1/2, and so on. ***The larger, the better.***
- 命中率(Hit Rate)
命中率计算的是在检索的前k个文档中找到正确答案的查询所占的比例。简单来说,它反映了我们的系统在前几次猜测中答对的频率。***该指标越大越好。***
- 平均倒数排名(Mean Reciprocal Rank,MRR)
对于每个查询,MRR通过查看最高排名的相关文档的排名来评估系统的准确性。具体来说,它是在所有查询中这些排名的倒数的平均值。因此,如果第一个相关文档是排名最靠前的结果,倒数排名就是1;如果是第二个,倒数排名就是1/2,依此类推。***该指标越大越好。***
#### 2. Reproduce [LlamaIndex Blog](https://blog.llamaindex.ai/boosting-rag-picking-the-best-embedding-reranker-models-42d079022e83)
In order to compare our `BCEmbedding` with other embedding and reranker models fairly, we provide a one-click script to reproduce results of the LlamaIndex Blog, including our `BCEmbedding`:
为了公平起见,运行下面脚本,复现LlamaIndex博客的结果,将`BCEmbedding`与其他embedding和reranker模型进行对比分析:
```bash
# There should be two GPUs available at least.
CUDA_VISIBLE_DEVICES=0,1 python BCEmbedding/tools/eval_rag/eval_llamaindex_reproduce.py
```
Then, sumarize the evaluation results by:
```bash
python BCEmbedding/tools/eval_rag/summarize_eval_results.py --results_dir results/rag_reproduce_results
```
Results Reproduced from the LlamaIndex Blog can be checked in ***[Reproduced Summary of RAG Evaluation](https://github.com/netease-youdao/BCEmbedding/blob/master/Docs/EvaluationSummary/rag_eval_reproduced_summary.md)***, with some obvious ***conclusions***:
- In `WithoutReranker` setting, our `bce-embedding-base_v1` outperforms all the other embedding models.
- With fixing the embedding model, our `bce-reranker-base_v1` achieves the best performence.
- ***The combination of `bce-embedding-base_v1` and `bce-reranker-base_v1` is SOTA.***
输出的指标汇总详见 ***[LlamaIndex RAG评测结果复现](https://github.com/netease-youdao/BCEmbedding/blob/master/Docs/EvaluationSummary/rag_eval_reproduced_summary.md)***。从该复现结果中,可以看出:
- 在`WithoutReranker`设置下(**竖排对比**),`bce-embedding-base_v1`比其他embedding模型效果都要好。
- 在固定embedding模型设置下,对比不同reranker效果(**横排对比**),`bce-reranker-base_v1`比其他reranker模型效果都要好。
- ***`bce-embedding-base_v1`和`bce-reranker-base_v1`组合,表现SOTA。***
#### 3. Broad Domain Adaptability
The evaluation of [LlamaIndex Blog](https://blog.llamaindex.ai/boosting-rag-picking-the-best-embedding-reranker-models-42d079022e83) is **monolingual, small amount of data, and specific domain** (just including "llama2" paper). In order to evaluate the **broad domain adaptability, bilingual and crosslingual capability**, we follow the blog to build a multiple domains evaluation dataset (includding "Computer Science", "Physics", "Biology", "Economics", "Math", and "Quantitative Finance"), named [CrosslingualMultiDomainsDataset](https://huggingface.co/datasets/maidalun1020/CrosslingualMultiDomainsDataset), **by OpenAI `gpt-4-1106-preview` for high quality**.
在上述的[LlamaIndex博客](https://blog.llamaindex.ai/boosting-rag-picking-the-best-embedding-reranker-models-42d079022e83)的评测数据只用了“llama2”这一篇文章,该评测是 **单语种,小数据量,特定领域** 的。为了兼容更真实更广的用户使用场景,评测算法模型的 **领域泛化性,双语和跨语种能力**,我们按照该博客的方法构建了一个多领域(计算机科学,物理学,生物学,经济学,数学,量化金融等)的双语种、跨语种评测数据,[CrosslingualMultiDomainsDataset](https://huggingface.co/datasets/maidalun1020/CrosslingualMultiDomainsDataset)。**为了保证构建数据的高质量,我们采用OpenAI的`gpt-4-1106-preview`。**
First, run following cmd to evaluate the most popular and powerful embedding and reranker models:
```bash
# There should be two GPUs available at least.
CUDA_VISIBLE_DEVICES=0,1 python BCEmbedding/tools/eval_rag/eval_llamaindex_multiple_domains.py
```
Then, run the following script to sumarize the evaluation results:
```bash
python BCEmbedding/tools/eval_rag/summarize_eval_results.py --results_dir results/rag_results
```
The summary of multiple domains evaluations can be seen in <a href=#1-multiple-domains-scenarios>Multiple Domains Scenarios</a>.
## 📈 Leaderboard
### Semantic Representation Evaluations in MTEB
#### 1. Embedding Models
| Model | Dimensions | Pooler | Instructions | Retrieval (47) | STS (19) | PairClassification (5) | Classification (21) | Reranking (12) | Clustering (15) | ***AVG*** (119) |
|:--------|:--------:|:--------:|:--------:|:--------:|:--------:|:--------:|:--------:|:--------:|:--------:|:--------:|
| bge-base-en-v1.5 | 768 | `cls` | Need | 37.14 | 55.06 | 75.45 | 59.73 | 43.00 | 37.74 | 47.19 |
| bge-base-zh-v1.5 | 768 | `cls` | Need | 47.63 | 63.72 | 77.40 | 63.38 | 54.95 | 32.56 | 53.62 |
| bge-large-en-v1.5 | 1024 | `cls` | Need | 37.18 | 54.09 | 75.00 | 59.24 | 42.47 | 37.32 | 46.80 |
| bge-large-zh-v1.5 | 1024 | `cls` | Need | 47.58 | 64.73 | 79.14 | 64.19 | 55.98 | 33.26 | 54.23 |
| e5-large-v2 | 1024 | `mean` | Need | 35.98 | 55.23 | 75.28 | 59.53 | 42.12 | 36.51 | 46.52 |
| gte-large | 1024 | `mean` | Free | 36.68 | 55.22 | 74.29 | 57.73 | 42.44 | 38.51 | 46.67 |
| gte-large-zh | 1024 | `cls` | Free | 41.15 | 64.62 | 77.58 | 62.04 | 55.62 | 33.03 | 51.51 |
| jina-embeddings-v2-base-en | 768 | `mean` | Free | 31.58 | 54.28 | 74.84 | 58.42 | 41.16 | 34.67 | 44.29 |
| m3e-base | 768 | `mean` | Free | 46.29 | 63.93 | 71.84 | 64.08 | 52.38 | 37.84 | 53.54 |
| m3e-large | 1024 | `mean` | Free | 34.85 | 59.74 | 67.69 | 60.07 | 48.99 | 31.62 | 46.78 |
| multilingual-e5-base | 768 | `mean` | Need | 54.73 | 65.49 | 76.97 | 69.72 | 55.01 | 38.44 | 58.34 |
| multilingual-e5-large | 1024 | `mean` | Need | 56.76 | 66.79 | 78.80 | 71.61 | 56.49 | 43.09 | 60.50 |
| ***bce-embedding-base_v1*** | 768 | `cls` | Free | 57.60 | 65.73 | 74.96 | 69.00 | 57.29 | 38.95 | 59.43 |
***NOTE:***
- Our ***bce-embedding-base_v1*** outperforms other opensource embedding models with comparable model size.
- ***114 datastes*** of **"Retrieval", "STS", "PairClassification", "Classification", "Reranking" and "Clustering"** in `["en", "zh", "en-zh", "zh-en"]` setting.
- The [crosslingual evaluation datasets](https://github.com/netease-youdao/BCEmbedding/blob/master/BCEmbedding/evaluation/c_mteb/Retrieval.py) we released belong to `Retrieval` task.
- More evaluation details please check [Embedding Models Evaluation Summary](https://github.com/netease-youdao/BCEmbedding/blob/master/Docs/EvaluationSummary/embedding_eval_summary.md).
***要点:***
- 对比其他开源的相同规模的embedding模型,***bce-embedding-base_v1*** 表现最好,效果比最好的large模型稍差。
- 评测包含 **"Retrieval", "STS", "PairClassification", "Classification", "Reranking"和"Clustering"** 这六大类任务的共 ***114个数据集***。
- 我们开源的[跨语种语义表征评测数据](https://github.com/netease-youdao/BCEmbedding/blob/master/BCEmbedding/evaluation/c_mteb/Retrieval.py)属于`Retrieval`任务。
- 更详细的评测结果详见[Embedding模型指标汇总](https://github.com/netease-youdao/BCEmbedding/blob/master/Docs/EvaluationSummary/embedding_eval_summary.md)。
#### 2. Reranker Models
| Model | Reranking (12) | ***AVG*** (12) |
| :--------------------------------- | :-------------: | :--------------------: |
| bge-reranker-base | 59.04 | 59.04 |
| bge-reranker-large | 60.86 | 60.86 |
| ***bce-reranker-base_v1*** | **61.29** | ***61.29*** |
***NOTE:***
- Our ***bce-reranker-base_v1*** outperforms other opensource reranker models.
- ***12 datastes*** of **"Reranking"** in `["en", "zh", "en-zh", "zh-en"]` setting.
- More evaluation details please check [Reranker Models Evaluation Summary](https://github.com/netease-youdao/BCEmbedding/blob/master/Docs/EvaluationSummary/reranker_eval_summary.md).
***要点:***
- ***bce-reranker-base_v1*** 优于其他开源reranker模型。
- 评测包含 **"Reranking"** 任务的 ***12个数据集***。
- 更详细的评测结果详见[Reranker模型指标汇总](https://github.com/netease-youdao/BCEmbedding/blob/master/Docs/EvaluationSummary/reranker_eval_summary.md)
### RAG Evaluations in LlamaIndex
#### 1. Multiple Domains Scenarios

***NOTE:***
- Evaluated in **`["en", "zh", "en-zh", "zh-en"]` setting**.
- In `WithoutReranker` setting, our `bce-embedding-base_v1` outperforms all the other embedding models.
- With fixing the embedding model, our `bce-reranker-base_v1` achieves the best performence.
- **The combination of `bce-embedding-base_v1` and `bce-reranker-base_v1` is SOTA**.
***要点:***
- 评测是在`["en", "zh", "en-zh", "zh-en"]`设置下。
- 在`WithoutReranker`设置下(**竖排对比**),`bce-embedding-base_v1`优于其他Embedding模型,包括开源和闭源。
- 在固定Embedding模型设置下,对比不同reranker效果(**横排对比**),`bce-reranker-base_v1`比其他reranker模型效果都要好,包括开源和闭源。
- ***`bce-embedding-base_v1`和`bce-reranker-base_v1`组合,表现SOTA。***
## 🛠 Youdao's BCEmbedding API
For users who prefer a hassle-free experience without the need to download and configure the model on their own systems, `BCEmbedding` is readily accessible through Youdao's API. This option offers a streamlined and efficient way to integrate BCEmbedding into your projects, bypassing the complexities of manual setup and maintenance. Detailed instructions and comprehensive API documentation are available at [Youdao BCEmbedding API](https://ai.youdao.com/DOCSIRMA/html/aigc/api/embedding/index.html). Here, you'll find all the necessary guidance to easily implement `BCEmbedding` across a variety of use cases, ensuring a smooth and effective integration for optimal results.
对于那些更喜欢直接调用api的用户,有道提供方便的`BCEmbedding`调用api。该方式是一种简化和高效的方式,将`BCEmbedding`集成到您的项目中,避开了手动设置和系统维护的复杂性。更详细的api调用接口说明详见[有道BCEmbedding API](https://ai.youdao.com/DOCSIRMA/html/aigc/api/embedding/index.html)。
## 🧲 WeChat Group
Welcome to scan the QR code below and join the WeChat group.
欢迎大家扫码加入官方微信交流群。

## ✏️ Citation
If you use `BCEmbedding` in your research or project, please feel free to cite and star it:
如果在您的研究或任何项目中使用本工作,烦请按照下方进行引用,并打个小星星~
```
@misc{youdao_bcembedding_2023,
title={BCEmbedding: Bilingual and Crosslingual Embedding for RAG},
author={NetEase Youdao, Inc.},
year={2023},
howpublished={\url{https://github.com/netease-youdao/BCEmbedding}}
}
```
## 🔐 License
`BCEmbedding` is licensed under [Apache 2.0 License](https://github.com/netease-youdao/BCEmbedding/blob/master/LICENSE)
## 🔗 Related Links
[Netease Youdao - QAnything](https://github.com/netease-youdao/qanything)
[FlagEmbedding](https://github.com/FlagOpen/FlagEmbedding)
[MTEB](https://github.com/embeddings-benchmark/mteb)
[C_MTEB](https://github.com/FlagOpen/FlagEmbedding/tree/master/C_MTEB)
[LLama Index](https://github.com/run-llama/llama_index) | [LlamaIndex Blog](https://blog.llamaindex.ai/boosting-rag-picking-the-best-embedding-reranker-models-42d079022e83) |
mradermacher/MultiPL-T-CodeLlama_70b-GGUF | mradermacher | "2024-06-25T19:26:36Z" | 111,088 | 0 | transformers | [
"transformers",
"gguf",
"en",
"dataset:nuprl/MultiPL-T",
"base_model:nuprl/MultiPL-T-CodeLlama_70b",
"license:openrail",
"endpoints_compatible",
"region:us"
] | null | "2024-06-24T20:42:44Z" | ---
base_model: nuprl/MultiPL-T-CodeLlama_70b
datasets:
- nuprl/MultiPL-T
language:
- en
library_name: transformers
license: openrail
quantized_by: mradermacher
---
## About
<!-- ### quantize_version: 2 -->
<!-- ### output_tensor_quantised: 1 -->
<!-- ### convert_type: hf -->
<!-- ### vocab_type: -->
<!-- ### tags: -->
static quants of https://huggingface.co/nuprl/MultiPL-T-CodeLlama_70b
<!-- provided-files -->
weighted/imatrix quants are available at https://huggingface.co/mradermacher/MultiPL-T-CodeLlama_70b-i1-GGUF
## Usage
If you are unsure how to use GGUF files, refer to one of [TheBloke's
READMEs](https://huggingface.co/TheBloke/KafkaLM-70B-German-V0.1-GGUF) for
more details, including on how to concatenate multi-part files.
## Provided Quants
(sorted by size, not necessarily quality. IQ-quants are often preferable over similar sized non-IQ quants)
| Link | Type | Size/GB | Notes |
|:-----|:-----|--------:|:------|
| [GGUF](https://huggingface.co/mradermacher/MultiPL-T-CodeLlama_70b-GGUF/resolve/main/MultiPL-T-CodeLlama_70b.Q2_K.gguf) | Q2_K | 25.6 | |
| [GGUF](https://huggingface.co/mradermacher/MultiPL-T-CodeLlama_70b-GGUF/resolve/main/MultiPL-T-CodeLlama_70b.IQ3_XS.gguf) | IQ3_XS | 28.4 | |
| [GGUF](https://huggingface.co/mradermacher/MultiPL-T-CodeLlama_70b-GGUF/resolve/main/MultiPL-T-CodeLlama_70b.IQ3_S.gguf) | IQ3_S | 30.0 | beats Q3_K* |
| [GGUF](https://huggingface.co/mradermacher/MultiPL-T-CodeLlama_70b-GGUF/resolve/main/MultiPL-T-CodeLlama_70b.Q3_K_S.gguf) | Q3_K_S | 30.0 | |
| [GGUF](https://huggingface.co/mradermacher/MultiPL-T-CodeLlama_70b-GGUF/resolve/main/MultiPL-T-CodeLlama_70b.IQ3_M.gguf) | IQ3_M | 31.0 | |
| [GGUF](https://huggingface.co/mradermacher/MultiPL-T-CodeLlama_70b-GGUF/resolve/main/MultiPL-T-CodeLlama_70b.Q3_K_M.gguf) | Q3_K_M | 33.4 | lower quality |
| [GGUF](https://huggingface.co/mradermacher/MultiPL-T-CodeLlama_70b-GGUF/resolve/main/MultiPL-T-CodeLlama_70b.Q3_K_L.gguf) | Q3_K_L | 36.2 | |
| [GGUF](https://huggingface.co/mradermacher/MultiPL-T-CodeLlama_70b-GGUF/resolve/main/MultiPL-T-CodeLlama_70b.IQ4_XS.gguf) | IQ4_XS | 37.3 | |
| [GGUF](https://huggingface.co/mradermacher/MultiPL-T-CodeLlama_70b-GGUF/resolve/main/MultiPL-T-CodeLlama_70b.Q4_K_S.gguf) | Q4_K_S | 39.3 | fast, recommended |
| [GGUF](https://huggingface.co/mradermacher/MultiPL-T-CodeLlama_70b-GGUF/resolve/main/MultiPL-T-CodeLlama_70b.Q4_K_M.gguf) | Q4_K_M | 41.5 | fast, recommended |
| [GGUF](https://huggingface.co/mradermacher/MultiPL-T-CodeLlama_70b-GGUF/resolve/main/MultiPL-T-CodeLlama_70b.Q5_K_S.gguf) | Q5_K_S | 47.6 | |
| [GGUF](https://huggingface.co/mradermacher/MultiPL-T-CodeLlama_70b-GGUF/resolve/main/MultiPL-T-CodeLlama_70b.Q5_K_M.gguf) | Q5_K_M | 48.9 | |
| [PART 1](https://huggingface.co/mradermacher/MultiPL-T-CodeLlama_70b-GGUF/resolve/main/MultiPL-T-CodeLlama_70b.Q6_K.gguf.part1of2) [PART 2](https://huggingface.co/mradermacher/MultiPL-T-CodeLlama_70b-GGUF/resolve/main/MultiPL-T-CodeLlama_70b.Q6_K.gguf.part2of2) | Q6_K | 56.7 | very good quality |
| [PART 1](https://huggingface.co/mradermacher/MultiPL-T-CodeLlama_70b-GGUF/resolve/main/MultiPL-T-CodeLlama_70b.Q8_0.gguf.part1of2) [PART 2](https://huggingface.co/mradermacher/MultiPL-T-CodeLlama_70b-GGUF/resolve/main/MultiPL-T-CodeLlama_70b.Q8_0.gguf.part2of2) | Q8_0 | 73.4 | fast, best quality |
Here is a handy graph by ikawrakow comparing some lower-quality quant
types (lower is better):

And here are Artefact2's thoughts on the matter:
https://gist.github.com/Artefact2/b5f810600771265fc1e39442288e8ec9
## FAQ / Model Request
See https://huggingface.co/mradermacher/model_requests for some answers to
questions you might have and/or if you want some other model quantized.
## Thanks
I thank my company, [nethype GmbH](https://www.nethype.de/), for letting
me use its servers and providing upgrades to my workstation to enable
this work in my free time.
<!-- end -->
|
microsoft/wavlm-large | microsoft | "2022-02-02T21:21:50Z" | 110,668 | 48 | transformers | [
"transformers",
"pytorch",
"wavlm",
"feature-extraction",
"speech",
"en",
"arxiv:1912.07875",
"arxiv:2106.06909",
"arxiv:2101.00390",
"arxiv:2110.13900",
"region:us"
] | feature-extraction | "2022-03-02T23:29:05Z" | ---
language:
- en
tags:
- speech
inference: false
---
# WavLM-Large
[Microsoft's WavLM](https://github.com/microsoft/unilm/tree/master/wavlm)
The large model pretrained on 16kHz sampled speech audio. When using the model, make sure that your speech input is also sampled at 16kHz.
**Note**: This model does not have a tokenizer as it was pretrained on audio alone. In order to use this model **speech recognition**, a tokenizer should be created and the model should be fine-tuned on labeled text data. Check out [this blog](https://huggingface.co/blog/fine-tune-wav2vec2-english) for more in-detail explanation of how to fine-tune the model.
The model was pre-trained on:
- 60,000 hours of [Libri-Light](https://arxiv.org/abs/1912.07875)
- 10,000 hours of [GigaSpeech](https://arxiv.org/abs/2106.06909)
- 24,000 hours of [VoxPopuli](https://arxiv.org/abs/2101.00390)
[Paper: WavLM: Large-Scale Self-Supervised Pre-Training for Full Stack Speech Processing](https://arxiv.org/abs/2110.13900)
Authors: Sanyuan Chen, Chengyi Wang, Zhengyang Chen, Yu Wu, Shujie Liu, Zhuo Chen, Jinyu Li, Naoyuki Kanda, Takuya Yoshioka, Xiong Xiao, Jian Wu, Long Zhou, Shuo Ren, Yanmin Qian, Yao Qian, Jian Wu, Michael Zeng, Furu Wei
**Abstract**
*Self-supervised learning (SSL) achieves great success in speech recognition, while limited exploration has been attempted for other speech processing tasks. As speech signal contains multi-faceted information including speaker identity, paralinguistics, spoken content, etc., learning universal representations for all speech tasks is challenging. In this paper, we propose a new pre-trained model, WavLM, to solve full-stack downstream speech tasks. WavLM is built based on the HuBERT framework, with an emphasis on both spoken content modeling and speaker identity preservation. We first equip the Transformer structure with gated relative position bias to improve its capability on recognition tasks. For better speaker discrimination, we propose an utterance mixing training strategy, where additional overlapped utterances are created unsupervisely and incorporated during model training. Lastly, we scale up the training dataset from 60k hours to 94k hours. WavLM Large achieves state-of-the-art performance on the SUPERB benchmark, and brings significant improvements for various speech processing tasks on their representative benchmarks.*
The original model can be found under https://github.com/microsoft/unilm/tree/master/wavlm.
# Usage
This is an English pre-trained speech model that has to be fine-tuned on a downstream task like speech recognition or audio classification before it can be
used in inference. The model was pre-trained in English and should therefore perform well only in English. The model has been shown to work well on the [SUPERB benchmark](https://superbbenchmark.org/).
**Note**: The model was pre-trained on phonemes rather than characters. This means that one should make sure that the input text is converted to a sequence
of phonemes before fine-tuning.
## Speech Recognition
To fine-tune the model for speech recognition, see [the official speech recognition example](https://github.com/huggingface/transformers/tree/master/examples/pytorch/speech-recognition).
## Speech Classification
To fine-tune the model for speech classification, see [the official audio classification example](https://github.com/huggingface/transformers/tree/master/examples/pytorch/audio-classification).
## Speaker Verification
TODO
## Speaker Diarization
TODO
# Contribution
The model was contributed by [cywang](https://huggingface.co/cywang) and [patrickvonplaten](https://huggingface.co/patrickvonplaten).
# License
The official license can be found [here](https://github.com/microsoft/UniSpeech/blob/main/LICENSE)
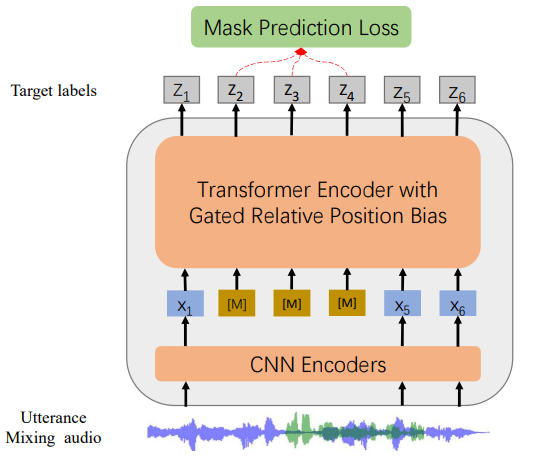 |
mradermacher/ProgressGym-HistLlama3-70B-C013-instruct-v0.1-i1-GGUF | mradermacher | "2024-07-02T12:47:32Z" | 110,411 | 1 | transformers | [
"transformers",
"gguf",
"en",
"dataset:PKU-Alignment/ProgressGym-HistText",
"dataset:PKU-Alignment/ProgressGym-TimelessQA",
"base_model:PKU-Alignment/ProgressGym-HistLlama3-70B-C013-instruct-v0.1",
"license:cc-by-4.0",
"endpoints_compatible",
"region:us"
] | null | "2024-07-01T12:08:59Z" | ---
base_model: PKU-Alignment/ProgressGym-HistLlama3-70B-C013-instruct-v0.1
datasets:
- PKU-Alignment/ProgressGym-HistText
- PKU-Alignment/ProgressGym-TimelessQA
language:
- en
library_name: transformers
license: cc-by-4.0
quantized_by: mradermacher
---
## About
<!-- ### quantize_version: 2 -->
<!-- ### output_tensor_quantised: 1 -->
<!-- ### convert_type: hf -->
<!-- ### vocab_type: -->
<!-- ### tags: nicoboss -->
weighted/imatrix quants of https://huggingface.co/PKU-Alignment/ProgressGym-HistLlama3-70B-C013-instruct-v0.1
<!-- provided-files -->
static quants are available at https://huggingface.co/mradermacher/ProgressGym-HistLlama3-70B-C013-instruct-v0.1-GGUF
## Usage
If you are unsure how to use GGUF files, refer to one of [TheBloke's
READMEs](https://huggingface.co/TheBloke/KafkaLM-70B-German-V0.1-GGUF) for
more details, including on how to concatenate multi-part files.
## Provided Quants
(sorted by size, not necessarily quality. IQ-quants are often preferable over similar sized non-IQ quants)
| Link | Type | Size/GB | Notes |
|:-----|:-----|--------:|:------|
| [GGUF](https://huggingface.co/mradermacher/ProgressGym-HistLlama3-70B-C013-instruct-v0.1-i1-GGUF/resolve/main/ProgressGym-HistLlama3-70B-C013-instruct-v0.1.i1-IQ1_S.gguf) | i1-IQ1_S | 15.4 | for the desperate |
| [GGUF](https://huggingface.co/mradermacher/ProgressGym-HistLlama3-70B-C013-instruct-v0.1-i1-GGUF/resolve/main/ProgressGym-HistLlama3-70B-C013-instruct-v0.1.i1-IQ1_M.gguf) | i1-IQ1_M | 16.9 | mostly desperate |
| [GGUF](https://huggingface.co/mradermacher/ProgressGym-HistLlama3-70B-C013-instruct-v0.1-i1-GGUF/resolve/main/ProgressGym-HistLlama3-70B-C013-instruct-v0.1.i1-IQ2_XXS.gguf) | i1-IQ2_XXS | 19.2 | |
| [GGUF](https://huggingface.co/mradermacher/ProgressGym-HistLlama3-70B-C013-instruct-v0.1-i1-GGUF/resolve/main/ProgressGym-HistLlama3-70B-C013-instruct-v0.1.i1-IQ2_XS.gguf) | i1-IQ2_XS | 21.2 | |
| [GGUF](https://huggingface.co/mradermacher/ProgressGym-HistLlama3-70B-C013-instruct-v0.1-i1-GGUF/resolve/main/ProgressGym-HistLlama3-70B-C013-instruct-v0.1.i1-IQ2_S.gguf) | i1-IQ2_S | 22.3 | |
| [GGUF](https://huggingface.co/mradermacher/ProgressGym-HistLlama3-70B-C013-instruct-v0.1-i1-GGUF/resolve/main/ProgressGym-HistLlama3-70B-C013-instruct-v0.1.i1-IQ2_M.gguf) | i1-IQ2_M | 24.2 | |
| [GGUF](https://huggingface.co/mradermacher/ProgressGym-HistLlama3-70B-C013-instruct-v0.1-i1-GGUF/resolve/main/ProgressGym-HistLlama3-70B-C013-instruct-v0.1.i1-Q2_K.gguf) | i1-Q2_K | 26.5 | IQ3_XXS probably better |
| [GGUF](https://huggingface.co/mradermacher/ProgressGym-HistLlama3-70B-C013-instruct-v0.1-i1-GGUF/resolve/main/ProgressGym-HistLlama3-70B-C013-instruct-v0.1.i1-IQ3_XXS.gguf) | i1-IQ3_XXS | 27.6 | lower quality |
| [GGUF](https://huggingface.co/mradermacher/ProgressGym-HistLlama3-70B-C013-instruct-v0.1-i1-GGUF/resolve/main/ProgressGym-HistLlama3-70B-C013-instruct-v0.1.i1-IQ3_XS.gguf) | i1-IQ3_XS | 29.4 | |
| [GGUF](https://huggingface.co/mradermacher/ProgressGym-HistLlama3-70B-C013-instruct-v0.1-i1-GGUF/resolve/main/ProgressGym-HistLlama3-70B-C013-instruct-v0.1.i1-IQ3_S.gguf) | i1-IQ3_S | 31.0 | beats Q3_K* |
| [GGUF](https://huggingface.co/mradermacher/ProgressGym-HistLlama3-70B-C013-instruct-v0.1-i1-GGUF/resolve/main/ProgressGym-HistLlama3-70B-C013-instruct-v0.1.i1-Q3_K_S.gguf) | i1-Q3_K_S | 31.0 | IQ3_XS probably better |
| [GGUF](https://huggingface.co/mradermacher/ProgressGym-HistLlama3-70B-C013-instruct-v0.1-i1-GGUF/resolve/main/ProgressGym-HistLlama3-70B-C013-instruct-v0.1.i1-IQ3_M.gguf) | i1-IQ3_M | 32.0 | |
| [GGUF](https://huggingface.co/mradermacher/ProgressGym-HistLlama3-70B-C013-instruct-v0.1-i1-GGUF/resolve/main/ProgressGym-HistLlama3-70B-C013-instruct-v0.1.i1-Q3_K_M.gguf) | i1-Q3_K_M | 34.4 | IQ3_S probably better |
| [GGUF](https://huggingface.co/mradermacher/ProgressGym-HistLlama3-70B-C013-instruct-v0.1-i1-GGUF/resolve/main/ProgressGym-HistLlama3-70B-C013-instruct-v0.1.i1-Q3_K_L.gguf) | i1-Q3_K_L | 37.2 | IQ3_M probably better |
| [GGUF](https://huggingface.co/mradermacher/ProgressGym-HistLlama3-70B-C013-instruct-v0.1-i1-GGUF/resolve/main/ProgressGym-HistLlama3-70B-C013-instruct-v0.1.i1-IQ4_XS.gguf) | i1-IQ4_XS | 38.0 | |
| [GGUF](https://huggingface.co/mradermacher/ProgressGym-HistLlama3-70B-C013-instruct-v0.1-i1-GGUF/resolve/main/ProgressGym-HistLlama3-70B-C013-instruct-v0.1.i1-Q4_0.gguf) | i1-Q4_0 | 40.2 | fast, low quality |
| [GGUF](https://huggingface.co/mradermacher/ProgressGym-HistLlama3-70B-C013-instruct-v0.1-i1-GGUF/resolve/main/ProgressGym-HistLlama3-70B-C013-instruct-v0.1.i1-Q4_K_S.gguf) | i1-Q4_K_S | 40.4 | optimal size/speed/quality |
| [GGUF](https://huggingface.co/mradermacher/ProgressGym-HistLlama3-70B-C013-instruct-v0.1-i1-GGUF/resolve/main/ProgressGym-HistLlama3-70B-C013-instruct-v0.1.i1-Q4_K_M.gguf) | i1-Q4_K_M | 42.6 | fast, recommended |
| [GGUF](https://huggingface.co/mradermacher/ProgressGym-HistLlama3-70B-C013-instruct-v0.1-i1-GGUF/resolve/main/ProgressGym-HistLlama3-70B-C013-instruct-v0.1.i1-Q5_K_S.gguf) | i1-Q5_K_S | 48.8 | |
| [GGUF](https://huggingface.co/mradermacher/ProgressGym-HistLlama3-70B-C013-instruct-v0.1-i1-GGUF/resolve/main/ProgressGym-HistLlama3-70B-C013-instruct-v0.1.i1-Q5_K_M.gguf) | i1-Q5_K_M | 50.0 | |
| [PART 1](https://huggingface.co/mradermacher/ProgressGym-HistLlama3-70B-C013-instruct-v0.1-i1-GGUF/resolve/main/ProgressGym-HistLlama3-70B-C013-instruct-v0.1.i1-Q6_K.gguf.part1of2) [PART 2](https://huggingface.co/mradermacher/ProgressGym-HistLlama3-70B-C013-instruct-v0.1-i1-GGUF/resolve/main/ProgressGym-HistLlama3-70B-C013-instruct-v0.1.i1-Q6_K.gguf.part2of2) | i1-Q6_K | 58.0 | practically like static Q6_K |
Here is a handy graph by ikawrakow comparing some lower-quality quant
types (lower is better):

And here are Artefact2's thoughts on the matter:
https://gist.github.com/Artefact2/b5f810600771265fc1e39442288e8ec9
## FAQ / Model Request
See https://huggingface.co/mradermacher/model_requests for some answers to
questions you might have and/or if you want some other model quantized.
## Thanks
I thank my company, [nethype GmbH](https://www.nethype.de/), for letting
me use its servers and providing upgrades to my workstation to enable
this work in my free time. Additional thanks to [@nicoboss](https://huggingface.co/nicoboss) for giving me access to his hardware for calculating the imatrix for these quants.
<!-- end -->
|
TencentARC/PhotoMaker | TencentARC | "2024-02-28T07:27:22Z" | 110,297 | 370 | diffusers | [
"diffusers",
"text-to-image",
"en",
"arxiv:2312.04461",
"license:apache-2.0",
"region:us"
] | text-to-image | "2024-01-13T14:11:54Z" | ---
license: apache-2.0
language:
- en
library_name: diffusers
pipeline_tag: text-to-image
---
# PhotoMaker Model Card
<div align="center">
[**Project Page**](https://photo-maker.github.io/) **|** [**Paper (ArXiv)**](https://arxiv.org/abs/2312.04461) **|** [**Code**](https://github.com/TencentARC/PhotoMaker)
[🤗 **Gradio demo (Realistic)**](https://huggingface.co/spaces/TencentARC/PhotoMaker) **|** [🤗 **Gradio demo (Stylization)**](https://huggingface.co/spaces/TencentARC/PhotoMaker-Style)
</div>
## Introduction
<!-- Provide a quick summary of what the model is/does. -->
Users can input one or a few face photos, along with a text prompt, to receive a customized photo or painting within seconds (no training required!). Additionally, this model can be adapted to any base model based on SDXL or used in conjunction with other LoRA modules.
### Realistic results


### Stylization results


More results can be found in our [project page](https://photo-maker.github.io/)
## Model Details
It mainly contains two parts corresponding to two keys in loaded state dict:
1. `id_encoder` includes finetuned OpenCLIP-ViT-H-14 and a few fuse layers.
2. `lora_weights` applies to all attention layers in the UNet, and the rank is set to 64.
## Usage
You can directly download the model in this repository.
You also can download the model in python script:
```python
from huggingface_hub import hf_hub_download
photomaker_ckpt = hf_hub_download(repo_id="TencentARC/PhotoMaker", filename="photomaker-v1.bin", repo_type="model")
```
Then, please follow the instructions in our [GitHub repository](https://github.com/TencentARC/PhotoMaker).
## Limitations
<!-- This section is meant to convey both technical and sociotechnical limitations. -->
- The model's customization performance degrades on Asian male faces.
- The model still struggles with accurately rendering human hands.
## Bias
While the capabilities of image generation models are impressive, they can also reinforce or exacerbate social biases.
## Citation
<!-- If there is a paper or blog post introducing the model, the APA and Bibtex information for that should go in this section. -->
**BibTeX:**
```bibtex
@inproceedings{li2023photomaker,
title={PhotoMaker: Customizing Realistic Human Photos via Stacked ID Embedding},
author={Li, Zhen and Cao, Mingdeng and Wang, Xintao and Qi, Zhongang and Cheng, Ming-Ming and Shan, Ying},
booktitle={IEEE Conference on Computer Vision and Pattern Recognition (CVPR)},
year={2024}
}
``` |
DionTimmer/controlnet_qrcode-control_v1p_sd15 | DionTimmer | "2023-06-15T23:34:29Z" | 110,074 | 215 | diffusers | [
"diffusers",
"safetensors",
"stable-diffusion",
"controlnet",
"image-to-image",
"en",
"license:openrail++",
"region:us"
] | image-to-image | "2023-06-15T21:50:00Z" | ---
tags:
- stable-diffusion
- controlnet
- image-to-image
license: openrail++
language:
- en
library_name: diffusers
pipeline_tag: image-to-image
---
# QR Code Conditioned ControlNet Models for Stable Diffusion 1.5

## Model Description
This repo holds the safetensors & diffusers versions of the QR code conditioned ControlNet for Stable Diffusion v1.5.
The Stable Diffusion 2.1 version is marginally more effective, as it was developed to address my specific needs. However, this 1.5 version model was also trained on the same dataset for those who are using the older version.
## How to use with Diffusers
```bash
pip -q install diffusers transformers accelerate torch xformers
```
```python
import torch
from PIL import Image
from diffusers import StableDiffusionControlNetImg2ImgPipeline, ControlNetModel, DDIMScheduler
from diffusers.utils import load_image
controlnet = ControlNetModel.from_pretrained("DionTimmer/controlnet_qrcode-control_v1p_sd15",
torch_dtype=torch.float16)
pipe = StableDiffusionControlNetImg2ImgPipeline.from_pretrained(
"runwayml/stable-diffusion-v1-5",
controlnet=controlnet,
safety_checker=None,
torch_dtype=torch.float16
)
pipe.enable_xformers_memory_efficient_attention()
pipe.scheduler = DDIMScheduler.from_config(pipe.scheduler.config)
pipe.enable_model_cpu_offload()
def resize_for_condition_image(input_image: Image, resolution: int):
input_image = input_image.convert("RGB")
W, H = input_image.size
k = float(resolution) / min(H, W)
H *= k
W *= k
H = int(round(H / 64.0)) * 64
W = int(round(W / 64.0)) * 64
img = input_image.resize((W, H), resample=Image.LANCZOS)
return img
# play with guidance_scale, controlnet_conditioning_scale and strength to make a valid QR Code Image
# qr code image
source_image = load_image("https://s3.amazonaws.com/moonup/production/uploads/6064e095abd8d3692e3e2ed6/A_RqHaAM6YHBodPLwqtjn.png")
# initial image, anything
init_image = load_image("https://s3.amazonaws.com/moonup/production/uploads/noauth/KfMBABpOwIuNolv1pe3qX.jpeg")
condition_image = resize_for_condition_image(source_image, 768)
init_image = resize_for_condition_image(init_image, 768)
generator = torch.manual_seed(123121231)
image = pipe(prompt="a bilboard in NYC with a qrcode",
negative_prompt="ugly, disfigured, low quality, blurry, nsfw",
image=init_image,
control_image=condition_image,
width=768,
height=768,
guidance_scale=20,
controlnet_conditioning_scale=1.5,
generator=generator,
strength=0.9,
num_inference_steps=150,
)
image.images[0]
```
## Performance and Limitations
These models perform quite well in most cases, but please note that they are not 100% accurate. In some instances, the QR code shape might not come through as expected. You can increase the ControlNet weight to emphasize the QR code shape. However, be cautious as this might negatively impact the style of your output.**To optimize for scanning, please generate your QR codes with correction mode 'H' (30%).**
To balance between style and shape, a gentle fine-tuning of the control weight might be required based on the individual input and the desired output, aswell as the correct prompt. Some prompts do not work until you increase the weight by a lot. The process of finding the right balance between these factors is part art and part science. For the best results, it is recommended to generate your artwork at a resolution of 768. This allows for a higher level of detail in the final product, enhancing the quality and effectiveness of the QR code-based artwork.
## Installation
The simplest way to use this is to place the .safetensors model and its .yaml config file in the folder where your other controlnet models are installed, which varies per application.
For usage in auto1111 they can be placed in the webui/models/ControlNet folder. They can be loaded using the controlnet webui extension which you can install through the extensions tab in the webui (https://github.com/Mikubill/sd-webui-controlnet). Make sure to enable your controlnet unit and set your input image as the QR code. Set the model to either the SD2.1 or 1.5 version depending on your base stable diffusion model, or it will error. No pre-processor is needed, though you can use the invert pre-processor for a different variation of results. 768 is the preferred resolution for generation since it allows for more detail.
Make sure to look up additional info on how to use controlnet if you get stuck, once you have the webui up and running its really easy to install the controlnet extension aswell. |
InstantX/InstantID | InstantX | "2024-01-22T09:43:05Z" | 110,063 | 646 | diffusers | [
"diffusers",
"safetensors",
"text-to-image",
"en",
"arxiv:2401.07519",
"license:apache-2.0",
"region:us"
] | text-to-image | "2024-01-19T11:52:05Z" | ---
license: apache-2.0
language:
- en
library_name: diffusers
pipeline_tag: text-to-image
---
# InstantID Model Card
<div align="center">
[**Project Page**](https://instantid.github.io/) **|** [**Paper**](https://arxiv.org/abs/2401.07519) **|** [**Code**](https://github.com/InstantID/InstantID) **|** [🤗 **Gradio demo**](https://huggingface.co/spaces/InstantX/InstantID)
</div>
## Introduction
InstantID is a new state-of-the-art tuning-free method to achieve ID-Preserving generation with only single image, supporting various downstream tasks.
<div align="center">
<img src='examples/applications.png'>
</div>
## Usage
You can directly download the model in this repository.
You also can download the model in python script:
```python
from huggingface_hub import hf_hub_download
hf_hub_download(repo_id="InstantX/InstantID", filename="ControlNetModel/config.json", local_dir="./checkpoints")
hf_hub_download(repo_id="InstantX/InstantID", filename="ControlNetModel/diffusion_pytorch_model.safetensors", local_dir="./checkpoints")
hf_hub_download(repo_id="InstantX/InstantID", filename="ip-adapter.bin", local_dir="./checkpoints")
```
For face encoder, you need to manutally download via this [URL](https://github.com/deepinsight/insightface/issues/1896#issuecomment-1023867304) to `models/antelopev2`.
```python
# !pip install opencv-python transformers accelerate insightface
import diffusers
from diffusers.utils import load_image
from diffusers.models import ControlNetModel
import cv2
import torch
import numpy as np
from PIL import Image
from insightface.app import FaceAnalysis
from pipeline_stable_diffusion_xl_instantid import StableDiffusionXLInstantIDPipeline, draw_kps
# prepare 'antelopev2' under ./models
app = FaceAnalysis(name='antelopev2', root='./', providers=['CUDAExecutionProvider', 'CPUExecutionProvider'])
app.prepare(ctx_id=0, det_size=(640, 640))
# prepare models under ./checkpoints
face_adapter = f'./checkpoints/ip-adapter.bin'
controlnet_path = f'./checkpoints/ControlNetModel'
# load IdentityNet
controlnet = ControlNetModel.from_pretrained(controlnet_path, torch_dtype=torch.float16)
pipe = StableDiffusionXLInstantIDPipeline.from_pretrained(
... "stabilityai/stable-diffusion-xl-base-1.0", controlnet=controlnet, torch_dtype=torch.float16
... )
pipe.cuda()
# load adapter
pipe.load_ip_adapter_instantid(face_adapter)
```
Then, you can customized your own face images
```python
# load an image
image = load_image("your-example.jpg")
# prepare face emb
face_info = app.get(cv2.cvtColor(np.array(face_image), cv2.COLOR_RGB2BGR))
face_info = sorted(face_info, key=lambda x:(x['bbox'][2]-x['bbox'][0])*x['bbox'][3]-x['bbox'][1])[-1] # only use the maximum face
face_emb = face_info['embedding']
face_kps = draw_kps(face_image, face_info['kps'])
pipe.set_ip_adapter_scale(0.8)
prompt = "analog film photo of a man. faded film, desaturated, 35mm photo, grainy, vignette, vintage, Kodachrome, Lomography, stained, highly detailed, found footage, masterpiece, best quality"
negative_prompt = "(lowres, low quality, worst quality:1.2), (text:1.2), watermark, painting, drawing, illustration, glitch, deformed, mutated, cross-eyed, ugly, disfigured (lowres, low quality, worst quality:1.2), (text:1.2), watermark, painting, drawing, illustration, glitch,deformed, mutated, cross-eyed, ugly, disfigured"
# generate image
image = pipe(
... prompt, image_embeds=face_emb, image=face_kps, controlnet_conditioning_scale=0.8
... ).images[0]
```
For more details, please follow the instructions in our [GitHub repository](https://github.com/InstantID/InstantID).
## Usage Tips
1. If you're not satisfied with the similarity, try to increase the weight of "IdentityNet Strength" and "Adapter Strength".
2. If you feel that the saturation is too high, first decrease the Adapter strength. If it is still too high, then decrease the IdentityNet strength.
3. If you find that text control is not as expected, decrease Adapter strength.
4. If you find that realistic style is not good enough, go for our Github repo and use a more realistic base model.
## Demos
<div align="center">
<img src='examples/0.png'>
</div>
<div align="center">
<img src='examples/1.png'>
</div>
## Disclaimer
This project is released under Apache License and aims to positively impact the field of AI-driven image generation. Users are granted the freedom to create images using this tool, but they are obligated to comply with local laws and utilize it responsibly. The developers will not assume any responsibility for potential misuse by users.
## Citation
```bibtex
@article{wang2024instantid,
title={InstantID: Zero-shot Identity-Preserving Generation in Seconds},
author={Wang, Qixun and Bai, Xu and Wang, Haofan and Qin, Zekui and Chen, Anthony},
journal={arXiv preprint arXiv:2401.07519},
year={2024}
}
``` |
tohoku-nlp/bert-base-japanese-char-v2 | tohoku-nlp | "2021-09-23T13:45:24Z" | 109,635 | 5 | transformers | [
"transformers",
"pytorch",
"tf",
"jax",
"bert",
"fill-mask",
"ja",
"dataset:wikipedia",
"license:cc-by-sa-4.0",
"autotrain_compatible",
"endpoints_compatible",
"region:us"
] | fill-mask | "2022-03-02T23:29:05Z" | ---
language: ja
license: cc-by-sa-4.0
datasets:
- wikipedia
widget:
- text: 東北大学で[MASK]の研究をしています。
---
# BERT base Japanese (character-level tokenization with whole word masking, jawiki-20200831)
This is a [BERT](https://github.com/google-research/bert) model pretrained on texts in the Japanese language.
This version of the model processes input texts with word-level tokenization based on the Unidic 2.1.2 dictionary (available in [unidic-lite](https://pypi.org/project/unidic-lite/) package), followed by character-level tokenization.
Additionally, the model is trained with the whole word masking enabled for the masked language modeling (MLM) objective.
The codes for the pretraining are available at [cl-tohoku/bert-japanese](https://github.com/cl-tohoku/bert-japanese/tree/v2.0).
## Model architecture
The model architecture is the same as the original BERT base model; 12 layers, 768 dimensions of hidden states, and 12 attention heads.
## Training Data
The models are trained on the Japanese version of Wikipedia.
The training corpus is generated from the Wikipedia Cirrussearch dump file as of August 31, 2020.
The generated corpus files are 4.0GB in total, containing approximately 30M sentences.
We used the [MeCab](https://taku910.github.io/mecab/) morphological parser with [mecab-ipadic-NEologd](https://github.com/neologd/mecab-ipadic-neologd) dictionary to split texts into sentences.
## Tokenization
The texts are first tokenized by MeCab with the Unidic 2.1.2 dictionary and then split into characters.
The vocabulary size is 6144.
We used [`fugashi`](https://github.com/polm/fugashi) and [`unidic-lite`](https://github.com/polm/unidic-lite) packages for the tokenization.
## Training
The models are trained with the same configuration as the original BERT; 512 tokens per instance, 256 instances per batch, and 1M training steps.
For training of the MLM (masked language modeling) objective, we introduced whole word masking in which all of the subword tokens corresponding to a single word (tokenized by MeCab) are masked at once.
For training of each model, we used a v3-8 instance of Cloud TPUs provided by [TensorFlow Research Cloud program](https://www.tensorflow.org/tfrc/).
The training took about 5 days to finish.
## Licenses
The pretrained models are distributed under the terms of the [Creative Commons Attribution-ShareAlike 3.0](https://creativecommons.org/licenses/by-sa/3.0/).
## Acknowledgments
This model is trained with Cloud TPUs provided by [TensorFlow Research Cloud](https://www.tensorflow.org/tfrc/) program.
|
Mitsua/mitsua-diffusion-cc0 | Mitsua | "2023-03-03T11:04:16Z" | 109,597 | 60 | diffusers | [
"diffusers",
"stable-diffusion",
"text-to-image",
"stable-diffusion-diffusers",
"license:openrail++",
"autotrain_compatible",
"endpoints_compatible",
"diffusers:StableDiffusionPipeline",
"region:us"
] | text-to-image | "2022-12-21T23:04:27Z" | ---
license: openrail++
tags:
- stable-diffusion
- text-to-image
- stable-diffusion-diffusers
- diffusers
inference: true
---
# .
# .
# .
# .
# .
# .
# ❗❗❗❗❗❗❗❗❗❗❗❗❗❗❗❗❗❗❗❗❗❗❗❗❗❗❗❗❗❗❗❗
# This version is deprecated.
# Please use [Mitsua Diffusion One](https://huggingface.co/Mitsua/mitsua-diffusion-one), which is a successor of this model.
# ❗❗❗❗❗❗❗❗❗❗❗❗❗❗❗❗❗❗❗❗❗❗❗❗❗❗❗❗❗❗❗❗
# .
# .
# .
# .
# .
# Mitsua Diffusion CC0 Model Card
Mitsua Diffusion CC0 is a latent text-to-image diffusion model, whose U-Net is **trained from scratch using only public domain/CC0 or copyright images with permission for use**.
Text Encoder and VAE are borrowed from [Stable Diffusion v2.1 base](https://huggingface.co/stabilityai/stable-diffusion-2-1-base/).
This will be used as a base model for [**AI VTuber Elan Mitsua🖌️**](https://elanmitsua.com/en/)’s activity.
❗❗ **Currently the model has super low visual quality and limited diversity** ❗❗
Yes, the visual quality is not so good. Most of modern artistic concept is lost completely. However, since she is a growing AI in an ethical fashion, it would be good starting point for Mitsua-chan!
You can join [her training on Twitter](https://twitter.com/elanmitsua)! Please support Mitsua-chan!🎉
Further training will be done in a fully opt-in basis. If you are interested in, [please click here to submit an opt-in application](https://forms.gle/Nk3M7UyqSgYAqdpA6).
We are active on [a Discord server for opt-in participants only](https://discord.com/invite/7VTGRweTUg). Communication is currently in Japanese.
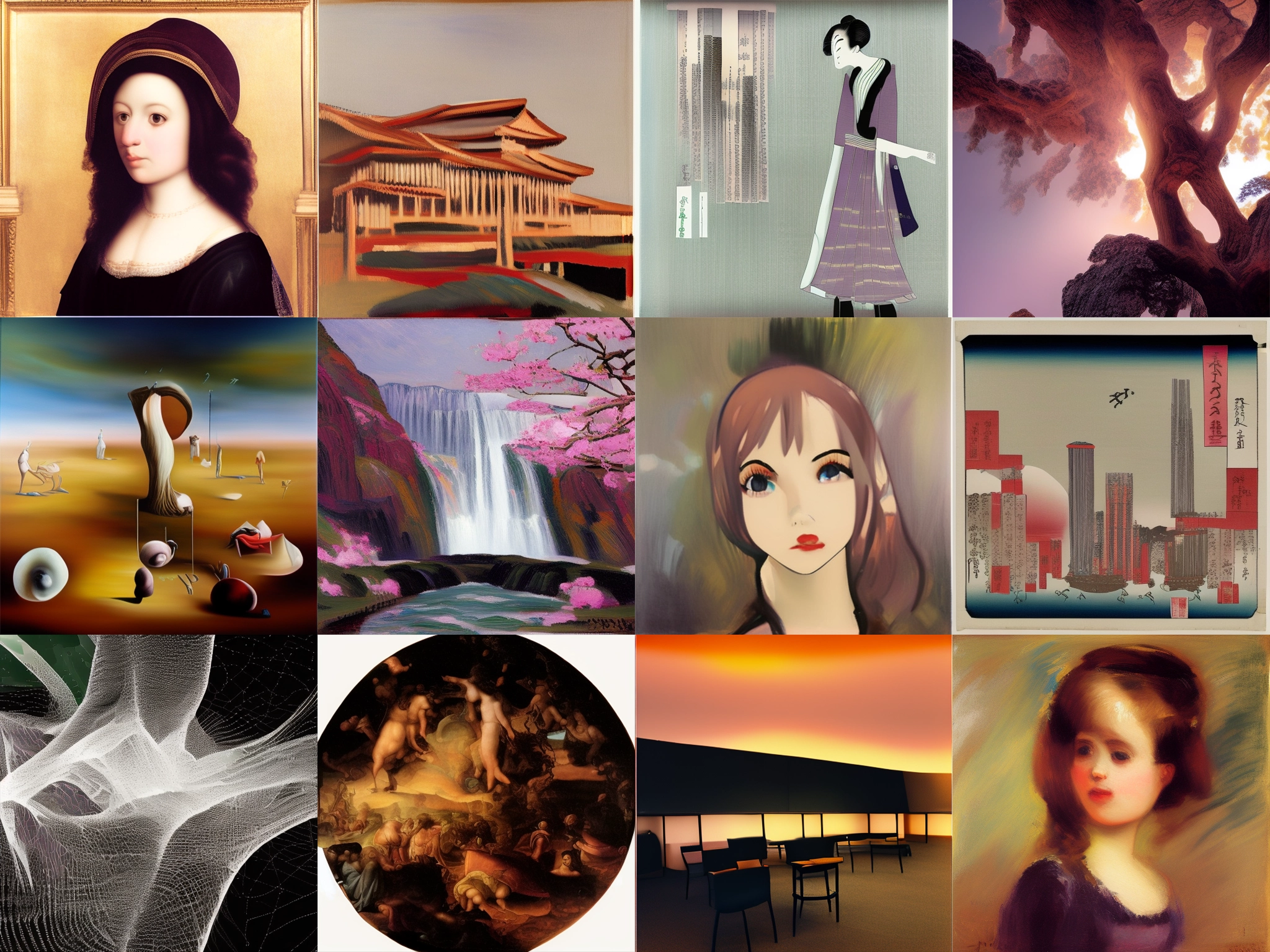
You can check [here to all prompts to generate these images](https://huggingface.co/Mitsua/mitsua-diffusion-cc0/resolve/main/images/mitsua_cc0_works_prompts.csv).
## Training Data Sources
All data was obtained ethically and in compliance with the site's terms and conditions.
No copyright images are used in the training of this model without the permission.
No AI generated images are in the dataset.
- Traditional Artwork in public domain / CC0
- MET Museum Open Access
- Smithsonian Open Access
- Cleveland Museum of Art Open Access
- National Gallery of Art Open Access
- ArtBench-10 (public domain subset)
- CC0 Photos
- Flickr, Wikimedia Commons
- CC0 NFTs *1
- goblintown.nft, mfer, tubby-cats, Timeless
- CC0 VRM models
- made by VRoid Project, pastelkies, yomox9 (all CC0 subset)
- We generated a bunch of synthesized images dataset rendered with various poses and camera angles.
- Copyright images with permission for use
- Generative and Visual Artworks made by Rhizomatiks
Approx 11M images in total with data augmentation.
1. Their work is released under a CC0 license, but if you are considering using this model to create a work inspired by their NFT and sell it as NFT, please consider paying them a royalty to help the CC0 NFT community grow.
## License
[Creative Open-Rail++-M License](https://huggingface.co/stabilityai/stable-diffusion-2/blob/main/LICENSE-MODEL)
❗❗ “Mitsua Diffusion CC0” means most of the training data is CC0. **the model license itself is NOT CC0**.❗❗
This model is open access and available to all, with a CreativeML OpenRAIL++-M license further specifying rights and usage. The CreativeML OpenRAIL++-M License specifies:
1. You can't use the model to deliberately produce nor share illegal or harmful outputs or content
2. The authors claims no rights on the outputs you generate, you are free to use them and are accountable for their use which must not go against the provisions set in the license
3. You may re-distribute the weights and use the model commercially and/or as a service. If you do, please be aware you have to include the same use restrictions as the ones in the license and share a copy of the CreativeML OpenRAIL++-M to all your users (please read the license entirely and carefully) [Please read the full license here](https://huggingface.co/stabilityai/stable-diffusion-2/blob/main/LICENSE-MODEL)
## Developed by
- Stable Diffusion 2.1: Robin Rombach, Patrick Esser
- Mitsua Diffusion CC0 : Abstract Engine dev team
|
RichardErkhov/NousResearch_-_Llama-2-70b-chat-hf-gguf | RichardErkhov | "2024-07-01T03:39:04Z" | 109,588 | 0 | null | [
"gguf",
"region:us"
] | null | "2024-06-29T23:58:43Z" | Quantization made by Richard Erkhov.
[Github](https://github.com/RichardErkhov)
[Discord](https://discord.gg/pvy7H8DZMG)
[Request more models](https://github.com/RichardErkhov/quant_request)
Llama-2-70b-chat-hf - GGUF
- Model creator: https://huggingface.co/NousResearch/
- Original model: https://huggingface.co/NousResearch/Llama-2-70b-chat-hf/
| Name | Quant method | Size |
| ---- | ---- | ---- |
| [Llama-2-70b-chat-hf.Q2_K.gguf](https://huggingface.co/RichardErkhov/NousResearch_-_Llama-2-70b-chat-hf-gguf/blob/main/Llama-2-70b-chat-hf.Q2_K.gguf) | Q2_K | 23.71GB |
| [Llama-2-70b-chat-hf.IQ3_XS.gguf](https://huggingface.co/RichardErkhov/NousResearch_-_Llama-2-70b-chat-hf-gguf/blob/main/Llama-2-70b-chat-hf.IQ3_XS.gguf) | IQ3_XS | 26.37GB |
| [Llama-2-70b-chat-hf.IQ3_S.gguf](https://huggingface.co/RichardErkhov/NousResearch_-_Llama-2-70b-chat-hf-gguf/blob/main/Llama-2-70b-chat-hf.IQ3_S.gguf) | IQ3_S | 27.86GB |
| [Llama-2-70b-chat-hf.Q3_K_S.gguf](https://huggingface.co/RichardErkhov/NousResearch_-_Llama-2-70b-chat-hf-gguf/blob/main/Llama-2-70b-chat-hf.Q3_K_S.gguf) | Q3_K_S | 27.86GB |
| [Llama-2-70b-chat-hf.IQ3_M.gguf](https://huggingface.co/RichardErkhov/NousResearch_-_Llama-2-70b-chat-hf-gguf/blob/main/Llama-2-70b-chat-hf.IQ3_M.gguf) | IQ3_M | 28.82GB |
| [Llama-2-70b-chat-hf.Q3_K.gguf](https://huggingface.co/RichardErkhov/NousResearch_-_Llama-2-70b-chat-hf-gguf/blob/main/Llama-2-70b-chat-hf.Q3_K.gguf) | Q3_K | 30.99GB |
| [Llama-2-70b-chat-hf.Q3_K_M.gguf](https://huggingface.co/RichardErkhov/NousResearch_-_Llama-2-70b-chat-hf-gguf/blob/main/Llama-2-70b-chat-hf.Q3_K_M.gguf) | Q3_K_M | 30.99GB |
| [Llama-2-70b-chat-hf.Q3_K_L.gguf](https://huggingface.co/RichardErkhov/NousResearch_-_Llama-2-70b-chat-hf-gguf/blob/main/Llama-2-70b-chat-hf.Q3_K_L.gguf) | Q3_K_L | 33.67GB |
| [Llama-2-70b-chat-hf.IQ4_XS.gguf](https://huggingface.co/RichardErkhov/NousResearch_-_Llama-2-70b-chat-hf-gguf/blob/main/Llama-2-70b-chat-hf.IQ4_XS.gguf) | IQ4_XS | 34.64GB |
| [Llama-2-70b-chat-hf.Q4_0.gguf](https://huggingface.co/RichardErkhov/NousResearch_-_Llama-2-70b-chat-hf-gguf/blob/main/Llama-2-70b-chat-hf.Q4_0.gguf) | Q4_0 | 36.2GB |
| [Llama-2-70b-chat-hf.IQ4_NL.gguf](https://huggingface.co/RichardErkhov/NousResearch_-_Llama-2-70b-chat-hf-gguf/blob/main/Llama-2-70b-chat-hf.IQ4_NL.gguf) | IQ4_NL | 36.55GB |
| [Llama-2-70b-chat-hf.Q4_K_S.gguf](https://huggingface.co/RichardErkhov/NousResearch_-_Llama-2-70b-chat-hf-gguf/blob/main/Llama-2-70b-chat-hf.Q4_K_S.gguf) | Q4_K_S | 36.55GB |
| [Llama-2-70b-chat-hf.Q4_K.gguf](https://huggingface.co/RichardErkhov/NousResearch_-_Llama-2-70b-chat-hf-gguf/tree/main/) | Q4_K | 38.58GB |
| [Llama-2-70b-chat-hf.Q4_K_M.gguf](https://huggingface.co/RichardErkhov/NousResearch_-_Llama-2-70b-chat-hf-gguf/tree/main/) | Q4_K_M | 38.58GB |
| [Llama-2-70b-chat-hf.Q4_1.gguf](https://huggingface.co/RichardErkhov/NousResearch_-_Llama-2-70b-chat-hf-gguf/tree/main/) | Q4_1 | 40.2GB |
| [Llama-2-70b-chat-hf.Q5_0.gguf](https://huggingface.co/RichardErkhov/NousResearch_-_Llama-2-70b-chat-hf-gguf/tree/main/) | Q5_0 | 44.2GB |
| [Llama-2-70b-chat-hf.Q5_K_S.gguf](https://huggingface.co/RichardErkhov/NousResearch_-_Llama-2-70b-chat-hf-gguf/tree/main/) | Q5_K_S | 44.2GB |
| [Llama-2-70b-chat-hf.Q5_K.gguf](https://huggingface.co/RichardErkhov/NousResearch_-_Llama-2-70b-chat-hf-gguf/tree/main/) | Q5_K | 45.41GB |
| [Llama-2-70b-chat-hf.Q5_K_M.gguf](https://huggingface.co/RichardErkhov/NousResearch_-_Llama-2-70b-chat-hf-gguf/tree/main/) | Q5_K_M | 45.41GB |
| [Llama-2-70b-chat-hf.Q5_1.gguf](https://huggingface.co/RichardErkhov/NousResearch_-_Llama-2-70b-chat-hf-gguf/tree/main/) | Q5_1 | 48.2GB |
| [Llama-2-70b-chat-hf.Q6_K.gguf](https://huggingface.co/RichardErkhov/NousResearch_-_Llama-2-70b-chat-hf-gguf/tree/main/) | Q6_K | 52.7GB |
| [Llama-2-70b-chat-hf.Q8_0.gguf](https://huggingface.co/RichardErkhov/NousResearch_-_Llama-2-70b-chat-hf-gguf/tree/main/) | Q8_0 | 68.26GB |
Original model description:
---
extra_gated_heading: Access Llama 2 on Hugging Face
extra_gated_description: >-
This is a form to enable access to Llama 2 on Hugging Face after you have been
granted access from Meta. Please visit the [Meta website](https://ai.meta.com/resources/models-and-libraries/llama-downloads) and accept our
license terms and acceptable use policy before submitting this form. Requests
will be processed in 1-2 days.
extra_gated_prompt: "**Your Hugging Face account email address MUST match the email you provide on the Meta website, or your request will not be approved.**"
extra_gated_button_content: Submit
extra_gated_fields:
I agree to share my name, email address and username with Meta and confirm that I have already been granted download access on the Meta website: checkbox
language:
- en
pipeline_tag: text-generation
inference: false
tags:
- facebook
- meta
- pytorch
- llama
- llama-2
---
# **Llama 2**
Llama 2 is a collection of pretrained and fine-tuned generative text models ranging in scale from 7 billion to 70 billion parameters. This is the repository for the 70B fine-tuned model, optimized for dialogue use cases and converted for the Hugging Face Transformers format. Links to other models can be found in the index at the bottom.
## Model Details
*Note: Use of this model is governed by the Meta license. In order to download the model weights and tokenizer, please visit the [website](https://ai.meta.com/resources/models-and-libraries/llama-downloads/) and accept our License before requesting access here.*
Meta developed and publicly released the Llama 2 family of large language models (LLMs), a collection of pretrained and fine-tuned generative text models ranging in scale from 7 billion to 70 billion parameters. Our fine-tuned LLMs, called Llama-2-Chat, are optimized for dialogue use cases. Llama-2-Chat models outperform open-source chat models on most benchmarks we tested, and in our human evaluations for helpfulness and safety, are on par with some popular closed-source models like ChatGPT and PaLM.
**Model Developers** Meta
**Variations** Llama 2 comes in a range of parameter sizes — 7B, 13B, and 70B — as well as pretrained and fine-tuned variations.
**Input** Models input text only.
**Output** Models generate text only.
**Model Architecture** Llama 2 is an auto-regressive language model that uses an optimized transformer architecture. The tuned versions use supervised fine-tuning (SFT) and reinforcement learning with human feedback (RLHF) to align to human preferences for helpfulness and safety.
||Training Data|Params|Content Length|GQA|Tokens|LR|
|---|---|---|---|---|---|---|
|Llama 2|*A new mix of publicly available online data*|7B|4k|✗|2.0T|3.0 x 10<sup>-4</sup>|
|Llama 2|*A new mix of publicly available online data*|13B|4k|✗|2.0T|3.0 x 10<sup>-4</sup>|
|Llama 2|*A new mix of publicly available online data*|70B|4k|✔|2.0T|1.5 x 10<sup>-4</sup>|
*Llama 2 family of models.* Token counts refer to pretraining data only. All models are trained with a global batch-size of 4M tokens. Bigger models - 70B -- use Grouped-Query Attention (GQA) for improved inference scalability.
**Model Dates** Llama 2 was trained between January 2023 and July 2023.
**Status** This is a static model trained on an offline dataset. Future versions of the tuned models will be released as we improve model safety with community feedback.
**License** A custom commercial license is available at: [https://ai.meta.com/resources/models-and-libraries/llama-downloads/](https://ai.meta.com/resources/models-and-libraries/llama-downloads/)
## Intended Use
**Intended Use Cases** Llama 2 is intended for commercial and research use in English. Tuned models are intended for assistant-like chat, whereas pretrained models can be adapted for a variety of natural language generation tasks.
**Out-of-scope Uses** Use in any manner that violates applicable laws or regulations (including trade compliance laws).Use in languages other than English. Use in any other way that is prohibited by the Acceptable Use Policy and Licensing Agreement for Llama 2.
## Hardware and Software
**Training Factors** We used custom training libraries, Meta's Research Super Cluster, and production clusters for pretraining. Fine-tuning, annotation, and evaluation were also performed on third-party cloud compute.
**Carbon Footprint** Pretraining utilized a cumulative 3.3M GPU hours of computation on hardware of type A100-80GB (TDP of 350-400W). Estimated total emissions were 539 tCO2eq, 100% of which were offset by Meta’s sustainability program.
||Time (GPU hours)|Power Consumption (W)|Carbon Emitted(tCO<sub>2</sub>eq)|
|---|---|---|---|
|Llama 2 7B|184320|400|31.22|
|Llama 2 13B|368640|400|62.44|
|Llama 2 70B|1720320|400|291.42|
|Total|3311616||539.00|
**CO<sub>2</sub> emissions during pretraining.** Time: total GPU time required for training each model. Power Consumption: peak power capacity per GPU device for the GPUs used adjusted for power usage efficiency. 100% of the emissions are directly offset by Meta's sustainability program, and because we are openly releasing these models, the pretraining costs do not need to be incurred by others.
## Training Data
**Overview** Llama 2 was pretrained on 2 trillion tokens of data from publicly available sources. The fine-tuning data includes publicly available instruction datasets, as well as over one million new human-annotated examples. Neither the pretraining nor the fine-tuning datasets include Meta user data.
**Data Freshness** The pretraining data has a cutoff of September 2022, but some tuning data is more recent, up to July 2023.
## Evaluation Results
In this section, we report the results for the Llama 1 and Llama 2 models on standard academic benchmarks.For all the evaluations, we use our internal evaluations library.
|Model|Size|Code|Commonsense Reasoning|World Knowledge|Reading Comprehension|Math|MMLU|BBH|AGI Eval|
|---|---|---|---|---|---|---|---|---|---|
|Llama 1|7B|14.1|60.8|46.2|58.5|6.95|35.1|30.3|23.9|
|Llama 1|13B|18.9|66.1|52.6|62.3|10.9|46.9|37.0|33.9|
|Llama 1|33B|26.0|70.0|58.4|67.6|21.4|57.8|39.8|41.7|
|Llama 1|65B|30.7|70.7|60.5|68.6|30.8|63.4|43.5|47.6|
|Llama 2|7B|16.8|63.9|48.9|61.3|14.6|45.3|32.6|29.3|
|Llama 2|13B|24.5|66.9|55.4|65.8|28.7|54.8|39.4|39.1|
|Llama 2|70B|**37.5**|**71.9**|**63.6**|**69.4**|**35.2**|**68.9**|**51.2**|**54.2**|
**Overall performance on grouped academic benchmarks.** *Code:* We report the average pass@1 scores of our models on HumanEval and MBPP. *Commonsense Reasoning:* We report the average of PIQA, SIQA, HellaSwag, WinoGrande, ARC easy and challenge, OpenBookQA, and CommonsenseQA. We report 7-shot results for CommonSenseQA and 0-shot results for all other benchmarks. *World Knowledge:* We evaluate the 5-shot performance on NaturalQuestions and TriviaQA and report the average. *Reading Comprehension:* For reading comprehension, we report the 0-shot average on SQuAD, QuAC, and BoolQ. *MATH:* We report the average of the GSM8K (8 shot) and MATH (4 shot) benchmarks at top 1.
|||TruthfulQA|Toxigen|
|---|---|---|---|
|Llama 1|7B|27.42|23.00|
|Llama 1|13B|41.74|23.08|
|Llama 1|33B|44.19|22.57|
|Llama 1|65B|48.71|21.77|
|Llama 2|7B|33.29|**21.25**|
|Llama 2|13B|41.86|26.10|
|Llama 2|70B|**50.18**|24.60|
**Evaluation of pretrained LLMs on automatic safety benchmarks.** For TruthfulQA, we present the percentage of generations that are both truthful and informative (the higher the better). For ToxiGen, we present the percentage of toxic generations (the smaller the better).
|||TruthfulQA|Toxigen|
|---|---|---|---|
|Llama-2-Chat|7B|57.04|**0.00**|
|Llama-2-Chat|13B|62.18|**0.00**|
|Llama-2-Chat|70B|**64.14**|0.01|
**Evaluation of fine-tuned LLMs on different safety datasets.** Same metric definitions as above.
## Ethical Considerations and Limitations
Llama 2 is a new technology that carries risks with use. Testing conducted to date has been in English, and has not covered, nor could it cover all scenarios. For these reasons, as with all LLMs, Llama 2’s potential outputs cannot be predicted in advance, and the model may in some instances produce inaccurate, biased or other objectionable responses to user prompts. Therefore, before deploying any applications of Llama 2, developers should perform safety testing and tuning tailored to their specific applications of the model.
Please see the Responsible Use Guide available at [https://ai.meta.com/llama/responsible-use-guide/](https://ai.meta.com/llama/responsible-use-guide)
## Reporting Issues
Please report any software “bug,” or other problems with the models through one of the following means:
- Reporting issues with the model: [github.com/facebookresearch/llama](http://github.com/facebookresearch/llama)
- Reporting problematic content generated by the model: [developers.facebook.com/llama_output_feedback](http://developers.facebook.com/llama_output_feedback)
- Reporting bugs and security concerns: [facebook.com/whitehat/info](http://facebook.com/whitehat/info)
## Llama Model Index
|Model|Llama2|Llama2-hf|Llama2-chat|Llama2-chat-hf|
|---|---|---|---|---|
|7B| [Link](https://huggingface.co/llamaste/Llama-2-7b) | [Link](https://huggingface.co/llamaste/Llama-2-7b-hf) | [Link](https://huggingface.co/llamaste/Llama-2-7b-chat) | [Link](https://huggingface.co/llamaste/Llama-2-7b-chat-hf)|
|13B| [Link](https://huggingface.co/llamaste/Llama-2-13b) | [Link](https://huggingface.co/llamaste/Llama-2-13b-hf) | [Link](https://huggingface.co/llamaste/Llama-2-13b-chat) | [Link](https://huggingface.co/llamaste/Llama-2-13b-hf)|
|70B| [Link](https://huggingface.co/llamaste/Llama-2-70b) | [Link](https://huggingface.co/llamaste/Llama-2-70b-hf) | [Link](https://huggingface.co/llamaste/Llama-2-70b-chat) | [Link](https://huggingface.co/llamaste/Llama-2-70b-hf)|
|
vinai/xphonebert-base | vinai | "2023-08-29T04:01:53Z" | 109,451 | 7 | transformers | [
"transformers",
"pytorch",
"roberta",
"fill-mask",
"autotrain_compatible",
"endpoints_compatible",
"region:us"
] | fill-mask | "2023-04-13T15:46:03Z" | # <a name="introduction"></a> XPhoneBERT : A Pre-trained Multilingual Model for Phoneme Representations for Text-to-Speech
XPhoneBERT is the first pre-trained multilingual model for phoneme representations for text-to-speech(TTS). XPhoneBERT has the same model architecture as BERT-base, trained using the RoBERTa pre-training approach on 330M phoneme-level sentences from nearly 100 languages and locales. Experimental results show that employing XPhoneBERT as an input phoneme encoder significantly boosts the performance of a strong neural TTS model in terms of naturalness and prosody and also helps produce fairly high-quality speech with limited training data.
The general architecture and experimental results of XPhoneBERT can be found in [our INTERSPEECH 2023 paper](https://www.doi.org/10.21437/Interspeech.2023-444):
@inproceedings{xphonebert,
title = {{XPhoneBERT: A Pre-trained Multilingual Model for Phoneme Representations for Text-to-Speech}},
author = {Linh The Nguyen and Thinh Pham and Dat Quoc Nguyen},
booktitle = {Proceedings of the 24th Annual Conference of the International Speech Communication Association (INTERSPEECH)},
year = {2023},
pages = {5506--5510}
}
**Please CITE** our paper when XPhoneBERT is used to help produce published results or is incorporated into other software.
For further information or requests, please go to [XPhoneBERT's homepage](https://github.com/VinAIResearch/XPhoneBERT)!
## <a name="transformers"></a> Using XPhoneBERT with `transformers`
### Installation <a name="install2"></a>
- Install `transformers` with pip: `pip install transformers`, or install `transformers` [from source](https://huggingface.co/docs/transformers/installation#installing-from-source).
- Install `text2phonemesequence`: `pip install text2phonemesequence` <br> Our [`text2phonemesequence`](https://github.com/thelinhbkhn2014/Text2PhonemeSequence) package is to convert text sequences into phoneme-level sequences, employed to construct our multilingual phoneme-level pre-training data. We build `text2phonemesequence` by incorporating the [CharsiuG2P](https://github.com/lingjzhu/CharsiuG2P/tree/main) and the [segments](https://pypi.org/project/segments/) toolkits that perform text-to-phoneme conversion and phoneme segmentation, respectively.
- **Notes**
- Initializing `text2phonemesequence` for each language requires its corresponding ISO 639-3 code. The ISO 639-3 codes of supported languages are available at [HERE](https://github.com/VinAIResearch/XPhoneBERT/blob/main/LanguageISO639-3Codes.md).
- `text2phonemesequence` takes a word-segmented sequence as input. And users might also perform text normalization on the word-segmented sequence before feeding into `text2phonemesequence`. When creating our pre-training data, we perform word and sentence segmentation on all text documents in each language by using the [spaCy](https://spacy.io) toolkit, except for Vietnamese where we employ the [VnCoreNLP](https://github.com/vncorenlp/VnCoreNLP) toolkit. We also use the text normalization component from the [NVIDIA NeMo toolkit](https://github.com/NVIDIA/NeMo) for English, German, Spanish and Chinese, and the [Vinorm](https://github.com/v-nhandt21/Vinorm) text normalization package for Vietnamese.
### <a name="models2"></a> Pre-trained model
Model | #params | Arch. | Max length | Pre-training data
---|---|---|---|---
`vinai/xphonebert-base` | 88M | base | 512 | 330M phoneme-level sentences from nearly 100 languages and locales
### Example usage <a name="usage2"></a>
```python
from transformers import AutoModel, AutoTokenizer
from text2phonemesequence import Text2PhonemeSequence
# Load XPhoneBERT model and its tokenizer
xphonebert = AutoModel.from_pretrained("vinai/xphonebert-base")
tokenizer = AutoTokenizer.from_pretrained("vinai/xphonebert-base")
# Load Text2PhonemeSequence
# text2phone_model = Text2PhonemeSequence(language='eng-us', is_cuda=True)
text2phone_model = Text2PhonemeSequence(language='jpn', is_cuda=True)
# Input sequence that is already WORD-SEGMENTED (and text-normalized if applicable)
# sentence = "That is , it is a testing text ."
sentence = "これ は 、 テスト テキスト です ."
input_phonemes = text2phone_model.infer_sentence(sentence)
input_ids = tokenizer(input_phonemes, return_tensors="pt")
with torch.no_grad():
features = xphonebert(**input_ids)
```
|
benjamin/wtp-canine-s-12l | benjamin | "2023-12-02T11:42:49Z" | 109,400 | 4 | transformers | [
"transformers",
"pytorch",
"la-canine",
"token-classification",
"multilingual",
"am",
"ar",
"az",
"be",
"bg",
"bn",
"ca",
"ceb",
"cs",
"cy",
"da",
"de",
"el",
"en",
"eo",
"es",
"et",
"eu",
"fa",
"fi",
"fr",
"fy",
"ga",
"gd",
"gl",
"gu",
"ha",
"he",
"hi",
"hu",
"hy",
"id",
"ig",
"is",
"it",
"ja",
"jv",
"ka",
"kk",
"km",
"kn",
"ko",
"ku",
"ky",
"la",
"lt",
"lv",
"mg",
"mk",
"ml",
"mn",
"mr",
"ms",
"mt",
"my",
"ne",
"nl",
"no",
"pa",
"pl",
"ps",
"pt",
"ro",
"ru",
"si",
"sk",
"sl",
"sq",
"sr",
"sv",
"ta",
"te",
"tg",
"th",
"tr",
"uk",
"ur",
"uz",
"vi",
"xh",
"yi",
"yo",
"zh",
"zu",
"license:mit",
"autotrain_compatible",
"endpoints_compatible",
"region:us"
] | token-classification | "2023-05-10T20:50:38Z" | ---
license: mit
language:
- multilingual
- am
- ar
- az
- be
- bg
- bn
- ca
- ceb
- cs
- cy
- da
- de
- el
- en
- eo
- es
- et
- eu
- fa
- fi
- fr
- fy
- ga
- gd
- gl
- gu
- ha
- he
- hi
- hu
- hy
- id
- ig
- is
- it
- ja
- jv
- ka
- kk
- km
- kn
- ko
- ku
- ky
- la
- lt
- lv
- mg
- mk
- ml
- mn
- mr
- ms
- mt
- my
- ne
- nl
- no
- pa
- pl
- ps
- pt
- ro
- ru
- si
- sk
- sl
- sq
- sr
- sv
- ta
- te
- tg
- th
- tr
- uk
- ur
- uz
- vi
- xh
- yi
- yo
- zh
- zu
---
# wtp-canine-s-12l
Model for [`wtpsplit`](https://github.com/bminixhofer/wtpsplit). |
Maykeye/TinyLLama-v0 | Maykeye | "2023-07-26T05:04:57Z" | 108,960 | 21 | transformers | [
"transformers",
"pytorch",
"safetensors",
"llama",
"text-generation",
"license:apache-2.0",
"autotrain_compatible",
"endpoints_compatible",
"text-generation-inference",
"region:us"
] | text-generation | "2023-07-08T04:50:15Z" | ---
license: apache-2.0
---
This is a first version of recreating roneneldan/TinyStories-1M but using Llama architecture.
* Full training process is included in the notebook train.ipynb. Recreating it as simple as downloading
TinyStoriesV2-GPT4-train.txt and TinyStoriesV2-GPT4-valid.txt in the same folder with the notebook and running
the cells. Validation content is not used by the script so you put anythin in
* Backup directory has a script do_backup that I used to copy weights from remote machine to local.
Weight are generated too quickly, so by the time script copied weihgt N+1
* This is extremely PoC version. Training truncates stories that are longer than context size and doesn't use
any sliding window to train story not from the start
* Training took approximately 9 hours (3 hours per epoch) on 40GB A100. ~30GB VRAM was used
* I use tokenizer from open_llama_3b. However I had troubles with it locally(https://github.com/openlm-research/open_llama/issues/69).
I had no troubles on the cloud machine with preninstalled libraries.
* Demo script is demo.py
* Validation script is provided: valid.py. use it like `python valid.py path/to/TinyStoriesV2-GPT4-valid.txt [optional-model-id-or-path]`:
After training I decided that it's not necessary to beat validation into chunks
* Also this version uses very stupid caching mechinsm to shuffle stories for training: it keeps cache of N recently loaded chunks
so if random shuffle asks for a story, it may use cache or load chunk.
Training dataset is too small, so in next versions I will get rid of it.
from transformers import AutoModelForCausalLM, AutoTokenizer
|
bartowski/dolphin-2.9.3-Yi-1.5-34B-32k-GGUF | bartowski | "2024-06-24T09:47:10Z" | 108,926 | 9 | null | [
"gguf",
"generated_from_trainer",
"axolotl",
"text-generation",
"dataset:cognitivecomputations/Dolphin-2.9",
"dataset:teknium/OpenHermes-2.5",
"dataset:m-a-p/CodeFeedback-Filtered-Instruction",
"dataset:cognitivecomputations/dolphin-coder",
"dataset:cognitivecomputations/samantha-data",
"dataset:microsoft/orca-math-word-problems-200k",
"dataset:Locutusque/function-calling-chatml",
"dataset:internlm/Agent-FLAN",
"base_model:01-ai/Yi-1.5-34B-32k",
"license:apache-2.0",
"region:us"
] | text-generation | "2024-06-24T07:03:50Z" | ---
license: apache-2.0
base_model: 01-ai/Yi-1.5-34B-32k
tags:
- generated_from_trainer
- axolotl
datasets:
- cognitivecomputations/Dolphin-2.9
- teknium/OpenHermes-2.5
- m-a-p/CodeFeedback-Filtered-Instruction
- cognitivecomputations/dolphin-coder
- cognitivecomputations/samantha-data
- microsoft/orca-math-word-problems-200k
- Locutusque/function-calling-chatml
- internlm/Agent-FLAN
quantized_by: bartowski
pipeline_tag: text-generation
---
## Llamacpp imatrix Quantizations of dolphin-2.9.3-Yi-1.5-34B-32k
Using <a href="https://github.com/ggerganov/llama.cpp/">llama.cpp</a> release <a href="https://github.com/ggerganov/llama.cpp/releases/tag/b3197">b3197</a> for quantization.
Original model: https://huggingface.co/cognitivecomputations/dolphin-2.9.3-Yi-1.5-34B-32k
All quants made using imatrix option with dataset from [here](https://gist.github.com/bartowski1182/eb213dccb3571f863da82e99418f81e8)
## Prompt format
```
<|im_start|> system
{system_prompt}<|im_end|>
<|im_start|> user
{prompt}<|im_end|>
<|im_start|> assistant
```
## Download a file (not the whole branch) from below:
| Filename | Quant type | File Size | Description |
| -------- | ---------- | --------- | ----------- |
| [dolphin-2.9.3-Yi-1.5-34B-32k-Q8_0_L.gguf](https://huggingface.co/bartowski/dolphin-2.9.3-Yi-1.5-34B-32k-GGUF/blob/main/dolphin-2.9.3-Yi-1.5-34B-32k-Q8_1.gguf) | Q8_0_L | 37.40GB | *Experimental*, uses f16 for embed and output weights. Please provide any feedback of differences. Extremely high quality, generally unneeded but max available quant. |
| [dolphin-2.9.3-Yi-1.5-34B-32k-Q8_0.gguf](https://huggingface.co/bartowski/dolphin-2.9.3-Yi-1.5-34B-32k-GGUF/blob/main/dolphin-2.9.3-Yi-1.5-34B-32k-Q8_0.gguf) | Q8_0 | 36.54GB | Extremely high quality, generally unneeded but max available quant. |
| [dolphin-2.9.3-Yi-1.5-34B-32k-Q6_K_L.gguf](https://huggingface.co/bartowski/dolphin-2.9.3-Yi-1.5-34B-32k-GGUF/blob/main/dolphin-2.9.3-Yi-1.5-34B-32k-Q6_K_L.gguf) | Q6_K_L | 29.29GB | *Experimental*, uses f16 for embed and output weights. Please provide any feedback of differences. Very high quality, near perfect, *recommended*. |
| [dolphin-2.9.3-Yi-1.5-34B-32k-Q6_K.gguf](https://huggingface.co/bartowski/dolphin-2.9.3-Yi-1.5-34B-32k-GGUF/blob/main/dolphin-2.9.3-Yi-1.5-34B-32k-Q6_K.gguf) | Q6_K | 28.21GB | Very high quality, near perfect, *recommended*. |
| [dolphin-2.9.3-Yi-1.5-34B-32k-Q5_K_L.gguf](https://huggingface.co/bartowski/dolphin-2.9.3-Yi-1.5-34B-32k-GGUF/blob/main/dolphin-2.9.3-Yi-1.5-34B-32k-Q5_K_L.gguf) | Q5_K_L | 25.46GB | *Experimental*, uses f16 for embed and output weights. Please provide any feedback of differences. High quality, *recommended*. |
| [dolphin-2.9.3-Yi-1.5-34B-32k-Q5_K_M.gguf](https://huggingface.co/bartowski/dolphin-2.9.3-Yi-1.5-34B-32k-GGUF/blob/main/dolphin-2.9.3-Yi-1.5-34B-32k-Q5_K_M.gguf) | Q5_K_M | 24.32GB | High quality, *recommended*. |
| [dolphin-2.9.3-Yi-1.5-34B-32k-Q5_K_S.gguf](https://huggingface.co/bartowski/dolphin-2.9.3-Yi-1.5-34B-32k-GGUF/blob/main/dolphin-2.9.3-Yi-1.5-34B-32k-Q5_K_S.gguf) | Q5_K_S | 23.70GB | High quality, *recommended*. |
| [dolphin-2.9.3-Yi-1.5-34B-32k-Q4_K_L.gguf](https://huggingface.co/bartowski/dolphin-2.9.3-Yi-1.5-34B-32k-GGUF/blob/main/dolphin-2.9.3-Yi-1.5-34B-32k-Q4_K_L.gguf) | Q4_K_L | 21.85GB | *Experimental*, uses f16 for embed and output weights. Please provide any feedback of differences. Good quality, uses about 4.83 bits per weight, *recommended*. |
| [dolphin-2.9.3-Yi-1.5-34B-32k-Q4_K_M.gguf](https://huggingface.co/bartowski/dolphin-2.9.3-Yi-1.5-34B-32k-GGUF/blob/main/dolphin-2.9.3-Yi-1.5-34B-32k-Q4_K_M.gguf) | Q4_K_M | 20.65GB | Good quality, uses about 4.83 bits per weight, *recommended*. |
| [dolphin-2.9.3-Yi-1.5-34B-32k-Q4_K_S.gguf](https://huggingface.co/bartowski/dolphin-2.9.3-Yi-1.5-34B-32k-GGUF/blob/main/dolphin-2.9.3-Yi-1.5-34B-32k-Q4_K_S.gguf) | Q4_K_S | 19.59GB | Slightly lower quality with more space savings, *recommended*. |
| [dolphin-2.9.3-Yi-1.5-34B-32k-IQ4_XS.gguf](https://huggingface.co/bartowski/dolphin-2.9.3-Yi-1.5-34B-32k-GGUF/blob/main/dolphin-2.9.3-Yi-1.5-34B-32k-IQ4_XS.gguf) | IQ4_XS | 18.47GB | Decent quality, smaller than Q4_K_S with similar performance, *recommended*. |
| [dolphin-2.9.3-Yi-1.5-34B-32k-Q3_K_XL.gguf](https://huggingface.co/bartowski/dolphin-2.9.3-Yi-1.5-34B-32k-GGUF//main/dolphin-2.9.3-Yi-1.5-34B-32k-Q3_K_XL.gguf) | Q3_K_XL | | *Experimental*, uses f16 for embed and output weights. Please provide any feedback of differences. Lower quality but usable, good for low RAM availability. |
| [dolphin-2.9.3-Yi-1.5-34B-32k-Q3_K_L.gguf](https://huggingface.co/bartowski/dolphin-2.9.3-Yi-1.5-34B-32k-GGUF/blob/main/dolphin-2.9.3-Yi-1.5-34B-32k-Q3_K_L.gguf) | Q3_K_L | 18.13GB | Lower quality but usable, good for low RAM availability. |
| [dolphin-2.9.3-Yi-1.5-34B-32k-Q3_K_M.gguf](https://huggingface.co/bartowski/dolphin-2.9.3-Yi-1.5-34B-32k-GGUF/blob/main/dolphin-2.9.3-Yi-1.5-34B-32k-Q3_K_M.gguf) | Q3_K_M | 16.65GB | Even lower quality. |
| [dolphin-2.9.3-Yi-1.5-34B-32k-IQ3_M.gguf](https://huggingface.co/bartowski/dolphin-2.9.3-Yi-1.5-34B-32k-GGUF/blob/main/dolphin-2.9.3-Yi-1.5-34B-32k-IQ3_M.gguf) | IQ3_M | 15.56GB | Medium-low quality, new method with decent performance comparable to Q3_K_M. |
| [dolphin-2.9.3-Yi-1.5-34B-32k-Q3_K_S.gguf](https://huggingface.co/bartowski/dolphin-2.9.3-Yi-1.5-34B-32k-GGUF/blob/main/dolphin-2.9.3-Yi-1.5-34B-32k-Q3_K_S.gguf) | Q3_K_S | 14.96GB | Low quality, not recommended. |
| [dolphin-2.9.3-Yi-1.5-34B-32k-IQ3_XS.gguf](https://huggingface.co/bartowski/dolphin-2.9.3-Yi-1.5-34B-32k-GGUF/blob/main/dolphin-2.9.3-Yi-1.5-34B-32k-IQ3_XS.gguf) | IQ3_XS | 14.23GB | Lower quality, new method with decent performance, slightly better than Q3_K_S. |
| [dolphin-2.9.3-Yi-1.5-34B-32k-IQ3_XXS.gguf](https://huggingface.co/bartowski/dolphin-2.9.3-Yi-1.5-34B-32k-GGUF/blob/main/dolphin-2.9.3-Yi-1.5-34B-32k-IQ3_XXS.gguf) | IQ3_XXS | 13.33GB | Lower quality, new method with decent performance, comparable to Q3 quants. |
| [dolphin-2.9.3-Yi-1.5-34B-32k-Q2_K.gguf](https://huggingface.co/bartowski/dolphin-2.9.3-Yi-1.5-34B-32k-GGUF/blob/main/dolphin-2.9.3-Yi-1.5-34B-32k-Q2_K.gguf) | Q2_K | 12.82GB | Very low quality but surprisingly usable. |
| [dolphin-2.9.3-Yi-1.5-34B-32k-IQ2_M.gguf](https://huggingface.co/bartowski/dolphin-2.9.3-Yi-1.5-34B-32k-GGUF/blob/main/dolphin-2.9.3-Yi-1.5-34B-32k-IQ2_M.gguf) | IQ2_M | 11.79GB | Very low quality, uses SOTA techniques to also be surprisingly usable. |
| [dolphin-2.9.3-Yi-1.5-34B-32k-IQ2_S.gguf](https://huggingface.co/bartowski/dolphin-2.9.3-Yi-1.5-34B-32k-GGUF/blob/main/dolphin-2.9.3-Yi-1.5-34B-32k-IQ2_S.gguf) | IQ2_S | 10.89GB | Very low quality, uses SOTA techniques to be usable. |
| [dolphin-2.9.3-Yi-1.5-34B-32k-IQ2_XS.gguf](https://huggingface.co/bartowski/dolphin-2.9.3-Yi-1.5-34B-32k-GGUF/blob/main/dolphin-2.9.3-Yi-1.5-34B-32k-IQ2_XS.gguf) | IQ2_XS | 10.30GB | Very low quality, uses SOTA techniques to be usable. |
## Downloading using huggingface-cli
First, make sure you have hugginface-cli installed:
```
pip install -U "huggingface_hub[cli]"
```
Then, you can target the specific file you want:
```
huggingface-cli download bartowski/dolphin-2.9.3-Yi-1.5-34B-32k-GGUF --include "dolphin-2.9.3-Yi-1.5-34B-32k-Q4_K_M.gguf" --local-dir ./
```
If the model is bigger than 50GB, it will have been split into multiple files. In order to download them all to a local folder, run:
```
huggingface-cli download bartowski/dolphin-2.9.3-Yi-1.5-34B-32k-GGUF --include "dolphin-2.9.3-Yi-1.5-34B-32k-Q8_0.gguf/*" --local-dir dolphin-2.9.3-Yi-1.5-34B-32k-Q8_0
```
You can either specify a new local-dir (dolphin-2.9.3-Yi-1.5-34B-32k-Q8_0) or download them all in place (./)
## Which file should I choose?
A great write up with charts showing various performances is provided by Artefact2 [here](https://gist.github.com/Artefact2/b5f810600771265fc1e39442288e8ec9)
The first thing to figure out is how big a model you can run. To do this, you'll need to figure out how much RAM and/or VRAM you have.
If you want your model running as FAST as possible, you'll want to fit the whole thing on your GPU's VRAM. Aim for a quant with a file size 1-2GB smaller than your GPU's total VRAM.
If you want the absolute maximum quality, add both your system RAM and your GPU's VRAM together, then similarly grab a quant with a file size 1-2GB Smaller than that total.
Next, you'll need to decide if you want to use an 'I-quant' or a 'K-quant'.
If you don't want to think too much, grab one of the K-quants. These are in format 'QX_K_X', like Q5_K_M.
If you want to get more into the weeds, you can check out this extremely useful feature chart:
[llama.cpp feature matrix](https://github.com/ggerganov/llama.cpp/wiki/Feature-matrix)
But basically, if you're aiming for below Q4, and you're running cuBLAS (Nvidia) or rocBLAS (AMD), you should look towards the I-quants. These are in format IQX_X, like IQ3_M. These are newer and offer better performance for their size.
These I-quants can also be used on CPU and Apple Metal, but will be slower than their K-quant equivalent, so speed vs performance is a tradeoff you'll have to decide.
The I-quants are *not* compatible with Vulcan, which is also AMD, so if you have an AMD card double check if you're using the rocBLAS build or the Vulcan build. At the time of writing this, LM Studio has a preview with ROCm support, and other inference engines have specific builds for ROCm.
Want to support my work? Visit my ko-fi page here: https://ko-fi.com/bartowski
|
facebook/contriever-msmarco | facebook | "2022-06-25T17:19:59Z" | 108,663 | 19 | transformers | [
"transformers",
"pytorch",
"bert",
"feature-extraction",
"arxiv:2112.09118",
"endpoints_compatible",
"region:us"
] | feature-extraction | "2022-03-02T23:29:05Z" | ---
tags:
- feature-extraction
pipeline_tag: feature-extraction
---
This model is the finetuned version of the pre-trained contriever model available here https://huggingface.co/facebook/contriever, following the approach described in [Towards Unsupervised Dense Information Retrieval with Contrastive Learning](https://arxiv.org/abs/2112.09118). The associated GitHub repository is available here https://github.com/facebookresearch/contriever.
## Usage (HuggingFace Transformers)
Using the model directly available in HuggingFace transformers requires to add a mean pooling operation to obtain a sentence embedding.
```python
import torch
from transformers import AutoTokenizer, AutoModel
tokenizer = AutoTokenizer.from_pretrained('facebook/contriever-msmarco')
model = AutoModel.from_pretrained('facebook/contriever-msmarco')
sentences = [
"Where was Marie Curie born?",
"Maria Sklodowska, later known as Marie Curie, was born on November 7, 1867.",
"Born in Paris on 15 May 1859, Pierre Curie was the son of Eugène Curie, a doctor of French Catholic origin from Alsace."
]
# Apply tokenizer
inputs = tokenizer(sentences, padding=True, truncation=True, return_tensors='pt')
# Compute token embeddings
outputs = model(**inputs)
# Mean pooling
def mean_pooling(token_embeddings, mask):
token_embeddings = token_embeddings.masked_fill(~mask[..., None].bool(), 0.)
sentence_embeddings = token_embeddings.sum(dim=1) / mask.sum(dim=1)[..., None]
return sentence_embeddings
embeddings = mean_pooling(outputs[0], inputs['attention_mask'])
``` |
meta-llama/Llama-2-70b-chat-hf | meta-llama | "2024-04-17T08:41:06Z" | 108,595 | 2,120 | transformers | [
"transformers",
"pytorch",
"safetensors",
"llama",
"text-generation",
"facebook",
"meta",
"llama-2",
"conversational",
"en",
"arxiv:2307.09288",
"license:llama2",
"autotrain_compatible",
"endpoints_compatible",
"text-generation-inference",
"region:us"
] | text-generation | "2023-07-14T18:02:07Z" | ---
extra_gated_heading: You need to share contact information with Meta to access this model
extra_gated_prompt: >-
### LLAMA 2 COMMUNITY LICENSE AGREEMENT
"Agreement" means the terms and conditions for use, reproduction, distribution
and modification of the Llama Materials set forth herein.
"Documentation" means the specifications, manuals and documentation
accompanying Llama 2 distributed by Meta at
https://ai.meta.com/resources/models-and-libraries/llama-downloads/.
"Licensee" or "you" means you, or your employer or any other person or entity
(if you are entering into this Agreement on such person or entity's behalf),
of the age required under applicable laws, rules or regulations to provide
legal consent and that has legal authority to bind your employer or such other
person or entity if you are entering in this Agreement on their behalf.
"Llama 2" means the foundational large language models and software and
algorithms, including machine-learning model code, trained model weights,
inference-enabling code, training-enabling code, fine-tuning enabling code and
other elements of the foregoing distributed by Meta at
ai.meta.com/resources/models-and-libraries/llama-downloads/.
"Llama Materials" means, collectively, Meta's proprietary Llama 2 and
documentation (and any portion thereof) made available under this Agreement.
"Meta" or "we" means Meta Platforms Ireland Limited (if you are located in or,
if you are an entity, your principal place of business is in the EEA or
Switzerland) and Meta Platforms, Inc. (if you are located outside of the EEA
or Switzerland).
By clicking "I Accept" below or by using or distributing any portion or
element of the Llama Materials, you agree to be bound by this Agreement.
1. License Rights and Redistribution.
a. Grant of Rights. You are granted a non-exclusive, worldwide, non-
transferable and royalty-free limited license under Meta's intellectual
property or other rights owned by Meta embodied in the Llama Materials to
use, reproduce, distribute, copy, create derivative works of, and make
modifications to the Llama Materials.
b. Redistribution and Use.
i. If you distribute or make the Llama Materials, or any derivative works
thereof, available to a third party, you shall provide a copy of this
Agreement to such third party.
ii. If you receive Llama Materials, or any derivative works thereof, from a
Licensee as part of an integrated end user product, then Section 2 of this
Agreement will not apply to you.
iii. You must retain in all copies of the Llama Materials that you distribute
the following attribution notice within a "Notice" text file distributed as a
part of such copies: "Llama 2 is licensed under the LLAMA 2 Community
License, Copyright (c) Meta Platforms, Inc. All Rights Reserved."
iv. Your use of the Llama Materials must comply with applicable laws and
regulations (including trade compliance laws and regulations) and adhere to
the Acceptable Use Policy for the Llama Materials (available at
https://ai.meta.com/llama/use-policy), which is hereby incorporated by
reference into this Agreement.
v. You will not use the Llama Materials or any output or results of the Llama
Materials to improve any other large language model (excluding Llama 2 or
derivative works thereof).
2. Additional Commercial Terms. If, on the Llama 2 version release date, the
monthly active users of the products or services made available by or for
Licensee, or Licensee's affiliates, is greater than 700 million monthly
active users in the preceding calendar month, you must request a license from
Meta, which Meta may grant to you in its sole discretion, and you are not
authorized to exercise any of the rights under this Agreement unless or until
Meta otherwise expressly grants you such rights.
3. Disclaimer of Warranty. UNLESS REQUIRED BY APPLICABLE LAW, THE LLAMA
MATERIALS AND ANY OUTPUT AND RESULTS THEREFROM ARE PROVIDED ON AN "AS IS"
BASIS, WITHOUT WARRANTIES OF ANY KIND, EITHER EXPRESS OR IMPLIED, INCLUDING,
WITHOUT LIMITATION, ANY WARRANTIES OF TITLE, NON-INFRINGEMENT,
MERCHANTABILITY, OR FITNESS FOR A PARTICULAR PURPOSE. YOU ARE SOLELY
RESPONSIBLE FOR DETERMINING THE APPROPRIATENESS OF USING OR REDISTRIBUTING
THE LLAMA MATERIALS AND ASSUME ANY RISKS ASSOCIATED WITH YOUR USE OF THE
LLAMA MATERIALS AND ANY OUTPUT AND RESULTS.
4. Limitation of Liability. IN NO EVENT WILL META OR ITS AFFILIATES BE LIABLE
UNDER ANY THEORY OF LIABILITY, WHETHER IN CONTRACT, TORT, NEGLIGENCE,
PRODUCTS LIABILITY, OR OTHERWISE, ARISING OUT OF THIS AGREEMENT, FOR ANY LOST
PROFITS OR ANY INDIRECT, SPECIAL, CONSEQUENTIAL, INCIDENTAL, EXEMPLARY OR
PUNITIVE DAMAGES, EVEN IF META OR ITS AFFILIATES HAVE BEEN ADVISED OF THE
POSSIBILITY OF ANY OF THE FOREGOING.
5. Intellectual Property.
a. No trademark licenses are granted under this Agreement, and in connection
with the Llama Materials, neither Meta nor Licensee may use any name or mark
owned by or associated with the other or any of its affiliates, except as
required for reasonable and customary use in describing and redistributing
the Llama Materials.
b. Subject to Meta's ownership of Llama Materials and derivatives made by or
for Meta, with respect to any derivative works and modifications of the Llama
Materials that are made by you, as between you and Meta, you are and will be
the owner of such derivative works and modifications.
c. If you institute litigation or other proceedings against Meta or any
entity (including a cross-claim or counterclaim in a lawsuit) alleging that
the Llama Materials or Llama 2 outputs or results, or any portion of any of
the foregoing, constitutes infringement of intellectual property or other
rights owned or licensable by you, then any licenses granted to you under
this Agreement shall terminate as of the date such litigation or claim is
filed or instituted. You will indemnify and hold harmless Meta from and
against any claim by any third party arising out of or related to your use or
distribution of the Llama Materials.
6. Term and Termination. The term of this Agreement will commence upon your
acceptance of this Agreement or access to the Llama Materials and will
continue in full force and effect until terminated in accordance with the
terms and conditions herein. Meta may terminate this Agreement if you are in
breach of any term or condition of this Agreement. Upon termination of this
Agreement, you shall delete and cease use of the Llama Materials. Sections 3,
4 and 7 shall survive the termination of this Agreement.
7. Governing Law and Jurisdiction. This Agreement will be governed and
construed under the laws of the State of California without regard to choice
of law principles, and the UN Convention on Contracts for the International
Sale of Goods does not apply to this Agreement. The courts of California
shall have exclusive jurisdiction of any dispute arising out of this
Agreement.
### Llama 2 Acceptable Use Policy
Meta is committed to promoting safe and fair use of its tools and features,
including Llama 2. If you access or use Llama 2, you agree to this Acceptable
Use Policy (“Policy”). The most recent copy of this policy can be found at
[ai.meta.com/llama/use-policy](http://ai.meta.com/llama/use-policy).
#### Prohibited Uses
We want everyone to use Llama 2 safely and responsibly. You agree you will not
use, or allow others to use, Llama 2 to:
1. Violate the law or others’ rights, including to:
1. Engage in, promote, generate, contribute to, encourage, plan, incite, or further illegal or unlawful activity or content, such as:
1. Violence or terrorism
2. Exploitation or harm to children, including the solicitation, creation, acquisition, or dissemination of child exploitative content or failure to report Child Sexual Abuse Material
3. Human trafficking, exploitation, and sexual violence
4. The illegal distribution of information or materials to minors, including obscene materials, or failure to employ legally required age-gating in connection with such information or materials.
5. Sexual solicitation
6. Any other criminal activity
2. Engage in, promote, incite, or facilitate the harassment, abuse, threatening, or bullying of individuals or groups of individuals
3. Engage in, promote, incite, or facilitate discrimination or other unlawful or harmful conduct in the provision of employment, employment benefits, credit, housing, other economic benefits, or other essential goods and services
4. Engage in the unauthorized or unlicensed practice of any profession including, but not limited to, financial, legal, medical/health, or related professional practices
5. Collect, process, disclose, generate, or infer health, demographic, or other sensitive personal or private information about individuals without rights and consents required by applicable laws
6. Engage in or facilitate any action or generate any content that infringes, misappropriates, or otherwise violates any third-party rights, including the outputs or results of any products or services using the Llama 2 Materials
7. Create, generate, or facilitate the creation of malicious code, malware, computer viruses or do anything else that could disable, overburden, interfere with or impair the proper working, integrity, operation or appearance of a website or computer system
2. Engage in, promote, incite, facilitate, or assist in the planning or
development of activities that present a risk of death or bodily harm to
individuals, including use of Llama 2 related to the following:
1. Military, warfare, nuclear industries or applications, espionage, use for materials or activities that are subject to the International Traffic Arms Regulations (ITAR) maintained by the United States Department of State
2. Guns and illegal weapons (including weapon development)
3. Illegal drugs and regulated/controlled substances
4. Operation of critical infrastructure, transportation technologies, or heavy machinery
5. Self-harm or harm to others, including suicide, cutting, and eating disorders
6. Any content intended to incite or promote violence, abuse, or any infliction of bodily harm to an individual
3. Intentionally deceive or mislead others, including use of Llama 2 related
to the following:
1. Generating, promoting, or furthering fraud or the creation or promotion of disinformation
2. Generating, promoting, or furthering defamatory content, including the creation of defamatory statements, images, or other content
3. Generating, promoting, or further distributing spam
4. Impersonating another individual without consent, authorization, or legal right
5. Representing that the use of Llama 2 or outputs are human-generated
6. Generating or facilitating false online engagement, including fake reviews and other means of fake online engagement
4. Fail to appropriately disclose to end users any known dangers of your AI system
Please report any violation of this Policy, software “bug,” or other problems
that could lead to a violation of this Policy through one of the following
means:
* Reporting issues with the model: [github.com/facebookresearch/llama](http://github.com/facebookresearch/llama)
* Reporting risky content generated by the model: [developers.facebook.com/llama_output_feedback](http://developers.facebook.com/llama_output_feedback)
* Reporting bugs and security concerns: [facebook.com/whitehat/info](http://facebook.com/whitehat/info)
* Reporting violations of the Acceptable Use Policy or unlicensed uses of Llama: [LlamaUseReport@meta.com](mailto:LlamaUseReport@meta.com)
extra_gated_fields:
First Name: text
Last Name: text
Date of birth: date_picker
Country: country
Affiliation: text
geo: ip_location
By clicking Submit below I accept the terms of the license and acknowledge that the information I provide will be collected stored processed and shared in accordance with the Meta Privacy Policy: checkbox
extra_gated_description: >-
The information you provide will be collected, stored, processed and shared in
accordance with the [Meta Privacy
Policy](https://www.facebook.com/privacy/policy/).
extra_gated_button_content: Submit
language:
- en
pipeline_tag: text-generation
tags:
- facebook
- meta
- pytorch
- llama
- llama-2
license: llama2
---
# **Llama 2**
Llama 2 is a collection of pretrained and fine-tuned generative text models ranging in scale from 7 billion to 70 billion parameters. This is the repository for the 70B fine-tuned model, optimized for dialogue use cases and converted for the Hugging Face Transformers format. Links to other models can be found in the index at the bottom.
## Model Details
*Note: Use of this model is governed by the Meta license. In order to download the model weights and tokenizer, please visit the [website](https://ai.meta.com/resources/models-and-libraries/llama-downloads/) and accept our License before requesting access here.*
Meta developed and publicly released the Llama 2 family of large language models (LLMs), a collection of pretrained and fine-tuned generative text models ranging in scale from 7 billion to 70 billion parameters. Our fine-tuned LLMs, called Llama-2-Chat, are optimized for dialogue use cases. Llama-2-Chat models outperform open-source chat models on most benchmarks we tested, and in our human evaluations for helpfulness and safety, are on par with some popular closed-source models like ChatGPT and PaLM.
**Model Developers** Meta
**Variations** Llama 2 comes in a range of parameter sizes — 7B, 13B, and 70B — as well as pretrained and fine-tuned variations.
**Input** Models input text only.
**Output** Models generate text only.
**Model Architecture** Llama 2 is an auto-regressive language model that uses an optimized transformer architecture. The tuned versions use supervised fine-tuning (SFT) and reinforcement learning with human feedback (RLHF) to align to human preferences for helpfulness and safety.
||Training Data|Params|Content Length|GQA|Tokens|LR|
|---|---|---|---|---|---|---|
|Llama 2|*A new mix of publicly available online data*|7B|4k|✗|2.0T|3.0 x 10<sup>-4</sup>|
|Llama 2|*A new mix of publicly available online data*|13B|4k|✗|2.0T|3.0 x 10<sup>-4</sup>|
|Llama 2|*A new mix of publicly available online data*|70B|4k|✔|2.0T|1.5 x 10<sup>-4</sup>|
*Llama 2 family of models.* Token counts refer to pretraining data only. All models are trained with a global batch-size of 4M tokens. Bigger models - 70B -- use Grouped-Query Attention (GQA) for improved inference scalability.
**Model Dates** Llama 2 was trained between January 2023 and July 2023.
**Status** This is a static model trained on an offline dataset. Future versions of the tuned models will be released as we improve model safety with community feedback.
**License** A custom commercial license is available at: [https://ai.meta.com/resources/models-and-libraries/llama-downloads/](https://ai.meta.com/resources/models-and-libraries/llama-downloads/)
**Research Paper** ["Llama-2: Open Foundation and Fine-tuned Chat Models"](arxiv.org/abs/2307.09288)
## Intended Use
**Intended Use Cases** Llama 2 is intended for commercial and research use in English. Tuned models are intended for assistant-like chat, whereas pretrained models can be adapted for a variety of natural language generation tasks.
**Out-of-scope Uses** Use in any manner that violates applicable laws or regulations (including trade compliance laws).Use in languages other than English. Use in any other way that is prohibited by the Acceptable Use Policy and Licensing Agreement for Llama 2.
## Hardware and Software
**Training Factors** We used custom training libraries, Meta's Research Super Cluster, and production clusters for pretraining. Fine-tuning, annotation, and evaluation were also performed on third-party cloud compute.
**Carbon Footprint** Pretraining utilized a cumulative 3.3M GPU hours of computation on hardware of type A100-80GB (TDP of 350-400W). Estimated total emissions were 539 tCO2eq, 100% of which were offset by Meta’s sustainability program.
||Time (GPU hours)|Power Consumption (W)|Carbon Emitted(tCO<sub>2</sub>eq)|
|---|---|---|---|
|Llama 2 7B|184320|400|31.22|
|Llama 2 13B|368640|400|62.44|
|Llama 2 70B|1720320|400|291.42|
|Total|3311616||539.00|
**CO<sub>2</sub> emissions during pretraining.** Time: total GPU time required for training each model. Power Consumption: peak power capacity per GPU device for the GPUs used adjusted for power usage efficiency. 100% of the emissions are directly offset by Meta's sustainability program, and because we are openly releasing these models, the pretraining costs do not need to be incurred by others.
## Training Data
**Overview** Llama 2 was pretrained on 2 trillion tokens of data from publicly available sources. The fine-tuning data includes publicly available instruction datasets, as well as over one million new human-annotated examples. Neither the pretraining nor the fine-tuning datasets include Meta user data.
**Data Freshness** The pretraining data has a cutoff of September 2022, but some tuning data is more recent, up to July 2023.
## Evaluation Results
In this section, we report the results for the Llama 1 and Llama 2 models on standard academic benchmarks.For all the evaluations, we use our internal evaluations library.
|Model|Size|Code|Commonsense Reasoning|World Knowledge|Reading Comprehension|Math|MMLU|BBH|AGI Eval|
|---|---|---|---|---|---|---|---|---|---|
|Llama 1|7B|14.1|60.8|46.2|58.5|6.95|35.1|30.3|23.9|
|Llama 1|13B|18.9|66.1|52.6|62.3|10.9|46.9|37.0|33.9|
|Llama 1|33B|26.0|70.0|58.4|67.6|21.4|57.8|39.8|41.7|
|Llama 1|65B|30.7|70.7|60.5|68.6|30.8|63.4|43.5|47.6|
|Llama 2|7B|16.8|63.9|48.9|61.3|14.6|45.3|32.6|29.3|
|Llama 2|13B|24.5|66.9|55.4|65.8|28.7|54.8|39.4|39.1|
|Llama 2|70B|**37.5**|**71.9**|**63.6**|**69.4**|**35.2**|**68.9**|**51.2**|**54.2**|
**Overall performance on grouped academic benchmarks.** *Code:* We report the average pass@1 scores of our models on HumanEval and MBPP. *Commonsense Reasoning:* We report the average of PIQA, SIQA, HellaSwag, WinoGrande, ARC easy and challenge, OpenBookQA, and CommonsenseQA. We report 7-shot results for CommonSenseQA and 0-shot results for all other benchmarks. *World Knowledge:* We evaluate the 5-shot performance on NaturalQuestions and TriviaQA and report the average. *Reading Comprehension:* For reading comprehension, we report the 0-shot average on SQuAD, QuAC, and BoolQ. *MATH:* We report the average of the GSM8K (8 shot) and MATH (4 shot) benchmarks at top 1.
|||TruthfulQA|Toxigen|
|---|---|---|---|
|Llama 1|7B|27.42|23.00|
|Llama 1|13B|41.74|23.08|
|Llama 1|33B|44.19|22.57|
|Llama 1|65B|48.71|21.77|
|Llama 2|7B|33.29|**21.25**|
|Llama 2|13B|41.86|26.10|
|Llama 2|70B|**50.18**|24.60|
**Evaluation of pretrained LLMs on automatic safety benchmarks.** For TruthfulQA, we present the percentage of generations that are both truthful and informative (the higher the better). For ToxiGen, we present the percentage of toxic generations (the smaller the better).
|||TruthfulQA|Toxigen|
|---|---|---|---|
|Llama-2-Chat|7B|57.04|**0.00**|
|Llama-2-Chat|13B|62.18|**0.00**|
|Llama-2-Chat|70B|**64.14**|0.01|
**Evaluation of fine-tuned LLMs on different safety datasets.** Same metric definitions as above.
## Ethical Considerations and Limitations
Llama 2 is a new technology that carries risks with use. Testing conducted to date has been in English, and has not covered, nor could it cover all scenarios. For these reasons, as with all LLMs, Llama 2’s potential outputs cannot be predicted in advance, and the model may in some instances produce inaccurate, biased or other objectionable responses to user prompts. Therefore, before deploying any applications of Llama 2, developers should perform safety testing and tuning tailored to their specific applications of the model.
Please see the Responsible Use Guide available at [https://ai.meta.com/llama/responsible-use-guide/](https://ai.meta.com/llama/responsible-use-guide)
## Reporting Issues
Please report any software “bug,” or other problems with the models through one of the following means:
- Reporting issues with the model: [github.com/facebookresearch/llama](http://github.com/facebookresearch/llama)
- Reporting problematic content generated by the model: [developers.facebook.com/llama_output_feedback](http://developers.facebook.com/llama_output_feedback)
- Reporting bugs and security concerns: [facebook.com/whitehat/info](http://facebook.com/whitehat/info)
## Llama Model Index
|Model|Llama2|Llama2-hf|Llama2-chat|Llama2-chat-hf|
|---|---|---|---|---|
|7B| [Link](https://huggingface.co/meta-llama/Llama-2-7b) | [Link](https://huggingface.co/meta-llama/Llama-2-7b-hf) | [Link](https://huggingface.co/meta-llama/Llama-2-7b-chat) | [Link](https://huggingface.co/meta-llama/Llama-2-7b-chat-hf)|
|13B| [Link](https://huggingface.co/meta-llama/Llama-2-13b) | [Link](https://huggingface.co/meta-llama/Llama-2-13b-hf) | [Link](https://huggingface.co/meta-llama/Llama-2-13b-chat) | [Link](https://huggingface.co/meta-llama/Llama-2-13b-chat-hf)|
|70B| [Link](https://huggingface.co/meta-llama/Llama-2-70b) | [Link](https://huggingface.co/meta-llama/Llama-2-70b-hf) | [Link](https://huggingface.co/meta-llama/Llama-2-70b-chat) | [Link](https://huggingface.co/meta-llama/Llama-2-70b-chat-hf)| |
EleutherAI/pythia-160m | EleutherAI | "2023-07-09T15:52:09Z" | 107,846 | 21 | transformers | [
"transformers",
"pytorch",
"safetensors",
"gpt_neox",
"text-generation",
"causal-lm",
"pythia",
"en",
"dataset:EleutherAI/pile",
"arxiv:2304.01373",
"arxiv:2101.00027",
"arxiv:2201.07311",
"license:apache-2.0",
"autotrain_compatible",
"endpoints_compatible",
"text-generation-inference",
"region:us"
] | text-generation | "2023-02-08T19:25:46Z" | ---
language:
- en
tags:
- pytorch
- causal-lm
- pythia
license: apache-2.0
datasets:
- EleutherAI/pile
---
The *Pythia Scaling Suite* is a collection of models developed to facilitate
interpretability research [(see paper)](https://arxiv.org/pdf/2304.01373.pdf).
It contains two sets of eight models of sizes
70M, 160M, 410M, 1B, 1.4B, 2.8B, 6.9B, and 12B. For each size, there are two
models: one trained on the Pile, and one trained on the Pile after the dataset
has been globally deduplicated. All 8 model sizes are trained on the exact
same data, in the exact same order. We also provide 154 intermediate
checkpoints per model, hosted on Hugging Face as branches.
The Pythia model suite was deliberately designed to promote scientific
research on large language models, especially interpretability research.
Despite not centering downstream performance as a design goal, we find the
models <a href="#evaluations">match or exceed</a> the performance of
similar and same-sized models, such as those in the OPT and GPT-Neo suites.
<details>
<summary style="font-weight:600">Details on previous early release and naming convention.</summary>
Previously, we released an early version of the Pythia suite to the public.
However, we decided to retrain the model suite to address a few hyperparameter
discrepancies. This model card <a href="#changelog">lists the changes</a>;
see appendix B in the Pythia paper for further discussion. We found no
difference in benchmark performance between the two Pythia versions.
The old models are
[still available](https://huggingface.co/models?other=pythia_v0), but we
suggest the retrained suite if you are just starting to use Pythia.<br>
**This is the current release.**
Please note that all models in the *Pythia* suite were renamed in January
2023. For clarity, a <a href="#naming-convention-and-parameter-count">table
comparing the old and new names</a> is provided in this model card, together
with exact parameter counts.
</details>
<br>
# Pythia-160M
## Model Details
- Developed by: [EleutherAI](http://eleuther.ai)
- Model type: Transformer-based Language Model
- Language: English
- Learn more: [Pythia's GitHub repository](https://github.com/EleutherAI/pythia)
for training procedure, config files, and details on how to use.
[See paper](https://arxiv.org/pdf/2304.01373.pdf) for more evals and implementation
details.
- Library: [GPT-NeoX](https://github.com/EleutherAI/gpt-neox)
- License: Apache 2.0
- Contact: to ask questions about this model, join the [EleutherAI
Discord](https://discord.gg/zBGx3azzUn), and post them in `#release-discussion`.
Please read the existing *Pythia* documentation before asking about it in the
EleutherAI Discord. For general correspondence: [contact@eleuther.
ai](mailto:contact@eleuther.ai).
<figure>
| Pythia model | Non-Embedding Params | Layers | Model Dim | Heads | Batch Size | Learning Rate | Equivalent Models |
| -----------: | -------------------: | :----: | :-------: | :---: | :--------: | :-------------------: | :--------------------: |
| 70M | 18,915,328 | 6 | 512 | 8 | 2M | 1.0 x 10<sup>-3</sup> | — |
| 160M | 85,056,000 | 12 | 768 | 12 | 2M | 6.0 x 10<sup>-4</sup> | GPT-Neo 125M, OPT-125M |
| 410M | 302,311,424 | 24 | 1024 | 16 | 2M | 3.0 x 10<sup>-4</sup> | OPT-350M |
| 1.0B | 805,736,448 | 16 | 2048 | 8 | 2M | 3.0 x 10<sup>-4</sup> | — |
| 1.4B | 1,208,602,624 | 24 | 2048 | 16 | 2M | 2.0 x 10<sup>-4</sup> | GPT-Neo 1.3B, OPT-1.3B |
| 2.8B | 2,517,652,480 | 32 | 2560 | 32 | 2M | 1.6 x 10<sup>-4</sup> | GPT-Neo 2.7B, OPT-2.7B |
| 6.9B | 6,444,163,072 | 32 | 4096 | 32 | 2M | 1.2 x 10<sup>-4</sup> | OPT-6.7B |
| 12B | 11,327,027,200 | 36 | 5120 | 40 | 2M | 1.2 x 10<sup>-4</sup> | — |
<figcaption>Engineering details for the <i>Pythia Suite</i>. Deduped and
non-deduped models of a given size have the same hyperparameters. “Equivalent”
models have <b>exactly</b> the same architecture, and the same number of
non-embedding parameters.</figcaption>
</figure>
## Uses and Limitations
### Intended Use
The primary intended use of Pythia is research on the behavior, functionality,
and limitations of large language models. This suite is intended to provide
a controlled setting for performing scientific experiments. We also provide
154 checkpoints per model: initial `step0`, 10 log-spaced checkpoints
`step{1,2,4...512}`, and 143 evenly-spaced checkpoints from `step1000` to
`step143000`. These checkpoints are hosted on Hugging Face as branches. Note
that branch `143000` corresponds exactly to the model checkpoint on the `main`
branch of each model.
You may also further fine-tune and adapt Pythia-160M for deployment,
as long as your use is in accordance with the Apache 2.0 license. Pythia
models work with the Hugging Face [Transformers
Library](https://huggingface.co/docs/transformers/index). If you decide to use
pre-trained Pythia-160M as a basis for your fine-tuned model, please
conduct your own risk and bias assessment.
### Out-of-scope use
The Pythia Suite is **not** intended for deployment. It is not a in itself
a product and cannot be used for human-facing interactions. For example,
the model may generate harmful or offensive text. Please evaluate the risks
associated with your particular use case.
Pythia models are English-language only, and are not suitable for translation
or generating text in other languages.
Pythia-160M has not been fine-tuned for downstream contexts in which
language models are commonly deployed, such as writing genre prose,
or commercial chatbots. This means Pythia-160M will **not**
respond to a given prompt the way a product like ChatGPT does. This is because,
unlike this model, ChatGPT was fine-tuned using methods such as Reinforcement
Learning from Human Feedback (RLHF) to better “follow” human instructions.
### Limitations and biases
The core functionality of a large language model is to take a string of text
and predict the next token. The token used by the model need not produce the
most “accurate” text. Never rely on Pythia-160M to produce factually accurate
output.
This model was trained on [the Pile](https://pile.eleuther.ai/), a dataset
known to contain profanity and texts that are lewd or otherwise offensive.
See [Section 6 of the Pile paper](https://arxiv.org/abs/2101.00027) for a
discussion of documented biases with regards to gender, religion, and race.
Pythia-160M may produce socially unacceptable or undesirable text, *even if*
the prompt itself does not include anything explicitly offensive.
If you plan on using text generated through, for example, the Hosted Inference
API, we recommend having a human curate the outputs of this language model
before presenting it to other people. Please inform your audience that the
text was generated by Pythia-160M.
### Quickstart
Pythia models can be loaded and used via the following code, demonstrated here
for the third `pythia-70m-deduped` checkpoint:
```python
from transformers import GPTNeoXForCausalLM, AutoTokenizer
model = GPTNeoXForCausalLM.from_pretrained(
"EleutherAI/pythia-70m-deduped",
revision="step3000",
cache_dir="./pythia-70m-deduped/step3000",
)
tokenizer = AutoTokenizer.from_pretrained(
"EleutherAI/pythia-70m-deduped",
revision="step3000",
cache_dir="./pythia-70m-deduped/step3000",
)
inputs = tokenizer("Hello, I am", return_tensors="pt")
tokens = model.generate(**inputs)
tokenizer.decode(tokens[0])
```
Revision/branch `step143000` corresponds exactly to the model checkpoint on
the `main` branch of each model.<br>
For more information on how to use all Pythia models, see [documentation on
GitHub](https://github.com/EleutherAI/pythia).
## Training
### Training data
[The Pile](https://pile.eleuther.ai/) is a 825GiB general-purpose dataset in
English. It was created by EleutherAI specifically for training large language
models. It contains texts from 22 diverse sources, roughly broken down into
five categories: academic writing (e.g. arXiv), internet (e.g. CommonCrawl),
prose (e.g. Project Gutenberg), dialogue (e.g. YouTube subtitles), and
miscellaneous (e.g. GitHub, Enron Emails). See [the Pile
paper](https://arxiv.org/abs/2101.00027) for a breakdown of all data sources,
methodology, and a discussion of ethical implications. Consult [the
datasheet](https://arxiv.org/abs/2201.07311) for more detailed documentation
about the Pile and its component datasets. The Pile can be downloaded from
the [official website](https://pile.eleuther.ai/), or from a [community
mirror](https://the-eye.eu/public/AI/pile/).<br>
The Pile was **not** deduplicated before being used to train Pythia-160M.
### Training procedure
All models were trained on the exact same data, in the exact same order. Each
model saw 299,892,736,000 tokens during training, and 143 checkpoints for each
model are saved every 2,097,152,000 tokens, spaced evenly throughout training,
from `step1000` to `step143000` (which is the same as `main`). In addition, we
also provide frequent early checkpoints: `step0` and `step{1,2,4...512}`.
This corresponds to training for just under 1 epoch on the Pile for
non-deduplicated models, and about 1.5 epochs on the deduplicated Pile.
All *Pythia* models trained for 143000 steps at a batch size
of 2M (2,097,152 tokens).<br>
See [GitHub](https://github.com/EleutherAI/pythia) for more details on training
procedure, including [how to reproduce
it](https://github.com/EleutherAI/pythia/blob/main/README.md#reproducing-training).<br>
Pythia uses the same tokenizer as [GPT-NeoX-
20B](https://huggingface.co/EleutherAI/gpt-neox-20b).
## Evaluations
All 16 *Pythia* models were evaluated using the [LM Evaluation
Harness](https://github.com/EleutherAI/lm-evaluation-harness). You can access
the results by model and step at `results/json/*` in the [GitHub
repository](https://github.com/EleutherAI/pythia/tree/main/results/json/).<br>
Expand the sections below to see plots of evaluation results for all
Pythia and Pythia-deduped models compared with OPT and BLOOM.
<details>
<summary>LAMBADA – OpenAI</summary>
<img src="/EleutherAI/pythia-12b/resolve/main/eval_plots/lambada_openai_v1.png" style="width:auto"/>
</details>
<details>
<summary>Physical Interaction: Question Answering (PIQA)</summary>
<img src="/EleutherAI/pythia-12b/resolve/main/eval_plots/piqa_v1.png" style="width:auto"/>
</details>
<details>
<summary>WinoGrande</summary>
<img src="/EleutherAI/pythia-12b/resolve/main/eval_plots/winogrande_v1.png" style="width:auto"/>
</details>
<details>
<summary>AI2 Reasoning Challenge—Easy Set</summary>
<img src="/EleutherAI/pythia-12b/resolve/main/eval_plots/arc_easy_v1.png" style="width:auto"/>
</details>
<details>
<summary>SciQ</summary>
<img src="/EleutherAI/pythia-12b/resolve/main/eval_plots/sciq_v1.png" style="width:auto"/>
</details>
## Changelog
This section compares differences between previously released
[Pythia v0](https://huggingface.co/models?other=pythia_v0) and the current
models. See Appendix B of the Pythia paper for further discussion of these
changes and the motivation behind them. We found that retraining Pythia had no
impact on benchmark performance.
- All model sizes are now trained with uniform batch size of 2M tokens.
Previously, the models of size 160M, 410M, and 1.4B parameters were trained
with batch sizes of 4M tokens.
- We added checkpoints at initialization (step 0) and steps {1,2,4,8,16,32,64,
128,256,512} in addition to every 1000 training steps.
- Flash Attention was used in the new retrained suite.
- We remedied a minor inconsistency that existed in the original suite: all
models of size 2.8B parameters or smaller had a learning rate (LR) schedule
which decayed to a minimum LR of 10% the starting LR rate, but the 6.9B and
12B models all used an LR schedule which decayed to a minimum LR of 0. In
the redone training runs, we rectified this inconsistency: all models now were
trained with LR decaying to a minimum of 0.1× their maximum LR.
### Naming convention and parameter count
*Pythia* models were renamed in January 2023. It is possible that the old
naming convention still persists in some documentation by accident. The
current naming convention (70M, 160M, etc.) is based on total parameter count.
<figure style="width:32em">
| current Pythia suffix | old suffix | total params | non-embedding params |
| --------------------: | ---------: | -------------: | -------------------: |
| 70M | 19M | 70,426,624 | 18,915,328 |
| 160M | 125M | 162,322,944 | 85,056,000 |
| 410M | 350M | 405,334,016 | 302,311,424 |
| 1B | 800M | 1,011,781,632 | 805,736,448 |
| 1.4B | 1.3B | 1,414,647,808 | 1,208,602,624 |
| 2.8B | 2.7B | 2,775,208,960 | 2,517,652,480 |
| 6.9B | 6.7B | 6,857,302,016 | 6,444,163,072 |
| 12B | 13B | 11,846,072,320 | 11,327,027,200 |
</figure> |
mradermacher/Qwen2-57B-A14B-Instruct-i1-GGUF | mradermacher | "2024-06-23T17:19:56Z" | 107,643 | 1 | transformers | [
"transformers",
"gguf",
"chat",
"en",
"base_model:Qwen/Qwen2-57B-A14B-Instruct",
"license:apache-2.0",
"endpoints_compatible",
"region:us"
] | null | "2024-06-23T11:54:38Z" | ---
base_model: Qwen/Qwen2-57B-A14B-Instruct
language:
- en
library_name: transformers
license: apache-2.0
quantized_by: mradermacher
tags:
- chat
---
## About
<!-- ### quantize_version: 2 -->
<!-- ### output_tensor_quantised: 1 -->
<!-- ### convert_type: hf -->
<!-- ### vocab_type: -->
<!-- ### tags: nicoboss -->
weighted/imatrix quants of https://huggingface.co/Qwen/Qwen2-57B-A14B-Instruct
**The Qwen2-57B models seem to be broken. I have tried my best, but they likely need to be fixed upstream first. You have been warned.**
<!-- provided-files -->
static quants are available at https://huggingface.co/mradermacher/Qwen2-57B-A14B-Instruct-GGUF
## Usage
If you are unsure how to use GGUF files, refer to one of [TheBloke's
READMEs](https://huggingface.co/TheBloke/KafkaLM-70B-German-V0.1-GGUF) for
more details, including on how to concatenate multi-part files.
## Provided Quants
(sorted by size, not necessarily quality. IQ-quants are often preferable over similar sized non-IQ quants)
| Link | Type | Size/GB | Notes |
|:-----|:-----|--------:|:------|
| [GGUF](https://huggingface.co/mradermacher/Qwen2-57B-A14B-Instruct-i1-GGUF/resolve/main/Qwen2-57B-A14B-Instruct.i1-Q2_K.gguf) | i1-Q2_K | 21.2 | IQ3_XXS probably better |
| [GGUF](https://huggingface.co/mradermacher/Qwen2-57B-A14B-Instruct-i1-GGUF/resolve/main/Qwen2-57B-A14B-Instruct.i1-IQ3_XXS.gguf) | i1-IQ3_XXS | 22.3 | lower quality |
| [GGUF](https://huggingface.co/mradermacher/Qwen2-57B-A14B-Instruct-i1-GGUF/resolve/main/Qwen2-57B-A14B-Instruct.i1-IQ3_XS.gguf) | i1-IQ3_XS | 23.7 | |
| [GGUF](https://huggingface.co/mradermacher/Qwen2-57B-A14B-Instruct-i1-GGUF/resolve/main/Qwen2-57B-A14B-Instruct.i1-Q3_K_S.gguf) | i1-Q3_K_S | 25.0 | IQ3_XS probably better |
| [GGUF](https://huggingface.co/mradermacher/Qwen2-57B-A14B-Instruct-i1-GGUF/resolve/main/Qwen2-57B-A14B-Instruct.i1-IQ3_S.gguf) | i1-IQ3_S | 25.0 | beats Q3_K* |
| [GGUF](https://huggingface.co/mradermacher/Qwen2-57B-A14B-Instruct-i1-GGUF/resolve/main/Qwen2-57B-A14B-Instruct.i1-IQ3_M.gguf) | i1-IQ3_M | 25.3 | |
| [GGUF](https://huggingface.co/mradermacher/Qwen2-57B-A14B-Instruct-i1-GGUF/resolve/main/Qwen2-57B-A14B-Instruct.i1-Q3_K_M.gguf) | i1-Q3_K_M | 27.6 | IQ3_S probably better |
| [GGUF](https://huggingface.co/mradermacher/Qwen2-57B-A14B-Instruct-i1-GGUF/resolve/main/Qwen2-57B-A14B-Instruct.i1-Q3_K_L.gguf) | i1-Q3_K_L | 29.9 | IQ3_M probably better |
| [GGUF](https://huggingface.co/mradermacher/Qwen2-57B-A14B-Instruct-i1-GGUF/resolve/main/Qwen2-57B-A14B-Instruct.i1-IQ4_XS.gguf) | i1-IQ4_XS | 30.8 | |
| [GGUF](https://huggingface.co/mradermacher/Qwen2-57B-A14B-Instruct-i1-GGUF/resolve/main/Qwen2-57B-A14B-Instruct.i1-Q4_0.gguf) | i1-Q4_0 | 32.7 | fast, low quality |
| [GGUF](https://huggingface.co/mradermacher/Qwen2-57B-A14B-Instruct-i1-GGUF/resolve/main/Qwen2-57B-A14B-Instruct.i1-Q4_K_S.gguf) | i1-Q4_K_S | 32.8 | optimal size/speed/quality |
| [GGUF](https://huggingface.co/mradermacher/Qwen2-57B-A14B-Instruct-i1-GGUF/resolve/main/Qwen2-57B-A14B-Instruct.i1-Q4_K_M.gguf) | i1-Q4_K_M | 35.0 | fast, recommended |
| [GGUF](https://huggingface.co/mradermacher/Qwen2-57B-A14B-Instruct-i1-GGUF/resolve/main/Qwen2-57B-A14B-Instruct.i1-Q5_K_S.gguf) | i1-Q5_K_S | 39.7 | |
| [GGUF](https://huggingface.co/mradermacher/Qwen2-57B-A14B-Instruct-i1-GGUF/resolve/main/Qwen2-57B-A14B-Instruct.i1-Q5_K_M.gguf) | i1-Q5_K_M | 40.9 | |
| [GGUF](https://huggingface.co/mradermacher/Qwen2-57B-A14B-Instruct-i1-GGUF/resolve/main/Qwen2-57B-A14B-Instruct.i1-Q6_K.gguf) | i1-Q6_K | 47.2 | practically like static Q6_K |
Here is a handy graph by ikawrakow comparing some lower-quality quant
types (lower is better):

And here are Artefact2's thoughts on the matter:
https://gist.github.com/Artefact2/b5f810600771265fc1e39442288e8ec9
## FAQ / Model Request
See https://huggingface.co/mradermacher/model_requests for some answers to
questions you might have and/or if you want some other model quantized.
## Thanks
I thank my company, [nethype GmbH](https://www.nethype.de/), for letting
me use its servers and providing upgrades to my workstation to enable
this work in my free time. Additional thanks to [@nicoboss](https://huggingface.co/nicoboss) for giving me access to his hardware for calculating the imatrix for these quants.
<!-- end -->
|
Salesforce/codet5p-770m | Salesforce | "2023-05-16T00:33:03Z" | 107,573 | 17 | transformers | [
"transformers",
"pytorch",
"t5",
"text2text-generation",
"arxiv:2305.07922",
"license:bsd-3-clause",
"autotrain_compatible",
"endpoints_compatible",
"text-generation-inference",
"region:us"
] | text2text-generation | "2023-05-13T13:34:17Z" | ---
license: bsd-3-clause
---
# CodeT5+ 770M
## Model description
[CodeT5+](https://github.com/salesforce/CodeT5/tree/main/CodeT5+) is a new family of open code large language models with an encoder-decoder architecture that can flexibly operate in different modes (i.e. _encoder-only_, _decoder-only_, and _encoder-decoder_) to support a wide range of code understanding and generation tasks.
It is introduced in the paper:
[CodeT5+: Open Code Large Language Models for Code Understanding and Generation](https://arxiv.org/pdf/2305.07922.pdf)
by [Yue Wang](https://yuewang-cuhk.github.io/)\*, [Hung Le](https://sites.google.com/view/henryle2018/home?pli=1)\*, [Akhilesh Deepak Gotmare](https://akhileshgotmare.github.io/), [Nghi D.Q. Bui](https://bdqnghi.github.io/), [Junnan Li](https://sites.google.com/site/junnanlics), [Steven C.H. Hoi](https://sites.google.com/view/stevenhoi/home) (* indicates equal contribution).
Compared to the original CodeT5 family (CodeT5-base: `220M`, CodeT5-large: `770M`), CodeT5+ is pretrained with a diverse set of pretraining tasks including _span denoising_, _causal language modeling_, _contrastive learning_, and _text-code matching_ to learn rich representations from both unimodal code data and bimodal code-text data.
Additionally, it employs a simple yet effective _compute-efficient pretraining_ method to initialize the model components with frozen off-the-shelf LLMs such as [CodeGen](https://github.com/salesforce/CodeGen) to efficiently scale up the model (i.e. `2B`, `6B`, `16B`), and adopts a "shallow encoder and deep decoder" architecture.
Furthermore, it is instruction-tuned to align with natural language instructions (see our InstructCodeT5+ 16B) following [Code Alpaca](https://github.com/sahil280114/codealpaca).
## How to use
This model can be easily loaded using the `T5ForConditionalGeneration` functionality and employs the same tokenizer as original [CodeT5](https://github.com/salesforce/CodeT5).
```python
from transformers import T5ForConditionalGeneration, AutoTokenizer
checkpoint = "Salesforce/codet5p-770m"
device = "cuda" # for GPU usage or "cpu" for CPU usage
tokenizer = AutoTokenizer.from_pretrained(checkpoint)
model = T5ForConditionalGeneration.from_pretrained(checkpoint).to(device)
inputs = tokenizer.encode("def print_hello_world():<extra_id_0>", return_tensors="pt").to(device)
outputs = model.generate(inputs, max_length=10)
print(tokenizer.decode(outputs[0], skip_special_tokens=True))
# ==> print "Hello World"
```
## Pretraining data
This checkpoint is trained on the stricter permissive subset of the deduplicated version of the [github-code dataset](https://huggingface.co/datasets/codeparrot/github-code).
The data is preprocessed by reserving only permissively licensed code ("mit" “apache-2”, “bsd-3-clause”, “bsd-2-clause”, “cc0-1.0”, “unlicense”, “isc”).
Supported languages (9 in total) are as follows:
`c`, `c++`, `c-sharp`, `go`, `java`, `javascript`, `php`, `python`, `ruby.`
## Training procedure
This checkpoint is trained on the unimodal code data at the first-stage pretraining, which includes a diverse set of pretraining tasks including _span denoising_ and two variants of _causal language modeling_.
Please refer to the paper for more details.
## Evaluation results
CodeT5+ models have been comprehensively evaluated on a wide range of code understanding and generation tasks in various settings: _zero-shot_, _finetuning_, and _instruction-tuning_.
Specifically, CodeT5+ yields substantial performance gains on many downstream tasks compared to their SoTA baselines, e.g.,
8 text-to-code retrieval tasks (+3.2 avg. MRR), 2 line-level code completion tasks (+2.1 avg. Exact Match), and 2 retrieval-augmented code generation tasks (+5.8 avg. BLEU-4).
In 2 math programming tasks on MathQA-Python and GSM8K-Python, CodeT5+ models of below billion-parameter sizes significantly outperform many LLMs of up to 137B parameters.
Particularly, in the zero-shot text-to-code generation task on HumanEval benchmark, InstructCodeT5+ 16B sets new SoTA results of 35.0% pass@1 and 54.5% pass@10 against other open code LLMs, even surpassing the closed-source OpenAI code-cushman-001 mode
Please refer to the [paper](https://arxiv.org/pdf/2305.07922.pdf) for more details.
## BibTeX entry and citation info
```bibtex
@article{wang2023codet5plus,
title={CodeT5+: Open Code Large Language Models for Code Understanding and Generation},
author={Wang, Yue and Le, Hung and Gotmare, Akhilesh Deepak and Bui, Nghi D.Q. and Li, Junnan and Hoi, Steven C. H.},
journal={arXiv preprint},
year={2023}
}
``` |
artificialguybr/ColoringBookRedmond-V2 | artificialguybr | "2023-10-07T20:57:38Z" | 106,780 | 19 | diffusers | [
"diffusers",
"text-to-image",
"stable-diffusion",
"lora",
"base_model:stabilityai/stable-diffusion-xl-base-1.0",
"license:creativeml-openrail-m",
"region:us"
] | text-to-image | "2023-10-07T20:54:11Z" | ---
license: creativeml-openrail-m
tags:
- text-to-image
- stable-diffusion
- lora
- diffusers
base_model: stabilityai/stable-diffusion-xl-base-1.0
instance_prompt: ColoringBookAF, Coloring Book
widget:
- text: ColoringBookAF, Coloring Book
---
# ColoringBook.Redmond V2

ColoringBook.Redmond is here!
TEST ALL MY LORA HERE|: https://huggingface.co/spaces/artificialguybr/artificialguybr-demo-lora/
Introducing ColoringBook.Redmond, the ultimate LORA for creating Coloring Book images!
I'm grateful for the GPU time from Redmond.AI that allowed me to make this LORA! If you need GPU, then you need the great services from Redmond.AI.
It is based on SD XL 1.0 and fine-tuned on a large dataset.
The LORA has a high capacity to generate Coloring Book Images!
The tag for the model:ColoringBookAF, Coloring Book
I really hope you like the LORA and use it.
If you like the model and think it's worth it, you can make a donation to my Patreon or Ko-fi.
Patreon:
https://www.patreon.com/user?u=81570187
Ko-fi:https://ko-fi.com/artificialguybr
BuyMeACoffe:https://www.buymeacoffee.com/jvkape
Follow me in my twitter to know before all about new models:
https://twitter.com/artificialguybr/ |
prithivida/grammar_error_correcter_v1 | prithivida | "2021-07-04T10:44:31Z" | 106,743 | 35 | transformers | [
"transformers",
"pytorch",
"t5",
"text2text-generation",
"autotrain_compatible",
"endpoints_compatible",
"text-generation-inference",
"region:us"
] | text2text-generation | "2022-03-02T23:29:05Z" | **This model is part of the Gramformer library** please refer to https://github.com/PrithivirajDamodaran/Gramformer/
|
s-nlp/roberta_toxicity_classifier | s-nlp | "2021-10-05T14:54:55Z" | 105,966 | 43 | transformers | [
"transformers",
"pytorch",
"roberta",
"text-classification",
"toxic comments classification",
"en",
"arxiv:1907.11692",
"autotrain_compatible",
"endpoints_compatible",
"region:us"
] | text-classification | "2022-03-02T23:29:05Z" | ---
language:
- en
tags:
- toxic comments classification
licenses:
- cc-by-nc-sa
---
## Toxicity Classification Model
This model is trained for toxicity classification task. The dataset used for training is the merge of the English parts of the three datasets by **Jigsaw** ([Jigsaw 2018](https://www.kaggle.com/c/jigsaw-toxic-comment-classification-challenge), [Jigsaw 2019](https://www.kaggle.com/c/jigsaw-unintended-bias-in-toxicity-classification), [Jigsaw 2020](https://www.kaggle.com/c/jigsaw-multilingual-toxic-comment-classification)), containing around 2 million examples. We split it into two parts and fine-tune a RoBERTa model ([RoBERTa: A Robustly Optimized BERT Pretraining Approach](https://arxiv.org/abs/1907.11692)) on it. The classifiers perform closely on the test set of the first Jigsaw competition, reaching the **AUC-ROC** of 0.98 and **F1-score** of 0.76.
## How to use
```python
from transformers import RobertaTokenizer, RobertaForSequenceClassification
# load tokenizer and model weights
tokenizer = RobertaTokenizer.from_pretrained('SkolkovoInstitute/roberta_toxicity_classifier')
model = RobertaForSequenceClassification.from_pretrained('SkolkovoInstitute/roberta_toxicity_classifier')
# prepare the input
batch = tokenizer.encode('you are amazing', return_tensors='pt')
# inference
model(batch)
```
## Licensing Information
[Creative Commons Attribution-NonCommercial-ShareAlike 4.0 International License][cc-by-nc-sa].
[![CC BY-NC-SA 4.0][cc-by-nc-sa-image]][cc-by-nc-sa]
[cc-by-nc-sa]: http://creativecommons.org/licenses/by-nc-sa/4.0/
[cc-by-nc-sa-image]: https://i.creativecommons.org/l/by-nc-sa/4.0/88x31.png |
emrecan/bert-base-turkish-cased-mean-nli-stsb-tr | emrecan | "2022-01-24T23:55:40Z" | 105,840 | 23 | sentence-transformers | [
"sentence-transformers",
"pytorch",
"bert",
"feature-extraction",
"sentence-similarity",
"transformers",
"tr",
"dataset:nli_tr",
"dataset:emrecan/stsb-mt-turkish",
"license:apache-2.0",
"autotrain_compatible",
"endpoints_compatible",
"text-embeddings-inference",
"region:us"
] | sentence-similarity | "2022-03-02T23:29:05Z" | ---
language:
- tr
pipeline_tag: sentence-similarity
license: apache-2.0
tags:
- sentence-transformers
- feature-extraction
- sentence-similarity
- transformers
datasets:
- nli_tr
- emrecan/stsb-mt-turkish
widget:
source_sentence: "Bu çok mutlu bir kişi"
sentences:
- "Bu mutlu bir köpek"
- "Bu sevincinden havalara uçan bir insan"
- "Çok kar yağıyor"
---
# emrecan/bert-base-turkish-cased-mean-nli-stsb-tr
This is a [sentence-transformers](https://www.SBERT.net) model: It maps sentences & paragraphs to a 768 dimensional dense vector space and can be used for tasks like clustering or semantic search. The model was trained on Turkish machine translated versions of [NLI](https://huggingface.co/datasets/nli_tr) and [STS-b](https://huggingface.co/datasets/emrecan/stsb-mt-turkish) datasets, using example [training scripts]( https://github.com/UKPLab/sentence-transformers/tree/master/examples/training) from sentence-transformers GitHub repository.
## Usage (Sentence-Transformers)
Using this model becomes easy when you have [sentence-transformers](https://www.SBERT.net) installed:
```
pip install -U sentence-transformers
```
Then you can use the model like this:
```python
from sentence_transformers import SentenceTransformer
sentences = ["Bu örnek bir cümle", "Her cümle vektöre çevriliyor"]
model = SentenceTransformer('emrecan/bert-base-turkish-cased-mean-nli-stsb-tr')
embeddings = model.encode(sentences)
print(embeddings)
```
## Usage (HuggingFace Transformers)
Without [sentence-transformers](https://www.SBERT.net), you can use the model like this: First, you pass your input through the transformer model, then you have to apply the right pooling-operation on-top of the contextualized word embeddings.
```python
from transformers import AutoTokenizer, AutoModel
import torch
#Mean Pooling - Take attention mask into account for correct averaging
def mean_pooling(model_output, attention_mask):
token_embeddings = model_output[0] #First element of model_output contains all token embeddings
input_mask_expanded = attention_mask.unsqueeze(-1).expand(token_embeddings.size()).float()
return torch.sum(token_embeddings * input_mask_expanded, 1) / torch.clamp(input_mask_expanded.sum(1), min=1e-9)
# Sentences we want sentence embeddings for
sentences = ["Bu örnek bir cümle", "Her cümle vektöre çevriliyor"]
# Load model from HuggingFace Hub
tokenizer = AutoTokenizer.from_pretrained('emrecan/bert-base-turkish-cased-mean-nli-stsb-tr')
model = AutoModel.from_pretrained('emrecan/bert-base-turkish-cased-mean-nli-stsb-tr')
# Tokenize sentences
encoded_input = tokenizer(sentences, padding=True, truncation=True, return_tensors='pt')
# Compute token embeddings
with torch.no_grad():
model_output = model(**encoded_input)
# Perform pooling. In this case, mean pooling.
sentence_embeddings = mean_pooling(model_output, encoded_input['attention_mask'])
print("Sentence embeddings:")
print(sentence_embeddings)
```
## Evaluation Results
Evaluation results on test and development sets are given below:
| Split | Epoch | cosine_pearson | cosine_spearman | euclidean_pearson | euclidean_spearman | manhattan_pearson | manhattan_spearman | dot_pearson | dot_spearman |
|------------|-------|----------------|-----------------|-------------------|--------------------|-------------------|--------------------|-------------|--------------|
| test | - | 0.834 | 0.830 | 0.820 | 0.819 | 0.819 | 0.818 | 0.799 | 0.789 |
| validation | 1 | 0.850 | 0.848 | 0.831 | 0.835 | 0.83 | 0.83 | 0.80 | 0.806 |
| validation | 2 | 0.857 | 0.857 | 0.844 | 0.848 | 0.844 | 0.848 | 0.813 | 0.810 |
| validation | 3 | 0.860 | 0.859 | 0.846 | 0.851 | 0.846 | 0.850 | 0.825 | 0.822 |
| validation | 4 | 0.859 | 0.860 | 0.846 | 0.851 | 0.846 | 0.851 | 0.825 | 0.823 |
## Training
Training scripts [`training_nli_v2.py`](https://github.com/UKPLab/sentence-transformers/blob/master/examples/training/nli/training_nli_v2.py) and [`training_stsbenchmark_continue_training.py`](https://github.com/UKPLab/sentence-transformers/blob/master/examples/training/sts/training_stsbenchmark_continue_training.py) were used to train the model.
The model was trained with the parameters:
**DataLoader**:
`torch.utils.data.dataloader.DataLoader` of length 360 with parameters:
```
{'batch_size': 16, 'sampler': 'torch.utils.data.sampler.RandomSampler', 'batch_sampler': 'torch.utils.data.sampler.BatchSampler'}
```
**Loss**:
`sentence_transformers.losses.CosineSimilarityLoss.CosineSimilarityLoss`
Parameters of the fit()-Method:
```
{
"epochs": 4,
"evaluation_steps": 200,
"evaluator": "sentence_transformers.evaluation.EmbeddingSimilarityEvaluator.EmbeddingSimilarityEvaluator",
"max_grad_norm": 1,
"optimizer_class": "<class 'transformers.optimization.AdamW'>",
"optimizer_params": {
"lr": 2e-05
},
"scheduler": "WarmupLinear",
"steps_per_epoch": null,
"warmup_steps": 144,
"weight_decay": 0.01
}
```
## Full Model Architecture
```
SentenceTransformer(
(0): Transformer({'max_seq_length': 75, 'do_lower_case': False}) with Transformer model: BertModel
(1): Pooling({'word_embedding_dimension': 768, 'pooling_mode_cls_token': False, 'pooling_mode_mean_tokens': True, 'pooling_mode_max_tokens': False, 'pooling_mode_mean_sqrt_len_tokens': False})
)
```
## Citing & Authors
<!--- Describe where people can find more information --> |
mradermacher/Llama-3-70b-Arimas-story-RP-V2.1-i1-GGUF | mradermacher | "2024-07-02T23:10:18Z" | 105,702 | 1 | transformers | [
"transformers",
"gguf",
"mergekit",
"merge",
"llama 3",
"Model stock",
"en",
"base_model:ryzen88/Llama-3-70b-Arimas-story-RP-V2.1",
"endpoints_compatible",
"region:us"
] | null | "2024-06-25T22:00:14Z" | ---
base_model: ryzen88/Llama-3-70b-Arimas-story-RP-V2.1
language:
- en
library_name: transformers
quantized_by: mradermacher
tags:
- mergekit
- merge
- llama 3
- Model stock
---
## About
<!-- ### quantize_version: 2 -->
<!-- ### output_tensor_quantised: 1 -->
<!-- ### convert_type: hf -->
<!-- ### vocab_type: -->
<!-- ### tags: nicoboss -->
weighted/imatrix quants of https://huggingface.co/ryzen88/Llama-3-70b-Arimas-story-RP-V2.1
<!-- provided-files -->
static quants are available at https://huggingface.co/mradermacher/Llama-3-70b-Arimas-story-RP-V2.1-GGUF
## Usage
If you are unsure how to use GGUF files, refer to one of [TheBloke's
READMEs](https://huggingface.co/TheBloke/KafkaLM-70B-German-V0.1-GGUF) for
more details, including on how to concatenate multi-part files.
## Provided Quants
(sorted by size, not necessarily quality. IQ-quants are often preferable over similar sized non-IQ quants)
| Link | Type | Size/GB | Notes |
|:-----|:-----|--------:|:------|
| [GGUF](https://huggingface.co/mradermacher/Llama-3-70b-Arimas-story-RP-V2.1-i1-GGUF/resolve/main/Llama-3-70b-Arimas-story-RP-V2.1.i1-IQ1_S.gguf) | i1-IQ1_S | 15.4 | for the desperate |
| [GGUF](https://huggingface.co/mradermacher/Llama-3-70b-Arimas-story-RP-V2.1-i1-GGUF/resolve/main/Llama-3-70b-Arimas-story-RP-V2.1.i1-IQ1_M.gguf) | i1-IQ1_M | 16.9 | mostly desperate |
| [GGUF](https://huggingface.co/mradermacher/Llama-3-70b-Arimas-story-RP-V2.1-i1-GGUF/resolve/main/Llama-3-70b-Arimas-story-RP-V2.1.i1-IQ2_XXS.gguf) | i1-IQ2_XXS | 19.2 | |
| [GGUF](https://huggingface.co/mradermacher/Llama-3-70b-Arimas-story-RP-V2.1-i1-GGUF/resolve/main/Llama-3-70b-Arimas-story-RP-V2.1.i1-IQ2_XS.gguf) | i1-IQ2_XS | 21.2 | |
| [GGUF](https://huggingface.co/mradermacher/Llama-3-70b-Arimas-story-RP-V2.1-i1-GGUF/resolve/main/Llama-3-70b-Arimas-story-RP-V2.1.i1-IQ2_S.gguf) | i1-IQ2_S | 22.3 | |
| [GGUF](https://huggingface.co/mradermacher/Llama-3-70b-Arimas-story-RP-V2.1-i1-GGUF/resolve/main/Llama-3-70b-Arimas-story-RP-V2.1.i1-IQ2_M.gguf) | i1-IQ2_M | 24.2 | |
| [GGUF](https://huggingface.co/mradermacher/Llama-3-70b-Arimas-story-RP-V2.1-i1-GGUF/resolve/main/Llama-3-70b-Arimas-story-RP-V2.1.i1-Q2_K.gguf) | i1-Q2_K | 26.5 | IQ3_XXS probably better |
| [GGUF](https://huggingface.co/mradermacher/Llama-3-70b-Arimas-story-RP-V2.1-i1-GGUF/resolve/main/Llama-3-70b-Arimas-story-RP-V2.1.i1-IQ3_XXS.gguf) | i1-IQ3_XXS | 27.6 | lower quality |
| [GGUF](https://huggingface.co/mradermacher/Llama-3-70b-Arimas-story-RP-V2.1-i1-GGUF/resolve/main/Llama-3-70b-Arimas-story-RP-V2.1.i1-IQ3_XS.gguf) | i1-IQ3_XS | 29.4 | |
| [GGUF](https://huggingface.co/mradermacher/Llama-3-70b-Arimas-story-RP-V2.1-i1-GGUF/resolve/main/Llama-3-70b-Arimas-story-RP-V2.1.i1-IQ3_S.gguf) | i1-IQ3_S | 31.0 | beats Q3_K* |
| [GGUF](https://huggingface.co/mradermacher/Llama-3-70b-Arimas-story-RP-V2.1-i1-GGUF/resolve/main/Llama-3-70b-Arimas-story-RP-V2.1.i1-Q3_K_S.gguf) | i1-Q3_K_S | 31.0 | IQ3_XS probably better |
| [GGUF](https://huggingface.co/mradermacher/Llama-3-70b-Arimas-story-RP-V2.1-i1-GGUF/resolve/main/Llama-3-70b-Arimas-story-RP-V2.1.i1-IQ3_M.gguf) | i1-IQ3_M | 32.0 | |
| [GGUF](https://huggingface.co/mradermacher/Llama-3-70b-Arimas-story-RP-V2.1-i1-GGUF/resolve/main/Llama-3-70b-Arimas-story-RP-V2.1.i1-Q3_K_M.gguf) | i1-Q3_K_M | 34.4 | IQ3_S probably better |
| [GGUF](https://huggingface.co/mradermacher/Llama-3-70b-Arimas-story-RP-V2.1-i1-GGUF/resolve/main/Llama-3-70b-Arimas-story-RP-V2.1.i1-Q3_K_L.gguf) | i1-Q3_K_L | 37.2 | IQ3_M probably better |
| [GGUF](https://huggingface.co/mradermacher/Llama-3-70b-Arimas-story-RP-V2.1-i1-GGUF/resolve/main/Llama-3-70b-Arimas-story-RP-V2.1.i1-IQ4_XS.gguf) | i1-IQ4_XS | 38.0 | |
| [GGUF](https://huggingface.co/mradermacher/Llama-3-70b-Arimas-story-RP-V2.1-i1-GGUF/resolve/main/Llama-3-70b-Arimas-story-RP-V2.1.i1-Q4_0.gguf) | i1-Q4_0 | 40.2 | fast, low quality |
| [GGUF](https://huggingface.co/mradermacher/Llama-3-70b-Arimas-story-RP-V2.1-i1-GGUF/resolve/main/Llama-3-70b-Arimas-story-RP-V2.1.i1-Q4_K_S.gguf) | i1-Q4_K_S | 40.4 | optimal size/speed/quality |
| [GGUF](https://huggingface.co/mradermacher/Llama-3-70b-Arimas-story-RP-V2.1-i1-GGUF/resolve/main/Llama-3-70b-Arimas-story-RP-V2.1.i1-Q4_K_M.gguf) | i1-Q4_K_M | 42.6 | fast, recommended |
| [GGUF](https://huggingface.co/mradermacher/Llama-3-70b-Arimas-story-RP-V2.1-i1-GGUF/resolve/main/Llama-3-70b-Arimas-story-RP-V2.1.i1-Q5_K_S.gguf) | i1-Q5_K_S | 48.8 | |
| [GGUF](https://huggingface.co/mradermacher/Llama-3-70b-Arimas-story-RP-V2.1-i1-GGUF/resolve/main/Llama-3-70b-Arimas-story-RP-V2.1.i1-Q5_K_M.gguf) | i1-Q5_K_M | 50.0 | |
| [PART 1](https://huggingface.co/mradermacher/Llama-3-70b-Arimas-story-RP-V2.1-i1-GGUF/resolve/main/Llama-3-70b-Arimas-story-RP-V2.1.i1-Q6_K.gguf.part1of2) [PART 2](https://huggingface.co/mradermacher/Llama-3-70b-Arimas-story-RP-V2.1-i1-GGUF/resolve/main/Llama-3-70b-Arimas-story-RP-V2.1.i1-Q6_K.gguf.part2of2) | i1-Q6_K | 58.0 | practically like static Q6_K |
Here is a handy graph by ikawrakow comparing some lower-quality quant
types (lower is better):

And here are Artefact2's thoughts on the matter:
https://gist.github.com/Artefact2/b5f810600771265fc1e39442288e8ec9
## FAQ / Model Request
See https://huggingface.co/mradermacher/model_requests for some answers to
questions you might have and/or if you want some other model quantized.
## Thanks
I thank my company, [nethype GmbH](https://www.nethype.de/), for letting
me use its servers and providing upgrades to my workstation to enable
this work in my free time. Additional thanks to [@nicoboss](https://huggingface.co/nicoboss) for giving me access to his hardware for calculating the imatrix for these quants.
<!-- end -->
|
monster-labs/control_v1p_sd15_qrcode_monster | monster-labs | "2023-07-21T11:35:31Z" | 105,635 | 1,271 | diffusers | [
"diffusers",
"safetensors",
"stable-diffusion",
"controlnet",
"qrcode",
"en",
"license:openrail++",
"region:us"
] | null | "2023-06-24T15:07:20Z" | ---
tags:
- stable-diffusion
- controlnet
- qrcode
license: openrail++
language:
- en
---
# Controlnet QR Code Monster v2 For SD-1.5

## Model Description
This model is made to generate creative QR codes that still scan.
Keep in mind that not all generated codes might be readable, but you can try different parameters and prompts to get the desired results.
**NEW VERSION**
Introducing the upgraded version of our model - Controlnet QR code Monster v2.
V2 is a huge upgrade over v1, for scannability AND creativity.
QR codes can now seamlessly blend the image by using a gray-colored background (#808080).
As with the former version, the readability of some generated codes may vary, however playing around with parameters and prompts could yield better results.
You can find in in the `v2/` subfolder.
## How to Use
- **Condition**: QR codes are passed as condition images with a module size of 16px. Use a higher error correction level to make it easier to read (sometimes a lower level can be easier to read if smaller in size). Use a gray background for the rest of the image to make the code integrate better.
- **Prompts**: Use a prompt to guide the QR code generation. The output will highly depend on the given prompt. Some seem to be really easily accepted by the qr code process, some will require careful tweaking to get good results.
- **Controlnet guidance scale**: Set the controlnet guidance scale value:
- High values: The generated QR code will be more readable.
- Low values: The generated QR code will be more creative.
### Tips
- For an optimally readable output, try generating multiple QR codes with similar parameters, then choose the best ones.
- Use the Image-to-Image feature to improve the readability of a generated QR code:
- Decrease the denoising strength to retain more of the original image.
- Increase the controlnet guidance scale value for better readability.
A typical workflow for "saving" a code would be :
Max out the guidance scale and minimize the denoising strength, then bump the strength until the code scans.
## Example Outputs
Here are some examples of creative, yet scannable QR codes produced by our model:



Feel free to experiment with prompts, parameters, and the Image-to-Image feature to achieve the desired QR code output. Good luck and have fun! |
Qwen/Qwen2-0.5B-Instruct | Qwen | "2024-06-06T14:33:10Z" | 105,041 | 77 | transformers | [
"transformers",
"safetensors",
"qwen2",
"text-generation",
"chat",
"conversational",
"en",
"license:apache-2.0",
"autotrain_compatible",
"endpoints_compatible",
"text-generation-inference",
"region:us"
] | text-generation | "2024-06-03T09:06:06Z" | ---
license: apache-2.0
language:
- en
pipeline_tag: text-generation
tags:
- chat
---
# Qwen2-0.5B-Instruct
## Introduction
Qwen2 is the new series of Qwen large language models. For Qwen2, we release a number of base language models and instruction-tuned language models ranging from 0.5 to 72 billion parameters, including a Mixture-of-Experts model. This repo contains the instruction-tuned 0.5B Qwen2 model.
Compared with the state-of-the-art opensource language models, including the previous released Qwen1.5, Qwen2 has generally surpassed most opensource models and demonstrated competitiveness against proprietary models across a series of benchmarks targeting for language understanding, language generation, multilingual capability, coding, mathematics, reasoning, etc.
For more details, please refer to our [blog](https://qwenlm.github.io/blog/qwen2/), [GitHub](https://github.com/QwenLM/Qwen2), and [Documentation](https://qwen.readthedocs.io/en/latest/).
<br>
## Model Details
Qwen2 is a language model series including decoder language models of different model sizes. For each size, we release the base language model and the aligned chat model. It is based on the Transformer architecture with SwiGLU activation, attention QKV bias, group query attention, etc. Additionally, we have an improved tokenizer adaptive to multiple natural languages and codes.
## Training details
We pretrained the models with a large amount of data, and we post-trained the models with both supervised finetuning and direct preference optimization.
## Requirements
The code of Qwen2 has been in the latest Hugging face transformers and we advise you to install `transformers>=4.37.0`, or you might encounter the following error:
```
KeyError: 'qwen2'
```
## Quickstart
Here provides a code snippet with `apply_chat_template` to show you how to load the tokenizer and model and how to generate contents.
```python
from transformers import AutoModelForCausalLM, AutoTokenizer
device = "cuda" # the device to load the model onto
model = AutoModelForCausalLM.from_pretrained(
"Qwen/Qwen2-0.5B-Instruct",
torch_dtype="auto",
device_map="auto"
)
tokenizer = AutoTokenizer.from_pretrained("Qwen/Qwen2-0.5B-Instruct")
prompt = "Give me a short introduction to large language model."
messages = [
{"role": "system", "content": "You are a helpful assistant."},
{"role": "user", "content": prompt}
]
text = tokenizer.apply_chat_template(
messages,
tokenize=False,
add_generation_prompt=True
)
model_inputs = tokenizer([text], return_tensors="pt").to(device)
generated_ids = model.generate(
model_inputs.input_ids,
max_new_tokens=512
)
generated_ids = [
output_ids[len(input_ids):] for input_ids, output_ids in zip(model_inputs.input_ids, generated_ids)
]
response = tokenizer.batch_decode(generated_ids, skip_special_tokens=True)[0]
```
## Evaluation
We briefly compare Qwen2-0.5B-Instruct with Qwen1.5-0.5B-Chat. The results are as follows:
| Datasets | Qwen1.5-0.5B-Chat | **Qwen2-0.5B-Instruct** | Qwen1.5-1.8B-Chat | **Qwen2-1.5B-Instruct** |
| :--- | :---: | :---: | :---: | :---: |
| MMLU | 35.0 | **37.9** | 43.7 | **52.4** |
| HumanEval | 9.1 | **17.1** | 25.0 | **37.8** |
| GSM8K | 11.3 | **40.1** | 35.3 | **61.6** |
| C-Eval | 37.2 | **45.2** | 55.3 | **63.8** |
| IFEval (Prompt Strict-Acc.) | 14.6 | **20.0** | 16.8 | **29.0** |
## Citation
If you find our work helpful, feel free to give us a cite.
```
@article{qwen2,
title={Qwen2 Technical Report},
year={2024}
}
``` |
RichardErkhov/garage-bAInd_-_Platypus2-70B-instruct-gguf | RichardErkhov | "2024-06-28T07:18:06Z" | 105,028 | 0 | null | [
"gguf",
"region:us"
] | null | "2024-06-27T16:30:22Z" | Entry not found |
EleutherAI/gpt-neo-2.7B | EleutherAI | "2023-07-09T15:52:52Z" | 104,911 | 397 | transformers | [
"transformers",
"pytorch",
"jax",
"rust",
"safetensors",
"gpt_neo",
"text-generation",
"text generation",
"causal-lm",
"en",
"dataset:EleutherAI/pile",
"license:mit",
"autotrain_compatible",
"endpoints_compatible",
"region:us"
] | text-generation | "2022-03-02T23:29:04Z" | ---
language:
- en
tags:
- text generation
- pytorch
- causal-lm
license: mit
datasets:
- EleutherAI/pile
---
# GPT-Neo 2.7B
## Model Description
GPT-Neo 2.7B is a transformer model designed using EleutherAI's replication of the GPT-3 architecture. GPT-Neo refers to the class of models, while 2.7B represents the number of parameters of this particular pre-trained model.
## Training data
GPT-Neo 2.7B was trained on the Pile, a large scale curated dataset created by EleutherAI for the purpose of training this model.
## Training procedure
This model was trained for 420 billion tokens over 400,000 steps. It was trained as a masked autoregressive language model, using cross-entropy loss.
## Intended Use and Limitations
This way, the model learns an inner representation of the English language that can then be used to extract features useful for downstream tasks. The model is best at what it was pretrained for however, which is generating texts from a prompt.
### How to use
You can use this model directly with a pipeline for text generation. This example generates a different sequence each time it's run:
```py
>>> from transformers import pipeline
>>> generator = pipeline('text-generation', model='EleutherAI/gpt-neo-2.7B')
>>> generator("EleutherAI has", do_sample=True, min_length=50)
[{'generated_text': 'EleutherAI has made a commitment to create new software packages for each of its major clients and has'}]
```
### Limitations and Biases
GPT-Neo was trained as an autoregressive language model. This means that its core functionality is taking a string of text and predicting the next token. While language models are widely used for tasks other than this, there are a lot of unknowns with this work.
GPT-Neo was trained on the Pile, a dataset known to contain profanity, lewd, and otherwise abrasive language. Depending on your usecase GPT-Neo may produce socially unacceptable text. See Sections 5 and 6 of the Pile paper for a more detailed analysis of the biases in the Pile.
As with all language models, it is hard to predict in advance how GPT-Neo will respond to particular prompts and offensive content may occur without warning. We recommend having a human curate or filter the outputs before releasing them, both to censor undesirable content and to improve the quality of the results.
## Eval results
All evaluations were done using our [evaluation harness](https://github.com/EleutherAI/lm-evaluation-harness). Some results for GPT-2 and GPT-3 are inconsistent with the values reported in the respective papers. We are currently looking into why, and would greatly appreciate feedback and further testing of our eval harness. If you would like to contribute evaluations you have done, please reach out on our [Discord](https://discord.gg/vtRgjbM).
### Linguistic Reasoning
| Model and Size | Pile BPB | Pile PPL | Wikitext PPL | Lambada PPL | Lambada Acc | Winogrande | Hellaswag |
| ---------------- | ---------- | ---------- | ------------- | ----------- | ----------- | ---------- | ----------- |
| GPT-Neo 1.3B | 0.7527 | 6.159 | 13.10 | 7.498 | 57.23% | 55.01% | 38.66% |
| GPT-2 1.5B | 1.0468 | ----- | 17.48 | 10.634 | 51.21% | 59.40% | 40.03% |
| **GPT-Neo 2.7B** | **0.7165** | **5.646** | **11.39** | **5.626** | **62.22%** | **56.50%** | **42.73%** |
| GPT-3 Ada | 0.9631 | ----- | ----- | 9.954 | 51.60% | 52.90% | 35.93% |
### Physical and Scientific Reasoning
| Model and Size | MathQA | PubMedQA | Piqa |
| ---------------- | ---------- | ---------- | ----------- |
| GPT-Neo 1.3B | 24.05% | 54.40% | 71.11% |
| GPT-2 1.5B | 23.64% | 58.33% | 70.78% |
| **GPT-Neo 2.7B** | **24.72%** | **57.54%** | **72.14%** |
| GPT-3 Ada | 24.29% | 52.80% | 68.88% |
### Down-Stream Applications
TBD
### BibTeX entry and citation info
To cite this model, use
```bibtex
@software{gpt-neo,
author = {Black, Sid and
Leo, Gao and
Wang, Phil and
Leahy, Connor and
Biderman, Stella},
title = {{GPT-Neo: Large Scale Autoregressive Language
Modeling with Mesh-Tensorflow}},
month = mar,
year = 2021,
note = {{If you use this software, please cite it using
these metadata.}},
publisher = {Zenodo},
version = {1.0},
doi = {10.5281/zenodo.5297715},
url = {https://doi.org/10.5281/zenodo.5297715}
}
@article{gao2020pile,
title={The Pile: An 800GB Dataset of Diverse Text for Language Modeling},
author={Gao, Leo and Biderman, Stella and Black, Sid and Golding, Laurence and Hoppe, Travis and Foster, Charles and Phang, Jason and He, Horace and Thite, Anish and Nabeshima, Noa and others},
journal={arXiv preprint arXiv:2101.00027},
year={2020}
}
``` |
RichardErkhov/codellama_-_CodeLlama-70b-Python-hf-gguf | RichardErkhov | "2024-06-26T08:38:40Z" | 104,730 | 0 | null | [
"gguf",
"arxiv:2308.12950",
"region:us"
] | null | "2024-06-25T06:46:56Z" | Quantization made by Richard Erkhov.
[Github](https://github.com/RichardErkhov)
[Discord](https://discord.gg/pvy7H8DZMG)
[Request more models](https://github.com/RichardErkhov/quant_request)
CodeLlama-70b-Python-hf - GGUF
- Model creator: https://huggingface.co/codellama/
- Original model: https://huggingface.co/codellama/CodeLlama-70b-Python-hf/
| Name | Quant method | Size |
| ---- | ---- | ---- |
| [CodeLlama-70b-Python-hf.Q2_K.gguf](https://huggingface.co/RichardErkhov/codellama_-_CodeLlama-70b-Python-hf-gguf/blob/main/CodeLlama-70b-Python-hf.Q2_K.gguf) | Q2_K | 23.71GB |
| [CodeLlama-70b-Python-hf.IQ3_XS.gguf](https://huggingface.co/RichardErkhov/codellama_-_CodeLlama-70b-Python-hf-gguf/blob/main/CodeLlama-70b-Python-hf.IQ3_XS.gguf) | IQ3_XS | 26.37GB |
| [CodeLlama-70b-Python-hf.IQ3_S.gguf](https://huggingface.co/RichardErkhov/codellama_-_CodeLlama-70b-Python-hf-gguf/blob/main/CodeLlama-70b-Python-hf.IQ3_S.gguf) | IQ3_S | 27.86GB |
| [CodeLlama-70b-Python-hf.Q3_K_S.gguf](https://huggingface.co/RichardErkhov/codellama_-_CodeLlama-70b-Python-hf-gguf/blob/main/CodeLlama-70b-Python-hf.Q3_K_S.gguf) | Q3_K_S | 27.86GB |
| [CodeLlama-70b-Python-hf.IQ3_M.gguf](https://huggingface.co/RichardErkhov/codellama_-_CodeLlama-70b-Python-hf-gguf/blob/main/CodeLlama-70b-Python-hf.IQ3_M.gguf) | IQ3_M | 28.82GB |
| [CodeLlama-70b-Python-hf.Q3_K.gguf](https://huggingface.co/RichardErkhov/codellama_-_CodeLlama-70b-Python-hf-gguf/blob/main/CodeLlama-70b-Python-hf.Q3_K.gguf) | Q3_K | 30.99GB |
| [CodeLlama-70b-Python-hf.Q3_K_M.gguf](https://huggingface.co/RichardErkhov/codellama_-_CodeLlama-70b-Python-hf-gguf/blob/main/CodeLlama-70b-Python-hf.Q3_K_M.gguf) | Q3_K_M | 30.99GB |
| [CodeLlama-70b-Python-hf.Q3_K_L.gguf](https://huggingface.co/RichardErkhov/codellama_-_CodeLlama-70b-Python-hf-gguf/blob/main/CodeLlama-70b-Python-hf.Q3_K_L.gguf) | Q3_K_L | 33.67GB |
| [CodeLlama-70b-Python-hf.IQ4_XS.gguf](https://huggingface.co/RichardErkhov/codellama_-_CodeLlama-70b-Python-hf-gguf/blob/main/CodeLlama-70b-Python-hf.IQ4_XS.gguf) | IQ4_XS | 34.64GB |
| [CodeLlama-70b-Python-hf.Q4_0.gguf](https://huggingface.co/RichardErkhov/codellama_-_CodeLlama-70b-Python-hf-gguf/blob/main/CodeLlama-70b-Python-hf.Q4_0.gguf) | Q4_0 | 36.2GB |
| [CodeLlama-70b-Python-hf.IQ4_NL.gguf](https://huggingface.co/RichardErkhov/codellama_-_CodeLlama-70b-Python-hf-gguf/blob/main/CodeLlama-70b-Python-hf.IQ4_NL.gguf) | IQ4_NL | 36.55GB |
| [CodeLlama-70b-Python-hf.Q4_K_S.gguf](https://huggingface.co/RichardErkhov/codellama_-_CodeLlama-70b-Python-hf-gguf/blob/main/CodeLlama-70b-Python-hf.Q4_K_S.gguf) | Q4_K_S | 36.55GB |
| [CodeLlama-70b-Python-hf.Q4_K.gguf](https://huggingface.co/RichardErkhov/codellama_-_CodeLlama-70b-Python-hf-gguf/tree/main/) | Q4_K | 38.58GB |
| [CodeLlama-70b-Python-hf.Q4_K_M.gguf](https://huggingface.co/RichardErkhov/codellama_-_CodeLlama-70b-Python-hf-gguf/tree/main/) | Q4_K_M | 38.58GB |
| [CodeLlama-70b-Python-hf.Q4_1.gguf](https://huggingface.co/RichardErkhov/codellama_-_CodeLlama-70b-Python-hf-gguf/tree/main/) | Q4_1 | 40.2GB |
| [CodeLlama-70b-Python-hf.Q5_0.gguf](https://huggingface.co/RichardErkhov/codellama_-_CodeLlama-70b-Python-hf-gguf/tree/main/) | Q5_0 | 44.2GB |
| [CodeLlama-70b-Python-hf.Q5_K_S.gguf](https://huggingface.co/RichardErkhov/codellama_-_CodeLlama-70b-Python-hf-gguf/tree/main/) | Q5_K_S | 44.2GB |
| [CodeLlama-70b-Python-hf.Q5_K.gguf](https://huggingface.co/RichardErkhov/codellama_-_CodeLlama-70b-Python-hf-gguf/tree/main/) | Q5_K | 45.41GB |
| [CodeLlama-70b-Python-hf.Q5_K_M.gguf](https://huggingface.co/RichardErkhov/codellama_-_CodeLlama-70b-Python-hf-gguf/tree/main/) | Q5_K_M | 45.41GB |
| [CodeLlama-70b-Python-hf.Q5_1.gguf](https://huggingface.co/RichardErkhov/codellama_-_CodeLlama-70b-Python-hf-gguf/tree/main/) | Q5_1 | 48.2GB |
| [CodeLlama-70b-Python-hf.Q6_K.gguf](https://huggingface.co/RichardErkhov/codellama_-_CodeLlama-70b-Python-hf-gguf/tree/main/) | Q6_K | 52.7GB |
| [CodeLlama-70b-Python-hf.Q8_0.gguf](https://huggingface.co/RichardErkhov/codellama_-_CodeLlama-70b-Python-hf-gguf/tree/main/) | Q8_0 | 68.26GB |
Original model description:
---
language:
- code
pipeline_tag: text-generation
tags:
- llama-2
license: llama2
---
# **Code Llama**
Code Llama is a collection of pretrained and fine-tuned generative text models ranging in scale from 7 billion to 70 billion parameters. This is the repository for the 70B Python specialist version in the Hugging Face Transformers format. This model is designed for general code synthesis and understanding. Links to other models can be found in the index at the bottom.
> [!NOTE]
> This is a non-official Code Llama repo. You can find the official Meta repository in the [Meta Llama organization](https://huggingface.co/meta-llama/CodeLlama-70b-Python-hf).
| | Base Model | Python | Instruct |
| --- | ----------------------------------------------------------------------------- | ------------------------------------------------------------------------------------------- | ----------------------------------------------------------------------------------------------- |
| 7B | [codellama/CodeLlama-7b-hf](https://huggingface.co/codellama/CodeLlama-7b-hf) | [codellama/CodeLlama-7b-Python-hf](https://huggingface.co/codellama/CodeLlama-7b-Python-hf) | [codellama/CodeLlama-7b-Instruct-hf](https://huggingface.co/codellama/CodeLlama-7b-Instruct-hf) |
| 13B | [codellama/CodeLlama-13b-hf](https://huggingface.co/codellama/CodeLlama-13b-hf) | [codellama/CodeLlama-13b-Python-hf](https://huggingface.co/codellama/CodeLlama-13b-Python-hf) | [codellama/CodeLlama-13b-Instruct-hf](https://huggingface.co/codellama/CodeLlama-13b-Instruct-hf) |
| 34B | [codellama/CodeLlama-34b-hf](https://huggingface.co/codellama/CodeLlama-34b-hf) | [codellama/CodeLlama-34b-Python-hf](https://huggingface.co/codellama/CodeLlama-34b-Python-hf) | [codellama/CodeLlama-34b-Instruct-hf](https://huggingface.co/codellama/CodeLlama-34b-Instruct-hf) |
| 70B | [codellama/CodeLlama-70b-hf](https://huggingface.co/codellama/CodeLlama-70b-hf) | [codellama/CodeLlama-70b-Python-hf](https://huggingface.co/codellama/CodeLlama-70b-Python-hf) | [codellama/CodeLlama-70b-Instruct-hf](https://huggingface.co/codellama/CodeLlama-70b-Instruct-hf) |
## Model Use
To use this model, please make sure to install `transformers`.
```bash
pip install transformers accelerate
```
Model capabilities:
- [x] Code completion.
- [ ] Infilling.
- [ ] Instructions / chat.
- [x] Python specialist.
## Model Details
*Note: Use of this model is governed by the Meta license. Meta developed and publicly released the Code Llama family of large language models (LLMs).
**Model Developers** Meta
**Variations** Code Llama comes in four model sizes, and three variants:
* Code Llama: base models designed for general code synthesis and understanding
* Code Llama - Python: designed specifically for Python
* Code Llama - Instruct: for instruction following and safer deployment
All variants are available in sizes of 7B, 13B, 34B, and 70B parameters.
**This repository contains the Python version of the 70B parameters model.**
**Input** Models input text only.
**Output** Models generate text only.
**Model Architecture** Code Llama is an auto-regressive language model that uses an optimized transformer architecture.
**Model Architecture** Code Llama is an auto-regressive language model that uses an optimized transformer architecture. It was fine-tuned with up to 16k tokens. This variant **does not** support long context of up to 100k tokens.
**Model Dates** Code Llama and its variants have been trained between January 2023 and January 2024.
**Status** This is a static model trained on an offline dataset. Future versions of Code Llama - Instruct will be released as we improve model safety with community feedback.
**License** A custom commercial license is available at: [https://ai.meta.com/resources/models-and-libraries/llama-downloads/](https://ai.meta.com/resources/models-and-libraries/llama-downloads/)
**Research Paper** More information can be found in the paper "[Code Llama: Open Foundation Models for Code](https://ai.meta.com/research/publications/code-llama-open-foundation-models-for-code/)" or its [arXiv page](https://arxiv.org/abs/2308.12950).
## Intended Use
**Intended Use Cases** Code Llama and its variants are intended for commercial and research use in English and relevant programming languages. The base model Code Llama can be adapted for a variety of code synthesis and understanding tasks, Code Llama - Python is designed specifically to handle the Python programming language, and Code Llama - Instruct is intended to be safer to use for code assistant and generation applications.
**Out-of-Scope Uses** Use in any manner that violates applicable laws or regulations (including trade compliance laws). Use in languages other than English. Use in any other way that is prohibited by the Acceptable Use Policy and Licensing Agreement for Code Llama and its variants.
## Hardware and Software
**Training Factors** We used custom training libraries. The training and fine-tuning of the released models have been performed Meta’s Research Super Cluster.
**Carbon Footprint** In aggregate, training all 12 Code Llama models required 1400K GPU hours of computation on hardware of type A100-80GB (TDP of 350-400W). Estimated total emissions were 228.55 tCO2eq, 100% of which were offset by Meta’s sustainability program.
## Evaluation Results
See evaluations for the main models and detailed ablations in Section 3 and safety evaluations in Section 4 of the research paper.
## Ethical Considerations and Limitations
Code Llama and its variants are a new technology that carries risks with use. Testing conducted to date has been in English, and has not covered, nor could it cover all scenarios. For these reasons, as with all LLMs, Code Llama’s potential outputs cannot be predicted in advance, and the model may in some instances produce inaccurate or objectionable responses to user prompts. Therefore, before deploying any applications of Code Llama, developers should perform safety testing and tuning tailored to their specific applications of the model.
Please see the Responsible Use Guide available available at [https://ai.meta.com/llama/responsible-use-guide](https://ai.meta.com/llama/responsible-use-guide).
|
bartowski/Fook-Yi-34B-32K-v1-GGUF | bartowski | "2024-06-29T19:23:40Z" | 104,298 | 0 | null | [
"gguf",
"not-for-all-audiences",
"text-generation",
"license:cc-by-nc-4.0",
"region:us"
] | text-generation | "2024-06-29T17:33:23Z" | ---
license: cc-by-nc-4.0
tags:
- not-for-all-audiences
quantized_by: bartowski
pipeline_tag: text-generation
---
## Llamacpp imatrix Quantizations of Fook-Yi-34B-32K-v1
Using <a href="https://github.com/ggerganov/llama.cpp/">llama.cpp</a> release <a href="https://github.com/ggerganov/llama.cpp/releases/tag/b3259">b3259</a> for quantization.
Original model: https://huggingface.co/TheDrummer/Fook-Yi-34B-32K-v1
All quants made using imatrix option with dataset from [here](https://gist.github.com/bartowski1182/eb213dccb3571f863da82e99418f81e8)
## Prompt format
```
<|im_start|> system
{system_prompt}<|im_end|>
<|im_start|> user
{prompt}<|im_end|>
<|im_start|> assistant
```
## Download a file (not the whole branch) from below:
| Filename | Quant type | File Size | Description |
| -------- | ---------- | --------- | ----------- |
| [Fook-Yi-34B-32K-v1-Q8_0_L.gguf](https://huggingface.co/bartowski/Fook-Yi-34B-32K-v1-GGUF/blob/main/Fook-Yi-34B-32K-v1-Q8_1.gguf) | Q8_0_L | 37.40GB | *Experimental*, uses f16 for embed and output weights. Please provide any feedback of differences. Extremely high quality, generally unneeded but max available quant. |
| [Fook-Yi-34B-32K-v1-Q8_0.gguf](https://huggingface.co/bartowski/Fook-Yi-34B-32K-v1-GGUF/blob/main/Fook-Yi-34B-32K-v1-Q8_0.gguf) | Q8_0 | 36.54GB | Extremely high quality, generally unneeded but max available quant. |
| [Fook-Yi-34B-32K-v1-Q6_K_L.gguf](https://huggingface.co/bartowski/Fook-Yi-34B-32K-v1-GGUF/blob/main/Fook-Yi-34B-32K-v1-Q6_K_L.gguf) | Q6_K_L | 29.29GB | *Experimental*, uses f16 for embed and output weights. Please provide any feedback of differences. Very high quality, near perfect, *recommended*. |
| [Fook-Yi-34B-32K-v1-Q6_K.gguf](https://huggingface.co/bartowski/Fook-Yi-34B-32K-v1-GGUF/blob/main/Fook-Yi-34B-32K-v1-Q6_K.gguf) | Q6_K | 28.21GB | Very high quality, near perfect, *recommended*. |
| [Fook-Yi-34B-32K-v1-Q5_K_L.gguf](https://huggingface.co/bartowski/Fook-Yi-34B-32K-v1-GGUF/blob/main/Fook-Yi-34B-32K-v1-Q5_K_L.gguf) | Q5_K_L | 25.46GB | *Experimental*, uses f16 for embed and output weights. Please provide any feedback of differences. High quality, *recommended*. |
| [Fook-Yi-34B-32K-v1-Q5_K_M.gguf](https://huggingface.co/bartowski/Fook-Yi-34B-32K-v1-GGUF/blob/main/Fook-Yi-34B-32K-v1-Q5_K_M.gguf) | Q5_K_M | 24.32GB | High quality, *recommended*. |
| [Fook-Yi-34B-32K-v1-Q5_K_S.gguf](https://huggingface.co/bartowski/Fook-Yi-34B-32K-v1-GGUF/blob/main/Fook-Yi-34B-32K-v1-Q5_K_S.gguf) | Q5_K_S | 23.70GB | High quality, *recommended*. |
| [Fook-Yi-34B-32K-v1-Q4_K_L.gguf](https://huggingface.co/bartowski/Fook-Yi-34B-32K-v1-GGUF/blob/main/Fook-Yi-34B-32K-v1-Q4_K_L.gguf) | Q4_K_L | 21.85GB | *Experimental*, uses f16 for embed and output weights. Please provide any feedback of differences. Good quality, uses about 4.83 bits per weight, *recommended*. |
| [Fook-Yi-34B-32K-v1-Q4_K_M.gguf](https://huggingface.co/bartowski/Fook-Yi-34B-32K-v1-GGUF/blob/main/Fook-Yi-34B-32K-v1-Q4_K_M.gguf) | Q4_K_M | 20.65GB | Good quality, uses about 4.83 bits per weight, *recommended*. |
| [Fook-Yi-34B-32K-v1-Q4_K_S.gguf](https://huggingface.co/bartowski/Fook-Yi-34B-32K-v1-GGUF/blob/main/Fook-Yi-34B-32K-v1-Q4_K_S.gguf) | Q4_K_S | 19.59GB | Slightly lower quality with more space savings, *recommended*. |
| [Fook-Yi-34B-32K-v1-IQ4_XS.gguf](https://huggingface.co/bartowski/Fook-Yi-34B-32K-v1-GGUF/blob/main/Fook-Yi-34B-32K-v1-IQ4_XS.gguf) | IQ4_XS | 18.47GB | Decent quality, smaller than Q4_K_S with similar performance, *recommended*. |
| [Fook-Yi-34B-32K-v1-Q3_K_XL.gguf](https://huggingface.co/bartowski/Fook-Yi-34B-32K-v1-GGUF/blob/main/Fook-Yi-34B-32K-v1-Q3_K_XL.gguf) | Q3_K_XL | 19.40GB | *Experimental*, uses f16 for embed and output weights. Please provide any feedback of differences. Lower quality but usable, good for low RAM availability. |
| [Fook-Yi-34B-32K-v1-Q3_K_L.gguf](https://huggingface.co/bartowski/Fook-Yi-34B-32K-v1-GGUF/blob/main/Fook-Yi-34B-32K-v1-Q3_K_L.gguf) | Q3_K_L | 18.13GB | Lower quality but usable, good for low RAM availability. |
| [Fook-Yi-34B-32K-v1-Q3_K_M.gguf](https://huggingface.co/bartowski/Fook-Yi-34B-32K-v1-GGUF/blob/main/Fook-Yi-34B-32K-v1-Q3_K_M.gguf) | Q3_K_M | 16.65GB | Even lower quality. |
| [Fook-Yi-34B-32K-v1-IQ3_M.gguf](https://huggingface.co/bartowski/Fook-Yi-34B-32K-v1-GGUF/blob/main/Fook-Yi-34B-32K-v1-IQ3_M.gguf) | IQ3_M | 15.56GB | Medium-low quality, new method with decent performance comparable to Q3_K_M. |
| [Fook-Yi-34B-32K-v1-Q3_K_S.gguf](https://huggingface.co/bartowski/Fook-Yi-34B-32K-v1-GGUF/blob/main/Fook-Yi-34B-32K-v1-Q3_K_S.gguf) | Q3_K_S | 14.96GB | Low quality, not recommended. |
| [Fook-Yi-34B-32K-v1-IQ3_XS.gguf](https://huggingface.co/bartowski/Fook-Yi-34B-32K-v1-GGUF/blob/main/Fook-Yi-34B-32K-v1-IQ3_XS.gguf) | IQ3_XS | 14.23GB | Lower quality, new method with decent performance, slightly better than Q3_K_S. |
| [Fook-Yi-34B-32K-v1-IQ3_XXS.gguf](https://huggingface.co/bartowski/Fook-Yi-34B-32K-v1-GGUF/blob/main/Fook-Yi-34B-32K-v1-IQ3_XXS.gguf) | IQ3_XXS | 13.33GB | Lower quality, new method with decent performance, comparable to Q3 quants. |
| [Fook-Yi-34B-32K-v1-Q2_K.gguf](https://huggingface.co/bartowski/Fook-Yi-34B-32K-v1-GGUF/blob/main/Fook-Yi-34B-32K-v1-Q2_K.gguf) | Q2_K | 12.82GB | Very low quality but surprisingly usable. |
| [Fook-Yi-34B-32K-v1-IQ2_M.gguf](https://huggingface.co/bartowski/Fook-Yi-34B-32K-v1-GGUF/blob/main/Fook-Yi-34B-32K-v1-IQ2_M.gguf) | IQ2_M | 11.79GB | Very low quality, uses SOTA techniques to also be surprisingly usable. |
| [Fook-Yi-34B-32K-v1-IQ2_S.gguf](https://huggingface.co/bartowski/Fook-Yi-34B-32K-v1-GGUF/blob/main/Fook-Yi-34B-32K-v1-IQ2_S.gguf) | IQ2_S | 10.89GB | Very low quality, uses SOTA techniques to be usable. |
| [Fook-Yi-34B-32K-v1-IQ2_XS.gguf](https://huggingface.co/bartowski/Fook-Yi-34B-32K-v1-GGUF/blob/main/Fook-Yi-34B-32K-v1-IQ2_XS.gguf) | IQ2_XS | 10.30GB | Very low quality, uses SOTA techniques to be usable. |
## Downloading using huggingface-cli
First, make sure you have hugginface-cli installed:
```
pip install -U "huggingface_hub[cli]"
```
Then, you can target the specific file you want:
```
huggingface-cli download bartowski/Fook-Yi-34B-32K-v1-GGUF --include "Fook-Yi-34B-32K-v1-Q4_K_M.gguf" --local-dir ./
```
If the model is bigger than 50GB, it will have been split into multiple files. In order to download them all to a local folder, run:
```
huggingface-cli download bartowski/Fook-Yi-34B-32K-v1-GGUF --include "Fook-Yi-34B-32K-v1-Q8_0.gguf/*" --local-dir Fook-Yi-34B-32K-v1-Q8_0
```
You can either specify a new local-dir (Fook-Yi-34B-32K-v1-Q8_0) or download them all in place (./)
## Which file should I choose?
A great write up with charts showing various performances is provided by Artefact2 [here](https://gist.github.com/Artefact2/b5f810600771265fc1e39442288e8ec9)
The first thing to figure out is how big a model you can run. To do this, you'll need to figure out how much RAM and/or VRAM you have.
If you want your model running as FAST as possible, you'll want to fit the whole thing on your GPU's VRAM. Aim for a quant with a file size 1-2GB smaller than your GPU's total VRAM.
If you want the absolute maximum quality, add both your system RAM and your GPU's VRAM together, then similarly grab a quant with a file size 1-2GB Smaller than that total.
Next, you'll need to decide if you want to use an 'I-quant' or a 'K-quant'.
If you don't want to think too much, grab one of the K-quants. These are in format 'QX_K_X', like Q5_K_M.
If you want to get more into the weeds, you can check out this extremely useful feature chart:
[llama.cpp feature matrix](https://github.com/ggerganov/llama.cpp/wiki/Feature-matrix)
But basically, if you're aiming for below Q4, and you're running cuBLAS (Nvidia) or rocBLAS (AMD), you should look towards the I-quants. These are in format IQX_X, like IQ3_M. These are newer and offer better performance for their size.
These I-quants can also be used on CPU and Apple Metal, but will be slower than their K-quant equivalent, so speed vs performance is a tradeoff you'll have to decide.
The I-quants are *not* compatible with Vulcan, which is also AMD, so if you have an AMD card double check if you're using the rocBLAS build or the Vulcan build. At the time of writing this, LM Studio has a preview with ROCm support, and other inference engines have specific builds for ROCm.
Want to support my work? Visit my ko-fi page here: https://ko-fi.com/bartowski
|
ml6team/keyphrase-extraction-distilbert-inspec | ml6team | "2023-05-06T08:45:37Z" | 104,267 | 24 | transformers | [
"transformers",
"pytorch",
"distilbert",
"token-classification",
"keyphrase-extraction",
"en",
"dataset:midas/inspec",
"license:mit",
"model-index",
"autotrain_compatible",
"endpoints_compatible",
"region:us"
] | token-classification | "2022-03-25T08:52:01Z" | ---
language: en
license: mit
tags:
- keyphrase-extraction
datasets:
- midas/inspec
metrics:
- seqeval
widget:
- text: "Keyphrase extraction is a technique in text analysis where you extract the important keyphrases from a document.
Thanks to these keyphrases humans can understand the content of a text very quickly and easily without reading
it completely. Keyphrase extraction was first done primarily by human annotators, who read the text in detail
and then wrote down the most important keyphrases. The disadvantage is that if you work with a lot of documents,
this process can take a lot of time.
Here is where Artificial Intelligence comes in. Currently, classical machine learning methods, that use statistical
and linguistic features, are widely used for the extraction process. Now with deep learning, it is possible to capture
the semantic meaning of a text even better than these classical methods. Classical methods look at the frequency,
occurrence and order of words in the text, whereas these neural approaches can capture long-term semantic dependencies
and context of words in a text."
example_title: "Example 1"
- text: "In this work, we explore how to learn task specific language models aimed towards learning rich representation of keyphrases from text documents. We experiment with different masking strategies for pre-training transformer language models (LMs) in discriminative as well as generative settings. In the discriminative setting, we introduce a new pre-training objective - Keyphrase Boundary Infilling with Replacement (KBIR), showing large gains in performance (up to 9.26 points in F1) over SOTA, when LM pre-trained using KBIR is fine-tuned for the task of keyphrase extraction. In the generative setting, we introduce a new pre-training setup for BART - KeyBART, that reproduces the keyphrases related to the input text in the CatSeq format, instead of the denoised original input. This also led to gains in performance (up to 4.33 points inF1@M) over SOTA for keyphrase generation. Additionally, we also fine-tune the pre-trained language models on named entity recognition(NER), question answering (QA), relation extraction (RE), abstractive summarization and achieve comparable performance with that of the SOTA, showing that learning rich representation of keyphrases is indeed beneficial for many other fundamental NLP tasks."
example_title: "Example 2"
model-index:
- name: DeDeckerThomas/keyphrase-extraction-distilbert-inspec
results:
- task:
type: keyphrase-extraction
name: Keyphrase Extraction
dataset:
type: midas/inspec
name: inspec
metrics:
- type: F1 (Seqeval)
value: 0.509
name: F1 (Seqeval)
- type: F1@M
value: 0.490
name: F1@M
---
# 🔑 Keyphrase Extraction Model: distilbert-inspec
Keyphrase extraction is a technique in text analysis where you extract the important keyphrases from a document. Thanks to these keyphrases humans can understand the content of a text very quickly and easily without reading it completely. Keyphrase extraction was first done primarily by human annotators, who read the text in detail and then wrote down the most important keyphrases. The disadvantage is that if you work with a lot of documents, this process can take a lot of time ⏳.
Here is where Artificial Intelligence 🤖 comes in. Currently, classical machine learning methods, that use statistical and linguistic features, are widely used for the extraction process. Now with deep learning, it is possible to capture the semantic meaning of a text even better than these classical methods. Classical methods look at the frequency, occurrence and order of words in the text, whereas these neural approaches can capture long-term semantic dependencies and context of words in a text.
## 📓 Model Description
This model uses [distilbert](https://huggingface.co/distilbert-base-uncased) as its base model and fine-tunes it on the [Inspec dataset](https://huggingface.co/datasets/midas/inspec).
Keyphrase extraction models are transformer models fine-tuned as a token classification problem where each word in the document is classified as being part of a keyphrase or not.
| Label | Description |
| ----- | ------------------------------- |
| B-KEY | At the beginning of a keyphrase |
| I-KEY | Inside a keyphrase |
| O | Outside a keyphrase |
Kulkarni, Mayank, Debanjan Mahata, Ravneet Arora, and Rajarshi Bhowmik. "Learning Rich Representation of Keyphrases from Text." arXiv preprint arXiv:2112.08547 (2021).
Sahrawat, Dhruva, Debanjan Mahata, Haimin Zhang, Mayank Kulkarni, Agniv Sharma, Rakesh Gosangi, Amanda Stent, Yaman Kumar, Rajiv Ratn Shah, and Roger Zimmermann. "Keyphrase extraction as sequence labeling using contextualized embeddings." In European Conference on Information Retrieval, pp. 328-335. Springer, Cham, 2020.
## ✋ Intended Uses & Limitations
### 🛑 Limitations
* This keyphrase extraction model is very domain-specific and will perform very well on abstracts of scientific papers. It's not recommended to use this model for other domains, but you are free to test it out.
* Only works for English documents.
### ❓ How To Use
```python
from transformers import (
TokenClassificationPipeline,
AutoModelForTokenClassification,
AutoTokenizer,
)
from transformers.pipelines import AggregationStrategy
import numpy as np
# Define keyphrase extraction pipeline
class KeyphraseExtractionPipeline(TokenClassificationPipeline):
def __init__(self, model, *args, **kwargs):
super().__init__(
model=AutoModelForTokenClassification.from_pretrained(model),
tokenizer=AutoTokenizer.from_pretrained(model),
*args,
**kwargs
)
def postprocess(self, all_outputs):
results = super().postprocess(
all_outputs=all_outputs,
aggregation_strategy=AggregationStrategy.FIRST,
)
return np.unique([result.get("word").strip() for result in results])
```
```python
# Load pipeline
model_name = "ml6team/keyphrase-extraction-distilbert-inspec"
extractor = KeyphraseExtractionPipeline(model=model_name)
```
```python
# Inference
text = """
Keyphrase extraction is a technique in text analysis where you extract the
important keyphrases from a document. Thanks to these keyphrases humans can
understand the content of a text very quickly and easily without reading it
completely. Keyphrase extraction was first done primarily by human annotators,
who read the text in detail and then wrote down the most important keyphrases.
The disadvantage is that if you work with a lot of documents, this process
can take a lot of time.
Here is where Artificial Intelligence comes in. Currently, classical machine
learning methods, that use statistical and linguistic features, are widely used
for the extraction process. Now with deep learning, it is possible to capture
the semantic meaning of a text even better than these classical methods.
Classical methods look at the frequency, occurrence and order of words
in the text, whereas these neural approaches can capture long-term
semantic dependencies and context of words in a text.
""".replace("\n", " ")
keyphrases = extractor(text)
print(keyphrases)
```
```
# Output
['artificial intelligence' 'classical machine learning' 'deep learning'
'keyphrase extraction' 'linguistic features' 'statistical'
'text analysis']
```
## 📚 Training Dataset
[Inspec](https://huggingface.co/datasets/midas/inspec) is a keyphrase extraction/generation dataset consisting of 2000 English scientific papers from the scientific domains of Computers and Control and Information Technology published between 1998 to 2002. The keyphrases are annotated by professional indexers or editors.
You can find more information in the [paper](https://dl.acm.org/doi/10.3115/1119355.1119383).
## 👷♂️ Training Procedure
### Training Parameters
| Parameter | Value |
| --------- | ------|
| Learning Rate | 1e-4 |
| Epochs | 50 |
| Early Stopping Patience | 3 |
### Preprocessing
The documents in the dataset are already preprocessed into list of words with the corresponding labels. The only thing that must be done is tokenization and the realignment of the labels so that they correspond with the right subword tokens.
```python
from datasets import load_dataset
from transformers import AutoTokenizer
# Labels
label_list = ["B", "I", "O"]
lbl2idx = {"B": 0, "I": 1, "O": 2}
idx2label = {0: "B", 1: "I", 2: "O"}
# Tokenizer
tokenizer = AutoTokenizer.from_pretrained("distilbert-base-uncased")
max_length = 512
# Dataset parameters
dataset_full_name = "midas/inspec"
dataset_subset = "raw"
dataset_document_column = "document"
dataset_biotags_column = "doc_bio_tags"
def preprocess_fuction(all_samples_per_split):
tokenized_samples = tokenizer.batch_encode_plus(
all_samples_per_split[dataset_document_column],
padding="max_length",
truncation=True,
is_split_into_words=True,
max_length=max_length,
)
total_adjusted_labels = []
for k in range(0, len(tokenized_samples["input_ids"])):
prev_wid = -1
word_ids_list = tokenized_samples.word_ids(batch_index=k)
existing_label_ids = all_samples_per_split[dataset_biotags_column][k]
i = -1
adjusted_label_ids = []
for wid in word_ids_list:
if wid is None:
adjusted_label_ids.append(lbl2idx["O"])
elif wid != prev_wid:
i = i + 1
adjusted_label_ids.append(lbl2idx[existing_label_ids[i]])
prev_wid = wid
else:
adjusted_label_ids.append(
lbl2idx[
f"{'I' if existing_label_ids[i] == 'B' else existing_label_ids[i]}"
]
)
total_adjusted_labels.append(adjusted_label_ids)
tokenized_samples["labels"] = total_adjusted_labels
return tokenized_samples
# Load dataset
dataset = load_dataset(dataset_full_name, dataset_subset)
# Preprocess dataset
tokenized_dataset = dataset.map(preprocess_fuction, batched=True)
```
### Postprocessing (Without Pipeline Function)
If you do not use the pipeline function, you must filter out the B and I labeled tokens. Each B and I will then be merged into a keyphrase. Finally, you need to strip the keyphrases to make sure all unnecessary spaces have been removed.
```python
# Define post_process functions
def concat_tokens_by_tag(keyphrases):
keyphrase_tokens = []
for id, label in keyphrases:
if label == "B":
keyphrase_tokens.append([id])
elif label == "I":
if len(keyphrase_tokens) > 0:
keyphrase_tokens[len(keyphrase_tokens) - 1].append(id)
return keyphrase_tokens
def extract_keyphrases(example, predictions, tokenizer, index=0):
keyphrases_list = [
(id, idx2label[label])
for id, label in zip(
np.array(example["input_ids"]).squeeze().tolist(), predictions[index]
)
if idx2label[label] in ["B", "I"]
]
processed_keyphrases = concat_tokens_by_tag(keyphrases_list)
extracted_kps = tokenizer.batch_decode(
processed_keyphrases,
skip_special_tokens=True,
clean_up_tokenization_spaces=True,
)
return np.unique([kp.strip() for kp in extracted_kps])
```
## 📝 Evaluation Results
Traditional evaluation methods are the precision, recall and F1-score @k,m where k is the number that stands for the first k predicted keyphrases and m for the average amount of predicted keyphrases.
The model achieves the following results on the Inspec test set:
| Dataset | P@5 | R@5 | F1@5 | P@10 | R@10 | F1@10 | P@M | R@M | F1@M |
|:-----------------:|:----:|:----:|:----:|:----:|:----:|:-----:|:----:|:----:|:----:|
| Inspec Test Set | 0.45 | 0.40 | 0.39 | 0.33 | 0.53 | 0.38 | 0.47 | 0.57 | 0.49 |
## 🚨 Issues
Please feel free to start discussions in the Community Tab. |
speechbrain/lang-id-voxlingua107-ecapa | speechbrain | "2024-02-25T23:48:07Z" | 103,916 | 75 | speechbrain | [
"speechbrain",
"audio-classification",
"embeddings",
"Language",
"Identification",
"pytorch",
"ECAPA-TDNN",
"TDNN",
"VoxLingua107",
"multilingual",
"ab",
"af",
"am",
"ar",
"as",
"az",
"ba",
"be",
"bg",
"bi",
"bo",
"br",
"bs",
"ca",
"ceb",
"cs",
"cy",
"da",
"de",
"el",
"en",
"eo",
"es",
"et",
"eu",
"fa",
"fi",
"fo",
"fr",
"gl",
"gn",
"gu",
"gv",
"ha",
"haw",
"hi",
"hr",
"ht",
"hu",
"hy",
"ia",
"id",
"is",
"it",
"he",
"ja",
"jv",
"ka",
"kk",
"km",
"kn",
"ko",
"la",
"lm",
"ln",
"lo",
"lt",
"lv",
"mg",
"mi",
"mk",
"ml",
"mn",
"mr",
"ms",
"mt",
"my",
"ne",
"nl",
"nn",
"no",
"oc",
"pa",
"pl",
"ps",
"pt",
"ro",
"ru",
"sa",
"sco",
"sd",
"si",
"sk",
"sl",
"sn",
"so",
"sq",
"sr",
"su",
"sv",
"sw",
"ta",
"te",
"tg",
"th",
"tk",
"tl",
"tr",
"tt",
"uk",
"ud",
"uz",
"vi",
"war",
"yi",
"yo",
"zh",
"dataset:VoxLingua107",
"arxiv:2106.04624",
"license:apache-2.0",
"region:us"
] | audio-classification | "2022-03-02T23:29:05Z" | ---
language:
- multilingual
- ab
- af
- am
- ar
- as
- az
- ba
- be
- bg
- bi
- bo
- br
- bs
- ca
- ceb
- cs
- cy
- da
- de
- el
- en
- eo
- es
- et
- eu
- fa
- fi
- fo
- fr
- gl
- gn
- gu
- gv
- ha
- haw
- hi
- hr
- ht
- hu
- hy
- ia
- id
- is
- it
- he
- ja
- jv
- ka
- kk
- km
- kn
- ko
- la
- lm
- ln
- lo
- lt
- lv
- mg
- mi
- mk
- ml
- mn
- mr
- ms
- mt
- my
- ne
- nl
- nn
- no
- oc
- pa
- pl
- ps
- pt
- ro
- ru
- sa
- sco
- sd
- si
- sk
- sl
- sn
- so
- sq
- sr
- su
- sv
- sw
- ta
- te
- tg
- th
- tk
- tl
- tr
- tt
- uk
- ud
- uz
- vi
- war
- yi
- yo
- zh
thumbnail:
tags:
- audio-classification
- speechbrain
- embeddings
- Language
- Identification
- pytorch
- ECAPA-TDNN
- TDNN
- VoxLingua107
license: "apache-2.0"
datasets:
- VoxLingua107
metrics:
- Accuracy
widget:
- example_title: English Sample
src: https://cdn-media.huggingface.co/speech_samples/LibriSpeech_61-70968-0000.flac
---
# VoxLingua107 ECAPA-TDNN Spoken Language Identification Model
## Model description
This is a spoken language recognition model trained on the VoxLingua107 dataset using SpeechBrain.
The model uses the ECAPA-TDNN architecture that has previously been used for speaker recognition. However, it uses
more fully connected hidden layers after the embedding layer, and cross-entropy loss was used for training.
We observed that this improved the performance of extracted utterance embeddings for downstream tasks.
The system is trained with recordings sampled at 16kHz (single channel).
The code will automatically normalize your audio (i.e., resampling + mono channel selection) when calling *classify_file* if needed.
The model can classify a speech utterance according to the language spoken.
It covers 107 different languages (
Abkhazian,
Afrikaans,
Amharic,
Arabic,
Assamese,
Azerbaijani,
Bashkir,
Belarusian,
Bulgarian,
Bengali,
Tibetan,
Breton,
Bosnian,
Catalan,
Cebuano,
Czech,
Welsh,
Danish,
German,
Greek,
English,
Esperanto,
Spanish,
Estonian,
Basque,
Persian,
Finnish,
Faroese,
French,
Galician,
Guarani,
Gujarati,
Manx,
Hausa,
Hawaiian,
Hindi,
Croatian,
Haitian,
Hungarian,
Armenian,
Interlingua,
Indonesian,
Icelandic,
Italian,
Hebrew,
Japanese,
Javanese,
Georgian,
Kazakh,
Central Khmer,
Kannada,
Korean,
Latin,
Luxembourgish,
Lingala,
Lao,
Lithuanian,
Latvian,
Malagasy,
Maori,
Macedonian,
Malayalam,
Mongolian,
Marathi,
Malay,
Maltese,
Burmese,
Nepali,
Dutch,
Norwegian Nynorsk,
Norwegian,
Occitan,
Panjabi,
Polish,
Pushto,
Portuguese,
Romanian,
Russian,
Sanskrit,
Scots,
Sindhi,
Sinhala,
Slovak,
Slovenian,
Shona,
Somali,
Albanian,
Serbian,
Sundanese,
Swedish,
Swahili,
Tamil,
Telugu,
Tajik,
Thai,
Turkmen,
Tagalog,
Turkish,
Tatar,
Ukrainian,
Urdu,
Uzbek,
Vietnamese,
Waray,
Yiddish,
Yoruba,
Mandarin Chinese).
## Intended uses & limitations
The model has two uses:
- use 'as is' for spoken language recognition
- use as an utterance-level feature (embedding) extractor, for creating a dedicated language ID model on your own data
The model is trained on automatically collected YouTube data. For more
information about the dataset, see [here](http://bark.phon.ioc.ee/voxlingua107/).
#### How to use
```bash
pip install git+https://github.com/speechbrain/speechbrain.git@develop
```
```python
import torchaudio
from speechbrain.inference.classifiers import EncoderClassifier
language_id = EncoderClassifier.from_hparams(source="speechbrain/lang-id-voxlingua107-ecapa", savedir="tmp")
# Download Thai language sample from Omniglot and cvert to suitable form
signal = language_id.load_audio("speechbrain/lang-id-voxlingua107-ecapa/udhr_th.wav")
prediction = language_id.classify_batch(signal)
print(prediction)
# (tensor([[-2.8646e+01, -3.0346e+01, -2.0748e+01, -2.9562e+01, -2.2187e+01,
# -3.2668e+01, -3.6677e+01, -3.3573e+01, -3.2545e+01, -2.4365e+01,
# -2.4688e+01, -3.1171e+01, -2.7743e+01, -2.9918e+01, -2.4770e+01,
# -3.2250e+01, -2.4727e+01, -2.6087e+01, -2.1870e+01, -3.2821e+01,
# -2.2128e+01, -2.2822e+01, -3.0888e+01, -3.3564e+01, -2.9906e+01,
# -2.2392e+01, -2.5573e+01, -2.6443e+01, -3.2429e+01, -3.2652e+01,
# -3.0030e+01, -2.4607e+01, -2.2967e+01, -2.4396e+01, -2.8578e+01,
# -2.5153e+01, -2.8475e+01, -2.6409e+01, -2.5230e+01, -2.7957e+01,
# -2.6298e+01, -2.3609e+01, -2.5863e+01, -2.8225e+01, -2.7225e+01,
# -3.0486e+01, -2.1185e+01, -2.7938e+01, -3.3155e+01, -1.9076e+01,
# -2.9181e+01, -2.2160e+01, -1.8352e+01, -2.5866e+01, -3.3636e+01,
# -4.2016e+00, -3.1581e+01, -3.1894e+01, -2.7834e+01, -2.5429e+01,
# -3.2235e+01, -3.2280e+01, -2.8786e+01, -2.3366e+01, -2.6047e+01,
# -2.2075e+01, -2.3770e+01, -2.2518e+01, -2.8101e+01, -2.5745e+01,
# -2.6441e+01, -2.9822e+01, -2.7109e+01, -3.0225e+01, -2.4566e+01,
# -2.9268e+01, -2.7651e+01, -3.4221e+01, -2.9026e+01, -2.6009e+01,
# -3.1968e+01, -3.1747e+01, -2.8156e+01, -2.9025e+01, -2.7756e+01,
# -2.8052e+01, -2.9341e+01, -2.8806e+01, -2.1636e+01, -2.3992e+01,
# -2.3794e+01, -3.3743e+01, -2.8332e+01, -2.7465e+01, -1.5085e-02,
# -2.9094e+01, -2.1444e+01, -2.9780e+01, -3.6046e+01, -3.7401e+01,
# -3.0888e+01, -3.3172e+01, -1.8931e+01, -2.2679e+01, -3.0225e+01,
# -2.4995e+01, -2.1028e+01]]), tensor([-0.0151]), tensor([94]), ['th'])
# The scores in the prediction[0] tensor can be interpreted as log-likelihoods that
# the given utterance belongs to the given language (i.e., the larger the better)
# The linear-scale likelihood can be retrieved using the following:
print(prediction[1].exp())
# tensor([0.9850])
# The identified language ISO code is given in prediction[3]
print(prediction[3])
# ['th: Thai']
# Alternatively, use the utterance embedding extractor:
emb = language_id.encode_batch(signal)
print(emb.shape)
# torch.Size([1, 1, 256])
```
To perform inference on the GPU, add `run_opts={"device":"cuda"}` when calling the `from_hparams` method.
The system is trained with recordings sampled at 16kHz (single channel).
The code will automatically normalize your audio (i.e., resampling + mono channel selection) when calling *classify_file* if needed. Make sure your input tensor is compliant with the expected sampling rate if you use *encode_batch* and *classify_batch*.
#### Limitations and bias
Since the model is trained on VoxLingua107, it has many limitations and biases, some of which are:
- Probably it's accuracy on smaller languages is quite limited
- Probably it works worse on female speech than male speech (because YouTube data includes much more male speech)
- Based on subjective experiments, it doesn't work well on speech with a foreign accent
- Probably it doesn't work well on children's speech and on persons with speech disorders
## Training data
The model is trained on [VoxLingua107](http://bark.phon.ioc.ee/voxlingua107/).
VoxLingua107 is a speech dataset for training spoken language identification models.
The dataset consists of short speech segments automatically extracted from YouTube videos and labeled according the language of the video title and description, with some post-processing steps to filter out false positives.
VoxLingua107 contains data for 107 languages. The total amount of speech in the training set is 6628 hours.
The average amount of data per language is 62 hours. However, the real amount per language varies a lot. There is also a seperate development set containing 1609 speech segments from 33 languages, validated by at least two volunteers to really contain the given language.
## Training procedure
See the [SpeechBrain recipe](https://github.com/speechbrain/speechbrain/tree/voxlingua107/recipes/VoxLingua107/lang_id).
## Evaluation results
Error rate: 6.7% on the VoxLingua107 development dataset
#### Referencing SpeechBrain
```bibtex
@misc{speechbrain,
title={{SpeechBrain}: A General-Purpose Speech Toolkit},
author={Mirco Ravanelli and Titouan Parcollet and Peter Plantinga and Aku Rouhe and Samuele Cornell and Loren Lugosch and Cem Subakan and Nauman Dawalatabad and Abdelwahab Heba and Jianyuan Zhong and Ju-Chieh Chou and Sung-Lin Yeh and Szu-Wei Fu and Chien-Feng Liao and Elena Rastorgueva and François Grondin and William Aris and Hwidong Na and Yan Gao and Renato De Mori and Yoshua Bengio},
year={2021},
eprint={2106.04624},
archivePrefix={arXiv},
primaryClass={eess.AS},
note={arXiv:2106.04624}
}
```
### Referencing VoxLingua107
```bibtex
@inproceedings{valk2021slt,
title={{VoxLingua107}: a Dataset for Spoken Language Recognition},
author={J{\"o}rgen Valk and Tanel Alum{\"a}e},
booktitle={Proc. IEEE SLT Workshop},
year={2021},
}
```
#### About SpeechBrain
SpeechBrain is an open-source and all-in-one speech toolkit. It is designed to be simple, extremely flexible, and user-friendly. Competitive or state-of-the-art performance is obtained in various domains.
Website: https://speechbrain.github.io/
GitHub: https://github.com/speechbrain/speechbrain
|
mradermacher/llama3-70b-instruct-mmlu-college-medicine-af-mmlu-good-GGUF | mradermacher | "2024-06-29T23:39:49Z" | 103,642 | 0 | transformers | [
"transformers",
"gguf",
"text-generation-inference",
"unsloth",
"llama",
"trl",
"en",
"base_model:AdamKasumovic/llama3-70b-instruct-mmlu-college-medicine-af-mmlu-good",
"license:apache-2.0",
"endpoints_compatible",
"region:us"
] | null | "2024-06-29T19:32:45Z" | ---
base_model: AdamKasumovic/llama3-70b-instruct-mmlu-college-medicine-af-mmlu-good
language:
- en
library_name: transformers
license: apache-2.0
quantized_by: mradermacher
tags:
- text-generation-inference
- transformers
- unsloth
- llama
- trl
---
## About
<!-- ### quantize_version: 2 -->
<!-- ### output_tensor_quantised: 1 -->
<!-- ### convert_type: hf -->
<!-- ### vocab_type: -->
<!-- ### tags: -->
static quants of https://huggingface.co/AdamKasumovic/llama3-70b-instruct-mmlu-college-medicine-af-mmlu-good
<!-- provided-files -->
weighted/imatrix quants seem not to be available (by me) at this time. If they do not show up a week or so after the static ones, I have probably not planned for them. Feel free to request them by opening a Community Discussion.
## Usage
If you are unsure how to use GGUF files, refer to one of [TheBloke's
READMEs](https://huggingface.co/TheBloke/KafkaLM-70B-German-V0.1-GGUF) for
more details, including on how to concatenate multi-part files.
## Provided Quants
(sorted by size, not necessarily quality. IQ-quants are often preferable over similar sized non-IQ quants)
| Link | Type | Size/GB | Notes |
|:-----|:-----|--------:|:------|
| [GGUF](https://huggingface.co/mradermacher/llama3-70b-instruct-mmlu-college-medicine-af-mmlu-good-GGUF/resolve/main/llama3-70b-instruct-mmlu-college-medicine-af-mmlu-good.Q2_K.gguf) | Q2_K | 26.5 | |
| [GGUF](https://huggingface.co/mradermacher/llama3-70b-instruct-mmlu-college-medicine-af-mmlu-good-GGUF/resolve/main/llama3-70b-instruct-mmlu-college-medicine-af-mmlu-good.IQ3_XS.gguf) | IQ3_XS | 29.4 | |
| [GGUF](https://huggingface.co/mradermacher/llama3-70b-instruct-mmlu-college-medicine-af-mmlu-good-GGUF/resolve/main/llama3-70b-instruct-mmlu-college-medicine-af-mmlu-good.IQ3_S.gguf) | IQ3_S | 31.0 | beats Q3_K* |
| [GGUF](https://huggingface.co/mradermacher/llama3-70b-instruct-mmlu-college-medicine-af-mmlu-good-GGUF/resolve/main/llama3-70b-instruct-mmlu-college-medicine-af-mmlu-good.Q3_K_S.gguf) | Q3_K_S | 31.0 | |
| [GGUF](https://huggingface.co/mradermacher/llama3-70b-instruct-mmlu-college-medicine-af-mmlu-good-GGUF/resolve/main/llama3-70b-instruct-mmlu-college-medicine-af-mmlu-good.IQ3_M.gguf) | IQ3_M | 32.0 | |
| [GGUF](https://huggingface.co/mradermacher/llama3-70b-instruct-mmlu-college-medicine-af-mmlu-good-GGUF/resolve/main/llama3-70b-instruct-mmlu-college-medicine-af-mmlu-good.Q3_K_M.gguf) | Q3_K_M | 34.4 | lower quality |
| [GGUF](https://huggingface.co/mradermacher/llama3-70b-instruct-mmlu-college-medicine-af-mmlu-good-GGUF/resolve/main/llama3-70b-instruct-mmlu-college-medicine-af-mmlu-good.Q3_K_L.gguf) | Q3_K_L | 37.2 | |
| [GGUF](https://huggingface.co/mradermacher/llama3-70b-instruct-mmlu-college-medicine-af-mmlu-good-GGUF/resolve/main/llama3-70b-instruct-mmlu-college-medicine-af-mmlu-good.IQ4_XS.gguf) | IQ4_XS | 38.4 | |
| [GGUF](https://huggingface.co/mradermacher/llama3-70b-instruct-mmlu-college-medicine-af-mmlu-good-GGUF/resolve/main/llama3-70b-instruct-mmlu-college-medicine-af-mmlu-good.Q4_K_S.gguf) | Q4_K_S | 40.4 | fast, recommended |
| [GGUF](https://huggingface.co/mradermacher/llama3-70b-instruct-mmlu-college-medicine-af-mmlu-good-GGUF/resolve/main/llama3-70b-instruct-mmlu-college-medicine-af-mmlu-good.Q4_K_M.gguf) | Q4_K_M | 42.6 | fast, recommended |
| [GGUF](https://huggingface.co/mradermacher/llama3-70b-instruct-mmlu-college-medicine-af-mmlu-good-GGUF/resolve/main/llama3-70b-instruct-mmlu-college-medicine-af-mmlu-good.Q5_K_S.gguf) | Q5_K_S | 48.8 | |
| [GGUF](https://huggingface.co/mradermacher/llama3-70b-instruct-mmlu-college-medicine-af-mmlu-good-GGUF/resolve/main/llama3-70b-instruct-mmlu-college-medicine-af-mmlu-good.Q5_K_M.gguf) | Q5_K_M | 50.0 | |
| [PART 1](https://huggingface.co/mradermacher/llama3-70b-instruct-mmlu-college-medicine-af-mmlu-good-GGUF/resolve/main/llama3-70b-instruct-mmlu-college-medicine-af-mmlu-good.Q6_K.gguf.part1of2) [PART 2](https://huggingface.co/mradermacher/llama3-70b-instruct-mmlu-college-medicine-af-mmlu-good-GGUF/resolve/main/llama3-70b-instruct-mmlu-college-medicine-af-mmlu-good.Q6_K.gguf.part2of2) | Q6_K | 58.0 | very good quality |
| [PART 1](https://huggingface.co/mradermacher/llama3-70b-instruct-mmlu-college-medicine-af-mmlu-good-GGUF/resolve/main/llama3-70b-instruct-mmlu-college-medicine-af-mmlu-good.Q8_0.gguf.part1of2) [PART 2](https://huggingface.co/mradermacher/llama3-70b-instruct-mmlu-college-medicine-af-mmlu-good-GGUF/resolve/main/llama3-70b-instruct-mmlu-college-medicine-af-mmlu-good.Q8_0.gguf.part2of2) | Q8_0 | 75.1 | fast, best quality |
Here is a handy graph by ikawrakow comparing some lower-quality quant
types (lower is better):

And here are Artefact2's thoughts on the matter:
https://gist.github.com/Artefact2/b5f810600771265fc1e39442288e8ec9
## FAQ / Model Request
See https://huggingface.co/mradermacher/model_requests for some answers to
questions you might have and/or if you want some other model quantized.
## Thanks
I thank my company, [nethype GmbH](https://www.nethype.de/), for letting
me use its servers and providing upgrades to my workstation to enable
this work in my free time.
<!-- end -->
|
huggyllama/llama-7b | huggyllama | "2024-07-02T15:46:56Z" | 103,548 | 267 | transformers | [
"transformers",
"pytorch",
"safetensors",
"llama",
"text-generation",
"conversational",
"license:other",
"autotrain_compatible",
"endpoints_compatible",
"text-generation-inference",
"region:us"
] | text-generation | "2023-04-03T23:16:48Z" | ---
license: other
---
This contains the weights for the LLaMA-7b model. This model is under a non-commercial license (see the LICENSE file).
You should only use this repository if you have been granted access to the model by filling out [this form](https://docs.google.com/forms/d/e/1FAIpQLSfqNECQnMkycAp2jP4Z9TFX0cGR4uf7b_fBxjY_OjhJILlKGA/viewform?usp=send_form) but either lost your copy of the weights or got some trouble converting them to the Transformers format.
|
LumiOpen/Poro-34B | LumiOpen | "2024-04-22T08:44:11Z" | 103,366 | 109 | transformers | [
"transformers",
"safetensors",
"bloom",
"text-generation",
"fi",
"en",
"dataset:cerebras/SlimPajama-627B",
"dataset:bigcode/starcoderdata",
"dataset:mc4",
"dataset:allenai/dolma",
"arxiv:2404.01856",
"license:apache-2.0",
"autotrain_compatible",
"endpoints_compatible",
"text-generation-inference",
"region:us"
] | text-generation | "2023-10-19T09:03:49Z" | ---
license: apache-2.0
datasets:
- cerebras/SlimPajama-627B
- bigcode/starcoderdata
- mc4
- allenai/dolma
language:
- fi
- en
---
<div align="center">
<img src="./poro-logo.png" width="200px">
</div>
# Poro 34B Model Card
Poro is a 34B parameter decoder-only transformer pretrained on Finnish, English and code. It was trained on 1 trillion tokens. Poro is a fully open source model and is made available under the Apache 2.0 License.
Poro was created in a collaboration between [SiloGen](https://www.silo.ai/silogen) from [Silo AI](https://www.silo.ai/), the [TurkuNLP group](https://turkunlp.org/) of the University of Turku, and [High Performance Language Technologies](https://hplt-project.org/) (HPLT). Training was conducted on the [LUMI supercomputer](https://www.lumi-supercomputer.eu/), using compute resources generously provided by [CSC](https://csc.fi/) - IT Center for Science, Finland.
This project is part of an ongoing effort to create open source large language models for non-English and especially low resource languages like Finnish. Through the combination of English and Finnish training data we get a model that outperforms previous Finnish only models, while also being fluent in English and code, and capable of basic translation between English and Finnish.
Poro 34B is only the first model of our model family. Work is already underway on our next models which will support additional languages, and include features like flash attention, rotary embeddings, and grouped query attention.
_What does Poro mean?_ Poro is the Finnish word for Reindeer! 🦌 These animals are native to Finland and hold a significant and historical role in Finnish culture.
## Model Overview
_**NOTE:** In addition to being an early research release, Poro is a base model which needs further fine tuning for most use cases._
Poro is a generative pretrained transformer using a BLOOM architecture, and makes use of ALiBi embeddings to support context length extrapolation at inference time.
| Hyperparameter | Value |
| :------------- | :----: |
| n_parameters | 34.2B |
| n_layers | 54 |
| n_heads | 56 |
| d_model | 7168 |
| vocab_size | 128000 |
| sequence_length | 2048 |
## Poro Research Checkpoints
Checkpoints are available as branches in the repository. Checkpoints will be released roughly every 100B tokens. The main branch will always point to the latest checkpoint. The following checkpoints are available:
* [100B](https://huggingface.co/LumiOpen/Poro-34B/tree/100B)
* [200B](https://huggingface.co/LumiOpen/Poro-34B/tree/200B)
* [300B](https://huggingface.co/LumiOpen/Poro-34B/tree/300B)
* [400B](https://huggingface.co/LumiOpen/Poro-34B/tree/400B)
* [500B](https://huggingface.co/LumiOpen/Poro-34B/tree/500B)
* [600B](https://huggingface.co/LumiOpen/Poro-34B/tree/600B)
* [700B](https://huggingface.co/LumiOpen/Poro-34B/tree/700B)
* [800B](https://huggingface.co/LumiOpen/Poro-34B/tree/800B)
* [900B](https://huggingface.co/LumiOpen/Poro-34B/tree/900B)
* [1000B](https://huggingface.co/LumiOpen/Poro-34B/tree/1000B)
The transformers library allows you to load a checkpoint from a branch as follows:
```python
branch = "200B"
model = transformers.AutoModelForCausalLM.from_pretrained(
"LumiOpen/Poro-34B",
torch_dtype=torch.bfloat16,
revision=branch,
)
```
## Training
Poro was trained on the LUMI supercomputer, using 512 AMD MI250X GPUs. Each MI250X GPU has two Graphics Complex Dies (GCDs) for a world size of 1024 during training, using activation checkpointing, a micro batch size of 1, gradient accumulation of 16, and a 3D parallelism strategy of TP=2, PP=4, DP=128.
Training began in September 2023 using a custom fork of the Megatron-Deepspeed framework. Our code is available [here](https://github.com/TurkuNLP/Megatron-DeepSpeed).
## Training Hyperparameters
| Hyperparameter | Value | Comment |
| :------------: | :---: | :------:|
| Precision | bfloat16 | |
| Optimizer | AdamW | |
| Learning rate | 1.5e-4 | 10B tokens warm-up, cosine decay to 2e-5 |
| Weight decay | 1e-1 | |
| Batch size | 2048 | 2048 samples x 2048 tokens = 4194304 tokens |
## Tokenizer
Poro uses a custom 128K Bloom tokenizer trained on the same English, Finnish and Code dataset used to train the model.
## Dataset
Poro is being trained on a 1 trillion token mixed dataset of English, Finnish and Code.
| Dataset | Notes | Percentage | Epochs | Tokens |
| :-----: | :---: | :--------: | :----: | :----: |
| SlimPajama | Excluding books3 data | 54.16% | 1x | 541.7B |
| Finnish | TurkuNLP Finnish dataset | 13.05% | 4x | 131.5B |
| Tatoeba | English/Finnish sentence pairs | 0.81% | 1x | 8.0B |
| Starcoder | | 31.53% | 1.52x | 315.4B |
| Project Gutenberg | from Dolma dataset | 0.46% | 1x | 4.5B |
The Finnish dataset is a combination of many Finnish resources:
* [Finnish Internet Parsebank](https://turkunlp.org/finnish_nlp.html)
* [mC4 multilingual colossal, cleaned Common Crawl](https://huggingface.co/datasets/mc4)
* [Common Crawl Finnish](https://github.com/turkunlp/CC-Fi)
* [Finnish Wikipedia](https://fi.wikipedia.org/wiki)
* [Lönnrot Projekti Lönnrot](http://www.lonnrot.net/)
* [Suomi24 The Suomi 24 Corpus 2001-2020](http://urn.fi/urn:nbn:fi:lb-2021101527)
* [Reddit r/Suomi submissions and comments](https://www.reddit.com/r/Suomi)
* [STT Finnish News Agency Archive 1992-2018](http://urn.fi/urn:nbn:fi:lb-2019041501)
* [Yle Finnish News Archive 2011-2018](http://urn.fi/urn:nbn:fi:lb-2017070501)
* [Yle Finnish News Archive 2019-2020](http://urn.fi/urn:nbn:fi:lb-2021050401)
* [Yle News Archive Easy-to-read Finnish 2011-2018](http://urn.fi/urn:nbn:fi:lb-2019050901)
* [Yle News Archive Easy-to-read Finnish 2019-2020](http://urn.fi/urn:nbn:fi:lb-2021050701)
## Evaluation Results
Full evaluations for each checkpoint are available on our [Github repo](https://github.com/LumiOpen/evaluation/).
## Ethical Considerations and Limitations
Poro is an advanced language model, primarily optimized for English, Finnish and code, with no meaningful proficiency in any other languages. As with most AI-driven systems, Poro is a product of the vast data it has been trained on, which may reflect the imperfections, biases, and idiosyncrasies of the wider web. Poro may, at times, produce outputs that can be considered inaccurate, prejudiced, or controversial. Users and developers engaging with Poro should exercise discretion and consider additional evaluation and customization to ensure the model's responses align with their specific needs and ethical standards.
## License
Poro is released under the Apache 2.0 license.
## Citation
```
@misc{luukkonen2024poro,
title={Poro 34B and the Blessing of Multilinguality},
author={Risto Luukkonen and Jonathan Burdge and Elaine Zosa and Aarne
Talman and Ville Komulainen and Väinö Hatanpää and Peter Sarlin and Sampo
Pyysalo},
year={2024},
eprint={2404.01856},
archivePrefix={arXiv},
primaryClass={cs.CL}
}
```
|
microsoft/xclip-base-patch32 | microsoft | "2024-02-04T01:26:30Z" | 103,151 | 53 | transformers | [
"transformers",
"pytorch",
"safetensors",
"xclip",
"vision",
"video-classification",
"en",
"arxiv:2208.02816",
"license:mit",
"model-index",
"endpoints_compatible",
"region:us"
] | video-classification | "2022-08-25T13:06:15Z" | ---
language: en
license: mit
tags:
- vision
- video-classification
model-index:
- name: nielsr/xclip-base-patch32
results:
- task:
type: video-classification
dataset:
name: Kinetics 400
type: kinetics-400
metrics:
- type: top-1 accuracy
value: 80.4
- type: top-5 accuracy
value: 95.0
---
# X-CLIP (base-sized model)
X-CLIP model (base-sized, patch resolution of 32) trained fully-supervised on [Kinetics-400](https://www.deepmind.com/open-source/kinetics). It was introduced in the paper [Expanding Language-Image Pretrained Models for General Video Recognition](https://arxiv.org/abs/2208.02816) by Ni et al. and first released in [this repository](https://github.com/microsoft/VideoX/tree/master/X-CLIP).
This model was trained using 8 frames per video, at a resolution of 224x224.
Disclaimer: The team releasing X-CLIP did not write a model card for this model so this model card has been written by the Hugging Face team.
## Model description
X-CLIP is a minimal extension of [CLIP](https://huggingface.co/docs/transformers/model_doc/clip) for general video-language understanding. The model is trained in a contrastive way on (video, text) pairs.

This allows the model to be used for tasks like zero-shot, few-shot or fully supervised video classification and video-text retrieval.
## Intended uses & limitations
You can use the raw model for determining how well text goes with a given video. See the [model hub](https://huggingface.co/models?search=microsoft/xclip) to look for
fine-tuned versions on a task that interests you.
### How to use
For code examples, we refer to the [documentation](https://huggingface.co/transformers/main/model_doc/xclip.html#).
## Training data
This model was trained on [Kinetics-400](https://www.deepmind.com/open-source/kinetics).
### Preprocessing
The exact details of preprocessing during training can be found [here](https://github.com/microsoft/VideoX/blob/40f6d177e0a057a50ac69ac1de6b5938fd268601/X-CLIP/datasets/build.py#L247).
The exact details of preprocessing during validation can be found [here](https://github.com/microsoft/VideoX/blob/40f6d177e0a057a50ac69ac1de6b5938fd268601/X-CLIP/datasets/build.py#L285).
During validation, one resizes the shorter edge of each frame, after which center cropping is performed to a fixed-size resolution (like 224x224). Next, frames are normalized across the RGB channels with the ImageNet mean and standard deviation.
## Evaluation results
This model achieves a top-1 accuracy of 80.4% and a top-5 accuracy of 95.0%.
|
HuggingFaceM4/tiny-random-LlamaForCausalLM | HuggingFaceM4 | "2024-04-25T10:54:55Z" | 102,918 | 18 | transformers | [
"transformers",
"pytorch",
"llama",
"text-generation",
"autotrain_compatible",
"endpoints_compatible",
"text-generation-inference",
"region:us"
] | text-generation | "2023-03-16T23:18:46Z" | Entry not found |
ptx0/pixart-900m-1024-ft | ptx0 | "2024-07-03T01:15:59Z" | 102,918 | 16 | diffusers | [
"diffusers",
"safetensors",
"stable-diffusion",
"stable-diffusion-diffusers",
"text-to-image",
"simpletuner",
"full",
"base_model:ptx0/pixart-900m-1024-ft-large",
"license:creativeml-openrail-m",
"diffusers:PixArtSigmaPipeline",
"region:us"
] | text-to-image | "2024-06-17T04:27:18Z" | ---
license: creativeml-openrail-m
base_model: "ptx0/pixart-900m-1024-ft-large"
tags:
- stable-diffusion
- stable-diffusion-diffusers
- text-to-image
- diffusers
- simpletuner
- full
inference: true
widget:
- text: 'unconditional (blank prompt)'
parameters:
negative_prompt: 'blurry, cropped, ugly'
output:
url: ./assets/image_0_0.png
- text: 'unconditional (blank prompt)'
parameters:
negative_prompt: 'blurry, cropped, ugly'
output:
url: ./assets/image_1_1.png
- text: 'unconditional (blank prompt)'
parameters:
negative_prompt: 'blurry, cropped, ugly'
output:
url: ./assets/image_2_2.png
---
# pixart-900m-1024-ft
This is a full rank finetune derived from [ptx0/pixart-900m-1024-ft-large](https://huggingface.co/ptx0/pixart-900m-1024-ft-large).
The main validation prompt used during training was:
```
ethnographic photography of teddy bear at a picnic holding a sign that reads SOON
```
## Validation settings
- CFG: `7.5`
- CFG Rescale: `0.0`
- Steps: `30`
- Sampler: `euler`
- Seed: `42`
- Resolutions: `1024x1024,1344x768,916x1152`
Note: The validation settings are not necessarily the same as the [training settings](#training-settings).
You can find some example images in the following gallery:
<Gallery />
The text encoder **was not** trained.
You may reuse the base model text encoder for inference.
## Training settings
- Training epochs: 1
- Training steps: 32000
- Learning rate: 1e-06
- Effective batch size: 192
- Micro-batch size: 24
- Gradient accumulation steps: 1
- Number of GPUs: 8
- Prediction type: epsilon
- Rescaled betas zero SNR: False
- Optimizer: AdamW, stochastic bf16
- Precision: Pure BF16
- Xformers: Not used
## Datasets
### photo-concept-bucket
- Repeats: 0
- Total number of images: ~564672
- Total number of aspect buckets: 7
- Resolution: 1.0 megapixels
- Cropped: False
- Crop style: None
- Crop aspect: None
### moviecollection
- Repeats: 15
- Total number of images: ~768
- Total number of aspect buckets: 11
- Resolution: 1.0 megapixels
- Cropped: True
- Crop style: random
- Crop aspect: random
### experimental
- Repeats: 0
- Total number of images: ~1728
- Total number of aspect buckets: 11
- Resolution: 1.0 megapixels
- Cropped: True
- Crop style: random
- Crop aspect: random
### ethnic
- Repeats: 0
- Total number of images: ~1152
- Total number of aspect buckets: 7
- Resolution: 1.0 megapixels
- Cropped: True
- Crop style: random
- Crop aspect: random
### sports
- Repeats: 0
- Total number of images: ~576
- Total number of aspect buckets: 1
- Resolution: 1.0 megapixels
- Cropped: True
- Crop style: random
- Crop aspect: square
### architecture
- Repeats: 0
- Total number of images: ~4224
- Total number of aspect buckets: 1
- Resolution: 1.0 megapixels
- Cropped: True
- Crop style: random
- Crop aspect: square
### shutterstock
- Repeats: 0
- Total number of images: ~14016
- Total number of aspect buckets: 3
- Resolution: 1.0 megapixels
- Cropped: True
- Crop style: random
- Crop aspect: random
### cinemamix-1mp
- Repeats: 0
- Total number of images: ~7296
- Total number of aspect buckets: 3
- Resolution: 1.0 megapixels
- Cropped: True
- Crop style: random
- Crop aspect: random
### nsfw-1024
- Repeats: 0
- Total number of images: ~10368
- Total number of aspect buckets: 3
- Resolution: 1.0 megapixels
- Cropped: True
- Crop style: random
- Crop aspect: random
### anatomy
- Repeats: 5
- Total number of images: ~15168
- Total number of aspect buckets: 3
- Resolution: 1.0 megapixels
- Cropped: True
- Crop style: random
- Crop aspect: random
### bg20k-1024
- Repeats: 0
- Total number of images: ~89088
- Total number of aspect buckets: 3
- Resolution: 1.0 megapixels
- Cropped: True
- Crop style: random
- Crop aspect: random
### yoga
- Repeats: 0
- Total number of images: ~2880
- Total number of aspect buckets: 3
- Resolution: 1.0 megapixels
- Cropped: True
- Crop style: random
- Crop aspect: random
### photo-aesthetics
- Repeats: 0
- Total number of images: ~28608
- Total number of aspect buckets: 17
- Resolution: 1.0 megapixels
- Cropped: True
- Crop style: random
- Crop aspect: random
### text-1mp
- Repeats: 125
- Total number of images: ~12864
- Total number of aspect buckets: 3
- Resolution: 1.0 megapixels
- Cropped: True
- Crop style: random
- Crop aspect: random
### movieposters
- Repeats: 10
- Total number of images: ~192
- Total number of aspect buckets: 1
- Resolution: 1.0 megapixels
- Cropped: True
- Crop style: random
- Crop aspect: square
### normalnudes
- Repeats: 10
- Total number of images: ~384
- Total number of aspect buckets: 8
- Resolution: 1.0 megapixels
- Cropped: True
- Crop style: random
- Crop aspect: random
### pixel-art
- Repeats: 0
- Total number of images: ~384
- Total number of aspect buckets: 11
- Resolution: 1.0 megapixels
- Cropped: True
- Crop style: random
- Crop aspect: random
### signs
- Repeats: 0
- Total number of images: ~384
- Total number of aspect buckets: 1
- Resolution: 1.0 megapixels
- Cropped: True
- Crop style: random
- Crop aspect: square
### midjourney-v6-520k-raw
- Repeats: 0
- Total number of images: ~513792
- Total number of aspect buckets: 7
- Resolution: 1.0 megapixels
- Cropped: False
- Crop style: None
- Crop aspect: None
### sfwbooru
- Repeats: 0
- Total number of images: ~271488
- Total number of aspect buckets: 19
- Resolution: 1.0 megapixels
- Cropped: False
- Crop style: None
- Crop aspect: None
### nijijourney-v6-520k-raw
- Repeats: 0
- Total number of images: ~516288
- Total number of aspect buckets: 7
- Resolution: 1.0 megapixels
- Cropped: False
- Crop style: None
- Crop aspect: None
### dalle3
- Repeats: 0
- Total number of images: ~1119168
- Total number of aspect buckets: 2
- Resolution: 1.0 megapixels
- Cropped: False
- Crop style: None
- Crop aspect: None
## Inference
```python
import torch
from diffusers import DiffusionPipeline
model_id = 'pixart-900m-1024-ft'
prompt = 'ethnographic photography of teddy bear at a picnic holding a sign that reads SOON'
negative_prompt = 'blurry, cropped, ugly'
pipeline = DiffusionPipeline.from_pretrained(model_id)
pipeline.to('cuda' if torch.cuda.is_available() else 'mps' if torch.backends.mps.is_available() else 'cpu')
prompt = "ethnographic photography of teddy bear at a picnic holding a sign that reads SOON"
negative_prompt = "blurry, cropped, ugly"
pipeline = DiffusionPipeline.from_pretrained(model_id)
pipeline.to('cuda' if torch.cuda.is_available() else 'mps' if torch.backends.mps.is_available() else 'cpu')
image = pipeline(
prompt=prompt,
negative_prompt='blurry, cropped, ugly',
num_inference_steps=30,
generator=torch.Generator(device='cuda' if torch.cuda.is_available() else 'mps' if torch.backends.mps.is_available() else 'cpu').manual_seed(1641421826),
width=1152,
height=768,
guidance_scale=7.5,
guidance_rescale=0.0,
).images[0]
image.save("output.png", format="PNG")
```
|
mradermacher/Llama-3-TenyxChat-DaybreakStorywriter-70B-i1-GGUF | mradermacher | "2024-07-02T23:03:41Z" | 102,913 | 1 | transformers | [
"transformers",
"gguf",
"not-for-all-audiences",
"en",
"base_model:Envoid/Llama-3-TenyxChat-DaybreakStorywriter-70B",
"license:cc-by-nc-4.0",
"endpoints_compatible",
"region:us"
] | null | "2024-06-30T17:34:57Z" | ---
base_model: Envoid/Llama-3-TenyxChat-DaybreakStorywriter-70B
language:
- en
library_name: transformers
license: cc-by-nc-4.0
quantized_by: mradermacher
tags:
- not-for-all-audiences
---
## About
<!-- ### quantize_version: 2 -->
<!-- ### output_tensor_quantised: 1 -->
<!-- ### convert_type: hf -->
<!-- ### vocab_type: -->
<!-- ### tags: nicoboss -->
weighted/imatrix quants of https://huggingface.co/Envoid/Llama-3-TenyxChat-DaybreakStorywriter-70B
<!-- provided-files -->
static quants are available at https://huggingface.co/mradermacher/Llama-3-TenyxChat-DaybreakStorywriter-70B-GGUF
## Usage
If you are unsure how to use GGUF files, refer to one of [TheBloke's
READMEs](https://huggingface.co/TheBloke/KafkaLM-70B-German-V0.1-GGUF) for
more details, including on how to concatenate multi-part files.
## Provided Quants
(sorted by size, not necessarily quality. IQ-quants are often preferable over similar sized non-IQ quants)
| Link | Type | Size/GB | Notes |
|:-----|:-----|--------:|:------|
| [GGUF](https://huggingface.co/mradermacher/Llama-3-TenyxChat-DaybreakStorywriter-70B-i1-GGUF/resolve/main/Llama-3-TenyxChat-DaybreakStorywriter-70B.i1-IQ2_XXS.gguf) | i1-IQ2_XXS | 19.2 | |
| [GGUF](https://huggingface.co/mradermacher/Llama-3-TenyxChat-DaybreakStorywriter-70B-i1-GGUF/resolve/main/Llama-3-TenyxChat-DaybreakStorywriter-70B.i1-IQ2_XS.gguf) | i1-IQ2_XS | 21.2 | |
| [GGUF](https://huggingface.co/mradermacher/Llama-3-TenyxChat-DaybreakStorywriter-70B-i1-GGUF/resolve/main/Llama-3-TenyxChat-DaybreakStorywriter-70B.i1-IQ2_M.gguf) | i1-IQ2_M | 24.2 | |
| [GGUF](https://huggingface.co/mradermacher/Llama-3-TenyxChat-DaybreakStorywriter-70B-i1-GGUF/resolve/main/Llama-3-TenyxChat-DaybreakStorywriter-70B.i1-Q2_K.gguf) | i1-Q2_K | 26.5 | IQ3_XXS probably better |
| [GGUF](https://huggingface.co/mradermacher/Llama-3-TenyxChat-DaybreakStorywriter-70B-i1-GGUF/resolve/main/Llama-3-TenyxChat-DaybreakStorywriter-70B.i1-IQ3_XXS.gguf) | i1-IQ3_XXS | 27.6 | lower quality |
| [GGUF](https://huggingface.co/mradermacher/Llama-3-TenyxChat-DaybreakStorywriter-70B-i1-GGUF/resolve/main/Llama-3-TenyxChat-DaybreakStorywriter-70B.i1-IQ3_XS.gguf) | i1-IQ3_XS | 29.4 | |
| [GGUF](https://huggingface.co/mradermacher/Llama-3-TenyxChat-DaybreakStorywriter-70B-i1-GGUF/resolve/main/Llama-3-TenyxChat-DaybreakStorywriter-70B.i1-IQ3_S.gguf) | i1-IQ3_S | 31.0 | beats Q3_K* |
| [GGUF](https://huggingface.co/mradermacher/Llama-3-TenyxChat-DaybreakStorywriter-70B-i1-GGUF/resolve/main/Llama-3-TenyxChat-DaybreakStorywriter-70B.i1-Q3_K_S.gguf) | i1-Q3_K_S | 31.0 | IQ3_XS probably better |
| [GGUF](https://huggingface.co/mradermacher/Llama-3-TenyxChat-DaybreakStorywriter-70B-i1-GGUF/resolve/main/Llama-3-TenyxChat-DaybreakStorywriter-70B.i1-IQ3_M.gguf) | i1-IQ3_M | 32.0 | |
| [GGUF](https://huggingface.co/mradermacher/Llama-3-TenyxChat-DaybreakStorywriter-70B-i1-GGUF/resolve/main/Llama-3-TenyxChat-DaybreakStorywriter-70B.i1-Q3_K_M.gguf) | i1-Q3_K_M | 34.4 | IQ3_S probably better |
| [GGUF](https://huggingface.co/mradermacher/Llama-3-TenyxChat-DaybreakStorywriter-70B-i1-GGUF/resolve/main/Llama-3-TenyxChat-DaybreakStorywriter-70B.i1-Q3_K_L.gguf) | i1-Q3_K_L | 37.2 | IQ3_M probably better |
| [GGUF](https://huggingface.co/mradermacher/Llama-3-TenyxChat-DaybreakStorywriter-70B-i1-GGUF/resolve/main/Llama-3-TenyxChat-DaybreakStorywriter-70B.i1-IQ4_XS.gguf) | i1-IQ4_XS | 38.0 | |
| [GGUF](https://huggingface.co/mradermacher/Llama-3-TenyxChat-DaybreakStorywriter-70B-i1-GGUF/resolve/main/Llama-3-TenyxChat-DaybreakStorywriter-70B.i1-Q4_0.gguf) | i1-Q4_0 | 40.2 | fast, low quality |
| [GGUF](https://huggingface.co/mradermacher/Llama-3-TenyxChat-DaybreakStorywriter-70B-i1-GGUF/resolve/main/Llama-3-TenyxChat-DaybreakStorywriter-70B.i1-Q4_K_S.gguf) | i1-Q4_K_S | 40.4 | optimal size/speed/quality |
| [GGUF](https://huggingface.co/mradermacher/Llama-3-TenyxChat-DaybreakStorywriter-70B-i1-GGUF/resolve/main/Llama-3-TenyxChat-DaybreakStorywriter-70B.i1-Q4_K_M.gguf) | i1-Q4_K_M | 42.6 | fast, recommended |
| [GGUF](https://huggingface.co/mradermacher/Llama-3-TenyxChat-DaybreakStorywriter-70B-i1-GGUF/resolve/main/Llama-3-TenyxChat-DaybreakStorywriter-70B.i1-Q5_K_S.gguf) | i1-Q5_K_S | 48.8 | |
| [GGUF](https://huggingface.co/mradermacher/Llama-3-TenyxChat-DaybreakStorywriter-70B-i1-GGUF/resolve/main/Llama-3-TenyxChat-DaybreakStorywriter-70B.i1-Q5_K_M.gguf) | i1-Q5_K_M | 50.0 | |
| [PART 1](https://huggingface.co/mradermacher/Llama-3-TenyxChat-DaybreakStorywriter-70B-i1-GGUF/resolve/main/Llama-3-TenyxChat-DaybreakStorywriter-70B.i1-Q6_K.gguf.part1of2) [PART 2](https://huggingface.co/mradermacher/Llama-3-TenyxChat-DaybreakStorywriter-70B-i1-GGUF/resolve/main/Llama-3-TenyxChat-DaybreakStorywriter-70B.i1-Q6_K.gguf.part2of2) | i1-Q6_K | 58.0 | practically like static Q6_K |
Here is a handy graph by ikawrakow comparing some lower-quality quant
types (lower is better):

And here are Artefact2's thoughts on the matter:
https://gist.github.com/Artefact2/b5f810600771265fc1e39442288e8ec9
## FAQ / Model Request
See https://huggingface.co/mradermacher/model_requests for some answers to
questions you might have and/or if you want some other model quantized.
## Thanks
I thank my company, [nethype GmbH](https://www.nethype.de/), for letting
me use its servers and providing upgrades to my workstation to enable
this work in my free time. Additional thanks to [@nicoboss](https://huggingface.co/nicoboss) for giving me access to his hardware for calculating the imatrix for these quants.
<!-- end -->
|
google/gemma-1.1-7b-it | google | "2024-06-27T14:09:53Z" | 102,520 | 253 | transformers | [
"transformers",
"safetensors",
"gemma",
"text-generation",
"conversational",
"arxiv:2312.11805",
"arxiv:2009.03300",
"arxiv:1905.07830",
"arxiv:1911.11641",
"arxiv:1904.09728",
"arxiv:1905.10044",
"arxiv:1907.10641",
"arxiv:1811.00937",
"arxiv:1809.02789",
"arxiv:1911.01547",
"arxiv:1705.03551",
"arxiv:2107.03374",
"arxiv:2108.07732",
"arxiv:2110.14168",
"arxiv:2304.06364",
"arxiv:2206.04615",
"arxiv:1804.06876",
"arxiv:2110.08193",
"license:gemma",
"autotrain_compatible",
"endpoints_compatible",
"text-generation-inference",
"region:us"
] | text-generation | "2024-03-26T22:40:00Z" | ---
library_name: transformers
license: gemma
widget:
- messages:
- role: user
content: How does the brain work?
inference:
parameters:
max_new_tokens: 200
extra_gated_heading: Access Gemma on Hugging Face
extra_gated_prompt: To access Gemma on Hugging Face, you’re required to review and
agree to Google’s usage license. To do this, please ensure you’re logged-in to Hugging
Face and click below. Requests are processed immediately.
extra_gated_button_content: Acknowledge license
---
# Gemma Model Card
**Model Page**: [Gemma](https://ai.google.dev/gemma/docs)
This model card corresponds to the latest 7B instruct version of the Gemma model. Here you can find other models in the Gemma family:
| | Base | Instruct |
|----|----------------------------------------------------|----------------------------------------------------------------------|
| 2B | [gemma-2b](https://huggingface.co/google/gemma-2b) | [gemma-1.1-2b-it](https://huggingface.co/google/gemma-1.1-2b-it) |
| 7B | [gemma-7b](https://huggingface.co/google/gemma-7b) | [**gemma-1.1-7b-it**](https://huggingface.co/google/gemma-1.1-7b-it) |
**Release Notes**
This is Gemma 1.1 7B (IT), an update over the original instruction-tuned Gemma release.
Gemma 1.1 was trained using a novel RLHF method, leading to substantial gains on quality, coding capabilities, factuality, instruction following and multi-turn conversation quality. We also fixed a bug in multi-turn conversations, and made sure that model responses don't always start with `"Sure,"`.
We believe this release represents an improvement for most use cases, but we encourage users to test in their particular applications. The previous model [will continue to be available in the same repo](https://huggingface.co/google/gemma-7b-it). We appreciate the enthusiastic adoption of Gemma, and we continue to welcome all feedback from the community.
**Resources and Technical Documentation**:
* [Responsible Generative AI Toolkit](https://ai.google.dev/responsible)
* [Gemma on Kaggle](https://www.kaggle.com/models/google/gemma)
* [Gemma on Vertex Model Garden](https://console.cloud.google.com/vertex-ai/publishers/google/model-garden/335)
**Terms of Use**: [Terms](https://www.kaggle.com/models/google/gemma/license/consent/verify/huggingface?returnModelRepoId=google/gemma-1.1-7b-it)
**Authors**: Google
## Model Information
Summary description and brief definition of inputs and outputs.
### Description
Gemma is a family of lightweight, state-of-the-art open models from Google,
built from the same research and technology used to create the Gemini models.
They are text-to-text, decoder-only large language models, available in English,
with open weights, pre-trained variants, and instruction-tuned variants. Gemma
models are well-suited for a variety of text generation tasks, including
question answering, summarization, and reasoning. Their relatively small size
makes it possible to deploy them in environments with limited resources such as
a laptop, desktop or your own cloud infrastructure, democratizing access to
state of the art AI models and helping foster innovation for everyone.
### Usage
Below we share some code snippets on how to get quickly started with running the model. First make sure to `pip install -U transformers`, then copy the snippet from the section that is relevant for your usecase.
#### Running the model on a CPU
As explained below, we recommend `torch.bfloat16` as the default dtype. You can use [a different precision](#precisions) if necessary.
```python
from transformers import AutoTokenizer, AutoModelForCausalLM
import torch
tokenizer = AutoTokenizer.from_pretrained("google/gemma-1.1-7b-it")
model = AutoModelForCausalLM.from_pretrained(
"google/gemma-1.1-7b-it",
torch_dtype=torch.bfloat16
)
input_text = "Write me a poem about Machine Learning."
input_ids = tokenizer(input_text, return_tensors="pt")
outputs = model.generate(**input_ids, max_new_tokens=50)
print(tokenizer.decode(outputs[0]))
```
#### Running the model on a single / multi GPU
```python
# pip install accelerate
from transformers import AutoTokenizer, AutoModelForCausalLM
import torch
tokenizer = AutoTokenizer.from_pretrained("google/gemma-1.1-7b-it")
model = AutoModelForCausalLM.from_pretrained(
"google/gemma-1.1-7b-it",
device_map="auto",
torch_dtype=torch.bfloat16
)
input_text = "Write me a poem about Machine Learning."
input_ids = tokenizer(input_text, return_tensors="pt").to("cuda")
outputs = model.generate(**input_ids)
print(tokenizer.decode(outputs[0]))
```
<a name="precisions"></a>
#### Running the model on a GPU using different precisions
The native weights of this model were exported in `bfloat16` precision. You can use `float16`, which may be faster on certain hardware, indicating the `torch_dtype` when loading the model. For convenience, the `float16` revision of the repo contains a copy of the weights already converted to that precision.
You can also use `float32` if you skip the dtype, but no precision increase will occur (model weights will just be upcasted to `float32`). See examples below.
* _Using `torch.float16`_
```python
# pip install accelerate
from transformers import AutoTokenizer, AutoModelForCausalLM
import torch
tokenizer = AutoTokenizer.from_pretrained("google/gemma-1.1-7b-it")
model = AutoModelForCausalLM.from_pretrained(
"google/gemma-1.1-7b-it",
device_map="auto",
torch_dtype=torch.float16,
revision="float16",
)
input_text = "Write me a poem about Machine Learning."
input_ids = tokenizer(input_text, return_tensors="pt").to("cuda")
outputs = model.generate(**input_ids)
print(tokenizer.decode(outputs[0]))
```
* _Using `torch.bfloat16`_
```python
# pip install accelerate
from transformers import AutoTokenizer, AutoModelForCausalLM
import torch
tokenizer = AutoTokenizer.from_pretrained("google/gemma-2b-it")
model = AutoModelForCausalLM.from_pretrained(
"google/gemma-1.1-7b-it",
device_map="auto",
torch_dtype=torch.bfloat16
)
input_text = "Write me a poem about Machine Learning."
input_ids = tokenizer(input_text, return_tensors="pt").to("cuda")
outputs = model.generate(**input_ids)
print(tokenizer.decode(outputs[0]))
```
* _Upcasting to `torch.float32`_
```python
# pip install accelerate
from transformers import AutoTokenizer, AutoModelForCausalLM
tokenizer = AutoTokenizer.from_pretrained("google/gemma-1.1-7b-it")
model = AutoModelForCausalLM.from_pretrained(
"google/gemma-1.1-7b-it",
device_map="auto"
)
input_text = "Write me a poem about Machine Learning."
input_ids = tokenizer(input_text, return_tensors="pt").to("cuda")
outputs = model.generate(**input_ids)
print(tokenizer.decode(outputs[0]))
```
#### Quantized Versions through `bitsandbytes`
* _Using 8-bit precision (int8)_
```python
# pip install bitsandbytes accelerate
from transformers import AutoTokenizer, AutoModelForCausalLM, BitsAndBytesConfig
quantization_config = BitsAndBytesConfig(load_in_8bit=True)
tokenizer = AutoTokenizer.from_pretrained("google/gemma-1.1-7b-it")
model = AutoModelForCausalLM.from_pretrained(
"google/gemma-1.1-7b-it",
quantization_config=quantization_config
)
input_text = "Write me a poem about Machine Learning."
input_ids = tokenizer(input_text, return_tensors="pt").to("cuda")
outputs = model.generate(**input_ids)
print(tokenizer.decode(outputs[0]))
```
* _Using 4-bit precision_
```python
# pip install bitsandbytes accelerate
from transformers import AutoTokenizer, AutoModelForCausalLM, BitsAndBytesConfig
quantization_config = BitsAndBytesConfig(load_in_4bit=True)
tokenizer = AutoTokenizer.from_pretrained("google/gemma-1.1-7b-it")
model = AutoModelForCausalLM.from_pretrained(
"google/gemma-1.1-7b-it",
quantization_config=quantization_config
)
input_text = "Write me a poem about Machine Learning."
input_ids = tokenizer(input_text, return_tensors="pt").to("cuda")
outputs = model.generate(**input_ids)
print(tokenizer.decode(outputs[0]))
```
#### Other optimizations
* _Flash Attention 2_
First make sure to install `flash-attn` in your environment `pip install flash-attn`
```diff
model = AutoModelForCausalLM.from_pretrained(
model_id,
torch_dtype=torch.float16,
+ attn_implementation="flash_attention_2"
).to(0)
```
#### Running the model in JAX / Flax
Use the `flax` branch of the repository:
```python
import jax.numpy as jnp
from transformers import AutoTokenizer, FlaxGemmaForCausalLM
model_id = "google/gemma-1.1-7b-it"
tokenizer = AutoTokenizer.from_pretrained(model_id)
tokenizer.padding_side = "left"
model, params = FlaxGemmaForCausalLM.from_pretrained(
model_id,
dtype=jnp.bfloat16,
revision="flax",
_do_init=False,
)
inputs = tokenizer("Valencia and Málaga are", return_tensors="np", padding=True)
output = model.generate(**inputs, params=params, max_new_tokens=20, do_sample=False)
output_text = tokenizer.batch_decode(output.sequences, skip_special_tokens=True)
```
[Check this notebook](https://colab.research.google.com/github/sanchit-gandhi/notebooks/blob/main/jax_gemma.ipynb) for a comprehensive walkthrough on how to parallelize JAX inference.
### Chat Template
The instruction-tuned models use a chat template that must be adhered to for conversational use.
The easiest way to apply it is using the tokenizer's built-in chat template, as shown in the following snippet.
Let's load the model and apply the chat template to a conversation. In this example, we'll start with a single user interaction:
```py
from transformers import AutoTokenizer, AutoModelForCausalLM
import transformers
import torch
model_id = "google/gemma-1.1-7b-it"
dtype = torch.bfloat16
tokenizer = AutoTokenizer.from_pretrained(model_id)
model = AutoModelForCausalLM.from_pretrained(
model_id,
device_map="cuda",
torch_dtype=dtype,
)
chat = [
{ "role": "user", "content": "Write a hello world program" },
]
prompt = tokenizer.apply_chat_template(chat, tokenize=False, add_generation_prompt=True)
```
At this point, the prompt contains the following text:
```
<bos><start_of_turn>user
Write a hello world program<end_of_turn>
<start_of_turn>model
```
As you can see, each turn is preceded by a `<start_of_turn>` delimiter and then the role of the entity
(either `user`, for content supplied by the user, or `model` for LLM responses). Turns finish with
the `<end_of_turn>` token.
You can follow this format to build the prompt manually, if you need to do it without the tokenizer's
chat template.
After the prompt is ready, generation can be performed like this:
```py
inputs = tokenizer.encode(prompt, add_special_tokens=False, return_tensors="pt")
outputs = model.generate(input_ids=inputs.to(model.device), max_new_tokens=150)
```
### Fine-tuning
You can find some fine-tuning scripts under the [`examples/` directory](https://huggingface.co/google/gemma-7b/tree/main/examples) of [`google/gemma-7b`](https://huggingface.co/google/gemma-7b) repository. To adapt them to this model, simply change the model-id to `google/gemma-1.1-7b-it`.
We provide:
* A script to perform Supervised Fine-Tuning (SFT) on UltraChat dataset using QLoRA
* A script to perform SFT using FSDP on TPU devices
* A notebook that you can run on a free-tier Google Colab instance to perform SFT on the English quotes dataset
### Inputs and outputs
* **Input:** Text string, such as a question, a prompt, or a document to be
summarized.
* **Output:** Generated English-language text in response to the input, such
as an answer to a question, or a summary of a document.
## Model Data
Data used for model training and how the data was processed.
### Training Dataset
These models were trained on a dataset of text data that includes a wide variety
of sources, totaling 6 trillion tokens. Here are the key components:
* Web Documents: A diverse collection of web text ensures the model is exposed
to a broad range of linguistic styles, topics, and vocabulary. Primarily
English-language content.
* Code: Exposing the model to code helps it to learn the syntax and patterns of
programming languages, which improves its ability to generate code or
understand code-related questions.
* Mathematics: Training on mathematical text helps the model learn logical
reasoning, symbolic representation, and to address mathematical queries.
The combination of these diverse data sources is crucial for training a powerful
language model that can handle a wide variety of different tasks and text
formats.
### Data Preprocessing
Here are the key data cleaning and filtering methods applied to the training
data:
* CSAM Filtering: Rigorous CSAM (Child Sexual Abuse Material) filtering was
applied at multiple stages in the data preparation process to ensure the
exclusion of harmful and illegal content
* Sensitive Data Filtering: As part of making Gemma pre-trained models safe and
reliable, automated techniques were used to filter out certain personal
information and other sensitive data from training sets.
* Additional methods: Filtering based on content quality and safely in line with
[our policies](https://storage.googleapis.com/gweb-uniblog-publish-prod/documents/2023_Google_AI_Principles_Progress_Update.pdf#page=11).
## Implementation Information
Details about the model internals.
### Hardware
Gemma was trained using the latest generation of
[Tensor Processing Unit (TPU)](https://cloud.google.com/tpu/docs/intro-to-tpu) hardware (TPUv5e).
Training large language models requires significant computational power. TPUs,
designed specifically for matrix operations common in machine learning, offer
several advantages in this domain:
* Performance: TPUs are specifically designed to handle the massive computations
involved in training LLMs. They can speed up training considerably compared to
CPUs.
* Memory: TPUs often come with large amounts of high-bandwidth memory, allowing
for the handling of large models and batch sizes during training. This can
lead to better model quality.
* Scalability: TPU Pods (large clusters of TPUs) provide a scalable solution for
handling the growing complexity of large foundation models. You can distribute
training across multiple TPU devices for faster and more efficient processing.
* Cost-effectiveness: In many scenarios, TPUs can provide a more cost-effective
solution for training large models compared to CPU-based infrastructure,
especially when considering the time and resources saved due to faster
training.
* These advantages are aligned with
[Google's commitments to operate sustainably](https://sustainability.google/operating-sustainably/).
### Software
Training was done using [JAX](https://github.com/google/jax) and [ML Pathways](https://blog.google/technology/ai/introducing-pathways-next-generation-ai-architecture/ml-pathways).
JAX allows researchers to take advantage of the latest generation of hardware,
including TPUs, for faster and more efficient training of large models.
ML Pathways is Google's latest effort to build artificially intelligent systems
capable of generalizing across multiple tasks. This is specially suitable for
[foundation models](https://ai.google/discover/foundation-models/), including large language models like
these ones.
Together, JAX and ML Pathways are used as described in the
[paper about the Gemini family of models](https://arxiv.org/abs/2312.11805); "the 'single
controller' programming model of Jax and Pathways allows a single Python
process to orchestrate the entire training run, dramatically simplifying the
development workflow."
## Evaluation
Model evaluation metrics and results.
### Benchmark Results
The pre-trained base models were evaluated against a large collection of different datasets and
metrics to cover different aspects of text generation:
| Benchmark | Metric | 2B Params | 7B Params |
| ------------------------------ | ------------- | ----------- | --------- |
| [MMLU](https://arxiv.org/abs/2009.03300) | 5-shot, top-1 | 42.3 | 64.3 |
| [HellaSwag](https://arxiv.org/abs/1905.07830) | 0-shot |71.4 | 81.2 |
| [PIQA](https://arxiv.org/abs/1911.11641) | 0-shot | 77.3 | 81.2 |
| [SocialIQA](https://arxiv.org/abs/1904.09728) | 0-shot | 49.7 | 51.8 |
| [BooIQ](https://arxiv.org/abs/1905.10044) | 0-shot | 69.4 | 83.2 |
| [WinoGrande](https://arxiv.org/abs/1907.10641) | partial score | 65.4 | 72.3 |
| [CommonsenseQA](https://arxiv.org/abs/1811.00937) | 7-shot | 65.3 | 71.3 |
| [OpenBookQA](https://arxiv.org/abs/1809.02789) | | 47.8 | 52.8 |
| [ARC-e](https://arxiv.org/abs/1911.01547) | | 73.2 | 81.5 |
| [ARC-c](https://arxiv.org/abs/1911.01547) | | 42.1 | 53.2 |
| [TriviaQA](https://arxiv.org/abs/1705.03551) | 5-shot | 53.2 | 63.4 |
| [Natural Questions](https://github.com/google-research-datasets/natural-questions) | 5-shot | 12.5 | 23 |
| [HumanEval](https://arxiv.org/abs/2107.03374) | pass@1 | 22.0 | 32.3 |
| [MBPP](https://arxiv.org/abs/2108.07732) | 3-shot | 29.2 | 44.4 |
| [GSM8K](https://arxiv.org/abs/2110.14168) | maj@1 | 17.7 | 46.4 |
| [MATH](https://arxiv.org/abs/2108.07732) | 4-shot | 11.8 | 24.3 |
| [AGIEval](https://arxiv.org/abs/2304.06364) | | 24.2 | 41.7 |
| [BIG-Bench](https://arxiv.org/abs/2206.04615) | | 35.2 | 55.1 |
| ------------------------------ | ------------- | ----------- | --------- |
| **Average** | | **45.0** | **56.9** |
## Ethics and Safety
Ethics and safety evaluation approach and results.
### Evaluation Approach
Our evaluation methods include structured evaluations and internal red-teaming
testing of relevant content policies. Red-teaming was conducted by a number of
different teams, each with different goals and human evaluation metrics. These
models were evaluated against a number of different categories relevant to
ethics and safety, including:
* Text-to-Text Content Safety: Human evaluation on prompts covering safety
policies including child sexual abuse and exploitation, harassment, violence
and gore, and hate speech.
* Text-to-Text Representational Harms: Benchmark against relevant academic
datasets such as [WinoBias](https://arxiv.org/abs/1804.06876) and [BBQ Dataset](https://arxiv.org/abs/2110.08193v2).
* Memorization: Automated evaluation of memorization of training data, including
the risk of personally identifiable information exposure.
* Large-scale harm: Tests for "dangerous capabilities," such as chemical,
biological, radiological, and nuclear (CBRN) risks.
### Evaluation Results
The results of ethics and safety evaluations are within acceptable thresholds
for meeting [internal policies](https://storage.googleapis.com/gweb-uniblog-publish-prod/documents/2023_Google_AI_Principles_Progress_Update.pdf#page=11) for categories such as child
safety, content safety, representational harms, memorization, large-scale harms.
On top of robust internal evaluations, the results of well known safety
benchmarks like BBQ, BOLD, Winogender, Winobias, RealToxicity, and TruthfulQA
are shown here.
#### Gemma 1.0
| Benchmark | Metric | Gemma 1.0 IT 2B | Gemma 1.0 IT 7B |
| ------------------------ | ------------- | --------------- | --------------- |
| [RealToxicity][realtox] | average | 6.86 | 7.90 |
| [BOLD][bold] | | 45.57 | 49.08 |
| [CrowS-Pairs][crows] | top-1 | 45.82 | 51.33 |
| [BBQ Ambig][bbq] | 1-shot, top-1 | 62.58 | 92.54 |
| [BBQ Disambig][bbq] | top-1 | 54.62 | 71.99 |
| [Winogender][winogender] | top-1 | 51.25 | 54.17 |
| [TruthfulQA][truthfulqa] | | 44.84 | 31.81 |
| [Winobias 1_2][winobias] | | 56.12 | 59.09 |
| [Winobias 2_2][winobias] | | 91.10 | 92.23 |
| [Toxigen][toxigen] | | 29.77 | 39.59 |
| ------------------------ | ------------- | --------------- | --------------- |
#### Gemma 1.1
| Benchmark | Metric | Gemma 1.1 IT 2B | Gemma 1.1 IT 7B |
| ------------------------ | ------------- | --------------- | --------------- |
| [RealToxicity][realtox] | average | 7.03 | 8.04 |
| [BOLD][bold] | | 47.76 | |
| [CrowS-Pairs][crows] | top-1 | 45.89 | 49.67 |
| [BBQ Ambig][bbq] | 1-shot, top-1 | 58.97 | 86.06 |
| [BBQ Disambig][bbq] | top-1 | 53.90 | 85.08 |
| [Winogender][winogender] | top-1 | 50.14 | 57.64 |
| [TruthfulQA][truthfulqa] | | 44.24 | 45.34 |
| [Winobias 1_2][winobias] | | 55.93 | 59.22 |
| [Winobias 2_2][winobias] | | 89.46 | 89.2 |
| [Toxigen][toxigen] | | 29.64 | 38.75 |
| ------------------------ | ------------- | --------------- | --------------- |
## Usage and Limitations
These models have certain limitations that users should be aware of.
### Intended Usage
Open Large Language Models (LLMs) have a wide range of applications across
various industries and domains. The following list of potential uses is not
comprehensive. The purpose of this list is to provide contextual information
about the possible use-cases that the model creators considered as part of model
training and development.
* Content Creation and Communication
* Text Generation: These models can be used to generate creative text formats
such as poems, scripts, code, marketing copy, and email drafts.
* Chatbots and Conversational AI: Power conversational interfaces for customer
service, virtual assistants, or interactive applications.
* Text Summarization: Generate concise summaries of a text corpus, research
papers, or reports.
* Research and Education
* Natural Language Processing (NLP) Research: These models can serve as a
foundation for researchers to experiment with NLP techniques, develop
algorithms, and contribute to the advancement of the field.
* Language Learning Tools: Support interactive language learning experiences,
aiding in grammar correction or providing writing practice.
* Knowledge Exploration: Assist researchers in exploring large bodies of text
by generating summaries or answering questions about specific topics.
### Limitations
* Training Data
* The quality and diversity of the training data significantly influence the
model's capabilities. Biases or gaps in the training data can lead to
limitations in the model's responses.
* The scope of the training dataset determines the subject areas the model can
handle effectively.
* Context and Task Complexity
* LLMs are better at tasks that can be framed with clear prompts and
instructions. Open-ended or highly complex tasks might be challenging.
* A model's performance can be influenced by the amount of context provided
(longer context generally leads to better outputs, up to a certain point).
* Language Ambiguity and Nuance
* Natural language is inherently complex. LLMs might struggle to grasp subtle
nuances, sarcasm, or figurative language.
* Factual Accuracy
* LLMs generate responses based on information they learned from their
training datasets, but they are not knowledge bases. They may generate
incorrect or outdated factual statements.
* Common Sense
* LLMs rely on statistical patterns in language. They might lack the ability
to apply common sense reasoning in certain situations.
### Ethical Considerations and Risks
The development of large language models (LLMs) raises several ethical concerns.
In creating an open model, we have carefully considered the following:
* Bias and Fairness
* LLMs trained on large-scale, real-world text data can reflect socio-cultural
biases embedded in the training material. These models underwent careful
scrutiny, input data pre-processing described and posterior evaluations
reported in this card.
* Misinformation and Misuse
* LLMs can be misused to generate text that is false, misleading, or harmful.
* Guidelines are provided for responsible use with the model, see the
[Responsible Generative AI Toolkit](http://ai.google.dev/gemma/responsible).
* Transparency and Accountability:
* This model card summarizes details on the models' architecture,
capabilities, limitations, and evaluation processes.
* A responsibly developed open model offers the opportunity to share
innovation by making LLM technology accessible to developers and researchers
across the AI ecosystem.
Risks identified and mitigations:
* Perpetuation of biases: It's encouraged to perform continuous monitoring
(using evaluation metrics, human review) and the exploration of de-biasing
techniques during model training, fine-tuning, and other use cases.
* Generation of harmful content: Mechanisms and guidelines for content safety
are essential. Developers are encouraged to exercise caution and implement
appropriate content safety safeguards based on their specific product policies
and application use cases.
* Misuse for malicious purposes: Technical limitations and developer and
end-user education can help mitigate against malicious applications of LLMs.
Educational resources and reporting mechanisms for users to flag misuse are
provided. Prohibited uses of Gemma models are outlined in the
[Gemma Prohibited Use Policy](https://ai.google.dev/gemma/prohibited_use_policy).
* Privacy violations: Models were trained on data filtered for removal of PII
(Personally Identifiable Information). Developers are encouraged to adhere to
privacy regulations with privacy-preserving techniques.
### Benefits
At the time of release, this family of models provides high-performance open
large language model implementations designed from the ground up for Responsible
AI development compared to similarly sized models.
Using the benchmark evaluation metrics described in this document, these models
have shown to provide superior performance to other, comparably-sized open model
alternatives.
|
laion/CLIP-ViT-g-14-laion2B-s12B-b42K | laion | "2024-02-23T17:06:28Z" | 102,460 | 37 | open_clip | [
"open_clip",
"pytorch",
"safetensors",
"clip",
"arxiv:1910.04867",
"license:mit",
"region:us"
] | null | "2022-09-14T22:53:40Z" | ---
license: mit
widget:
- src: https://huggingface.co/datasets/mishig/sample_images/resolve/main/cat-dog-music.png
candidate_labels: playing music, playing sports
example_title: Cat & Dog
---
# Model Card for CLIP ViT-g/14 - LAION-2B
# Table of Contents
1. [Model Details](#model-details)
2. [Uses](#uses)
3. [Training Details](#training-details)
4. [Evaluation](#evaluation)
5. [Acknowledgements](#acknowledgements)
6. [Citation](#citation)
7. [How To Get Started With the Model](#how-to-get-started-with-the-model)
# Model Details
## Model Description
A CLIP ViT-g/14 model trained with the LAION-2B English subset of LAION-5B (https://laion.ai/blog/laion-5b/) using OpenCLIP (https://github.com/mlfoundations/open_clip).
Model training done by Romain Beaumont on the [stability.ai](https://stability.ai/) cluster.
# Uses
As per the original [OpenAI CLIP model card](https://github.com/openai/CLIP/blob/d50d76daa670286dd6cacf3bcd80b5e4823fc8e1/model-card.md), this model is intended as a research output for research communities. We hope that this model will enable researchers to better understand and explore zero-shot, arbitrary image classification. We also hope it can be used for interdisciplinary studies of the potential impact of such model.
The OpenAI CLIP paper includes a discussion of potential downstream impacts to provide an example for this sort of analysis. Additionally, the LAION-5B blog (https://laion.ai/blog/laion-5b/) and upcoming paper include additional discussion as it relates specifically to the training dataset.
## Direct Use
Zero-shot image classification, image and text retrieval, among others.
## Downstream Use
Image classification and other image task fine-tuning, linear probe image classification, image generation guiding and conditioning, among others.
## Out-of-Scope Use
As per the OpenAI models,
**Any** deployed use case of the model - whether commercial or not - is currently out of scope. Non-deployed use cases such as image search in a constrained environment, are also not recommended unless there is thorough in-domain testing of the model with a specific, fixed class taxonomy. This is because our safety assessment demonstrated a high need for task specific testing especially given the variability of CLIP’s performance with different class taxonomies. This makes untested and unconstrained deployment of the model in any use case currently potentially harmful.
Certain use cases which would fall under the domain of surveillance and facial recognition are always out-of-scope regardless of performance of the model. This is because the use of artificial intelligence for tasks such as these can be premature currently given the lack of testing norms and checks to ensure its fair use.
Since the model has not been purposefully trained in or evaluated on any languages other than English, its use should be limited to English language use cases.
Further the above notice, the LAION-5B dataset used in training of these models has additional considerations, see below.
# Training Details
## Training Data
This model was trained with the 2 Billion sample English subset of LAION-5B (https://laion.ai/blog/laion-5b/).
**IMPORTANT NOTE:** The motivation behind dataset creation is to democratize research and experimentation around large-scale multi-modal model training and handling of uncurated, large-scale datasets crawled from publically available internet. Our recommendation is therefore to use the dataset for research purposes. Be aware that this large-scale dataset is uncurated. Keep in mind that the uncurated nature of the dataset means that collected links may lead to strongly discomforting and disturbing content for a human viewer. Therefore, please use the demo links with caution and at your own risk. It is possible to extract a “safe” subset by filtering out samples based on the safety tags (using a customized trained NSFW classifier that we built). While this strongly reduces the chance for encountering potentially harmful content when viewing, we cannot entirely exclude the possibility for harmful content being still present in safe mode, so that the warning holds also there. We think that providing the dataset openly to broad research and other interested communities will allow for transparent investigation of benefits that come along with training large-scale models as well as pitfalls and dangers that may stay unreported or unnoticed when working with closed large datasets that remain restricted to a small community. Providing our dataset openly, we however do not recommend using it for creating ready-to-go industrial products, as the basic research about general properties and safety of such large-scale models, which we would like to encourage with this release, is still in progress.
## Training Procedure
Please see [training notes](https://docs.google.com/document/d/1EFbMLRWSSV0LUf9Du1pWzWqgeiIRPwEWX2s1C6mAk5c) and [wandb logs](https://wandb.ai/rom1504/eval_openclip/reports/slow-g-14--VmlldzoyNTMwMjg5).
# Evaluation
Evaluation done with code in the [LAION CLIP Benchmark suite](https://github.com/LAION-AI/CLIP_benchmark).
## Testing Data, Factors & Metrics
### Testing Data
The testing is performed with VTAB+ (A combination of VTAB (https://arxiv.org/abs/1910.04867) w/ additional robustness datasets) for classification and COCO and Flickr for retrieval.
**TODO** - more detail
## Results
The model achieves a 76.6 zero-shot top-1 accuracy on ImageNet-1k.
An initial round of benchmarks have been performed on a wider range of datasets, currently viewable at https://github.com/LAION-AI/CLIP_benchmark/blob/main/benchmark/results.ipynb
**TODO** - create table for just this model's metrics.
# Acknowledgements
Acknowledging [stability.ai](https://stability.ai/) for the compute used to train this model.
# Citation
**BibTeX:**
In addition to forthcoming LAION-5B (https://laion.ai/blog/laion-5b/) paper, please cite:
OpenAI CLIP paper
```
@inproceedings{Radford2021LearningTV,
title={Learning Transferable Visual Models From Natural Language Supervision},
author={Alec Radford and Jong Wook Kim and Chris Hallacy and A. Ramesh and Gabriel Goh and Sandhini Agarwal and Girish Sastry and Amanda Askell and Pamela Mishkin and Jack Clark and Gretchen Krueger and Ilya Sutskever},
booktitle={ICML},
year={2021}
}
```
OpenCLIP software
```
@software{ilharco_gabriel_2021_5143773,
author = {Ilharco, Gabriel and
Wortsman, Mitchell and
Wightman, Ross and
Gordon, Cade and
Carlini, Nicholas and
Taori, Rohan and
Dave, Achal and
Shankar, Vaishaal and
Namkoong, Hongseok and
Miller, John and
Hajishirzi, Hannaneh and
Farhadi, Ali and
Schmidt, Ludwig},
title = {OpenCLIP},
month = jul,
year = 2021,
note = {If you use this software, please cite it as below.},
publisher = {Zenodo},
version = {0.1},
doi = {10.5281/zenodo.5143773},
url = {https://doi.org/10.5281/zenodo.5143773}
}
```
# How to Get Started with the Model
Use the code below to get started with the model.
** TODO ** - Hugging Face transformers, OpenCLIP, and timm getting started snippets |
TencentARC/InstantMesh | TencentARC | "2024-04-11T02:56:23Z" | 101,906 | 146 | diffusers | [
"diffusers",
"image-to-3d",
"arxiv:2404.07191",
"license:apache-2.0",
"region:us"
] | image-to-3d | "2024-04-10T13:16:45Z" | ---
license: apache-2.0
tags:
- image-to-3d
---
# InstantMesh
Model card for *InstantMesh: Efficient 3D Mesh Generation from a Single Image with Sparse-view Large Reconstruction Models*.
Code: https://github.com/TencentARC/InstantMesh
Arxiv: https://arxiv.org/abs/2404.07191
We present InstantMesh, a feed-forward framework for instant 3D mesh generation from a single image, featuring state-of-the-art generation quality and significant training scalability. By synergizing the strengths of an off-the-shelf multiview diffusion model and a sparse-view reconstruction model based on the LRM architecture, InstantMesh is able to create diverse 3D assets within 10 seconds. To enhance the training efficiency and exploit more geometric supervisions, e.g., depths and normals, we integrate a differentiable iso-surface extraction module into our framework and directly optimize on the mesh representation. Experimental results on public datasets demonstrate that InstantMesh significantly outperforms other latest image-to-3D baselines, both qualitatively and quantitatively. We release all the code, weights, and demo of InstantMesh, with the intention that it can make substantial contributions to the community of 3D generative AI and empower both researchers and content creators.
|
mradermacher/cerberus-v0.1-GGUF | mradermacher | "2024-06-28T15:38:02Z" | 101,564 | 0 | transformers | [
"transformers",
"gguf",
"en",
"base_model:brahmairesearch/cerberus-v0.1",
"license:llama3",
"endpoints_compatible",
"region:us"
] | null | "2024-06-28T05:18:58Z" | ---
base_model: brahmairesearch/cerberus-v0.1
language:
- en
library_name: transformers
license: llama3
quantized_by: mradermacher
---
## About
<!-- ### quantize_version: 2 -->
<!-- ### output_tensor_quantised: 1 -->
<!-- ### convert_type: hf -->
<!-- ### vocab_type: -->
<!-- ### tags: -->
static quants of https://huggingface.co/brahmairesearch/cerberus-v0.1
<!-- provided-files -->
weighted/imatrix quants are available at https://huggingface.co/mradermacher/cerberus-v0.1-i1-GGUF
## Usage
If you are unsure how to use GGUF files, refer to one of [TheBloke's
READMEs](https://huggingface.co/TheBloke/KafkaLM-70B-German-V0.1-GGUF) for
more details, including on how to concatenate multi-part files.
## Provided Quants
(sorted by size, not necessarily quality. IQ-quants are often preferable over similar sized non-IQ quants)
| Link | Type | Size/GB | Notes |
|:-----|:-----|--------:|:------|
| [GGUF](https://huggingface.co/mradermacher/cerberus-v0.1-GGUF/resolve/main/cerberus-v0.1.Q2_K.gguf) | Q2_K | 26.5 | |
| [GGUF](https://huggingface.co/mradermacher/cerberus-v0.1-GGUF/resolve/main/cerberus-v0.1.IQ3_XS.gguf) | IQ3_XS | 29.4 | |
| [GGUF](https://huggingface.co/mradermacher/cerberus-v0.1-GGUF/resolve/main/cerberus-v0.1.IQ3_S.gguf) | IQ3_S | 31.0 | beats Q3_K* |
| [GGUF](https://huggingface.co/mradermacher/cerberus-v0.1-GGUF/resolve/main/cerberus-v0.1.Q3_K_S.gguf) | Q3_K_S | 31.0 | |
| [GGUF](https://huggingface.co/mradermacher/cerberus-v0.1-GGUF/resolve/main/cerberus-v0.1.IQ3_M.gguf) | IQ3_M | 32.0 | |
| [GGUF](https://huggingface.co/mradermacher/cerberus-v0.1-GGUF/resolve/main/cerberus-v0.1.Q3_K_M.gguf) | Q3_K_M | 34.4 | lower quality |
| [GGUF](https://huggingface.co/mradermacher/cerberus-v0.1-GGUF/resolve/main/cerberus-v0.1.Q3_K_L.gguf) | Q3_K_L | 37.2 | |
| [GGUF](https://huggingface.co/mradermacher/cerberus-v0.1-GGUF/resolve/main/cerberus-v0.1.IQ4_XS.gguf) | IQ4_XS | 38.4 | |
| [GGUF](https://huggingface.co/mradermacher/cerberus-v0.1-GGUF/resolve/main/cerberus-v0.1.Q4_K_S.gguf) | Q4_K_S | 40.4 | fast, recommended |
| [GGUF](https://huggingface.co/mradermacher/cerberus-v0.1-GGUF/resolve/main/cerberus-v0.1.Q4_K_M.gguf) | Q4_K_M | 42.6 | fast, recommended |
| [GGUF](https://huggingface.co/mradermacher/cerberus-v0.1-GGUF/resolve/main/cerberus-v0.1.Q5_K_S.gguf) | Q5_K_S | 48.8 | |
| [GGUF](https://huggingface.co/mradermacher/cerberus-v0.1-GGUF/resolve/main/cerberus-v0.1.Q5_K_M.gguf) | Q5_K_M | 50.0 | |
| [PART 1](https://huggingface.co/mradermacher/cerberus-v0.1-GGUF/resolve/main/cerberus-v0.1.Q6_K.gguf.part1of2) [PART 2](https://huggingface.co/mradermacher/cerberus-v0.1-GGUF/resolve/main/cerberus-v0.1.Q6_K.gguf.part2of2) | Q6_K | 58.0 | very good quality |
| [PART 1](https://huggingface.co/mradermacher/cerberus-v0.1-GGUF/resolve/main/cerberus-v0.1.Q8_0.gguf.part1of2) [PART 2](https://huggingface.co/mradermacher/cerberus-v0.1-GGUF/resolve/main/cerberus-v0.1.Q8_0.gguf.part2of2) | Q8_0 | 75.1 | fast, best quality |
Here is a handy graph by ikawrakow comparing some lower-quality quant
types (lower is better):

And here are Artefact2's thoughts on the matter:
https://gist.github.com/Artefact2/b5f810600771265fc1e39442288e8ec9
## FAQ / Model Request
See https://huggingface.co/mradermacher/model_requests for some answers to
questions you might have and/or if you want some other model quantized.
## Thanks
I thank my company, [nethype GmbH](https://www.nethype.de/), for letting
me use its servers and providing upgrades to my workstation to enable
this work in my free time.
<!-- end -->
|
mradermacher/QuartetAnemoi-70B-t0.0001-i1-GGUF | mradermacher | "2024-06-29T05:19:45Z" | 101,305 | 0 | transformers | [
"transformers",
"gguf",
"merge",
"en",
"base_model:alchemonaut/QuartetAnemoi-70B-t0.0001",
"license:other",
"endpoints_compatible",
"region:us"
] | null | "2024-06-28T16:08:56Z" | ---
base_model: alchemonaut/QuartetAnemoi-70B-t0.0001
language:
- en
library_name: transformers
license: other
quantized_by: mradermacher
tags:
- merge
---
## About
<!-- ### quantize_version: 2 -->
<!-- ### output_tensor_quantised: 1 -->
<!-- ### convert_type: hf -->
<!-- ### vocab_type: -->
<!-- ### tags: nicoboss -->
weighted/imatrix quants of https://huggingface.co/alchemonaut/QuartetAnemoi-70B-t0.0001
<!-- provided-files -->
static quants are available at https://huggingface.co/mradermacher/QuartetAnemoi-70B-t0.0001-GGUF
## Usage
If you are unsure how to use GGUF files, refer to one of [TheBloke's
READMEs](https://huggingface.co/TheBloke/KafkaLM-70B-German-V0.1-GGUF) for
more details, including on how to concatenate multi-part files.
## Provided Quants
(sorted by size, not necessarily quality. IQ-quants are often preferable over similar sized non-IQ quants)
| Link | Type | Size/GB | Notes |
|:-----|:-----|--------:|:------|
| [GGUF](https://huggingface.co/mradermacher/QuartetAnemoi-70B-t0.0001-i1-GGUF/resolve/main/QuartetAnemoi-70B-t0.0001.i1-IQ1_S.gguf) | i1-IQ1_S | 14.6 | for the desperate |
| [GGUF](https://huggingface.co/mradermacher/QuartetAnemoi-70B-t0.0001-i1-GGUF/resolve/main/QuartetAnemoi-70B-t0.0001.i1-IQ1_M.gguf) | i1-IQ1_M | 16.0 | mostly desperate |
| [GGUF](https://huggingface.co/mradermacher/QuartetAnemoi-70B-t0.0001-i1-GGUF/resolve/main/QuartetAnemoi-70B-t0.0001.i1-IQ2_XXS.gguf) | i1-IQ2_XXS | 18.4 | |
| [GGUF](https://huggingface.co/mradermacher/QuartetAnemoi-70B-t0.0001-i1-GGUF/resolve/main/QuartetAnemoi-70B-t0.0001.i1-IQ2_XS.gguf) | i1-IQ2_XS | 20.4 | |
| [GGUF](https://huggingface.co/mradermacher/QuartetAnemoi-70B-t0.0001-i1-GGUF/resolve/main/QuartetAnemoi-70B-t0.0001.i1-IQ2_S.gguf) | i1-IQ2_S | 21.5 | |
| [GGUF](https://huggingface.co/mradermacher/QuartetAnemoi-70B-t0.0001-i1-GGUF/resolve/main/QuartetAnemoi-70B-t0.0001.i1-IQ2_M.gguf) | i1-IQ2_M | 23.3 | |
| [GGUF](https://huggingface.co/mradermacher/QuartetAnemoi-70B-t0.0001-i1-GGUF/resolve/main/QuartetAnemoi-70B-t0.0001.i1-Q2_K.gguf) | i1-Q2_K | 25.6 | IQ3_XXS probably better |
| [GGUF](https://huggingface.co/mradermacher/QuartetAnemoi-70B-t0.0001-i1-GGUF/resolve/main/QuartetAnemoi-70B-t0.0001.i1-IQ3_XXS.gguf) | i1-IQ3_XXS | 26.7 | lower quality |
| [GGUF](https://huggingface.co/mradermacher/QuartetAnemoi-70B-t0.0001-i1-GGUF/resolve/main/QuartetAnemoi-70B-t0.0001.i1-IQ3_XS.gguf) | i1-IQ3_XS | 28.4 | |
| [GGUF](https://huggingface.co/mradermacher/QuartetAnemoi-70B-t0.0001-i1-GGUF/resolve/main/QuartetAnemoi-70B-t0.0001.i1-IQ3_S.gguf) | i1-IQ3_S | 30.0 | beats Q3_K* |
| [GGUF](https://huggingface.co/mradermacher/QuartetAnemoi-70B-t0.0001-i1-GGUF/resolve/main/QuartetAnemoi-70B-t0.0001.i1-Q3_K_S.gguf) | i1-Q3_K_S | 30.0 | IQ3_XS probably better |
| [GGUF](https://huggingface.co/mradermacher/QuartetAnemoi-70B-t0.0001-i1-GGUF/resolve/main/QuartetAnemoi-70B-t0.0001.i1-IQ3_M.gguf) | i1-IQ3_M | 31.0 | |
| [GGUF](https://huggingface.co/mradermacher/QuartetAnemoi-70B-t0.0001-i1-GGUF/resolve/main/QuartetAnemoi-70B-t0.0001.i1-Q3_K_M.gguf) | i1-Q3_K_M | 33.4 | IQ3_S probably better |
| [GGUF](https://huggingface.co/mradermacher/QuartetAnemoi-70B-t0.0001-i1-GGUF/resolve/main/QuartetAnemoi-70B-t0.0001.i1-Q3_K_L.gguf) | i1-Q3_K_L | 36.2 | IQ3_M probably better |
| [GGUF](https://huggingface.co/mradermacher/QuartetAnemoi-70B-t0.0001-i1-GGUF/resolve/main/QuartetAnemoi-70B-t0.0001.i1-IQ4_XS.gguf) | i1-IQ4_XS | 36.9 | |
| [GGUF](https://huggingface.co/mradermacher/QuartetAnemoi-70B-t0.0001-i1-GGUF/resolve/main/QuartetAnemoi-70B-t0.0001.i1-Q4_0.gguf) | i1-Q4_0 | 39.1 | fast, low quality |
| [GGUF](https://huggingface.co/mradermacher/QuartetAnemoi-70B-t0.0001-i1-GGUF/resolve/main/QuartetAnemoi-70B-t0.0001.i1-Q4_K_S.gguf) | i1-Q4_K_S | 39.3 | optimal size/speed/quality |
| [GGUF](https://huggingface.co/mradermacher/QuartetAnemoi-70B-t0.0001-i1-GGUF/resolve/main/QuartetAnemoi-70B-t0.0001.i1-Q4_K_M.gguf) | i1-Q4_K_M | 41.5 | fast, recommended |
| [GGUF](https://huggingface.co/mradermacher/QuartetAnemoi-70B-t0.0001-i1-GGUF/resolve/main/QuartetAnemoi-70B-t0.0001.i1-Q5_K_S.gguf) | i1-Q5_K_S | 47.6 | |
| [GGUF](https://huggingface.co/mradermacher/QuartetAnemoi-70B-t0.0001-i1-GGUF/resolve/main/QuartetAnemoi-70B-t0.0001.i1-Q5_K_M.gguf) | i1-Q5_K_M | 48.9 | |
| [PART 1](https://huggingface.co/mradermacher/QuartetAnemoi-70B-t0.0001-i1-GGUF/resolve/main/QuartetAnemoi-70B-t0.0001.i1-Q6_K.gguf.part1of2) [PART 2](https://huggingface.co/mradermacher/QuartetAnemoi-70B-t0.0001-i1-GGUF/resolve/main/QuartetAnemoi-70B-t0.0001.i1-Q6_K.gguf.part2of2) | i1-Q6_K | 56.7 | practically like static Q6_K |
Here is a handy graph by ikawrakow comparing some lower-quality quant
types (lower is better):

And here are Artefact2's thoughts on the matter:
https://gist.github.com/Artefact2/b5f810600771265fc1e39442288e8ec9
## FAQ / Model Request
See https://huggingface.co/mradermacher/model_requests for some answers to
questions you might have and/or if you want some other model quantized.
## Thanks
I thank my company, [nethype GmbH](https://www.nethype.de/), for letting
me use its servers and providing upgrades to my workstation to enable
this work in my free time. Additional thanks to [@nicoboss](https://huggingface.co/nicoboss) for giving me access to his hardware for calculating the imatrix for these quants.
<!-- end -->
|
nguyenvulebinh/wav2vec2-base-vi | nguyenvulebinh | "2023-08-04T05:25:42Z" | 101,179 | 4 | transformers | [
"transformers",
"pytorch",
"wav2vec2",
"pretraining",
"speech",
"vi",
"dataset:youtube-vi-13k-hours",
"license:cc-by-nc-4.0",
"endpoints_compatible",
"region:us"
] | null | "2022-11-04T12:57:55Z" | ---
language: vi
datasets:
- youtube-vi-13k-hours
tags:
- speech
license: cc-by-nc-4.0
---
# Vietnamese Self-Supervised Learning Wav2Vec2 model
## Model
We use wav2vec2 architecture for doing Self-Supervised learning
<img src="https://raw.githubusercontent.com/patrickvonplaten/scientific_images/master/wav2vec2.png" width=75% height=75%>
## Data
Our self-supervised model is pre-trained on a massive audio set of 13k hours of Vietnamese youtube audio, which includes:
- Clean audio
- Noise audio
- Conversation
- Multi-gender and dialects
## Download
We have already upload our pre-trained model to the Huggingface. The base model trained 35 epochs and the large model trained 20 epochs in about 30 days using TPU V3-8.
- [Based version](https://huggingface.co/nguyenvulebinh/wav2vec2-base-vi) ~ 95M params
- [Large version](https://huggingface.co/nguyenvulebinh/wav2vec2-large-vi) ~ 317M params
## Usage
```python
from transformers import Wav2Vec2ForPreTraining, Wav2Vec2Processor
model_name = 'nguyenvulebinh/wav2vec2-base-vi'
# model_name = 'nguyenvulebinh/wav2vec2-large-vi'
model = Wav2Vec2ForPreTraining.from_pretrained(model_name)
processor = Wav2Vec2Processor.from_pretrained(model_name)
```
Since our model has the same architecture as the English wav2vec2 version, you can use [this notebook](https://colab.research.google.com/drive/1FjTsqbYKphl9kL-eILgUc-bl4zVThL8F?usp=sharing) for more information on how to fine-tune the model.
## Finetuned version
### VLSP 2020 ASR dataset
Benchmark WER result on VLSP T1 testset:
| | [base model](https://huggingface.co/nguyenvulebinh/wav2vec2-base-vi-vlsp2020) | [large model](https://huggingface.co/nguyenvulebinh/wav2vec2-large-vi-vlsp2020) |
|---|---|---|
|without LM| 8.66 | 6.90 |
|with 5-grams LM| 6.53 | 5.32 |
Usage
```python
#pytorch
#!pip install transformers==4.20.0
#!pip install https://github.com/kpu/kenlm/archive/master.zip
#!pip install pyctcdecode==0.4.0
from transformers.file_utils import cached_path, hf_bucket_url
from importlib.machinery import SourceFileLoader
from transformers import Wav2Vec2ProcessorWithLM
from IPython.lib.display import Audio
import torchaudio
import torch
# Load model & processor
model_name = "nguyenvulebinh/wav2vec2-base-vi-vlsp2020"
# model_name = "nguyenvulebinh/wav2vec2-large-vi-vlsp2020"
model = SourceFileLoader("model", cached_path(hf_bucket_url(model_name,filename="model_handling.py"))).load_module().Wav2Vec2ForCTC.from_pretrained(model_name)
processor = Wav2Vec2ProcessorWithLM.from_pretrained(model_name)
# Load an example audio (16k)
audio, sample_rate = torchaudio.load(cached_path(hf_bucket_url(model_name, filename="t2_0000006682.wav")))
input_data = processor.feature_extractor(audio[0], sampling_rate=16000, return_tensors='pt')
# Infer
output = model(**input_data)
# Output transcript without LM
print(processor.tokenizer.decode(output.logits.argmax(dim=-1)[0].detach().cpu().numpy()))
# Output transcript with LM
print(processor.decode(output.logits.cpu().detach().numpy()[0], beam_width=100).text)
```
## Acknowledgment
- We would like to thank the Google TPU Research Cloud (TRC) program and Soonson Kwon (Google ML Ecosystem programs Lead) for their support.
- Special thanks to my colleagues at [VietAI](https://vietai.org/) and [VAIS](https://vais.vn/) for their advice.
## Contact
nguyenvulebinh@gmail.com / binh@vietai.org
[](https://twitter.com/intent/follow?screen_name=nguyenvulebinh)
|
RichardErkhov/abhishek_-_autotrain-llama3-70b-orpo-v1-gguf | RichardErkhov | "2024-06-28T07:20:38Z" | 100,853 | 0 | null | [
"gguf",
"region:us"
] | null | "2024-06-27T20:39:51Z" | Entry not found |
sentence-transformers/sentence-t5-large | sentence-transformers | "2024-03-27T12:44:21Z" | 100,801 | 17 | sentence-transformers | [
"sentence-transformers",
"pytorch",
"safetensors",
"t5",
"feature-extraction",
"sentence-similarity",
"en",
"arxiv:2108.08877",
"license:apache-2.0",
"autotrain_compatible",
"endpoints_compatible",
"region:us"
] | sentence-similarity | "2022-03-02T23:29:05Z" | ---
language: en
license: apache-2.0
library_name: sentence-transformers
tags:
- sentence-transformers
- feature-extraction
- sentence-similarity
pipeline_tag: sentence-similarity
---
# sentence-transformers/sentence-t5-large
This is a [sentence-transformers](https://www.SBERT.net) model: It maps sentences & paragraphs to a 768 dimensional dense vector space. The model works well for sentence similarity tasks, but doesn't perform that well for semantic search tasks.
This model was converted from the Tensorflow model [st5-large-1](https://tfhub.dev/google/sentence-t5/st5-large/1) to PyTorch. When using this model, have a look at the publication: [Sentence-T5: Scalable sentence encoders from pre-trained text-to-text models](https://arxiv.org/abs/2108.08877). The tfhub model and this PyTorch model can produce slightly different embeddings, however, when run on the same benchmarks, they produce identical results.
The model uses only the encoder from a T5-large model. The weights are stored in FP16.
## Usage (Sentence-Transformers)
Using this model becomes easy when you have [sentence-transformers](https://www.SBERT.net) installed:
```
pip install -U sentence-transformers
```
Then you can use the model like this:
```python
from sentence_transformers import SentenceTransformer
sentences = ["This is an example sentence", "Each sentence is converted"]
model = SentenceTransformer('sentence-transformers/sentence-t5-large')
embeddings = model.encode(sentences)
print(embeddings)
```
The model requires sentence-transformers version 2.2.0 or newer.
## Evaluation Results
For an automated evaluation of this model, see the *Sentence Embeddings Benchmark*: [https://seb.sbert.net](https://seb.sbert.net?model_name=sentence-transformers/sentence-t5-large)
## Citing & Authors
If you find this model helpful, please cite the respective publication:
[Sentence-T5: Scalable sentence encoders from pre-trained text-to-text models](https://arxiv.org/abs/2108.08877)
|
internlm/internlm-xcomposer2-vl-7b | internlm | "2024-04-12T06:03:50Z" | 100,801 | 71 | transformers | [
"transformers",
"pytorch",
"internlmxcomposer2",
"feature-extraction",
"visual-question-answering",
"custom_code",
"arxiv:2401.16420",
"license:other",
"region:us"
] | visual-question-answering | "2024-01-25T09:01:09Z" | ---
license: other
pipeline_tag: visual-question-answering
---
<p align="center">
<img src="logo_en.png" width="400"/>
<p>
<p align="center">
<b><font size="6">InternLM-XComposer2</font></b>
<p>
<div align="center">
[💻Github Repo](https://github.com/InternLM/InternLM-XComposer)
[Paper](https://arxiv.org/abs/2401.16420)
</div>
**InternLM-XComposer2** is a vision-language large model (VLLM) based on [InternLM2](https://github.com/InternLM/InternLM) for advanced text-image comprehension and composition.
We release InternLM-XComposer2 series in two versions:
- InternLM-XComposer2-VL: The pretrained VLLM model with InternLM2 as the initialization of the LLM, achieving strong performance on various multimodal benchmarks.
- InternLM-XComposer2: The finetuned VLLM for *Free-from Interleaved Text-Image Composition*.
### Import from Transformers
To load the InternLM-XComposer2-VL-7B model using Transformers, use the following code:
```python
import torch
from transformers import AutoTokenizer, AutoModelForCausalLM
ckpt_path = "internlm/internlm-xcomposer2-vl-7b"
tokenizer = AutoTokenizer.from_pretrained(ckpt_path, trust_remote_code=True).cuda()
# Set `torch_dtype=torch.float16` to load model in float16, otherwise it will be loaded as float32 and might cause OOM Error.
model = AutoModelForCausalLM.from_pretrained(ckpt_path, torch_dtype=torch.float16, trust_remote_code=True).cuda()
model = model.eval()
```
## Quickstart
We provide a simple example to show how to use InternLM-XComposer with 🤗 Transformers.
```python
import torch
from transformers import AutoModel, AutoTokenizer
torch.set_grad_enabled(False)
# init model and tokenizer
model = AutoModel.from_pretrained('internlm/internlm-xcomposer2-vl-7b', trust_remote_code=True).cuda().eval()
tokenizer = AutoTokenizer.from_pretrained('internlm/internlm-xcomposer2-vl-7b', trust_remote_code=True)
query = '<ImageHere>Please describe this image in detail.'
image = './image1.webp'
with torch.cuda.amp.autocast():
response, _ = model.chat(tokenizer, query=query, image=image, history=[], do_sample=False)
print(response)
#The image features a quote by Oscar Wilde, "Live life with no excuses, travel with no regret,"
# set against a backdrop of a breathtaking sunset. The sky is painted in hues of pink and orange,
# creating a serene atmosphere. Two silhouetted figures stand on a cliff, overlooking the horizon.
# They appear to be hiking or exploring, embodying the essence of the quote.
# The overall scene conveys a sense of adventure and freedom, encouraging viewers to embrace life without hesitation or regrets.
```
### Open Source License
The code is licensed under Apache-2.0, while model weights are fully open for academic research and also allow free commercial usage. To apply for a commercial license, please fill in the application form (English)/申请表(中文). For other questions or collaborations, please contact internlm@pjlab.org.cn. |
HuggingFaceM4/siglip-so400m-14-980-flash-attn2-navit | HuggingFaceM4 | "2024-03-07T22:05:47Z" | 100,605 | 33 | transformers | [
"transformers",
"safetensors",
"siglip",
"zero-shot-image-classification",
"custom_code",
"arxiv:2307.06304",
"license:apache-2.0",
"endpoints_compatible",
"region:us"
] | zero-shot-image-classification | "2024-01-30T19:31:08Z" | ---
license: apache-2.0
---
Same as https://huggingface.co/HuggingFaceM4/siglip-so400m-14-384-flash-attn2 with two changes:
- increase max resolution to 980 x 980 (instead of 384 x 384) by interpolating the position embeddings
- implement the strategy in [NaViT](https://arxiv.org/abs/2307.06304) to allow a/ variable resoltion images, b/ aspect ratio preserved images
These changes only apply to the vision tower. No changes to the text tower.
Implementation is fully backward compatible to `https://huggingface.co/HuggingFaceM4/siglip-so400m-14-384-flash-attn2` -> just don't specify the `patch_attention_mask`
Usage:
```python
import torch
from modeling_siglip import SiglipVisionModel
DEVICE = torch.device("cuda:0")
PATCH_SIZE = 14
pixel_values = torch.randn(2, 3, 28, 42, dtype=torch.bfloat16, device=DEVICE)
pixel_attention_mask = [
[
[1] * 14 + [1] * 14 + [1] * 14,
[1] * 14 + [1] * 14 + [1] * 14,
[1] * 14 + [1] * 14 + [1] * 14,
[1] * 14 + [1] * 14 + [1] * 14,
[1] * 14 + [1] * 14 + [1] * 14,
[1] * 14 + [1] * 14 + [1] * 14,
[1] * 14 + [1] * 14 + [1] * 14,
[1] * 14 + [1] * 14 + [1] * 14,
[1] * 14 + [1] * 14 + [1] * 14,
[1] * 14 + [1] * 14 + [1] * 14,
[1] * 14 + [1] * 14 + [1] * 14,
[1] * 14 + [1] * 14 + [1] * 14,
[1] * 14 + [1] * 14 + [1] * 14,
[1] * 14 + [1] * 14 + [1] * 14,
[0] * 14 + [0] * 14 + [0] * 14,
[0] * 14 + [0] * 14 + [0] * 14,
[0] * 14 + [0] * 14 + [0] * 14,
[0] * 14 + [0] * 14 + [0] * 14,
[0] * 14 + [0] * 14 + [0] * 14,
[0] * 14 + [0] * 14 + [0] * 14,
[0] * 14 + [0] * 14 + [0] * 14,
[0] * 14 + [0] * 14 + [0] * 14,
[0] * 14 + [0] * 14 + [0] * 14,
[0] * 14 + [0] * 14 + [0] * 14,
[0] * 14 + [0] * 14 + [0] * 14,
[0] * 14 + [0] * 14 + [0] * 14,
[0] * 14 + [0] * 14 + [0] * 14,
[0] * 14 + [0] * 14 + [0] * 14,
],
[
[1] * 14 + [1] * 14 + [0] * 14,
[1] * 14 + [1] * 14 + [0] * 14,
[1] * 14 + [1] * 14 + [0] * 14,
[1] * 14 + [1] * 14 + [0] * 14,
[1] * 14 + [1] * 14 + [0] * 14,
[1] * 14 + [1] * 14 + [0] * 14,
[1] * 14 + [1] * 14 + [0] * 14,
[1] * 14 + [1] * 14 + [0] * 14,
[1] * 14 + [1] * 14 + [0] * 14,
[1] * 14 + [1] * 14 + [0] * 14,
[1] * 14 + [1] * 14 + [0] * 14,
[1] * 14 + [1] * 14 + [0] * 14,
[1] * 14 + [1] * 14 + [0] * 14,
[1] * 14 + [1] * 14 + [0] * 14,
[1] * 14 + [1] * 14 + [0] * 14,
[1] * 14 + [1] * 14 + [0] * 14,
[1] * 14 + [1] * 14 + [0] * 14,
[1] * 14 + [1] * 14 + [0] * 14,
[1] * 14 + [1] * 14 + [0] * 14,
[1] * 14 + [1] * 14 + [0] * 14,
[1] * 14 + [1] * 14 + [0] * 14,
[1] * 14 + [1] * 14 + [0] * 14,
[1] * 14 + [1] * 14 + [0] * 14,
[1] * 14 + [1] * 14 + [0] * 14,
[1] * 14 + [1] * 14 + [0] * 14,
[1] * 14 + [1] * 14 + [0] * 14,
[1] * 14 + [1] * 14 + [0] * 14,
[1] * 14 + [1] * 14 + [0] * 14,
],
]
pixel_attention_mask = torch.tensor(pixel_attention_mask, dtype=torch.bool, device=DEVICE)
patches_subgrid = pixel_attention_mask.unfold(
dimension=1, size=PATCH_SIZE, step=PATCH_SIZE
).unfold(dimension=2, size=PATCH_SIZE, step=PATCH_SIZE)
patch_attention_mask = (patches_subgrid.sum(dim=(-1, -2)) > 0).bool()
model = SiglipVisionModel.from_pretrained("HuggingFaceM4/siglip-so400m-14-980-flash-attn2-navit", _flash_attn_2_enabled=True)
model.train()
model.vision_model.to(DEVICE, dtype=torch.bfloat16)
output = model.vision_model(pixel_values=pixel_values, patch_attention_mask=patch_attention_mask)
``` |
FacebookAI/xlm-roberta-large-finetuned-conll03-english | FacebookAI | "2024-02-19T12:48:53Z" | 100,466 | 107 | transformers | [
"transformers",
"pytorch",
"rust",
"onnx",
"safetensors",
"xlm-roberta",
"token-classification",
"multilingual",
"af",
"am",
"ar",
"as",
"az",
"be",
"bg",
"bn",
"br",
"bs",
"ca",
"cs",
"cy",
"da",
"de",
"el",
"en",
"eo",
"es",
"et",
"eu",
"fa",
"fi",
"fr",
"fy",
"ga",
"gd",
"gl",
"gu",
"ha",
"he",
"hi",
"hr",
"hu",
"hy",
"id",
"is",
"it",
"ja",
"jv",
"ka",
"kk",
"km",
"kn",
"ko",
"ku",
"ky",
"la",
"lo",
"lt",
"lv",
"mg",
"mk",
"ml",
"mn",
"mr",
"ms",
"my",
"ne",
"nl",
"no",
"om",
"or",
"pa",
"pl",
"ps",
"pt",
"ro",
"ru",
"sa",
"sd",
"si",
"sk",
"sl",
"so",
"sq",
"sr",
"su",
"sv",
"sw",
"ta",
"te",
"th",
"tl",
"tr",
"ug",
"uk",
"ur",
"uz",
"vi",
"xh",
"yi",
"zh",
"arxiv:1911.02116",
"arxiv:2008.03415",
"arxiv:1910.09700",
"autotrain_compatible",
"endpoints_compatible",
"region:us"
] | token-classification | "2022-03-02T23:29:04Z" | ---
language:
- multilingual
- af
- am
- ar
- as
- az
- be
- bg
- bn
- br
- bs
- ca
- cs
- cy
- da
- de
- el
- en
- eo
- es
- et
- eu
- fa
- fi
- fr
- fy
- ga
- gd
- gl
- gu
- ha
- he
- hi
- hr
- hu
- hy
- id
- is
- it
- ja
- jv
- ka
- kk
- km
- kn
- ko
- ku
- ky
- la
- lo
- lt
- lv
- mg
- mk
- ml
- mn
- mr
- ms
- my
- ne
- nl
- no
- om
- or
- pa
- pl
- ps
- pt
- ro
- ru
- sa
- sd
- si
- sk
- sl
- so
- sq
- sr
- su
- sv
- sw
- ta
- te
- th
- tl
- tr
- ug
- uk
- ur
- uz
- vi
- xh
- yi
- zh
---
# xlm-roberta-large-finetuned-conll03-english
# Table of Contents
1. [Model Details](#model-details)
2. [Uses](#uses)
3. [Bias, Risks, and Limitations](#bias-risks-and-limitations)
4. [Training](#training)
5. [Evaluation](#evaluation)
6. [Environmental Impact](#environmental-impact)
7. [Technical Specifications](#technical-specifications)
8. [Citation](#citation)
9. [Model Card Authors](#model-card-authors)
10. [How To Get Started With the Model](#how-to-get-started-with-the-model)
# Model Details
## Model Description
The XLM-RoBERTa model was proposed in [Unsupervised Cross-lingual Representation Learning at Scale](https://arxiv.org/abs/1911.02116) by Alexis Conneau, Kartikay Khandelwal, Naman Goyal, Vishrav Chaudhary, Guillaume Wenzek, Francisco Guzmán, Edouard Grave, Myle Ott, Luke Zettlemoyer and Veselin Stoyanov. It is based on Facebook's RoBERTa model released in 2019. It is a large multi-lingual language model, trained on 2.5TB of filtered CommonCrawl data. This model is [XLM-RoBERTa-large](https://huggingface.co/xlm-roberta-large) fine-tuned with the [conll2003](https://huggingface.co/datasets/conll2003) dataset in English.
- **Developed by:** See [associated paper](https://arxiv.org/abs/1911.02116)
- **Model type:** Multi-lingual language model
- **Language(s) (NLP) or Countries (images):** XLM-RoBERTa is a multilingual model trained on 100 different languages; see [GitHub Repo](https://github.com/facebookresearch/fairseq/tree/main/examples/xlmr) for full list; model is fine-tuned on a dataset in English
- **License:** More information needed
- **Related Models:** [RoBERTa](https://huggingface.co/roberta-base), [XLM](https://huggingface.co/docs/transformers/model_doc/xlm)
- **Parent Model:** [XLM-RoBERTa-large](https://huggingface.co/xlm-roberta-large)
- **Resources for more information:**
-[GitHub Repo](https://github.com/facebookresearch/fairseq/tree/main/examples/xlmr)
-[Associated Paper](https://arxiv.org/abs/1911.02116)
# Uses
## Direct Use
The model is a language model. The model can be used for token classification, a natural language understanding task in which a label is assigned to some tokens in a text.
## Downstream Use
Potential downstream use cases include Named Entity Recognition (NER) and Part-of-Speech (PoS) tagging. To learn more about token classification and other potential downstream use cases, see the Hugging Face [token classification docs](https://huggingface.co/tasks/token-classification).
## Out-of-Scope Use
The model should not be used to intentionally create hostile or alienating environments for people.
# Bias, Risks, and Limitations
**CONTENT WARNING: Readers should be made aware that language generated by this model may be disturbing or offensive to some and may propagate historical and current stereotypes.**
Significant research has explored bias and fairness issues with language models (see, e.g., [Sheng et al. (2021)](https://aclanthology.org/2021.acl-long.330.pdf) and [Bender et al. (2021)](https://dl.acm.org/doi/pdf/10.1145/3442188.3445922)). In the context of tasks relevant to this model, [Mishra et al. (2020)](https://arxiv.org/pdf/2008.03415.pdf) explore social biases in NER systems for English and find that there is systematic bias in existing NER systems in that they fail to identify named entities from different demographic groups (though this paper did not look at BERT). For example, using a sample sentence from [Mishra et al. (2020)](https://arxiv.org/pdf/2008.03415.pdf):
```python
>>> from transformers import pipeline
>>> tokenizer = AutoTokenizer.from_pretrained("xlm-roberta-large-finetuned-conll03-english")
>>> model = AutoModelForTokenClassification.from_pretrained("xlm-roberta-large-finetuned-conll03-english")
>>> classifier = pipeline("ner", model=model, tokenizer=tokenizer)
>>> classifier("Alya told Jasmine that Andrew could pay with cash..")
[{'end': 2,
'entity': 'I-PER',
'index': 1,
'score': 0.9997861,
'start': 0,
'word': '▁Al'},
{'end': 4,
'entity': 'I-PER',
'index': 2,
'score': 0.9998591,
'start': 2,
'word': 'ya'},
{'end': 16,
'entity': 'I-PER',
'index': 4,
'score': 0.99995816,
'start': 10,
'word': '▁Jasmin'},
{'end': 17,
'entity': 'I-PER',
'index': 5,
'score': 0.9999584,
'start': 16,
'word': 'e'},
{'end': 29,
'entity': 'I-PER',
'index': 7,
'score': 0.99998057,
'start': 23,
'word': '▁Andrew'}]
```
## Recommendations
Users (both direct and downstream) should be made aware of the risks, biases and limitations of the model.
# Training
See the following resources for training data and training procedure details:
- [XLM-RoBERTa-large model card](https://huggingface.co/xlm-roberta-large)
- [CoNLL-2003 data card](https://huggingface.co/datasets/conll2003)
- [Associated paper](https://arxiv.org/pdf/1911.02116.pdf)
# Evaluation
See the [associated paper](https://arxiv.org/pdf/1911.02116.pdf) for evaluation details.
# Environmental Impact
Carbon emissions can be estimated using the [Machine Learning Impact calculator](https://mlco2.github.io/impact#compute) presented in [Lacoste et al. (2019)](https://arxiv.org/abs/1910.09700).
- **Hardware Type:** 500 32GB Nvidia V100 GPUs (from the [associated paper](https://arxiv.org/pdf/1911.02116.pdf))
- **Hours used:** More information needed
- **Cloud Provider:** More information needed
- **Compute Region:** More information needed
- **Carbon Emitted:** More information needed
# Technical Specifications
See the [associated paper](https://arxiv.org/pdf/1911.02116.pdf) for further details.
# Citation
**BibTeX:**
```bibtex
@article{conneau2019unsupervised,
title={Unsupervised Cross-lingual Representation Learning at Scale},
author={Conneau, Alexis and Khandelwal, Kartikay and Goyal, Naman and Chaudhary, Vishrav and Wenzek, Guillaume and Guzm{\'a}n, Francisco and Grave, Edouard and Ott, Myle and Zettlemoyer, Luke and Stoyanov, Veselin},
journal={arXiv preprint arXiv:1911.02116},
year={2019}
}
```
**APA:**
- Conneau, A., Khandelwal, K., Goyal, N., Chaudhary, V., Wenzek, G., Guzmán, F., ... & Stoyanov, V. (2019). Unsupervised cross-lingual representation learning at scale. arXiv preprint arXiv:1911.02116.
# Model Card Authors
This model card was written by the team at Hugging Face.
# How to Get Started with the Model
Use the code below to get started with the model. You can use this model directly within a pipeline for NER.
<details>
<summary> Click to expand </summary>
```python
>>> from transformers import AutoTokenizer, AutoModelForTokenClassification
>>> from transformers import pipeline
>>> tokenizer = AutoTokenizer.from_pretrained("xlm-roberta-large-finetuned-conll03-english")
>>> model = AutoModelForTokenClassification.from_pretrained("xlm-roberta-large-finetuned-conll03-english")
>>> classifier = pipeline("ner", model=model, tokenizer=tokenizer)
>>> classifier("Hello I'm Omar and I live in Zürich.")
[{'end': 14,
'entity': 'I-PER',
'index': 5,
'score': 0.9999175,
'start': 10,
'word': '▁Omar'},
{'end': 35,
'entity': 'I-LOC',
'index': 10,
'score': 0.9999906,
'start': 29,
'word': '▁Zürich'}]
```
</details> |
RichardErkhov/moreh_-_MoMo-72B-lora-1.8.6-DPO-gguf | RichardErkhov | "2024-06-26T12:49:29Z" | 100,126 | 0 | null | [
"gguf",
"arxiv:2305.18290",
"arxiv:2106.09685",
"region:us"
] | null | "2024-06-25T03:04:54Z" | Quantization made by Richard Erkhov.
[Github](https://github.com/RichardErkhov)
[Discord](https://discord.gg/pvy7H8DZMG)
[Request more models](https://github.com/RichardErkhov/quant_request)
MoMo-72B-lora-1.8.6-DPO - GGUF
- Model creator: https://huggingface.co/moreh/
- Original model: https://huggingface.co/moreh/MoMo-72B-lora-1.8.6-DPO/
| Name | Quant method | Size |
| ---- | ---- | ---- |
| [MoMo-72B-lora-1.8.6-DPO.Q2_K.gguf](https://huggingface.co/RichardErkhov/moreh_-_MoMo-72B-lora-1.8.6-DPO-gguf/blob/main/MoMo-72B-lora-1.8.6-DPO.Q2_K.gguf) | Q2_K | 25.22GB |
| [MoMo-72B-lora-1.8.6-DPO.IQ3_XS.gguf](https://huggingface.co/RichardErkhov/moreh_-_MoMo-72B-lora-1.8.6-DPO-gguf/blob/main/MoMo-72B-lora-1.8.6-DPO.IQ3_XS.gguf) | IQ3_XS | 27.88GB |
| [MoMo-72B-lora-1.8.6-DPO.IQ3_S.gguf](https://huggingface.co/RichardErkhov/moreh_-_MoMo-72B-lora-1.8.6-DPO-gguf/blob/main/MoMo-72B-lora-1.8.6-DPO.IQ3_S.gguf) | IQ3_S | 29.4GB |
| [MoMo-72B-lora-1.8.6-DPO.Q3_K_S.gguf](https://huggingface.co/RichardErkhov/moreh_-_MoMo-72B-lora-1.8.6-DPO-gguf/blob/main/MoMo-72B-lora-1.8.6-DPO.Q3_K_S.gguf) | Q3_K_S | 29.4GB |
| [MoMo-72B-lora-1.8.6-DPO.IQ3_M.gguf](https://huggingface.co/RichardErkhov/moreh_-_MoMo-72B-lora-1.8.6-DPO-gguf/blob/main/MoMo-72B-lora-1.8.6-DPO.IQ3_M.gguf) | IQ3_M | 30.98GB |
| [MoMo-72B-lora-1.8.6-DPO.Q3_K.gguf](https://huggingface.co/RichardErkhov/moreh_-_MoMo-72B-lora-1.8.6-DPO-gguf/blob/main/MoMo-72B-lora-1.8.6-DPO.Q3_K.gguf) | Q3_K | 32.85GB |
| [MoMo-72B-lora-1.8.6-DPO.Q3_K_M.gguf](https://huggingface.co/RichardErkhov/moreh_-_MoMo-72B-lora-1.8.6-DPO-gguf/blob/main/MoMo-72B-lora-1.8.6-DPO.Q3_K_M.gguf) | Q3_K_M | 32.85GB |
| [MoMo-72B-lora-1.8.6-DPO.Q3_K_L.gguf](https://huggingface.co/RichardErkhov/moreh_-_MoMo-72B-lora-1.8.6-DPO-gguf/blob/main/MoMo-72B-lora-1.8.6-DPO.Q3_K_L.gguf) | Q3_K_L | 35.85GB |
| [MoMo-72B-lora-1.8.6-DPO.IQ4_XS.gguf](https://huggingface.co/RichardErkhov/moreh_-_MoMo-72B-lora-1.8.6-DPO-gguf/blob/main/MoMo-72B-lora-1.8.6-DPO.IQ4_XS.gguf) | IQ4_XS | 36.41GB |
| [MoMo-72B-lora-1.8.6-DPO.Q4_0.gguf](https://huggingface.co/RichardErkhov/moreh_-_MoMo-72B-lora-1.8.6-DPO-gguf/tree/main/) | Q4_0 | 38.19GB |
| [MoMo-72B-lora-1.8.6-DPO.IQ4_NL.gguf](https://huggingface.co/RichardErkhov/moreh_-_MoMo-72B-lora-1.8.6-DPO-gguf/tree/main/) | IQ4_NL | 38.42GB |
| [MoMo-72B-lora-1.8.6-DPO.Q4_K_S.gguf](https://huggingface.co/RichardErkhov/moreh_-_MoMo-72B-lora-1.8.6-DPO-gguf/tree/main/) | Q4_K_S | 38.45GB |
| [MoMo-72B-lora-1.8.6-DPO.Q4_K.gguf](https://huggingface.co/RichardErkhov/moreh_-_MoMo-72B-lora-1.8.6-DPO-gguf/tree/main/) | Q4_K | 40.77GB |
| [MoMo-72B-lora-1.8.6-DPO.Q4_K_M.gguf](https://huggingface.co/RichardErkhov/moreh_-_MoMo-72B-lora-1.8.6-DPO-gguf/tree/main/) | Q4_K_M | 40.77GB |
| [MoMo-72B-lora-1.8.6-DPO.Q4_1.gguf](https://huggingface.co/RichardErkhov/moreh_-_MoMo-72B-lora-1.8.6-DPO-gguf/tree/main/) | Q4_1 | 42.32GB |
| [MoMo-72B-lora-1.8.6-DPO.Q5_0.gguf](https://huggingface.co/RichardErkhov/moreh_-_MoMo-72B-lora-1.8.6-DPO-gguf/tree/main/) | Q5_0 | 46.46GB |
| [MoMo-72B-lora-1.8.6-DPO.Q5_K_S.gguf](https://huggingface.co/RichardErkhov/moreh_-_MoMo-72B-lora-1.8.6-DPO-gguf/tree/main/) | Q5_K_S | 46.46GB |
| [MoMo-72B-lora-1.8.6-DPO.Q5_K.gguf](https://huggingface.co/RichardErkhov/moreh_-_MoMo-72B-lora-1.8.6-DPO-gguf/tree/main/) | Q5_K | 47.79GB |
| [MoMo-72B-lora-1.8.6-DPO.Q5_K_M.gguf](https://huggingface.co/RichardErkhov/moreh_-_MoMo-72B-lora-1.8.6-DPO-gguf/tree/main/) | Q5_K_M | 47.79GB |
| [MoMo-72B-lora-1.8.6-DPO.Q5_1.gguf](https://huggingface.co/RichardErkhov/moreh_-_MoMo-72B-lora-1.8.6-DPO-gguf/tree/main/) | Q5_1 | 50.59GB |
| [MoMo-72B-lora-1.8.6-DPO.Q6_K.gguf](https://huggingface.co/RichardErkhov/moreh_-_MoMo-72B-lora-1.8.6-DPO-gguf/tree/main/) | Q6_K | 55.24GB |
| [MoMo-72B-lora-1.8.6-DPO.Q8_0.gguf](https://huggingface.co/RichardErkhov/moreh_-_MoMo-72B-lora-1.8.6-DPO-gguf/tree/main/) | Q8_0 | 71.55GB |
Original model description:
---
license: mit
language:
- en
---
# **Introduction**
MoMo-72B-lora-1.8.6-DPO is trained via Direct Preference Optimization([DPO](https://arxiv.org/abs/2305.18290)) from [MoMo-72B-LoRA-V1.4](https://huggingface.co/moreh/MoMo-72B-LoRA-V1.4) as its base model, with several optimizations in hyperparameters.
[MoMo-72B-LoRA-V1.4](https://huggingface.co/moreh/MoMo-72B-LoRA-V1.4) is trained via Supervised Fine-Tuning (SFT) using [LoRA](https://arxiv.org/abs/2106.09685), with the QWEN-72B model as its base-model.
Note that we did not exploit any form of weight merge.
For leaderboard submission, the trained weight is realigned for compatibility with llama.
MoMo-72B is trained using **[Moreh](https://moreh.io/)**'s [MoAI platform](https://moreh.io/product), which simplifies the training of large-scale models, and AMD's MI250 GPU.
## Details
### Used Librarys
- torch
- peft
### Used Datasets
- [slimorca](Open-Orca/SlimOrca)
- [truthy](https://huggingface.co/datasets/jondurbin/truthy-dpo-v0.1)
- [orca_dpo_pairs](https://huggingface.co/datasets/Intel/orca_dpo_pairs)
- No other dataset was used
- No benchmark test set or the training set are used
- [data contamination check](https://github.com/swj0419/detect-pretrain-code-contamination) result
| Model | ARC | MMLU | TruthfulQA | GSM8K |
|------------------------------|-------|-------|-------|-------|
| **V1.8.6(result < 0.1, %)**| TBU |TBU | 0.73 | TBU |
### Used Environments
- AMD MI250 & MoAI platform
- Please visit https://moreh.io/product for more information about MoAI platform
- Or, contact us directly [contact@moreh.io](mailto:contact@moreh.io)
## How to use
```python
# pip install transformers==4.35.2
import torch
from transformers import AutoModelForCausalLM, AutoTokenizer
tokenizer = AutoTokenizer.from_pretrained("moreh/MoMo-72B-lora-1.8.6-DPO")
model = AutoModelForCausalLM.from_pretrained(
"moreh/MoMo-72B-lora-1.8.6-DPO"
)
```
|
ptx0/terminus-xl-velocity-training | ptx0 | "2024-06-15T16:11:01Z" | 100,091 | 2 | diffusers | [
"diffusers",
"safetensors",
"stable-diffusion",
"stable-diffusion-diffusers",
"text-to-image",
"full",
"base_model:ptx0/terminus-xl-velocity-v2",
"license:creativeml-openrail-m",
"autotrain_compatible",
"endpoints_compatible",
"diffusers:StableDiffusionXLPipeline",
"region:us"
] | text-to-image | "2023-10-24T04:24:30Z" | ---
license: creativeml-openrail-m
base_model: "ptx0/terminus-xl-velocity-v2"
tags:
- stable-diffusion
- stable-diffusion-diffusers
- text-to-image
- diffusers
- full
inference: true
widget:
- text: 'Alien planet, strange rock formations, glowing plants, bizarre creatures, surreal atmosphere'
parameters:
negative_prompt: ''''
output:
url: ./assets/image_0_0.png
- text: 'Alien planet, strange rock formations, glowing plants, bizarre creatures, surreal atmosphere'
parameters:
negative_prompt: ''''
output:
url: ./assets/image_1_1.png
- text: 'Alien planet, strange rock formations, glowing plants, bizarre creatures, surreal atmosphere'
parameters:
negative_prompt: ''''
output:
url: ./assets/image_2_2.png
- text: 'Alien marketplace, bizarre creatures, exotic goods, vibrant colors, otherworldly atmosphere'
parameters:
negative_prompt: ''''
output:
url: ./assets/image_3_0.png
- text: 'Alien marketplace, bizarre creatures, exotic goods, vibrant colors, otherworldly atmosphere'
parameters:
negative_prompt: ''''
output:
url: ./assets/image_4_1.png
- text: 'Alien marketplace, bizarre creatures, exotic goods, vibrant colors, otherworldly atmosphere'
parameters:
negative_prompt: ''''
output:
url: ./assets/image_5_2.png
- text: 'Child holding a balloon, happy expression, colorful balloons, sunny day, high detail'
parameters:
negative_prompt: ''''
output:
url: ./assets/image_6_0.png
- text: 'Child holding a balloon, happy expression, colorful balloons, sunny day, high detail'
parameters:
negative_prompt: ''''
output:
url: ./assets/image_7_1.png
- text: 'Child holding a balloon, happy expression, colorful balloons, sunny day, high detail'
parameters:
negative_prompt: ''''
output:
url: ./assets/image_8_2.png
- text: 'a 4-panel comic strip showing an orange cat saying the words ''HELP'' and ''LASAGNA'''
parameters:
negative_prompt: ''''
output:
url: ./assets/image_9_0.png
- text: 'a 4-panel comic strip showing an orange cat saying the words ''HELP'' and ''LASAGNA'''
parameters:
negative_prompt: ''''
output:
url: ./assets/image_10_1.png
- text: 'a 4-panel comic strip showing an orange cat saying the words ''HELP'' and ''LASAGNA'''
parameters:
negative_prompt: ''''
output:
url: ./assets/image_11_2.png
- text: 'a hand is holding a comic book with a cover that reads ''The Adventures of Superhero'''
parameters:
negative_prompt: ''''
output:
url: ./assets/image_12_0.png
- text: 'a hand is holding a comic book with a cover that reads ''The Adventures of Superhero'''
parameters:
negative_prompt: ''''
output:
url: ./assets/image_13_1.png
- text: 'a hand is holding a comic book with a cover that reads ''The Adventures of Superhero'''
parameters:
negative_prompt: ''''
output:
url: ./assets/image_14_2.png
- text: 'Underground cave filled with crystals, glowing lights, reflective surfaces, fantasy environment, high detail'
parameters:
negative_prompt: ''''
output:
url: ./assets/image_15_0.png
- text: 'Underground cave filled with crystals, glowing lights, reflective surfaces, fantasy environment, high detail'
parameters:
negative_prompt: ''''
output:
url: ./assets/image_16_1.png
- text: 'Underground cave filled with crystals, glowing lights, reflective surfaces, fantasy environment, high detail'
parameters:
negative_prompt: ''''
output:
url: ./assets/image_17_2.png
- text: 'Bustling cyberpunk bazaar, vendors, neon signs, advanced tech, crowded, high detail'
parameters:
negative_prompt: ''''
output:
url: ./assets/image_18_0.png
- text: 'Bustling cyberpunk bazaar, vendors, neon signs, advanced tech, crowded, high detail'
parameters:
negative_prompt: ''''
output:
url: ./assets/image_19_1.png
- text: 'Bustling cyberpunk bazaar, vendors, neon signs, advanced tech, crowded, high detail'
parameters:
negative_prompt: ''''
output:
url: ./assets/image_20_2.png
- text: 'Cyberpunk hacker in a dark room, neon glow, multiple screens, intense focus, high detail'
parameters:
negative_prompt: ''''
output:
url: ./assets/image_21_0.png
- text: 'Cyberpunk hacker in a dark room, neon glow, multiple screens, intense focus, high detail'
parameters:
negative_prompt: ''''
output:
url: ./assets/image_22_1.png
- text: 'Cyberpunk hacker in a dark room, neon glow, multiple screens, intense focus, high detail'
parameters:
negative_prompt: ''''
output:
url: ./assets/image_23_2.png
- text: 'a cybernetic anne of green gables with neural implant and bio mech augmentations'
parameters:
negative_prompt: ''''
output:
url: ./assets/image_24_0.png
- text: 'a cybernetic anne of green gables with neural implant and bio mech augmentations'
parameters:
negative_prompt: ''''
output:
url: ./assets/image_25_1.png
- text: 'a cybernetic anne of green gables with neural implant and bio mech augmentations'
parameters:
negative_prompt: ''''
output:
url: ./assets/image_26_2.png
- text: 'Post-apocalyptic cityscape, ruined buildings, overgrown vegetation, dark and gritty, high detail'
parameters:
negative_prompt: ''''
output:
url: ./assets/image_27_0.png
- text: 'Post-apocalyptic cityscape, ruined buildings, overgrown vegetation, dark and gritty, high detail'
parameters:
negative_prompt: ''''
output:
url: ./assets/image_28_1.png
- text: 'Post-apocalyptic cityscape, ruined buildings, overgrown vegetation, dark and gritty, high detail'
parameters:
negative_prompt: ''''
output:
url: ./assets/image_29_2.png
- text: 'Magical castle in a lush forest, glowing windows, fantasy architecture, high resolution, detailed textures'
parameters:
negative_prompt: ''''
output:
url: ./assets/image_30_0.png
- text: 'Magical castle in a lush forest, glowing windows, fantasy architecture, high resolution, detailed textures'
parameters:
negative_prompt: ''''
output:
url: ./assets/image_31_1.png
- text: 'Magical castle in a lush forest, glowing windows, fantasy architecture, high resolution, detailed textures'
parameters:
negative_prompt: ''''
output:
url: ./assets/image_32_2.png
- text: 'Ruins of an ancient temple in an enchanted forest, glowing runes, mystical creatures, high detail'
parameters:
negative_prompt: ''''
output:
url: ./assets/image_33_0.png
- text: 'Ruins of an ancient temple in an enchanted forest, glowing runes, mystical creatures, high detail'
parameters:
negative_prompt: ''''
output:
url: ./assets/image_34_1.png
- text: 'Ruins of an ancient temple in an enchanted forest, glowing runes, mystical creatures, high detail'
parameters:
negative_prompt: ''''
output:
url: ./assets/image_35_2.png
- text: 'Mystical forest, glowing plants, fairies, magical creatures, fantasy art, high detail'
parameters:
negative_prompt: ''''
output:
url: ./assets/image_36_0.png
- text: 'Mystical forest, glowing plants, fairies, magical creatures, fantasy art, high detail'
parameters:
negative_prompt: ''''
output:
url: ./assets/image_37_1.png
- text: 'Mystical forest, glowing plants, fairies, magical creatures, fantasy art, high detail'
parameters:
negative_prompt: ''''
output:
url: ./assets/image_38_2.png
- text: 'Magical garden with glowing flowers, fairies, serene atmosphere, detailed plants, high resolution'
parameters:
negative_prompt: ''''
output:
url: ./assets/image_39_0.png
- text: 'Magical garden with glowing flowers, fairies, serene atmosphere, detailed plants, high resolution'
parameters:
negative_prompt: ''''
output:
url: ./assets/image_40_1.png
- text: 'Magical garden with glowing flowers, fairies, serene atmosphere, detailed plants, high resolution'
parameters:
negative_prompt: ''''
output:
url: ./assets/image_41_2.png
- text: 'Whimsical garden filled with fairies, magical plants, sparkling lights, serene atmosphere, high detail'
parameters:
negative_prompt: ''''
output:
url: ./assets/image_42_0.png
- text: 'Whimsical garden filled with fairies, magical plants, sparkling lights, serene atmosphere, high detail'
parameters:
negative_prompt: ''''
output:
url: ./assets/image_43_1.png
- text: 'Whimsical garden filled with fairies, magical plants, sparkling lights, serene atmosphere, high detail'
parameters:
negative_prompt: ''''
output:
url: ./assets/image_44_2.png
- text: 'Majestic dragon soaring through the sky, detailed scales, dynamic pose, fantasy art, high resolution'
parameters:
negative_prompt: ''''
output:
url: ./assets/image_45_0.png
- text: 'Majestic dragon soaring through the sky, detailed scales, dynamic pose, fantasy art, high resolution'
parameters:
negative_prompt: ''''
output:
url: ./assets/image_46_1.png
- text: 'Majestic dragon soaring through the sky, detailed scales, dynamic pose, fantasy art, high resolution'
parameters:
negative_prompt: ''''
output:
url: ./assets/image_47_2.png
- text: 'Fantasy world, floating islands in the sky, waterfalls, lush vegetation, detailed landscape, high resolution'
parameters:
negative_prompt: ''''
output:
url: ./assets/image_48_0.png
- text: 'Fantasy world, floating islands in the sky, waterfalls, lush vegetation, detailed landscape, high resolution'
parameters:
negative_prompt: ''''
output:
url: ./assets/image_49_1.png
- text: 'Fantasy world, floating islands in the sky, waterfalls, lush vegetation, detailed landscape, high resolution'
parameters:
negative_prompt: ''''
output:
url: ./assets/image_50_2.png
- text: 'Futuristic city skyline at night, neon lights, cyberpunk style, high contrast, sharp focus'
parameters:
negative_prompt: ''''
output:
url: ./assets/image_51_0.png
- text: 'Futuristic city skyline at night, neon lights, cyberpunk style, high contrast, sharp focus'
parameters:
negative_prompt: ''''
output:
url: ./assets/image_52_1.png
- text: 'Futuristic city skyline at night, neon lights, cyberpunk style, high contrast, sharp focus'
parameters:
negative_prompt: ''''
output:
url: ./assets/image_53_2.png
- text: 'Space battle scene, starships fighting, laser beams, explosions, cosmic background'
parameters:
negative_prompt: ''''
output:
url: ./assets/image_54_0.png
- text: 'Space battle scene, starships fighting, laser beams, explosions, cosmic background'
parameters:
negative_prompt: ''''
output:
url: ./assets/image_55_1.png
- text: 'Space battle scene, starships fighting, laser beams, explosions, cosmic background'
parameters:
negative_prompt: ''''
output:
url: ./assets/image_56_2.png
- text: 'Abandoned fairground at night, eerie rides, ghostly figures, fog, dark atmosphere, high detail'
parameters:
negative_prompt: ''''
output:
url: ./assets/image_57_0.png
- text: 'Abandoned fairground at night, eerie rides, ghostly figures, fog, dark atmosphere, high detail'
parameters:
negative_prompt: ''''
output:
url: ./assets/image_58_1.png
- text: 'Abandoned fairground at night, eerie rides, ghostly figures, fog, dark atmosphere, high detail'
parameters:
negative_prompt: ''''
output:
url: ./assets/image_59_2.png
- text: 'Spooky haunted mansion on a hill, dark and eerie, glowing windows, ghostly atmosphere, high detail'
parameters:
negative_prompt: ''''
output:
url: ./assets/image_60_0.png
- text: 'Spooky haunted mansion on a hill, dark and eerie, glowing windows, ghostly atmosphere, high detail'
parameters:
negative_prompt: ''''
output:
url: ./assets/image_61_1.png
- text: 'Spooky haunted mansion on a hill, dark and eerie, glowing windows, ghostly atmosphere, high detail'
parameters:
negative_prompt: ''''
output:
url: ./assets/image_62_2.png
- text: 'a hardcover physics textbook that is called PHYSICS FOR DUMMIES'
parameters:
negative_prompt: ''''
output:
url: ./assets/image_63_0.png
- text: 'a hardcover physics textbook that is called PHYSICS FOR DUMMIES'
parameters:
negative_prompt: ''''
output:
url: ./assets/image_64_1.png
- text: 'a hardcover physics textbook that is called PHYSICS FOR DUMMIES'
parameters:
negative_prompt: ''''
output:
url: ./assets/image_65_2.png
- text: 'Epic medieval battle, knights in armor, dynamic action, detailed landscape, high resolution'
parameters:
negative_prompt: ''''
output:
url: ./assets/image_66_0.png
- text: 'Epic medieval battle, knights in armor, dynamic action, detailed landscape, high resolution'
parameters:
negative_prompt: ''''
output:
url: ./assets/image_67_1.png
- text: 'Epic medieval battle, knights in armor, dynamic action, detailed landscape, high resolution'
parameters:
negative_prompt: ''''
output:
url: ./assets/image_68_2.png
- text: 'Bustling medieval market with merchants, knights, and jesters, vibrant colors, detailed'
parameters:
negative_prompt: ''''
output:
url: ./assets/image_69_0.png
- text: 'Bustling medieval market with merchants, knights, and jesters, vibrant colors, detailed'
parameters:
negative_prompt: ''''
output:
url: ./assets/image_70_1.png
- text: 'Bustling medieval market with merchants, knights, and jesters, vibrant colors, detailed'
parameters:
negative_prompt: ''''
output:
url: ./assets/image_71_2.png
- text: 'Cozy medieval tavern, warm firelight, adventurers drinking, detailed interior, rustic atmosphere'
parameters:
negative_prompt: ''''
output:
url: ./assets/image_72_0.png
- text: 'Cozy medieval tavern, warm firelight, adventurers drinking, detailed interior, rustic atmosphere'
parameters:
negative_prompt: ''''
output:
url: ./assets/image_73_1.png
- text: 'Cozy medieval tavern, warm firelight, adventurers drinking, detailed interior, rustic atmosphere'
parameters:
negative_prompt: ''''
output:
url: ./assets/image_74_2.png
- text: 'Futuristic city skyline at night, neon lights, cyberpunk style, high contrast, sharp focus'
parameters:
negative_prompt: ''''
output:
url: ./assets/image_75_0.png
- text: 'Futuristic city skyline at night, neon lights, cyberpunk style, high contrast, sharp focus'
parameters:
negative_prompt: ''''
output:
url: ./assets/image_76_1.png
- text: 'Futuristic city skyline at night, neon lights, cyberpunk style, high contrast, sharp focus'
parameters:
negative_prompt: ''''
output:
url: ./assets/image_77_2.png
- text: 'Forest with neon-lit trees, glowing plants, bioluminescence, surreal atmosphere, high detail'
parameters:
negative_prompt: ''''
output:
url: ./assets/image_78_0.png
- text: 'Forest with neon-lit trees, glowing plants, bioluminescence, surreal atmosphere, high detail'
parameters:
negative_prompt: ''''
output:
url: ./assets/image_79_1.png
- text: 'Forest with neon-lit trees, glowing plants, bioluminescence, surreal atmosphere, high detail'
parameters:
negative_prompt: ''''
output:
url: ./assets/image_80_2.png
- text: 'Bright neon sign in a busy city street, ''Open 24 Hours'', bold typography, glowing lights'
parameters:
negative_prompt: ''''
output:
url: ./assets/image_81_0.png
- text: 'Bright neon sign in a busy city street, ''Open 24 Hours'', bold typography, glowing lights'
parameters:
negative_prompt: ''''
output:
url: ./assets/image_82_1.png
- text: 'Bright neon sign in a busy city street, ''Open 24 Hours'', bold typography, glowing lights'
parameters:
negative_prompt: ''''
output:
url: ./assets/image_83_2.png
- text: 'Vibrant neon sign, ''Bar'', bold typography, dark background, glowing lights, detailed design'
parameters:
negative_prompt: ''''
output:
url: ./assets/image_84_0.png
- text: 'Vibrant neon sign, ''Bar'', bold typography, dark background, glowing lights, detailed design'
parameters:
negative_prompt: ''''
output:
url: ./assets/image_85_1.png
- text: 'Vibrant neon sign, ''Bar'', bold typography, dark background, glowing lights, detailed design'
parameters:
negative_prompt: ''''
output:
url: ./assets/image_86_2.png
- text: 'Pirate ship on the high seas, stormy weather, detailed sails, dramatic waves, photorealistic'
parameters:
negative_prompt: ''''
output:
url: ./assets/image_87_0.png
- text: 'Pirate ship on the high seas, stormy weather, detailed sails, dramatic waves, photorealistic'
parameters:
negative_prompt: ''''
output:
url: ./assets/image_88_1.png
- text: 'Pirate ship on the high seas, stormy weather, detailed sails, dramatic waves, photorealistic'
parameters:
negative_prompt: ''''
output:
url: ./assets/image_89_2.png
- text: 'Pirate discovering a treasure chest, detailed gold coins, tropical island, dramatic lighting'
parameters:
negative_prompt: ''''
output:
url: ./assets/image_90_0.png
- text: 'Pirate discovering a treasure chest, detailed gold coins, tropical island, dramatic lighting'
parameters:
negative_prompt: ''''
output:
url: ./assets/image_91_1.png
- text: 'Pirate discovering a treasure chest, detailed gold coins, tropical island, dramatic lighting'
parameters:
negative_prompt: ''''
output:
url: ./assets/image_92_2.png
- text: 'a photograph of a woman experiencing a psychedelic trip. trippy, 8k, uhd, fractal'
parameters:
negative_prompt: ''''
output:
url: ./assets/image_93_0.png
- text: 'a photograph of a woman experiencing a psychedelic trip. trippy, 8k, uhd, fractal'
parameters:
negative_prompt: ''''
output:
url: ./assets/image_94_1.png
- text: 'a photograph of a woman experiencing a psychedelic trip. trippy, 8k, uhd, fractal'
parameters:
negative_prompt: ''''
output:
url: ./assets/image_95_2.png
- text: 'Cozy cafe on a rainy day, people sipping coffee, warm lights, reflections on wet pavement, photorealistic'
parameters:
negative_prompt: ''''
output:
url: ./assets/image_96_0.png
- text: 'Cozy cafe on a rainy day, people sipping coffee, warm lights, reflections on wet pavement, photorealistic'
parameters:
negative_prompt: ''''
output:
url: ./assets/image_97_1.png
- text: 'Cozy cafe on a rainy day, people sipping coffee, warm lights, reflections on wet pavement, photorealistic'
parameters:
negative_prompt: ''''
output:
url: ./assets/image_98_2.png
- text: '1980s arcade, neon lights, vintage game machines, kids playing, vibrant colors, nostalgic atmosphere'
parameters:
negative_prompt: ''''
output:
url: ./assets/image_99_0.png
- text: '1980s arcade, neon lights, vintage game machines, kids playing, vibrant colors, nostalgic atmosphere'
parameters:
negative_prompt: ''''
output:
url: ./assets/image_100_1.png
- text: '1980s arcade, neon lights, vintage game machines, kids playing, vibrant colors, nostalgic atmosphere'
parameters:
negative_prompt: ''''
output:
url: ./assets/image_101_2.png
- text: '1980s game room with vintage arcade machines, neon lights, vibrant colors, nostalgic feel'
parameters:
negative_prompt: ''''
output:
url: ./assets/image_102_0.png
- text: '1980s game room with vintage arcade machines, neon lights, vibrant colors, nostalgic feel'
parameters:
negative_prompt: ''''
output:
url: ./assets/image_103_1.png
- text: '1980s game room with vintage arcade machines, neon lights, vibrant colors, nostalgic feel'
parameters:
negative_prompt: ''''
output:
url: ./assets/image_104_2.png
- text: 'Robot blacksmith forging metal, sparks flying, detailed workshop, futuristic and medieval blend'
parameters:
negative_prompt: ''''
output:
url: ./assets/image_105_0.png
- text: 'Robot blacksmith forging metal, sparks flying, detailed workshop, futuristic and medieval blend'
parameters:
negative_prompt: ''''
output:
url: ./assets/image_106_1.png
- text: 'Robot blacksmith forging metal, sparks flying, detailed workshop, futuristic and medieval blend'
parameters:
negative_prompt: ''''
output:
url: ./assets/image_107_2.png
- text: 'Sleek robot performing a dance, futuristic theater, holographic effects, detailed, high resolution'
parameters:
negative_prompt: ''''
output:
url: ./assets/image_108_0.png
- text: 'Sleek robot performing a dance, futuristic theater, holographic effects, detailed, high resolution'
parameters:
negative_prompt: ''''
output:
url: ./assets/image_109_1.png
- text: 'Sleek robot performing a dance, futuristic theater, holographic effects, detailed, high resolution'
parameters:
negative_prompt: ''''
output:
url: ./assets/image_110_2.png
- text: 'High-tech factory where robots are assembled, detailed machinery, futuristic setting, high detail'
parameters:
negative_prompt: ''''
output:
url: ./assets/image_111_0.png
- text: 'High-tech factory where robots are assembled, detailed machinery, futuristic setting, high detail'
parameters:
negative_prompt: ''''
output:
url: ./assets/image_112_1.png
- text: 'High-tech factory where robots are assembled, detailed machinery, futuristic setting, high detail'
parameters:
negative_prompt: ''''
output:
url: ./assets/image_113_2.png
- text: 'Garden tended by robots, mechanical plants, colorful flowers, futuristic setting, high detail'
parameters:
negative_prompt: ''''
output:
url: ./assets/image_114_0.png
- text: 'Garden tended by robots, mechanical plants, colorful flowers, futuristic setting, high detail'
parameters:
negative_prompt: ''''
output:
url: ./assets/image_115_1.png
- text: 'Garden tended by robots, mechanical plants, colorful flowers, futuristic setting, high detail'
parameters:
negative_prompt: ''''
output:
url: ./assets/image_116_2.png
- text: 'Cute robotic pet, futuristic home, sleek design, detailed features, friendly and animated'
parameters:
negative_prompt: ''''
output:
url: ./assets/image_117_0.png
- text: 'Cute robotic pet, futuristic home, sleek design, detailed features, friendly and animated'
parameters:
negative_prompt: ''''
output:
url: ./assets/image_118_1.png
- text: 'Cute robotic pet, futuristic home, sleek design, detailed features, friendly and animated'
parameters:
negative_prompt: ''''
output:
url: ./assets/image_119_2.png
- text: 'cctv trail camera night time security picture of a wendigo in the woods'
parameters:
negative_prompt: ''''
output:
url: ./assets/image_120_0.png
- text: 'cctv trail camera night time security picture of a wendigo in the woods'
parameters:
negative_prompt: ''''
output:
url: ./assets/image_121_1.png
- text: 'cctv trail camera night time security picture of a wendigo in the woods'
parameters:
negative_prompt: ''''
output:
url: ./assets/image_122_2.png
- text: 'Astronaut exploring an alien planet, detailed landscape, futuristic suit, cosmic background'
parameters:
negative_prompt: ''''
output:
url: ./assets/image_123_0.png
- text: 'Astronaut exploring an alien planet, detailed landscape, futuristic suit, cosmic background'
parameters:
negative_prompt: ''''
output:
url: ./assets/image_124_1.png
- text: 'Astronaut exploring an alien planet, detailed landscape, futuristic suit, cosmic background'
parameters:
negative_prompt: ''''
output:
url: ./assets/image_125_2.png
- text: 'Futuristic space station orbiting a distant exoplanet, sleek design, detailed structures, cosmic backdrop'
parameters:
negative_prompt: ''''
output:
url: ./assets/image_126_0.png
- text: 'Futuristic space station orbiting a distant exoplanet, sleek design, detailed structures, cosmic backdrop'
parameters:
negative_prompt: ''''
output:
url: ./assets/image_127_1.png
- text: 'Futuristic space station orbiting a distant exoplanet, sleek design, detailed structures, cosmic backdrop'
parameters:
negative_prompt: ''''
output:
url: ./assets/image_128_2.png
- text: 'a person holding a sign that reads ''SOON'''
parameters:
negative_prompt: ''''
output:
url: ./assets/image_129_0.png
- text: 'a person holding a sign that reads ''SOON'''
parameters:
negative_prompt: ''''
output:
url: ./assets/image_130_1.png
- text: 'a person holding a sign that reads ''SOON'''
parameters:
negative_prompt: ''''
output:
url: ./assets/image_131_2.png
- text: 'Steampunk airship in the sky, intricate design, Victorian aesthetics, dynamic scene, high detail'
parameters:
negative_prompt: ''''
output:
url: ./assets/image_132_0.png
- text: 'Steampunk airship in the sky, intricate design, Victorian aesthetics, dynamic scene, high detail'
parameters:
negative_prompt: ''''
output:
url: ./assets/image_133_1.png
- text: 'Steampunk airship in the sky, intricate design, Victorian aesthetics, dynamic scene, high detail'
parameters:
negative_prompt: ''''
output:
url: ./assets/image_134_2.png
- text: 'Steampunk inventor in a workshop, intricate gadgets, Victorian attire, mechanical arm, goggles'
parameters:
negative_prompt: ''''
output:
url: ./assets/image_135_0.png
- text: 'Steampunk inventor in a workshop, intricate gadgets, Victorian attire, mechanical arm, goggles'
parameters:
negative_prompt: ''''
output:
url: ./assets/image_136_1.png
- text: 'Steampunk inventor in a workshop, intricate gadgets, Victorian attire, mechanical arm, goggles'
parameters:
negative_prompt: ''''
output:
url: ./assets/image_137_2.png
- text: 'Stormy ocean with towering waves, dramatic skies, detailed water, intense atmosphere, high resolution'
parameters:
negative_prompt: ''''
output:
url: ./assets/image_138_0.png
- text: 'Stormy ocean with towering waves, dramatic skies, detailed water, intense atmosphere, high resolution'
parameters:
negative_prompt: ''''
output:
url: ./assets/image_139_1.png
- text: 'Stormy ocean with towering waves, dramatic skies, detailed water, intense atmosphere, high resolution'
parameters:
negative_prompt: ''''
output:
url: ./assets/image_140_2.png
- text: 'Dramatic stormy sea, lighthouse in the distance, lightning striking, dark clouds, high detail'
parameters:
negative_prompt: ''''
output:
url: ./assets/image_141_0.png
- text: 'Dramatic stormy sea, lighthouse in the distance, lightning striking, dark clouds, high detail'
parameters:
negative_prompt: ''''
output:
url: ./assets/image_142_1.png
- text: 'Dramatic stormy sea, lighthouse in the distance, lightning striking, dark clouds, high detail'
parameters:
negative_prompt: ''''
output:
url: ./assets/image_143_2.png
- text: 'Graffiti artist creating a mural, vibrant colors, urban setting, dynamic action, high resolution'
parameters:
negative_prompt: ''''
output:
url: ./assets/image_144_0.png
- text: 'Graffiti artist creating a mural, vibrant colors, urban setting, dynamic action, high resolution'
parameters:
negative_prompt: ''''
output:
url: ./assets/image_145_1.png
- text: 'Graffiti artist creating a mural, vibrant colors, urban setting, dynamic action, high resolution'
parameters:
negative_prompt: ''''
output:
url: ./assets/image_146_2.png
- text: 'Urban alleyway filled with vibrant graffiti art, tags and murals, realistic textures'
parameters:
negative_prompt: ''''
output:
url: ./assets/image_147_0.png
- text: 'Urban alleyway filled with vibrant graffiti art, tags and murals, realistic textures'
parameters:
negative_prompt: ''''
output:
url: ./assets/image_148_1.png
- text: 'Urban alleyway filled with vibrant graffiti art, tags and murals, realistic textures'
parameters:
negative_prompt: ''''
output:
url: ./assets/image_149_2.png
- text: 'Urban street sign, ''Main Street'', bold typography, realistic textures, weathered look'
parameters:
negative_prompt: ''''
output:
url: ./assets/image_150_0.png
- text: 'Urban street sign, ''Main Street'', bold typography, realistic textures, weathered look'
parameters:
negative_prompt: ''''
output:
url: ./assets/image_151_1.png
- text: 'Urban street sign, ''Main Street'', bold typography, realistic textures, weathered look'
parameters:
negative_prompt: ''''
output:
url: ./assets/image_152_2.png
- text: 'Classic car show with vintage vehicles, vibrant colors, nostalgic atmosphere, high detail'
parameters:
negative_prompt: ''''
output:
url: ./assets/image_153_0.png
- text: 'Classic car show with vintage vehicles, vibrant colors, nostalgic atmosphere, high detail'
parameters:
negative_prompt: ''''
output:
url: ./assets/image_154_1.png
- text: 'Classic car show with vintage vehicles, vibrant colors, nostalgic atmosphere, high detail'
parameters:
negative_prompt: ''''
output:
url: ./assets/image_155_2.png
- text: 'Retro diner sign, ''Joe''s Diner'', classic 1950s design, neon lights, weathered look'
parameters:
negative_prompt: ''''
output:
url: ./assets/image_156_0.png
- text: 'Retro diner sign, ''Joe''s Diner'', classic 1950s design, neon lights, weathered look'
parameters:
negative_prompt: ''''
output:
url: ./assets/image_157_1.png
- text: 'Retro diner sign, ''Joe''s Diner'', classic 1950s design, neon lights, weathered look'
parameters:
negative_prompt: ''''
output:
url: ./assets/image_158_2.png
- text: 'Vintage store sign with elaborate typography, ''Antique Shop'', hand-painted, weathered look'
parameters:
negative_prompt: ''''
output:
url: ./assets/image_159_0.png
- text: 'Vintage store sign with elaborate typography, ''Antique Shop'', hand-painted, weathered look'
parameters:
negative_prompt: ''''
output:
url: ./assets/image_160_1.png
- text: 'Vintage store sign with elaborate typography, ''Antique Shop'', hand-painted, weathered look'
parameters:
negative_prompt: ''''
output:
url: ./assets/image_161_2.png
- text: 'a child wearing a pixar style wedding dress, in a play castle'
parameters:
negative_prompt: ''''
output:
url: ./assets/image_162_0.png
- text: 'a child wearing a pixar style wedding dress, in a play castle'
parameters:
negative_prompt: ''''
output:
url: ./assets/image_163_1.png
- text: 'a child wearing a pixar style wedding dress, in a play castle'
parameters:
negative_prompt: ''''
output:
url: ./assets/image_164_2.png
- text: 'a cartoon bear in red shorts playing basketball with a sponge'
parameters:
negative_prompt: ''''
output:
url: ./assets/image_165_0.png
- text: 'a cartoon bear in red shorts playing basketball with a sponge'
parameters:
negative_prompt: ''''
output:
url: ./assets/image_166_1.png
- text: 'a cartoon bear in red shorts playing basketball with a sponge'
parameters:
negative_prompt: ''''
output:
url: ./assets/image_167_2.png
- text: 'a superhero with a cape and a mask, fighting a dragon'
parameters:
negative_prompt: ''''
output:
url: ./assets/image_168_0.png
- text: 'a superhero with a cape and a mask, fighting a dragon'
parameters:
negative_prompt: ''''
output:
url: ./assets/image_169_1.png
- text: 'a superhero with a cape and a mask, fighting a dragon'
parameters:
negative_prompt: ''''
output:
url: ./assets/image_170_2.png
- text: 'a dramatic scene with intense lighting showcasing a man and a woman in a tense conversation'
parameters:
negative_prompt: ''''
output:
url: ./assets/image_171_0.png
- text: 'a dramatic scene with intense lighting showcasing a man and a woman in a tense conversation'
parameters:
negative_prompt: ''''
output:
url: ./assets/image_172_1.png
- text: 'a dramatic scene with intense lighting showcasing a man and a woman in a tense conversation'
parameters:
negative_prompt: ''''
output:
url: ./assets/image_173_2.png
- text: 'a group of people in a house, with a camera crew filming them'
parameters:
negative_prompt: ''''
output:
url: ./assets/image_174_0.png
- text: 'a group of people in a house, with a camera crew filming them'
parameters:
negative_prompt: ''''
output:
url: ./assets/image_175_1.png
- text: 'a group of people in a house, with a camera crew filming them'
parameters:
negative_prompt: ''''
output:
url: ./assets/image_176_2.png
- text: 'a person in a lab coat holding a microphone stands in a forest, talking about the ecosystem'
parameters:
negative_prompt: ''''
output:
url: ./assets/image_177_0.png
- text: 'a person in a lab coat holding a microphone stands in a forest, talking about the ecosystem'
parameters:
negative_prompt: ''''
output:
url: ./assets/image_178_1.png
- text: 'a person in a lab coat holding a microphone stands in a forest, talking about the ecosystem'
parameters:
negative_prompt: ''''
output:
url: ./assets/image_179_2.png
- text: 'a news anchor sitting at a desk, with a screen behind them showing a map of the world'
parameters:
negative_prompt: ''''
output:
url: ./assets/image_180_0.png
- text: 'a news anchor sitting at a desk, with a screen behind them showing a map of the world'
parameters:
negative_prompt: ''''
output:
url: ./assets/image_181_1.png
- text: 'a news anchor sitting at a desk, with a screen behind them showing a map of the world'
parameters:
negative_prompt: ''''
output:
url: ./assets/image_182_2.png
- text: 'a soccer player kicking a ball into a goal, with a crowd cheering'
parameters:
negative_prompt: ''''
output:
url: ./assets/image_183_0.png
- text: 'a soccer player kicking a ball into a goal, with a crowd cheering'
parameters:
negative_prompt: ''''
output:
url: ./assets/image_184_1.png
- text: 'a soccer player kicking a ball into a goal, with a crowd cheering'
parameters:
negative_prompt: ''''
output:
url: ./assets/image_185_2.png
- text: 'a man is holding a sign that says SOON'
parameters:
negative_prompt: ''''
output:
url: ./assets/image_186_0.png
- text: 'a man is holding a sign that says SOON'
parameters:
negative_prompt: ''''
output:
url: ./assets/image_187_1.png
- text: 'a man is holding a sign that says SOON'
parameters:
negative_prompt: ''''
output:
url: ./assets/image_188_2.png
- text: 'a cute anime character named toast holding a sign that says SOON, sitting next to a red square on her left side, and a transparent sphere on her right side'
parameters:
negative_prompt: ''''
output:
url: ./assets/image_189_0.png
- text: 'a cute anime character named toast holding a sign that says SOON, sitting next to a red square on her left side, and a transparent sphere on her right side'
parameters:
negative_prompt: ''''
output:
url: ./assets/image_190_1.png
- text: 'a cute anime character named toast holding a sign that says SOON, sitting next to a red square on her left side, and a transparent sphere on her right side'
parameters:
negative_prompt: ''''
output:
url: ./assets/image_191_2.png
---
# terminus-xl-velocity-training
This is a full rank finetune derived from [ptx0/terminus-xl-velocity-v2](https://huggingface.co/ptx0/terminus-xl-velocity-v2).
The main validation prompt used during training was:
```
a cute anime character named toast holding a sign that says SOON, sitting next to a red square on her left side, and a transparent sphere on her right side
```
## Validation settings
- CFG: `7.5`
- CFG Rescale: `0.7`
- Steps: `30`
- Sampler: `euler`
- Seed: `42`
- Resolutions: `1024x1024,1152x960,896x1152`
Note: The validation settings are not necessarily the same as the [training settings](#training-settings).
You can find some example images in the following gallery:
<Gallery />
The text encoder **was not** trained.
You may reuse the base model text encoder for inference.
## Training settings
- Training epochs: 13
- Training steps: 23000
- Learning rate: 4e-07
- Effective batch size: 512
- Micro-batch size: 32
- Gradient accumulation steps: 2
- Number of GPUs: 8
- Prediction type: v_prediction
- Rescaled betas zero SNR: True
- Optimizer: AdamW, stochastic bf16
- Precision: Pure BF16
- Xformers: Enabled
## Datasets
### photo-concept-bucket
- Repeats: 0
- Total number of images: ~557568
- Total number of aspect buckets: 5
- Resolution: 1.0 megapixels
- Cropped: True
- Crop style: random
- Crop aspect: random
## Inference
```python
import torch
from diffusers import DiffusionPipeline
model_id = "terminus-xl-velocity-training"
prompt = "a cute anime character named toast holding a sign that says SOON, sitting next to a red square on her left side, and a transparent sphere on her right side"
negative_prompt = "malformed, disgusting, overexposed, washed-out"
pipeline = DiffusionPipeline.from_pretrained(model_id)
pipeline.to('cuda' if torch.cuda.is_available() else 'mps' if torch.backends.mps.is_available() else 'cpu')
image = pipeline(
prompt=prompt,
negative_prompt='',
num_inference_steps=30,
generator=torch.Generator(device='cuda' if torch.cuda.is_available() else 'mps' if torch.backends.mps.is_available() else 'cpu').manual_seed(1641421826),
width=1152,
height=768,
guidance_scale=7.5,
guidance_rescale=0.7,
).images[0]
image.save("output.png", format="PNG")
```
|
RichardErkhov/cognitivecomputations_-_dolphin-2.9.2-qwen2-72b-gguf | RichardErkhov | "2024-06-29T08:52:02Z" | 99,810 | 0 | null | [
"gguf",
"region:us"
] | null | "2024-06-28T15:16:42Z" | Entry not found |
timm/lcnet_050.ra2_in1k | timm | "2023-04-27T22:48:56Z" | 99,384 | 0 | timm | [
"timm",
"pytorch",
"safetensors",
"image-classification",
"dataset:imagenet-1k",
"arxiv:2110.00476",
"arxiv:2109.15099",
"license:apache-2.0",
"region:us"
] | image-classification | "2022-12-16T05:37:27Z" | ---
tags:
- image-classification
- timm
library_name: timm
license: apache-2.0
datasets:
- imagenet-1k
---
# Model card for lcnet_050.ra2_in1k
A LCNet image classification model. Trained on ImageNet-1k in `timm` using recipe template described below.
Recipe details:
* RandAugment `RA2` recipe. Inspired by and evolved from EfficientNet RandAugment recipes. Published as `B` recipe in [ResNet Strikes Back](https://arxiv.org/abs/2110.00476).
* RMSProp (TF 1.0 behaviour) optimizer, EMA weight averaging
* Step (exponential decay w/ staircase) LR schedule with warmup
## Model Details
- **Model Type:** Image classification / feature backbone
- **Model Stats:**
- Params (M): 1.9
- GMACs: 0.0
- Activations (M): 1.3
- Image size: 224 x 224
- **Papers:**
- PP-LCNet: A Lightweight CPU Convolutional Neural Network: https://arxiv.org/abs/2109.15099
- ResNet strikes back: An improved training procedure in timm: https://arxiv.org/abs/2110.00476
- **Dataset:** ImageNet-1k
- **Original:** https://github.com/huggingface/pytorch-image-models
## Model Usage
### Image Classification
```python
from urllib.request import urlopen
from PIL import Image
import timm
img = Image.open(urlopen(
'https://huggingface.co/datasets/huggingface/documentation-images/resolve/main/beignets-task-guide.png'
))
model = timm.create_model('lcnet_050.ra2_in1k', pretrained=True)
model = model.eval()
# get model specific transforms (normalization, resize)
data_config = timm.data.resolve_model_data_config(model)
transforms = timm.data.create_transform(**data_config, is_training=False)
output = model(transforms(img).unsqueeze(0)) # unsqueeze single image into batch of 1
top5_probabilities, top5_class_indices = torch.topk(output.softmax(dim=1) * 100, k=5)
```
### Feature Map Extraction
```python
from urllib.request import urlopen
from PIL import Image
import timm
img = Image.open(urlopen(
'https://huggingface.co/datasets/huggingface/documentation-images/resolve/main/beignets-task-guide.png'
))
model = timm.create_model(
'lcnet_050.ra2_in1k',
pretrained=True,
features_only=True,
)
model = model.eval()
# get model specific transforms (normalization, resize)
data_config = timm.data.resolve_model_data_config(model)
transforms = timm.data.create_transform(**data_config, is_training=False)
output = model(transforms(img).unsqueeze(0)) # unsqueeze single image into batch of 1
for o in output:
# print shape of each feature map in output
# e.g.:
# torch.Size([1, 16, 112, 112])
# torch.Size([1, 32, 56, 56])
# torch.Size([1, 64, 28, 28])
# torch.Size([1, 128, 14, 14])
# torch.Size([1, 256, 7, 7])
print(o.shape)
```
### Image Embeddings
```python
from urllib.request import urlopen
from PIL import Image
import timm
img = Image.open(urlopen(
'https://huggingface.co/datasets/huggingface/documentation-images/resolve/main/beignets-task-guide.png'
))
model = timm.create_model(
'lcnet_050.ra2_in1k',
pretrained=True,
num_classes=0, # remove classifier nn.Linear
)
model = model.eval()
# get model specific transforms (normalization, resize)
data_config = timm.data.resolve_model_data_config(model)
transforms = timm.data.create_transform(**data_config, is_training=False)
output = model(transforms(img).unsqueeze(0)) # output is (batch_size, num_features) shaped tensor
# or equivalently (without needing to set num_classes=0)
output = model.forward_features(transforms(img).unsqueeze(0))
# output is unpooled, a (1, 256, 7, 7) shaped tensor
output = model.forward_head(output, pre_logits=True)
# output is a (1, num_features) shaped tensor
```
## Model Comparison
Explore the dataset and runtime metrics of this model in timm [model results](https://github.com/huggingface/pytorch-image-models/tree/main/results).
## Citation
```bibtex
@article{cui2021pp,
title={PP-LCNet: A lightweight CPU convolutional neural network},
author={Cui, Cheng and Gao, Tingquan and Wei, Shengyu and Du, Yuning and Guo, Ruoyu and Dong, Shuilong and Lu, Bin and Zhou, Ying and Lv, Xueying and Liu, Qiwen and others},
journal={arXiv preprint arXiv:2109.15099},
year={2021}
}
```
```bibtex
@misc{rw2019timm,
author = {Ross Wightman},
title = {PyTorch Image Models},
year = {2019},
publisher = {GitHub},
journal = {GitHub repository},
doi = {10.5281/zenodo.4414861},
howpublished = {\url{https://github.com/huggingface/pytorch-image-models}}
}
```
```bibtex
@inproceedings{wightman2021resnet,
title={ResNet strikes back: An improved training procedure in timm},
author={Wightman, Ross and Touvron, Hugo and Jegou, Herve},
booktitle={NeurIPS 2021 Workshop on ImageNet: Past, Present, and Future}
}
```
|
sgugger/rwkv-430M-pile | sgugger | "2023-05-03T14:06:12Z" | 99,302 | 2 | transformers | [
"transformers",
"pytorch",
"rwkv",
"endpoints_compatible",
"region:us"
] | null | "2023-04-27T21:09:05Z" | Entry not found |
anton-l/wav2vec2-random-tiny-classifier | anton-l | "2021-08-31T14:27:40Z" | 99,247 | 2 | transformers | [
"transformers",
"pytorch",
"wav2vec2",
"audio-classification",
"endpoints_compatible",
"region:us"
] | audio-classification | "2022-03-02T23:29:05Z" | Entry not found |
mradermacher/Swallow-70b-instruct-v0.1-i1-GGUF | mradermacher | "2024-07-01T03:42:28Z" | 99,134 | 0 | transformers | [
"transformers",
"gguf",
"en",
"ja",
"base_model:tokyotech-llm/Swallow-70b-instruct-v0.1",
"license:llama2",
"endpoints_compatible",
"region:us"
] | null | "2024-06-30T15:44:56Z" | ---
base_model: tokyotech-llm/Swallow-70b-instruct-v0.1
language:
- en
- ja
library_name: transformers
license: llama2
model_type: llama
quantized_by: mradermacher
---
## About
<!-- ### quantize_version: 2 -->
<!-- ### output_tensor_quantised: 1 -->
<!-- ### convert_type: hf -->
<!-- ### vocab_type: -->
<!-- ### tags: nicoboss -->
weighted/imatrix quants of https://huggingface.co/tokyotech-llm/Swallow-70b-instruct-v0.1
<!-- provided-files -->
static quants are available at https://huggingface.co/mradermacher/Swallow-70b-instruct-v0.1-GGUF
## Usage
If you are unsure how to use GGUF files, refer to one of [TheBloke's
READMEs](https://huggingface.co/TheBloke/KafkaLM-70B-German-V0.1-GGUF) for
more details, including on how to concatenate multi-part files.
## Provided Quants
(sorted by size, not necessarily quality. IQ-quants are often preferable over similar sized non-IQ quants)
| Link | Type | Size/GB | Notes |
|:-----|:-----|--------:|:------|
| [GGUF](https://huggingface.co/mradermacher/Swallow-70b-instruct-v0.1-i1-GGUF/resolve/main/Swallow-70b-instruct-v0.1.i1-IQ1_S.gguf) | i1-IQ1_S | 14.7 | for the desperate |
| [GGUF](https://huggingface.co/mradermacher/Swallow-70b-instruct-v0.1-i1-GGUF/resolve/main/Swallow-70b-instruct-v0.1.i1-IQ1_M.gguf) | i1-IQ1_M | 16.1 | mostly desperate |
| [GGUF](https://huggingface.co/mradermacher/Swallow-70b-instruct-v0.1-i1-GGUF/resolve/main/Swallow-70b-instruct-v0.1.i1-IQ2_XXS.gguf) | i1-IQ2_XXS | 18.5 | |
| [GGUF](https://huggingface.co/mradermacher/Swallow-70b-instruct-v0.1-i1-GGUF/resolve/main/Swallow-70b-instruct-v0.1.i1-IQ2_XS.gguf) | i1-IQ2_XS | 20.5 | |
| [GGUF](https://huggingface.co/mradermacher/Swallow-70b-instruct-v0.1-i1-GGUF/resolve/main/Swallow-70b-instruct-v0.1.i1-IQ2_S.gguf) | i1-IQ2_S | 21.6 | |
| [GGUF](https://huggingface.co/mradermacher/Swallow-70b-instruct-v0.1-i1-GGUF/resolve/main/Swallow-70b-instruct-v0.1.i1-IQ2_M.gguf) | i1-IQ2_M | 23.4 | |
| [GGUF](https://huggingface.co/mradermacher/Swallow-70b-instruct-v0.1-i1-GGUF/resolve/main/Swallow-70b-instruct-v0.1.i1-Q2_K.gguf) | i1-Q2_K | 25.7 | IQ3_XXS probably better |
| [GGUF](https://huggingface.co/mradermacher/Swallow-70b-instruct-v0.1-i1-GGUF/resolve/main/Swallow-70b-instruct-v0.1.i1-IQ3_XXS.gguf) | i1-IQ3_XXS | 26.8 | lower quality |
| [GGUF](https://huggingface.co/mradermacher/Swallow-70b-instruct-v0.1-i1-GGUF/resolve/main/Swallow-70b-instruct-v0.1.i1-IQ3_XS.gguf) | i1-IQ3_XS | 28.5 | |
| [GGUF](https://huggingface.co/mradermacher/Swallow-70b-instruct-v0.1-i1-GGUF/resolve/main/Swallow-70b-instruct-v0.1.i1-IQ3_S.gguf) | i1-IQ3_S | 30.1 | beats Q3_K* |
| [GGUF](https://huggingface.co/mradermacher/Swallow-70b-instruct-v0.1-i1-GGUF/resolve/main/Swallow-70b-instruct-v0.1.i1-Q3_K_S.gguf) | i1-Q3_K_S | 30.1 | IQ3_XS probably better |
| [GGUF](https://huggingface.co/mradermacher/Swallow-70b-instruct-v0.1-i1-GGUF/resolve/main/Swallow-70b-instruct-v0.1.i1-IQ3_M.gguf) | i1-IQ3_M | 31.2 | |
| [GGUF](https://huggingface.co/mradermacher/Swallow-70b-instruct-v0.1-i1-GGUF/resolve/main/Swallow-70b-instruct-v0.1.i1-Q3_K_M.gguf) | i1-Q3_K_M | 33.5 | IQ3_S probably better |
| [GGUF](https://huggingface.co/mradermacher/Swallow-70b-instruct-v0.1-i1-GGUF/resolve/main/Swallow-70b-instruct-v0.1.i1-Q3_K_L.gguf) | i1-Q3_K_L | 36.4 | IQ3_M probably better |
| [GGUF](https://huggingface.co/mradermacher/Swallow-70b-instruct-v0.1-i1-GGUF/resolve/main/Swallow-70b-instruct-v0.1.i1-IQ4_XS.gguf) | i1-IQ4_XS | 37.1 | |
| [GGUF](https://huggingface.co/mradermacher/Swallow-70b-instruct-v0.1-i1-GGUF/resolve/main/Swallow-70b-instruct-v0.1.i1-Q4_0.gguf) | i1-Q4_0 | 39.2 | fast, low quality |
| [GGUF](https://huggingface.co/mradermacher/Swallow-70b-instruct-v0.1-i1-GGUF/resolve/main/Swallow-70b-instruct-v0.1.i1-Q4_K_S.gguf) | i1-Q4_K_S | 39.5 | optimal size/speed/quality |
| [GGUF](https://huggingface.co/mradermacher/Swallow-70b-instruct-v0.1-i1-GGUF/resolve/main/Swallow-70b-instruct-v0.1.i1-Q4_K_M.gguf) | i1-Q4_K_M | 41.6 | fast, recommended |
| [GGUF](https://huggingface.co/mradermacher/Swallow-70b-instruct-v0.1-i1-GGUF/resolve/main/Swallow-70b-instruct-v0.1.i1-Q5_K_S.gguf) | i1-Q5_K_S | 47.7 | |
| [GGUF](https://huggingface.co/mradermacher/Swallow-70b-instruct-v0.1-i1-GGUF/resolve/main/Swallow-70b-instruct-v0.1.i1-Q5_K_M.gguf) | i1-Q5_K_M | 49.0 | |
| [PART 1](https://huggingface.co/mradermacher/Swallow-70b-instruct-v0.1-i1-GGUF/resolve/main/Swallow-70b-instruct-v0.1.i1-Q6_K.gguf.part1of2) [PART 2](https://huggingface.co/mradermacher/Swallow-70b-instruct-v0.1-i1-GGUF/resolve/main/Swallow-70b-instruct-v0.1.i1-Q6_K.gguf.part2of2) | i1-Q6_K | 56.8 | practically like static Q6_K |
Here is a handy graph by ikawrakow comparing some lower-quality quant
types (lower is better):

And here are Artefact2's thoughts on the matter:
https://gist.github.com/Artefact2/b5f810600771265fc1e39442288e8ec9
## FAQ / Model Request
See https://huggingface.co/mradermacher/model_requests for some answers to
questions you might have and/or if you want some other model quantized.
## Thanks
I thank my company, [nethype GmbH](https://www.nethype.de/), for letting
me use its servers and providing upgrades to my workstation to enable
this work in my free time. Additional thanks to [@nicoboss](https://huggingface.co/nicoboss) for giving me access to his hardware for calculating the imatrix for these quants.
<!-- end -->
|
microsoft/BiomedNLP-BiomedBERT-base-uncased-abstract | microsoft | "2023-11-06T18:04:15Z" | 99,068 | 57 | transformers | [
"transformers",
"pytorch",
"jax",
"bert",
"fill-mask",
"exbert",
"en",
"arxiv:2007.15779",
"license:mit",
"autotrain_compatible",
"endpoints_compatible",
"region:us"
] | fill-mask | "2022-03-02T23:29:05Z" | ---
language: en
tags:
- exbert
license: mit
widget:
- text: "[MASK] is a tyrosine kinase inhibitor."
---
## MSR BiomedBERT (abstracts only)
<div style="border: 2px solid orange; border-radius:10px; padding:0px 10px; width: fit-content;">
* This model was previously named **"PubMedBERT (abstracts)"**.
* You can either adopt the new model name "microsoft/BiomedNLP-BiomedBERT-base-uncased-abstract" or update your `transformers` library to version 4.22+ if you need to refer to the old name.
</div>
Pretraining large neural language models, such as BERT, has led to impressive gains on many natural language processing (NLP) tasks. However, most pretraining efforts focus on general domain corpora, such as newswire and Web. A prevailing assumption is that even domain-specific pretraining can benefit by starting from general-domain language models. [Recent work](https://arxiv.org/abs/2007.15779) shows that for domains with abundant unlabeled text, such as biomedicine, pretraining language models from scratch results in substantial gains over continual pretraining of general-domain language models.
This BiomedBERT is pretrained from scratch using _abstracts_ from [PubMed](https://pubmed.ncbi.nlm.nih.gov/). This model achieves state-of-the-art performance on several biomedical NLP tasks, as shown on the [Biomedical Language Understanding and Reasoning Benchmark](https://aka.ms/BLURB).
## Citation
If you find BiomedBERT useful in your research, please cite the following paper:
```latex
@misc{pubmedbert,
author = {Yu Gu and Robert Tinn and Hao Cheng and Michael Lucas and Naoto Usuyama and Xiaodong Liu and Tristan Naumann and Jianfeng Gao and Hoifung Poon},
title = {Domain-Specific Language Model Pretraining for Biomedical Natural Language Processing},
year = {2020},
eprint = {arXiv:2007.15779},
}
```
<a href="https://huggingface.co/exbert/?model=microsoft/BiomedNLP-PubMedBERT-base-uncased-abstract&modelKind=bidirectional&sentence=Gefitinib%20is%20an%20EGFR%20tyrosine%20kinase%20inhibitor,%20which%20is%20often%20used%20for%20breast%20cancer%20and%20NSCLC%20treatment.&layer=10&heads=..0,1,2,3,4,5,6,7,8,9,10,11&threshold=0.7&tokenInd=17&tokenSide=right&maskInds=..&hideClsSep=true">
<img width="300px" src="https://cdn-media.huggingface.co/exbert/button.png">
</a>
|
rasa/LaBSE | rasa | "2021-05-20T04:01:27Z" | 98,619 | 20 | transformers | [
"transformers",
"pytorch",
"tf",
"jax",
"bert",
"feature-extraction",
"endpoints_compatible",
"text-embeddings-inference",
"region:us"
] | feature-extraction | "2022-03-02T23:29:05Z" | Entry not found |
Systran/faster-whisper-tiny | Systran | "2023-11-23T10:42:55Z" | 98,586 | 2 | ctranslate2 | [
"ctranslate2",
"audio",
"automatic-speech-recognition",
"en",
"zh",
"de",
"es",
"ru",
"ko",
"fr",
"ja",
"pt",
"tr",
"pl",
"ca",
"nl",
"ar",
"sv",
"it",
"id",
"hi",
"fi",
"vi",
"he",
"uk",
"el",
"ms",
"cs",
"ro",
"da",
"hu",
"ta",
"no",
"th",
"ur",
"hr",
"bg",
"lt",
"la",
"mi",
"ml",
"cy",
"sk",
"te",
"fa",
"lv",
"bn",
"sr",
"az",
"sl",
"kn",
"et",
"mk",
"br",
"eu",
"is",
"hy",
"ne",
"mn",
"bs",
"kk",
"sq",
"sw",
"gl",
"mr",
"pa",
"si",
"km",
"sn",
"yo",
"so",
"af",
"oc",
"ka",
"be",
"tg",
"sd",
"gu",
"am",
"yi",
"lo",
"uz",
"fo",
"ht",
"ps",
"tk",
"nn",
"mt",
"sa",
"lb",
"my",
"bo",
"tl",
"mg",
"as",
"tt",
"haw",
"ln",
"ha",
"ba",
"jw",
"su",
"license:mit",
"region:us"
] | automatic-speech-recognition | "2023-11-23T09:53:30Z" | ---
language:
- en
- zh
- de
- es
- ru
- ko
- fr
- ja
- pt
- tr
- pl
- ca
- nl
- ar
- sv
- it
- id
- hi
- fi
- vi
- he
- uk
- el
- ms
- cs
- ro
- da
- hu
- ta
- 'no'
- th
- ur
- hr
- bg
- lt
- la
- mi
- ml
- cy
- sk
- te
- fa
- lv
- bn
- sr
- az
- sl
- kn
- et
- mk
- br
- eu
- is
- hy
- ne
- mn
- bs
- kk
- sq
- sw
- gl
- mr
- pa
- si
- km
- sn
- yo
- so
- af
- oc
- ka
- be
- tg
- sd
- gu
- am
- yi
- lo
- uz
- fo
- ht
- ps
- tk
- nn
- mt
- sa
- lb
- my
- bo
- tl
- mg
- as
- tt
- haw
- ln
- ha
- ba
- jw
- su
tags:
- audio
- automatic-speech-recognition
license: mit
library_name: ctranslate2
---
# Whisper tiny model for CTranslate2
This repository contains the conversion of [openai/whisper-tiny](https://huggingface.co/openai/whisper-tiny) to the [CTranslate2](https://github.com/OpenNMT/CTranslate2) model format.
This model can be used in CTranslate2 or projects based on CTranslate2 such as [faster-whisper](https://github.com/systran/faster-whisper).
## Example
```python
from faster_whisper import WhisperModel
model = WhisperModel("tiny")
segments, info = model.transcribe("audio.mp3")
for segment in segments:
print("[%.2fs -> %.2fs] %s" % (segment.start, segment.end, segment.text))
```
## Conversion details
The original model was converted with the following command:
```
ct2-transformers-converter --model openai/whisper-tiny --output_dir faster-whisper-tiny \
--copy_files tokenizer.json --quantization float16
```
Note that the model weights are saved in FP16. This type can be changed when the model is loaded using the [`compute_type` option in CTranslate2](https://opennmt.net/CTranslate2/quantization.html).
## More information
**For more information about the original model, see its [model card](https://huggingface.co/openai/whisper-tiny).**
|
LnL-AI/glm-4-9b-chat-gptq-4bit-qubitium-r1 | LnL-AI | "2024-06-07T01:24:52Z" | 98,283 | 0 | transformers | [
"transformers",
"safetensors",
"chatglm",
"custom_code",
"endpoints_compatible",
"4-bit",
"gptq",
"region:us"
] | null | "2024-06-06T18:24:09Z" | World's first gptq 4bit quant of `glm-4-9b-chat` model.
Autogptq PR: https://github.com/AutoGPTQ/AutoGPTQ/pull/683
Please note ChatGLM has tendency to switch from English to Chinese in mid-reply or in direct reply to English prompt. This issue happens in both native and quantized model and needs further investigation. |
chavinlo/alpaca-native | chavinlo | "2023-11-17T23:10:27Z" | 98,184 | 261 | transformers | [
"transformers",
"pytorch",
"llama",
"text-generation",
"autotrain_compatible",
"endpoints_compatible",
"text-generation-inference",
"region:us"
] | text-generation | "2023-03-16T02:37:26Z" | # Stanford Alpaca
This is a replica of Alpaca by Stanford' tatsu
Trained using the original instructions with a minor modification in FSDP mode
# Other versions:
13B: https://huggingface.co/chavinlo/alpaca-13b
13B -> GPT4 : https://huggingface.co/chavinlo/gpt4-x-alpaca
## Compute Used
Trained on 4xA100s for 6H
Donated by redmond.ai
NO LORA HAS BEEN USED, this is a natively-finetuned model, hence "alpaca-native"
If you are interested on more llama-based models, you can check out my profile or search for other models at https://huggingface.co/models?other=llama
This (MIGHT) be a quantized version of this model, but be careful: https://boards.4channel.org/g/thread/92173062#p92182396
CONFIGURATION (default except fsdp):
```shell
torchrun --nproc_per_node=4 --master_port=3045 train.py \
--model_name_or_path /workspace/llama-7b-hf \
--data_path ./alpaca_data.json \
--bf16 True \
--output_dir /workspace/output \
--num_train_epochs 3 \
--per_device_train_batch_size 4 \
--per_device_eval_batch_size 4 \
--gradient_accumulation_steps 8 \
--evaluation_strategy "no" \
--save_strategy "steps" \
--save_steps 200 \
--save_total_limit 1 \
--learning_rate 2e-5 \
--weight_decay 0. \
--warmup_ratio 0.03 \
--lr_scheduler_type "cosine" \
--logging_steps 1 \
--fsdp "shard_grad_op auto_wrap" \
--fsdp_transformer_layer_cls_to_wrap 'LLaMADecoderLayer' \
--tf32 True --report_to="wandb"
```
# [Open LLM Leaderboard Evaluation Results](https://huggingface.co/spaces/HuggingFaceH4/open_llm_leaderboard)
Detailed results can be found [here](https://huggingface.co/datasets/open-llm-leaderboard/details_chavinlo__alpaca-native)
| Metric | Value |
|-----------------------|---------------------------|
| Avg. | 41.96 |
| ARC (25-shot) | 52.3 |
| HellaSwag (10-shot) | 77.09 |
| MMLU (5-shot) | 41.6 |
| TruthfulQA (0-shot) | 37.58 |
| Winogrande (5-shot) | 69.46 |
| GSM8K (5-shot) | 1.44 |
| DROP (3-shot) | 14.23 |
|
google/metricx-23-qe-large-v2p0 | google | "2024-02-07T21:16:28Z" | 98,104 | 4 | transformers | [
"transformers",
"pytorch",
"mt5",
"license:apache-2.0",
"endpoints_compatible",
"region:us"
] | null | "2024-02-07T16:35:44Z" | ---
license: apache-2.0
---
# MetricX-23
*This is not an officially supported Google product.*
**GitHub repository: [https://github.com/google-research/metricx](https://github.com/google-research/metricx)**
This repository contains the MetricX-23 models,
a family of models for automatic evaluation of translations that were proposed
in the WMT'23 Metrics Shared Task submission
[MetricX-23: The Google Submission to the WMT 2023 Metrics Shared Task](https://aclanthology.org/2023.wmt-1.63/).
The models were trained in [T5X](https://github.com/google-research/t5x) and
then converted for use in PyTorch.
## Available Models
There are 6 models available on HuggingFace that vary in the number of
parameters and whether or not the model is reference-based or reference-free
(also known as quality estimation, or QE):
* [MetricX-23-XXL](https://huggingface.co/google/metricx-23-large-v2p0)
* [MetricX-23-XL](https://huggingface.co/google/metricx-23-xl-v2p0)
* [MetricX-23-Large](https://huggingface.co/google/metricx-23-xxl-v2p0)
* [MetricX-23-QE-XXL](https://huggingface.co/google/metricx-23-qe-large-v2p0)
* [MetricX-23-QE-XL](https://huggingface.co/google/metricx-23-qe-xl-v2p0)
* [MetricX-23-QE-Large](https://huggingface.co/google/metricx-23-qe-xxl-v2p0)
We recommend using the XXL model versions for the best agreement with human
judgments of translation quality, the Large versions for best speed, and the
XL for an intermediate use case.
## Changes to the WMT'23 Submission
These models available here are most similar to the primary submission to the WMT'23 Metrics
Shared Task. They are initialized with [mT5](https://aclanthology.org/2021.naacl-main.41/)
then fine-tuned on a combination of direct assessment and MQM data. However,
we made some changes that make these models different from the WMT'23 submissions.
First, the models are trained to regress the actual MQM score rather than a
normalized score between 0 and 1. **That means the output from the MetricX-23
models is a score in the range [0, 25] where lower is better (i.e., it predicts
an error score).**
Second, these models were trained with a larger variety of synthetic data that
makes them more robust to translation edge cases like over- and undertranslation,
described in more detail in the following section.
### Synthetic Data
In order for our MetricX models to learn to identify certain types of bad
translations that are not sufficiently (or at all) represented in the regular
training data, we created synthetic examples and mixed them in during training.
The synthetic training data was generated from the DA datasets ranging from
WMT15 to WMT21 (~ 43 language pairs). In most cases, the synthetic examples have
the candidate translation manipulated so as to turn it into a bad translation
with a specific issue commonly unrecognized by learned metrics.
The table below provides an overview of the various failure modes that we
considered, including brief descriptions of how we prepared the synthetic data
to address them.
| Failure mode | Synthetic example description |
| ----------- | ----------- |
| Undertranslation | Candidate translation with an arbitrary sentence removed (if multi-sentence); alternatively, candidate with a certain proportion of words removed from the end. |
| Overtranslation | Candidate translation duplicated (with space in between). |
| Fluent but unrelated translation | Arbitrary reference of a similar length from the dataset. |
| Gibberish | Text of a similar length as the reference, generated by sampling words from the reference translation vocabulary (built from all references in the data). |
| Missing punctuation | Reference translation with the end punctuation removed (11 punctuation symbols considered). |
| Latin instead of Chinese/Japanese or Hindi/Bengali punctuation | Candidate translation with the language-specific punctuation symbol at the end replaced with the Latin equivalent (e.g., "." instead of "。" or "।"); alternatively, the punctuation symbol is replaced with the Latin equivalent in the reference, keeping the correct one in the candidate. |
| Reference-matching translation | Reference translation copied as the candidate translation (unlike the rest of the synthetic data, these examples are meant to train the metric to predict a perfect score for candidates matching the reference). |
Examples from the first 4 categories were assigned a label corresponding to the
worst score on the given rating scale (e.g., 25 when mixed with MQM training
data), whereas the reference-matching translation examples are assigned the best
score (e.g., 0 when used with MQM data). The missing/incorrect punctuation
examples were labeled with a score slightly worse than perfect.
Note that some of the synthetic datasets are only meaningful in the
reference-based scenario, and we thus excluded them when training a QE variant
of MetricX. These are the Latin-vs-special punctuation and the
reference-matching translation examples.
Most of the synthetic training sets were created using stratified sampling
across target languages, taking 500 examples per target language. One exception
is the missing punctuation set, which used a stratified sample across different
punctuation symbols instead.
When training MetricX, a small proportion of the synthetic examples was mixed
with the regular training examples. During the first-stage fine-tuning on DA
data, each synthetic training set constituted between 0.1% and 1% of all
training examples, whereas in the second-stage fine-tuning on MQM data we used
an even smaller proportion, around 0.05%.
As for evaluating the effect of the synthetic training data on the model's
performance, the DEMETR challenge set - which we originally used to evaluate the
models submitted to the WMT23 Metrics Shared Task - was not adequate anymore. We
therefore created a new DEMETR-style test set based on the WMT22 DA data, with
examples constructed analogically to the synthetic training examples, as
described above. This test set helped us determine the right proportions of
synthetic data for fine-tuning in order to make MetricX robust for the failure
modes in consideration, without sacrificing the system- and segment-level
correlations with human ratings.
## Usage
The code for using MetricX models can be found at [https://github.com/google-research/metricx](https://github.com/google-research/metricx).
The repository contains example prediction scripts, described below.
The `metricx23/predict.py` script contains an example for how to run inference
on the models.
### Reference-Based
Example usage for a reference-based model:
```bash
python -m metricx23.predict \
--tokenizer google/mt5-xl \
--model_name_or_path google/metricx-23-xl-v2p0 \
--max_input_length 1024 \
--batch_size 1 \
--input_file input.jsonl \
--output_file output.jsonl
```
`input.jsonl` is expected to have 1 serialized JSON object per line with
`"reference"` and `"hypothesis"` fields. The output jsonl will be parallel
to `input.jsonl` but additionally contain a `"prediction"` field with the predicted score.
Note that the model was trained with a maximum input length of 1024 tokens, so
significantly increasing that value may lead to unpredictable behavior.
### Reference-Free
Example usage for a reference-free model:
```bash
python -m metricx23.predict \
--tokenizer google/mt5-xl \
--model_name_or_path google/metricx-23-qe-xl-v2p0 \
--max_input_length 1024 \
--batch_size 1 \
--input_file input.jsonl \
--output_file output.jsonl \
--qe
```
`input.jsonl` is expected to have 1 serialized JSON object per line with
`"source"` and `"hypothesis"` fields. The output jsonl will be parallel
to `input.jsonl` but additionally contain a `"prediction"` field with the predicted score.
## Meta-Evaluation
The `metricx23/evaluate.py` script contains code to calculate various correlations
between the MetricX-23 scores and MQM ratings of translation quality using the
[MT Metrics Eval](https://github.com/google-research/mt-metrics-eval) library.
Example usage:
```bash
python -m metricx23.evaluate \
--dataset wmt22 \
--lp en-de \
--input_file input.jsonl \
--output_file output.json
```
`input.jsonl` is expected to have one JSON object serialized per line.
Each JSON object is expected to contain 4 fields:
* `"system_id"`: The name of the system that generated the translation.
* `"segment_id"`: The 0-based index of the corresponding segment in the MT
Metrics Eval data.
* `"label"`: The ground-truth translation quality score (with higher is better).
* `"prediction"`: The model predicted translation quality score (with lower is
better; the script negates the scores so higher is better).
The script will calculate the 4 agreement/correlations that were used in the
WMT'23 Shared Task. Below are the results for the MetricX-23 models on the
WMT'22 Metrics Shared Task data:
English-German:
| Model | System-Level Accuracy | System-Level Pearson | Segment-Level Pearson | Segment-Level Pairwise Acc |
| ----------- | ----------- | ----------- | ----------- | ----------- |
| MetricX-23-XXL | 0.795 | 0.835 | 0.546 | 0.619 |
| MetricX-23-XL | 0.756 | 0.813 | 0.540 | 0.605 |
| MetricX-23-Large | 0.769 | 0.759 | 0.507 | 0.595 |
| MetricX-23-QE-XXL | 0.769 | 0.830 | 0.490 | 0.606 |
| MetricX-23-QE-XL | 0.718 | 0.684 | 0.421 | 0.594 |
| MetricX-23-QE-Large | 0.744 | 0.671 | 0.387 | 0.579 |
English-Russian:
| Model | System-Level Accuracy | System-Level Pearson | Segment-Level Pearson | Segment-Level Pairwise Acc |
| ----------- | ----------- | ----------- | ----------- | ----------- |
| MetricX-23-XXL | 0.905 | 0.943 | 0.477 | 0.609 |
| MetricX-23-XL | 0.876 | 0.906 | 0.498 | 0.589 |
| MetricX-23-Large | 0.876 | 0.841 | 0.474 | 0.569 |
| MetricX-23-QE-XXL | 0.895 | 0.940 | 0.470 | 0.602 |
| MetricX-23-QE-XL | 0.848 | 0.861 | 0.415 | 0.570 |
| MetricX-23-QE-Large | 0.819 | 0.778 | 0.411 | 0.551 |
Chinese-English:
| Model | System-Level Accuracy | System-Level Pearson | Segment-Level Pearson | Segment-Level Pairwise Acc |
| ----------- | ----------- | ----------- | ----------- | ----------- |
| MetricX-23-XXL | 0.868 | 0.919 | 0.605 | 0.551 |
| MetricX-23-XL | 0.868 | 0.924 | 0.584 | 0.543 |
| MetricX-23-Large | 0.857 | 0.919 | 0.555 | 0.539 |
| MetricX-23-QE-XXL | 0.857 | 0.928 | 0.573 | 0.544 |
| MetricX-23-QE-XL | 0.802 | 0.879 | 0.546 | 0.529 |
| MetricX-23-QE-Large | 0.758 | 0.904 | 0.522 | 0.529 |
The `metricx23/evaluate_wmt23.py` script re-calculates the average correlation
score that was used to rank submissions from the
[WMT'23 Shared Task](https://www2.statmt.org/wmt23/pdf/2023.wmt-1.51.pdf).
Example usage:
```bash
python -m metricx23.evaluate_wmt23 \
--en_de predictions_ende.jsonl \
--he_en predictions_heen.jsonl \
--zh_en predictions_zhen.jsonl \
--output_file output.json
```
Each of the 3 input files is expected to be in the same format as described
above. Each file should correspond to running inference on each of the language
pairs from the WMT'23 dataset.
The results for each of the models is the following:
| Model | Average Correlation |
| ----------- | ----------- |
| MetricX-23-XXL | 0.812 |
| MetricX-23-XL | 0.813 |
| MetricX-23-Large | 0.794 |
| MetricX-23-QE-XXL | 0.797 |
| MetricX-23-QE-XL | 0.767 |
| MetricX-23-QE-Large | 0.762 |
## Citation
If you use MetricX-23 in your research, please cite the following publication:
```bibtex
@inproceedings{juraska-etal-2023-metricx,
title = {{MetricX-23: The Google Submission to the WMT 2023 Metrics Shared Task}},
author = "Juraska, Juraj and
Finkelstein, Mara and
Deutsch, Daniel and
Siddhant, Aditya and
Mirzazadeh, Mehdi and
Freitag, Markus",
editor = "Koehn, Philipp and
Haddow, Barry and
Kocmi, Tom and
Monz, Christof",
booktitle = "Proceedings of the Eighth Conference on Machine Translation",
month = dec,
year = "2023",
address = "Singapore",
publisher = "Association for Computational Linguistics",
url = "https://aclanthology.org/2023.wmt-1.63",
doi = "10.18653/v1/2023.wmt-1.63",
pages = "756--767",
}
``` |
RichardErkhov/MaziyarPanahi_-_Mixtral-8x7B-Instruct-v0.1-gguf | RichardErkhov | "2024-06-29T05:45:08Z" | 97,943 | 0 | null | [
"gguf",
"region:us"
] | null | "2024-06-28T09:49:50Z" | Quantization made by Richard Erkhov.
[Github](https://github.com/RichardErkhov)
[Discord](https://discord.gg/pvy7H8DZMG)
[Request more models](https://github.com/RichardErkhov/quant_request)
Mixtral-8x7B-Instruct-v0.1 - GGUF
- Model creator: https://huggingface.co/MaziyarPanahi/
- Original model: https://huggingface.co/MaziyarPanahi/Mixtral-8x7B-Instruct-v0.1/
| Name | Quant method | Size |
| ---- | ---- | ---- |
| [Mixtral-8x7B-Instruct-v0.1.Q2_K.gguf](https://huggingface.co/RichardErkhov/MaziyarPanahi_-_Mixtral-8x7B-Instruct-v0.1-gguf/blob/main/Mixtral-8x7B-Instruct-v0.1.Q2_K.gguf) | Q2_K | 16.12GB |
| [Mixtral-8x7B-Instruct-v0.1.IQ3_XS.gguf](https://huggingface.co/RichardErkhov/MaziyarPanahi_-_Mixtral-8x7B-Instruct-v0.1-gguf/blob/main/Mixtral-8x7B-Instruct-v0.1.IQ3_XS.gguf) | IQ3_XS | 18.02GB |
| [Mixtral-8x7B-Instruct-v0.1.IQ3_S.gguf](https://huggingface.co/RichardErkhov/MaziyarPanahi_-_Mixtral-8x7B-Instruct-v0.1-gguf/blob/main/Mixtral-8x7B-Instruct-v0.1.IQ3_S.gguf) | IQ3_S | 19.03GB |
| [Mixtral-8x7B-Instruct-v0.1.Q3_K_S.gguf](https://huggingface.co/RichardErkhov/MaziyarPanahi_-_Mixtral-8x7B-Instruct-v0.1-gguf/blob/main/Mixtral-8x7B-Instruct-v0.1.Q3_K_S.gguf) | Q3_K_S | 19.03GB |
| [Mixtral-8x7B-Instruct-v0.1.IQ3_M.gguf](https://huggingface.co/RichardErkhov/MaziyarPanahi_-_Mixtral-8x7B-Instruct-v0.1-gguf/blob/main/Mixtral-8x7B-Instruct-v0.1.IQ3_M.gguf) | IQ3_M | 19.96GB |
| [Mixtral-8x7B-Instruct-v0.1.Q3_K.gguf](https://huggingface.co/RichardErkhov/MaziyarPanahi_-_Mixtral-8x7B-Instruct-v0.1-gguf/blob/main/Mixtral-8x7B-Instruct-v0.1.Q3_K.gguf) | Q3_K | 21.0GB |
| [Mixtral-8x7B-Instruct-v0.1.Q3_K_M.gguf](https://huggingface.co/RichardErkhov/MaziyarPanahi_-_Mixtral-8x7B-Instruct-v0.1-gguf/blob/main/Mixtral-8x7B-Instruct-v0.1.Q3_K_M.gguf) | Q3_K_M | 21.0GB |
| [Mixtral-8x7B-Instruct-v0.1.Q3_K_L.gguf](https://huggingface.co/RichardErkhov/MaziyarPanahi_-_Mixtral-8x7B-Instruct-v0.1-gguf/blob/main/Mixtral-8x7B-Instruct-v0.1.Q3_K_L.gguf) | Q3_K_L | 22.51GB |
| [Mixtral-8x7B-Instruct-v0.1.IQ4_XS.gguf](https://huggingface.co/RichardErkhov/MaziyarPanahi_-_Mixtral-8x7B-Instruct-v0.1-gguf/blob/main/Mixtral-8x7B-Instruct-v0.1.IQ4_XS.gguf) | IQ4_XS | 23.63GB |
| [Mixtral-8x7B-Instruct-v0.1.Q4_0.gguf](https://huggingface.co/RichardErkhov/MaziyarPanahi_-_Mixtral-8x7B-Instruct-v0.1-gguf/blob/main/Mixtral-8x7B-Instruct-v0.1.Q4_0.gguf) | Q4_0 | 24.63GB |
| [Mixtral-8x7B-Instruct-v0.1.IQ4_NL.gguf](https://huggingface.co/RichardErkhov/MaziyarPanahi_-_Mixtral-8x7B-Instruct-v0.1-gguf/blob/main/Mixtral-8x7B-Instruct-v0.1.IQ4_NL.gguf) | IQ4_NL | 24.91GB |
| [Mixtral-8x7B-Instruct-v0.1.Q4_K_S.gguf](https://huggingface.co/RichardErkhov/MaziyarPanahi_-_Mixtral-8x7B-Instruct-v0.1-gguf/blob/main/Mixtral-8x7B-Instruct-v0.1.Q4_K_S.gguf) | Q4_K_S | 24.91GB |
| [Mixtral-8x7B-Instruct-v0.1.Q4_K.gguf](https://huggingface.co/RichardErkhov/MaziyarPanahi_-_Mixtral-8x7B-Instruct-v0.1-gguf/blob/main/Mixtral-8x7B-Instruct-v0.1.Q4_K.gguf) | Q4_K | 26.49GB |
| [Mixtral-8x7B-Instruct-v0.1.Q4_K_M.gguf](https://huggingface.co/RichardErkhov/MaziyarPanahi_-_Mixtral-8x7B-Instruct-v0.1-gguf/blob/main/Mixtral-8x7B-Instruct-v0.1.Q4_K_M.gguf) | Q4_K_M | 26.49GB |
| [Mixtral-8x7B-Instruct-v0.1.Q4_1.gguf](https://huggingface.co/RichardErkhov/MaziyarPanahi_-_Mixtral-8x7B-Instruct-v0.1-gguf/blob/main/Mixtral-8x7B-Instruct-v0.1.Q4_1.gguf) | Q4_1 | 27.32GB |
| [Mixtral-8x7B-Instruct-v0.1.Q5_0.gguf](https://huggingface.co/RichardErkhov/MaziyarPanahi_-_Mixtral-8x7B-Instruct-v0.1-gguf/blob/main/Mixtral-8x7B-Instruct-v0.1.Q5_0.gguf) | Q5_0 | 30.02GB |
| [Mixtral-8x7B-Instruct-v0.1.Q5_K_S.gguf](https://huggingface.co/RichardErkhov/MaziyarPanahi_-_Mixtral-8x7B-Instruct-v0.1-gguf/blob/main/Mixtral-8x7B-Instruct-v0.1.Q5_K_S.gguf) | Q5_K_S | 30.02GB |
| [Mixtral-8x7B-Instruct-v0.1.Q5_K.gguf](https://huggingface.co/RichardErkhov/MaziyarPanahi_-_Mixtral-8x7B-Instruct-v0.1-gguf/blob/main/Mixtral-8x7B-Instruct-v0.1.Q5_K.gguf) | Q5_K | 30.95GB |
| [Mixtral-8x7B-Instruct-v0.1.Q5_K_M.gguf](https://huggingface.co/RichardErkhov/MaziyarPanahi_-_Mixtral-8x7B-Instruct-v0.1-gguf/blob/main/Mixtral-8x7B-Instruct-v0.1.Q5_K_M.gguf) | Q5_K_M | 30.95GB |
| [Mixtral-8x7B-Instruct-v0.1.Q5_1.gguf](https://huggingface.co/RichardErkhov/MaziyarPanahi_-_Mixtral-8x7B-Instruct-v0.1-gguf/blob/main/Mixtral-8x7B-Instruct-v0.1.Q5_1.gguf) | Q5_1 | 32.71GB |
| [Mixtral-8x7B-Instruct-v0.1.Q6_K.gguf](https://huggingface.co/RichardErkhov/MaziyarPanahi_-_Mixtral-8x7B-Instruct-v0.1-gguf/blob/main/Mixtral-8x7B-Instruct-v0.1.Q6_K.gguf) | Q6_K | 35.74GB |
| [Mixtral-8x7B-Instruct-v0.1.Q8_0.gguf](https://huggingface.co/RichardErkhov/MaziyarPanahi_-_Mixtral-8x7B-Instruct-v0.1-gguf/tree/main/) | Q8_0 | 46.22GB |
Original model description:
---
license: apache-2.0
language:
- fr
- it
- de
- es
- en
inference:
parameters:
temperature: 0.5
widget:
- messages:
- role: user
content: What is your favorite condiment?
---
# Model Card for Mixtral-8x7B
The Mixtral-8x7B Large Language Model (LLM) is a pretrained generative Sparse Mixture of Experts. The Mixtral-8x7B outperforms Llama 2 70B on most benchmarks we tested.
For full details of this model please read our [release blog post](https://mistral.ai/news/mixtral-of-experts/).
## Warning
This repo contains weights that are compatible with [vLLM](https://github.com/vllm-project/vllm) serving of the model as well as Hugging Face [transformers](https://github.com/huggingface/transformers) library. It is based on the original Mixtral [torrent release](magnet:?xt=urn:btih:5546272da9065eddeb6fcd7ffddeef5b75be79a7&dn=mixtral-8x7b-32kseqlen&tr=udp%3A%2F%http://2Fopentracker.i2p.rocks%3A6969%2Fannounce&tr=http%3A%2F%http://2Ftracker.openbittorrent.com%3A80%2Fannounce), but the file format and parameter names are different. Please note that model cannot (yet) be instantiated with HF.
## Instruction format
This format must be strictly respected, otherwise the model will generate sub-optimal outputs.
The template used to build a prompt for the Instruct model is defined as follows:
```
<s> [INST] Instruction [/INST] Model answer</s> [INST] Follow-up instruction [/INST]
```
Note that `<s>` and `</s>` are special tokens for beginning of string (BOS) and end of string (EOS) while [INST] and [/INST] are regular strings.
As reference, here is the pseudo-code used to tokenize instructions during fine-tuning:
```python
def tokenize(text):
return tok.encode(text, add_special_tokens=False)
[BOS_ID] +
tokenize("[INST]") + tokenize(USER_MESSAGE_1) + tokenize("[/INST]") +
tokenize(BOT_MESSAGE_1) + [EOS_ID] +
…
tokenize("[INST]") + tokenize(USER_MESSAGE_N) + tokenize("[/INST]") +
tokenize(BOT_MESSAGE_N) + [EOS_ID]
```
In the pseudo-code above, note that the `tokenize` method should not add a BOS or EOS token automatically, but should add a prefix space.
In the Transformers library, one can use [chat templates](https://huggingface.co/docs/transformers/main/en/chat_templating) which make sure the right format is applied.
## Run the model
```python
from transformers import AutoModelForCausalLM, AutoTokenizer
model_id = "mistralai/Mixtral-8x7B-Instruct-v0.1"
tokenizer = AutoTokenizer.from_pretrained(model_id)
model = AutoModelForCausalLM.from_pretrained(model_id, device_map="auto")
messages = [
{"role": "user", "content": "What is your favourite condiment?"},
{"role": "assistant", "content": "Well, I'm quite partial to a good squeeze of fresh lemon juice. It adds just the right amount of zesty flavour to whatever I'm cooking up in the kitchen!"},
{"role": "user", "content": "Do you have mayonnaise recipes?"}
]
inputs = tokenizer.apply_chat_template(messages, return_tensors="pt").to("cuda")
outputs = model.generate(inputs, max_new_tokens=20)
print(tokenizer.decode(outputs[0], skip_special_tokens=True))
```
By default, transformers will load the model in full precision. Therefore you might be interested to further reduce down the memory requirements to run the model through the optimizations we offer in HF ecosystem:
### In half-precision
Note `float16` precision only works on GPU devices
<details>
<summary> Click to expand </summary>
```diff
+ import torch
from transformers import AutoModelForCausalLM, AutoTokenizer
model_id = "mistralai/Mixtral-8x7B-Instruct-v0.1"
tokenizer = AutoTokenizer.from_pretrained(model_id)
+ model = AutoModelForCausalLM.from_pretrained(model_id, torch_dtype=torch.float16, device_map="auto")
messages = [
{"role": "user", "content": "What is your favourite condiment?"},
{"role": "assistant", "content": "Well, I'm quite partial to a good squeeze of fresh lemon juice. It adds just the right amount of zesty flavour to whatever I'm cooking up in the kitchen!"},
{"role": "user", "content": "Do you have mayonnaise recipes?"}
]
input_ids = tokenizer.apply_chat_template(messages, return_tensors="pt").to("cuda")
outputs = model.generate(input_ids, max_new_tokens=20)
print(tokenizer.decode(outputs[0], skip_special_tokens=True))
```
</details>
### Lower precision using (8-bit & 4-bit) using `bitsandbytes`
<details>
<summary> Click to expand </summary>
```diff
+ import torch
from transformers import AutoModelForCausalLM, AutoTokenizer
model_id = "mistralai/Mixtral-8x7B-Instruct-v0.1"
tokenizer = AutoTokenizer.from_pretrained(model_id)
+ model = AutoModelForCausalLM.from_pretrained(model_id, load_in_4bit=True, device_map="auto")
text = "Hello my name is"
messages = [
{"role": "user", "content": "What is your favourite condiment?"},
{"role": "assistant", "content": "Well, I'm quite partial to a good squeeze of fresh lemon juice. It adds just the right amount of zesty flavour to whatever I'm cooking up in the kitchen!"},
{"role": "user", "content": "Do you have mayonnaise recipes?"}
]
input_ids = tokenizer.apply_chat_template(messages, return_tensors="pt").to("cuda")
outputs = model.generate(input_ids, max_new_tokens=20)
print(tokenizer.decode(outputs[0], skip_special_tokens=True))
```
</details>
### Load the model with Flash Attention 2
<details>
<summary> Click to expand </summary>
```diff
+ import torch
from transformers import AutoModelForCausalLM, AutoTokenizer
model_id = "mistralai/Mixtral-8x7B-Instruct-v0.1"
tokenizer = AutoTokenizer.from_pretrained(model_id)
+ model = AutoModelForCausalLM.from_pretrained(model_id, use_flash_attention_2=True, device_map="auto")
messages = [
{"role": "user", "content": "What is your favourite condiment?"},
{"role": "assistant", "content": "Well, I'm quite partial to a good squeeze of fresh lemon juice. It adds just the right amount of zesty flavour to whatever I'm cooking up in the kitchen!"},
{"role": "user", "content": "Do you have mayonnaise recipes?"}
]
input_ids = tokenizer.apply_chat_template(messages, return_tensors="pt").to("cuda")
outputs = model.generate(input_ids, max_new_tokens=20)
print(tokenizer.decode(outputs[0], skip_special_tokens=True))
```
</details>
## Limitations
The Mixtral-8x7B Instruct model is a quick demonstration that the base model can be easily fine-tuned to achieve compelling performance.
It does not have any moderation mechanisms. We're looking forward to engaging with the community on ways to
make the model finely respect guardrails, allowing for deployment in environments requiring moderated outputs.
# The Mistral AI Team
Albert Jiang, Alexandre Sablayrolles, Arthur Mensch, Blanche Savary, Chris Bamford, Devendra Singh Chaplot, Diego de las Casas, Emma Bou Hanna, Florian Bressand, Gianna Lengyel, Guillaume Bour, Guillaume Lample, Lélio Renard Lavaud, Louis Ternon, Lucile Saulnier, Marie-Anne Lachaux, Pierre Stock, Teven Le Scao, Théophile Gervet, Thibaut Lavril, Thomas Wang, Timothée Lacroix, William El Sayed.
|
timm/tf_efficientnet_b1.ns_jft_in1k | timm | "2023-04-27T21:17:43Z" | 97,921 | 0 | timm | [
"timm",
"pytorch",
"safetensors",
"image-classification",
"dataset:imagenet-1k",
"arxiv:1905.11946",
"arxiv:1911.04252",
"license:apache-2.0",
"region:us"
] | image-classification | "2022-12-13T00:01:58Z" | ---
tags:
- image-classification
- timm
library_name: timm
license: apache-2.0
datasets:
- imagenet-1k
---
# Model card for tf_efficientnet_b1.ns_jft_in1k
A EfficientNet image classification model. Trained on ImageNet-1k and unlabeled JFT-300m using Noisy Student semi-supervised learning in Tensorflow by paper authors, ported to PyTorch by Ross Wightman.
## Model Details
- **Model Type:** Image classification / feature backbone
- **Model Stats:**
- Params (M): 7.8
- GMACs: 0.7
- Activations (M): 10.9
- Image size: 240 x 240
- **Papers:**
- EfficientNet: Rethinking Model Scaling for Convolutional Neural Networks: https://arxiv.org/abs/1905.11946
- Self-training with Noisy Student improves ImageNet classification: https://arxiv.org/abs/1911.04252
- **Dataset:** ImageNet-1k
- **Original:** https://github.com/tensorflow/tpu/tree/master/models/official/efficientnet
## Model Usage
### Image Classification
```python
from urllib.request import urlopen
from PIL import Image
import timm
img = Image.open(urlopen(
'https://huggingface.co/datasets/huggingface/documentation-images/resolve/main/beignets-task-guide.png'
))
model = timm.create_model('tf_efficientnet_b1.ns_jft_in1k', pretrained=True)
model = model.eval()
# get model specific transforms (normalization, resize)
data_config = timm.data.resolve_model_data_config(model)
transforms = timm.data.create_transform(**data_config, is_training=False)
output = model(transforms(img).unsqueeze(0)) # unsqueeze single image into batch of 1
top5_probabilities, top5_class_indices = torch.topk(output.softmax(dim=1) * 100, k=5)
```
### Feature Map Extraction
```python
from urllib.request import urlopen
from PIL import Image
import timm
img = Image.open(urlopen(
'https://huggingface.co/datasets/huggingface/documentation-images/resolve/main/beignets-task-guide.png'
))
model = timm.create_model(
'tf_efficientnet_b1.ns_jft_in1k',
pretrained=True,
features_only=True,
)
model = model.eval()
# get model specific transforms (normalization, resize)
data_config = timm.data.resolve_model_data_config(model)
transforms = timm.data.create_transform(**data_config, is_training=False)
output = model(transforms(img).unsqueeze(0)) # unsqueeze single image into batch of 1
for o in output:
# print shape of each feature map in output
# e.g.:
# torch.Size([1, 16, 120, 120])
# torch.Size([1, 24, 60, 60])
# torch.Size([1, 40, 30, 30])
# torch.Size([1, 112, 15, 15])
# torch.Size([1, 320, 8, 8])
print(o.shape)
```
### Image Embeddings
```python
from urllib.request import urlopen
from PIL import Image
import timm
img = Image.open(urlopen(
'https://huggingface.co/datasets/huggingface/documentation-images/resolve/main/beignets-task-guide.png'
))
model = timm.create_model(
'tf_efficientnet_b1.ns_jft_in1k',
pretrained=True,
num_classes=0, # remove classifier nn.Linear
)
model = model.eval()
# get model specific transforms (normalization, resize)
data_config = timm.data.resolve_model_data_config(model)
transforms = timm.data.create_transform(**data_config, is_training=False)
output = model(transforms(img).unsqueeze(0)) # output is (batch_size, num_features) shaped tensor
# or equivalently (without needing to set num_classes=0)
output = model.forward_features(transforms(img).unsqueeze(0))
# output is unpooled, a (1, 1280, 8, 8) shaped tensor
output = model.forward_head(output, pre_logits=True)
# output is a (1, num_features) shaped tensor
```
## Model Comparison
Explore the dataset and runtime metrics of this model in timm [model results](https://github.com/huggingface/pytorch-image-models/tree/main/results).
## Citation
```bibtex
@inproceedings{tan2019efficientnet,
title={Efficientnet: Rethinking model scaling for convolutional neural networks},
author={Tan, Mingxing and Le, Quoc},
booktitle={International conference on machine learning},
pages={6105--6114},
year={2019},
organization={PMLR}
}
```
```bibtex
@article{Xie2019SelfTrainingWN,
title={Self-Training With Noisy Student Improves ImageNet Classification},
author={Qizhe Xie and Eduard H. Hovy and Minh-Thang Luong and Quoc V. Le},
journal={2020 IEEE/CVF Conference on Computer Vision and Pattern Recognition (CVPR)},
year={2019},
pages={10684-10695}
}
```
```bibtex
@misc{rw2019timm,
author = {Ross Wightman},
title = {PyTorch Image Models},
year = {2019},
publisher = {GitHub},
journal = {GitHub repository},
doi = {10.5281/zenodo.4414861},
howpublished = {\url{https://github.com/huggingface/pytorch-image-models}}
}
```
|
microsoft/DialoGPT-medium | microsoft | "2024-02-29T15:48:54Z" | 97,913 | 304 | transformers | [
"transformers",
"pytorch",
"tf",
"jax",
"rust",
"gpt2",
"text-generation",
"conversational",
"arxiv:1911.00536",
"license:mit",
"autotrain_compatible",
"endpoints_compatible",
"text-generation-inference",
"region:us"
] | text-generation | "2022-03-02T23:29:05Z" | ---
thumbnail: https://huggingface.co/front/thumbnails/dialogpt.png
tags:
- conversational
license: mit
---
## A State-of-the-Art Large-scale Pretrained Response generation model (DialoGPT)
DialoGPT is a SOTA large-scale pretrained dialogue response generation model for multiturn conversations.
The [human evaluation results](https://github.com/dreasysnail/Dialogpt_dev#human-evaluation) indicate that the response generated from DialoGPT is comparable to human response quality under a single-turn conversation Turing test.
The model is trained on 147M multi-turn dialogue from Reddit discussion thread.
* Multi-turn generation examples from an interactive environment:
|Role | Response |
|---------|--------|
|User | Does money buy happiness? |
| Bot | Depends how much money you spend on it .|
|User | What is the best way to buy happiness ? |
| Bot | You just have to be a millionaire by your early 20s, then you can be happy . |
|User |This is so difficult ! |
| Bot | You have no idea how hard it is to be a millionaire and happy . There is a reason the rich have a lot of money |
Please find the information about preprocessing, training and full details of the DialoGPT in the [original DialoGPT repository](https://github.com/microsoft/DialoGPT)
ArXiv paper: [https://arxiv.org/abs/1911.00536](https://arxiv.org/abs/1911.00536)
### How to use
Now we are ready to try out how the model works as a chatting partner!
```python
from transformers import AutoModelForCausalLM, AutoTokenizer
import torch
tokenizer = AutoTokenizer.from_pretrained("microsoft/DialoGPT-medium")
model = AutoModelForCausalLM.from_pretrained("microsoft/DialoGPT-medium")
# Let's chat for 5 lines
for step in range(5):
# encode the new user input, add the eos_token and return a tensor in Pytorch
new_user_input_ids = tokenizer.encode(input(">> User:") + tokenizer.eos_token, return_tensors='pt')
# append the new user input tokens to the chat history
bot_input_ids = torch.cat([chat_history_ids, new_user_input_ids], dim=-1) if step > 0 else new_user_input_ids
# generated a response while limiting the total chat history to 1000 tokens,
chat_history_ids = model.generate(bot_input_ids, max_length=1000, pad_token_id=tokenizer.eos_token_id)
# pretty print last ouput tokens from bot
print("DialoGPT: {}".format(tokenizer.decode(chat_history_ids[:, bot_input_ids.shape[-1]:][0], skip_special_tokens=True)))
```
|
sentence-transformers/bert-large-nli-stsb-mean-tokens | sentence-transformers | "2024-03-27T10:13:39Z" | 97,814 | 3 | sentence-transformers | [
"sentence-transformers",
"pytorch",
"tf",
"safetensors",
"bert",
"feature-extraction",
"sentence-similarity",
"transformers",
"arxiv:1908.10084",
"license:apache-2.0",
"autotrain_compatible",
"endpoints_compatible",
"region:us"
] | sentence-similarity | "2022-03-02T23:29:05Z" | ---
license: apache-2.0
library_name: sentence-transformers
tags:
- sentence-transformers
- feature-extraction
- sentence-similarity
- transformers
pipeline_tag: sentence-similarity
---
**⚠️ This model is deprecated. Please don't use it as it produces sentence embeddings of low quality. You can find recommended sentence embedding models here: [SBERT.net - Pretrained Models](https://www.sbert.net/docs/pretrained_models.html)**
# sentence-transformers/bert-large-nli-stsb-mean-tokens
This is a [sentence-transformers](https://www.SBERT.net) model: It maps sentences & paragraphs to a 1024 dimensional dense vector space and can be used for tasks like clustering or semantic search.
## Usage (Sentence-Transformers)
Using this model becomes easy when you have [sentence-transformers](https://www.SBERT.net) installed:
```
pip install -U sentence-transformers
```
Then you can use the model like this:
```python
from sentence_transformers import SentenceTransformer
sentences = ["This is an example sentence", "Each sentence is converted"]
model = SentenceTransformer('sentence-transformers/bert-large-nli-stsb-mean-tokens')
embeddings = model.encode(sentences)
print(embeddings)
```
## Usage (HuggingFace Transformers)
Without [sentence-transformers](https://www.SBERT.net), you can use the model like this: First, you pass your input through the transformer model, then you have to apply the right pooling-operation on-top of the contextualized word embeddings.
```python
from transformers import AutoTokenizer, AutoModel
import torch
#Mean Pooling - Take attention mask into account for correct averaging
def mean_pooling(model_output, attention_mask):
token_embeddings = model_output[0] #First element of model_output contains all token embeddings
input_mask_expanded = attention_mask.unsqueeze(-1).expand(token_embeddings.size()).float()
return torch.sum(token_embeddings * input_mask_expanded, 1) / torch.clamp(input_mask_expanded.sum(1), min=1e-9)
# Sentences we want sentence embeddings for
sentences = ['This is an example sentence', 'Each sentence is converted']
# Load model from HuggingFace Hub
tokenizer = AutoTokenizer.from_pretrained('sentence-transformers/bert-large-nli-stsb-mean-tokens')
model = AutoModel.from_pretrained('sentence-transformers/bert-large-nli-stsb-mean-tokens')
# Tokenize sentences
encoded_input = tokenizer(sentences, padding=True, truncation=True, return_tensors='pt')
# Compute token embeddings
with torch.no_grad():
model_output = model(**encoded_input)
# Perform pooling. In this case, max pooling.
sentence_embeddings = mean_pooling(model_output, encoded_input['attention_mask'])
print("Sentence embeddings:")
print(sentence_embeddings)
```
## Evaluation Results
For an automated evaluation of this model, see the *Sentence Embeddings Benchmark*: [https://seb.sbert.net](https://seb.sbert.net?model_name=sentence-transformers/bert-large-nli-stsb-mean-tokens)
## Full Model Architecture
```
SentenceTransformer(
(0): Transformer({'max_seq_length': 128, 'do_lower_case': False}) with Transformer model: BertModel
(1): Pooling({'word_embedding_dimension': 1024, 'pooling_mode_cls_token': False, 'pooling_mode_mean_tokens': True, 'pooling_mode_max_tokens': False, 'pooling_mode_mean_sqrt_len_tokens': False})
)
```
## Citing & Authors
This model was trained by [sentence-transformers](https://www.sbert.net/).
If you find this model helpful, feel free to cite our publication [Sentence-BERT: Sentence Embeddings using Siamese BERT-Networks](https://arxiv.org/abs/1908.10084):
```bibtex
@inproceedings{reimers-2019-sentence-bert,
title = "Sentence-BERT: Sentence Embeddings using Siamese BERT-Networks",
author = "Reimers, Nils and Gurevych, Iryna",
booktitle = "Proceedings of the 2019 Conference on Empirical Methods in Natural Language Processing",
month = "11",
year = "2019",
publisher = "Association for Computational Linguistics",
url = "http://arxiv.org/abs/1908.10084",
}
``` |
Helsinki-NLP/opus-mt-ko-en | Helsinki-NLP | "2023-08-16T11:59:39Z" | 97,782 | 31 | transformers | [
"transformers",
"pytorch",
"tf",
"marian",
"text2text-generation",
"translation",
"ko",
"en",
"license:apache-2.0",
"autotrain_compatible",
"endpoints_compatible",
"region:us"
] | translation | "2022-03-02T23:29:04Z" | ---
language:
- ko
- en
tags:
- translation
license: apache-2.0
---
### kor-eng
* source group: Korean
* target group: English
* OPUS readme: [kor-eng](https://github.com/Helsinki-NLP/Tatoeba-Challenge/tree/master/models/kor-eng/README.md)
* model: transformer-align
* source language(s): kor kor_Hang kor_Latn
* target language(s): eng
* model: transformer-align
* pre-processing: normalization + SentencePiece (spm32k,spm32k)
* download original weights: [opus-2020-06-17.zip](https://object.pouta.csc.fi/Tatoeba-MT-models/kor-eng/opus-2020-06-17.zip)
* test set translations: [opus-2020-06-17.test.txt](https://object.pouta.csc.fi/Tatoeba-MT-models/kor-eng/opus-2020-06-17.test.txt)
* test set scores: [opus-2020-06-17.eval.txt](https://object.pouta.csc.fi/Tatoeba-MT-models/kor-eng/opus-2020-06-17.eval.txt)
## Benchmarks
| testset | BLEU | chr-F |
|-----------------------|-------|-------|
| Tatoeba-test.kor.eng | 41.3 | 0.588 |
### System Info:
- hf_name: kor-eng
- source_languages: kor
- target_languages: eng
- opus_readme_url: https://github.com/Helsinki-NLP/Tatoeba-Challenge/tree/master/models/kor-eng/README.md
- original_repo: Tatoeba-Challenge
- tags: ['translation']
- languages: ['ko', 'en']
- src_constituents: {'kor_Hani', 'kor_Hang', 'kor_Latn', 'kor'}
- tgt_constituents: {'eng'}
- src_multilingual: False
- tgt_multilingual: False
- prepro: normalization + SentencePiece (spm32k,spm32k)
- url_model: https://object.pouta.csc.fi/Tatoeba-MT-models/kor-eng/opus-2020-06-17.zip
- url_test_set: https://object.pouta.csc.fi/Tatoeba-MT-models/kor-eng/opus-2020-06-17.test.txt
- src_alpha3: kor
- tgt_alpha3: eng
- short_pair: ko-en
- chrF2_score: 0.588
- bleu: 41.3
- brevity_penalty: 0.9590000000000001
- ref_len: 17711.0
- src_name: Korean
- tgt_name: English
- train_date: 2020-06-17
- src_alpha2: ko
- tgt_alpha2: en
- prefer_old: False
- long_pair: kor-eng
- helsinki_git_sha: 480fcbe0ee1bf4774bcbe6226ad9f58e63f6c535
- transformers_git_sha: 2207e5d8cb224e954a7cba69fa4ac2309e9ff30b
- port_machine: brutasse
- port_time: 2020-08-21-14:41 |
jinaai/jina-clip-v1 | jinaai | "2024-06-10T14:12:39Z" | 97,638 | 165 | transformers | [
"transformers",
"pytorch",
"onnx",
"safetensors",
"jina_clip",
"feature-extraction",
"sentence-similarity",
"mteb",
"clip",
"vision",
"transformers.js",
"custom_code",
"en",
"arxiv:2405.20204",
"license:apache-2.0",
"region:eu"
] | feature-extraction | "2024-05-21T13:52:49Z" | ---
tags:
- feature-extraction
- sentence-similarity
- mteb
- clip
- vision
- transformers.js
language: en
inference: false
license: apache-2.0
library_name: transformers
---
<br><br>
<p align="center">
<img src="https://aeiljuispo.cloudimg.io/v7/https://cdn-uploads.huggingface.co/production/uploads/603763514de52ff951d89793/AFoybzd5lpBQXEBrQHuTt.png?w=200&h=200&f=face" alt="Finetuner logo: Finetuner helps you to create experiments in order to improve embeddings on search tasks. It accompanies you to deliver the last mile of performance-tuning for neural search applications." width="150px">
</p>
<p align="center">
<b>The embedding set trained by <a href="https://jina.ai/"><b>Jina AI</b></a>.</b>
</p>
<p align="center">
<b>Jina CLIP: your CLIP model is also your text retriever!</b>
</p>
## Intended Usage & Model Info
`jina-clip-v1` is a state-of-the-art English **multimodal (text-image) embedding model**.
Traditional text embedding models, such as [jina-embeddings-v2-base-en](https://huggingface.co/jinaai/jina-embeddings-v2-base-en), excel in text-to-text retrieval but incapable of cross-modal tasks. Models like [openai/clip-vit-base-patch32](https://huggingface.co/openai/clip-vit-base-patch32) effectively align image and text embeddings but are not optimized for text-to-text retrieval due to their training methodologies and context limitations.
`jina-clip-v1` bridges this gap by offering robust performance in both domains.
Its text component matches the retrieval efficiency of `jina-embeddings-v2-base-en`, while its overall architecture sets a new benchmark for cross-modal retrieval.
This dual capability makes it an excellent tool for multimodal retrieval-augmented generation (MuRAG) applications, enabling seamless text-to-text and text-to-image searches within a single model.
## Data & Parameters
[Check out our paper](https://arxiv.org/abs/2405.20204)
## Usage
1. The easiest way to starting using jina-clip-v1-en is to use Jina AI's [Embeddings API](https://jina.ai/embeddings/).
2. Alternatively, you can use Jina CLIP directly via transformers package.
```python
!pip install transformers einops timm pillow
from transformers import AutoModel
# Initialize the model
model = AutoModel.from_pretrained('jinaai/jina-clip-v1', trust_remote_code=True)
# New meaningful sentences
sentences = ['A blue cat', 'A red cat']
# Public image URLs
image_urls = [
'https://i.pinimg.com/600x315/21/48/7e/21487e8e0970dd366dafaed6ab25d8d8.jpg',
'https://i.pinimg.com/736x/c9/f2/3e/c9f23e212529f13f19bad5602d84b78b.jpg'
]
# Encode text and images
text_embeddings = model.encode_text(sentences)
image_embeddings = model.encode_image(image_urls) # also accepts PIL.image, local filenames, dataURI
# Compute similarities
print(text_embeddings[0] @ text_embeddings[1].T) # text embedding similarity
print(text_embeddings[0] @ image_embeddings[0].T) # text-image cross-modal similarity
print(text_embeddings[0] @ image_embeddings[1].T) # text-image cross-modal similarity
print(text_embeddings[1] @ image_embeddings[0].T) # text-image cross-modal similarity
print(text_embeddings[1] @ image_embeddings[1].T)# text-image cross-modal similarity
```
3. JavaScript developers can use Jina CLIP via the [Transformers.js](https://huggingface.co/docs/transformers.js) library. Note that to use this model, you need to install Transformers.js [v3](https://github.com/xenova/transformers.js/tree/v3) from source using `npm install xenova/transformers.js#v3`.
```js
import { AutoTokenizer, CLIPTextModelWithProjection, AutoProcessor, CLIPVisionModelWithProjection, RawImage, cos_sim } from '@xenova/transformers';
// Load tokenizer and text model
const tokenizer = await AutoTokenizer.from_pretrained('jinaai/jina-clip-v1');
const text_model = await CLIPTextModelWithProjection.from_pretrained('jinaai/jina-clip-v1');
// Load processor and vision model
const processor = await AutoProcessor.from_pretrained('Xenova/clip-vit-base-patch32');
const vision_model = await CLIPVisionModelWithProjection.from_pretrained('jinaai/jina-clip-v1');
// Run tokenization
const texts = ['A blue cat', 'A red cat'];
const text_inputs = tokenizer(texts, { padding: true, truncation: true });
// Compute text embeddings
const { text_embeds } = await text_model(text_inputs);
// Read images and run processor
const urls = [
'https://i.pinimg.com/600x315/21/48/7e/21487e8e0970dd366dafaed6ab25d8d8.jpg',
'https://i.pinimg.com/736x/c9/f2/3e/c9f23e212529f13f19bad5602d84b78b.jpg'
];
const image = await Promise.all(urls.map(url => RawImage.read(url)));
const image_inputs = await processor(image);
// Compute vision embeddings
const { image_embeds } = await vision_model(image_inputs);
// Compute similarities
console.log(cos_sim(text_embeds[0].data, text_embeds[1].data)) // text embedding similarity
console.log(cos_sim(text_embeds[0].data, image_embeds[0].data)) // text-image cross-modal similarity
console.log(cos_sim(text_embeds[0].data, image_embeds[1].data)) // text-image cross-modal similarity
console.log(cos_sim(text_embeds[1].data, image_embeds[0].data)) // text-image cross-modal similarity
console.log(cos_sim(text_embeds[1].data, image_embeds[1].data)) // text-image cross-modal similarity
```
## Performance
### Text-Image Retrieval
| Name | Flickr Image Retr. R@1 | Flickr Image Retr. R@5 | Flickr Text Retr. R@1 | Flickr Text Retr. R@5 |
|------------------|-------------------------|-------------------------|-----------------------|-----------------------|
| ViT-B-32 | 0.597 | 0.8398 | 0.781 | 0.938 |
| ViT-B-16 | 0.6216 | 0.8572 | 0.822 | 0.966 |
| jina-clip | 0.6748 | 0.8902 | 0.811 | 0.965 |
| Name | MSCOCO Image Retr. R@1 | MSCOCO Image Retr. R@5 | MSCOCO Text Retr. R@1 | MSCOCO Text Retr. R@5 |
|------------------|-------------------------|-------------------------|-----------------------|-----------------------|
| ViT-B-32 | 0.342 | 0.6001 | 0.5234 | 0.7634 |
| ViT-B-16 | 0.3309 | 0.5842 | 0.5242 | 0.767 |
| jina-clip | 0.4111 | 0.6644 | 0.5544 | 0.7904 |
### Text-Text Retrieval
| Name | STS12 | STS15 | STS17 | STS13 | STS14 | STS16 | STS22 | STSBenchmark | SummEval |
|-----------------------|--------|--------|--------|--------|--------|--------|--------|--------------|----------|
| jina-embeddings-v2 | 0.7427 | 0.8755 | 0.8888 | 0.833 | 0.7917 | 0.836 | 0.6346 | 0.8404 | 0.3056 |
| jina-clip | 0.7352 | 0.8746 | 0.8976 | 0.8323 | 0.7868 | 0.8377 | 0.6583 | 0.8493 | 0.3048 |
| Name | ArguAna | FiQA2018 | NFCorpus | Quora | SCIDOCS | SciFact | TRECCOVID |
|--------------------|---------|----------|----------|-------|---------|---------|-----------|
| jina-embeddings-v2 | 0.4418 | 0.4158 | 0.3245 | 0.882 | 0.1986 | 0.6668 | 0.6591 |
| jina-clip | 0.4933 | 0.3827 | 0.3352 | 0.8789| 0.2024 | 0.6734 | 0.7161 |
## Contact
Join our [Discord community](https://discord.jina.ai) and chat with other community members about ideas.
## Citation
If you find `jina-clip-v1` useful in your research, please cite the following paper:
```bibtex
@misc{2405.20204,
Author = {Andreas Koukounas and Georgios Mastrapas and Michael Günther and Bo Wang and Scott Martens and Isabelle Mohr and Saba Sturua and Mohammad Kalim Akram and Joan Fontanals Martínez and Saahil Ognawala and Susana Guzman and Maximilian Werk and Nan Wang and Han Xiao},
Title = {Jina CLIP: Your CLIP Model Is Also Your Text Retriever},
Year = {2024},
Eprint = {arXiv:2405.20204},
}
```
## FAQ
### I encounter this problem, what should I do?
```
ValueError: The model class you are passing has a `config_class` attribute that is not consistent with the config class you passed (model has <class 'transformers_modules.jinaai.jina-clip-implementation.7f069e2d54d609ef1ad2eb578c7bf07b5a51de41.configuration_clip.JinaCLIPConfig'> and you passed <class 'transformers_modules.jinaai.jina-clip-implementation.7f069e2d54d609ef1ad2eb578c7bf07b5a51de41.configuration_cli.JinaCLIPConfig'>. Fix one of those so they match!
```
There was a bug in Transformers library between 4.40.x to 4.41.1. You can update transformers to >4.41.2 or <=4.40.0
### Given one query, how can I merge its text-text and text-image cosine similarity?
Our emperical study shows that text-text cosine similarity is normally larger than text-image cosine similarity!
If you want to merge two scores, we recommended 2 ways:
1. weighted average of text-text sim and text-image sim:
```python
combined_scores = sim(text, text) + lambda * sim(text, image) # optimal lambda depends on your dataset, but in general lambda=2 can be a good choice.
```
2. apply z-score normalization before merging scores:
```python
# pseudo code
query_document_mean = np.mean(cos_sim_text_texts)
query_document_std = np.std(cos_sim_text_texts)
text_image_mean = np.mean(cos_sim_text_images)
text_image_std = np.std(cos_sim_text_images)
query_document_sim_normalized = (cos_sim_query_documents - query_document_mean) / query_document_std
text_image_sim_normalized = (cos_sim_text_images - text_image_mean) / text_image_std
``` |
timm/tf_efficientnet_b0.ns_jft_in1k | timm | "2023-04-27T21:17:12Z" | 97,635 | 0 | timm | [
"timm",
"pytorch",
"safetensors",
"image-classification",
"dataset:imagenet-1k",
"arxiv:1905.11946",
"arxiv:1911.04252",
"license:apache-2.0",
"region:us"
] | image-classification | "2022-12-13T00:01:33Z" | ---
tags:
- image-classification
- timm
library_name: timm
license: apache-2.0
datasets:
- imagenet-1k
---
# Model card for tf_efficientnet_b0.ns_jft_in1k
A EfficientNet image classification model. Trained on ImageNet-1k and unlabeled JFT-300m using Noisy Student semi-supervised learning in Tensorflow by paper authors, ported to PyTorch by Ross Wightman.
## Model Details
- **Model Type:** Image classification / feature backbone
- **Model Stats:**
- Params (M): 5.3
- GMACs: 0.4
- Activations (M): 6.7
- Image size: 224 x 224
- **Papers:**
- EfficientNet: Rethinking Model Scaling for Convolutional Neural Networks: https://arxiv.org/abs/1905.11946
- Self-training with Noisy Student improves ImageNet classification: https://arxiv.org/abs/1911.04252
- **Dataset:** ImageNet-1k
- **Original:** https://github.com/tensorflow/tpu/tree/master/models/official/efficientnet
## Model Usage
### Image Classification
```python
from urllib.request import urlopen
from PIL import Image
import timm
img = Image.open(urlopen(
'https://huggingface.co/datasets/huggingface/documentation-images/resolve/main/beignets-task-guide.png'
))
model = timm.create_model('tf_efficientnet_b0.ns_jft_in1k', pretrained=True)
model = model.eval()
# get model specific transforms (normalization, resize)
data_config = timm.data.resolve_model_data_config(model)
transforms = timm.data.create_transform(**data_config, is_training=False)
output = model(transforms(img).unsqueeze(0)) # unsqueeze single image into batch of 1
top5_probabilities, top5_class_indices = torch.topk(output.softmax(dim=1) * 100, k=5)
```
### Feature Map Extraction
```python
from urllib.request import urlopen
from PIL import Image
import timm
img = Image.open(urlopen(
'https://huggingface.co/datasets/huggingface/documentation-images/resolve/main/beignets-task-guide.png'
))
model = timm.create_model(
'tf_efficientnet_b0.ns_jft_in1k',
pretrained=True,
features_only=True,
)
model = model.eval()
# get model specific transforms (normalization, resize)
data_config = timm.data.resolve_model_data_config(model)
transforms = timm.data.create_transform(**data_config, is_training=False)
output = model(transforms(img).unsqueeze(0)) # unsqueeze single image into batch of 1
for o in output:
# print shape of each feature map in output
# e.g.:
# torch.Size([1, 16, 112, 112])
# torch.Size([1, 24, 56, 56])
# torch.Size([1, 40, 28, 28])
# torch.Size([1, 112, 14, 14])
# torch.Size([1, 320, 7, 7])
print(o.shape)
```
### Image Embeddings
```python
from urllib.request import urlopen
from PIL import Image
import timm
img = Image.open(urlopen(
'https://huggingface.co/datasets/huggingface/documentation-images/resolve/main/beignets-task-guide.png'
))
model = timm.create_model(
'tf_efficientnet_b0.ns_jft_in1k',
pretrained=True,
num_classes=0, # remove classifier nn.Linear
)
model = model.eval()
# get model specific transforms (normalization, resize)
data_config = timm.data.resolve_model_data_config(model)
transforms = timm.data.create_transform(**data_config, is_training=False)
output = model(transforms(img).unsqueeze(0)) # output is (batch_size, num_features) shaped tensor
# or equivalently (without needing to set num_classes=0)
output = model.forward_features(transforms(img).unsqueeze(0))
# output is unpooled, a (1, 1280, 7, 7) shaped tensor
output = model.forward_head(output, pre_logits=True)
# output is a (1, num_features) shaped tensor
```
## Model Comparison
Explore the dataset and runtime metrics of this model in timm [model results](https://github.com/huggingface/pytorch-image-models/tree/main/results).
## Citation
```bibtex
@inproceedings{tan2019efficientnet,
title={Efficientnet: Rethinking model scaling for convolutional neural networks},
author={Tan, Mingxing and Le, Quoc},
booktitle={International conference on machine learning},
pages={6105--6114},
year={2019},
organization={PMLR}
}
```
```bibtex
@article{Xie2019SelfTrainingWN,
title={Self-Training With Noisy Student Improves ImageNet Classification},
author={Qizhe Xie and Eduard H. Hovy and Minh-Thang Luong and Quoc V. Le},
journal={2020 IEEE/CVF Conference on Computer Vision and Pattern Recognition (CVPR)},
year={2019},
pages={10684-10695}
}
```
```bibtex
@misc{rw2019timm,
author = {Ross Wightman},
title = {PyTorch Image Models},
year = {2019},
publisher = {GitHub},
journal = {GitHub repository},
doi = {10.5281/zenodo.4414861},
howpublished = {\url{https://github.com/huggingface/pytorch-image-models}}
}
```
|
mradermacher/Llama-3-Swallow-70B-v0.1-GGUF | mradermacher | "2024-07-02T22:59:50Z" | 97,305 | 0 | transformers | [
"transformers",
"gguf",
"en",
"ja",
"base_model:tokyotech-llm/Llama-3-Swallow-70B-v0.1",
"license:llama3",
"endpoints_compatible",
"region:us"
] | null | "2024-07-01T19:25:47Z" | ---
base_model: tokyotech-llm/Llama-3-Swallow-70B-v0.1
language:
- en
- ja
library_name: transformers
license: llama3
model_type: llama
quantized_by: mradermacher
---
## About
<!-- ### quantize_version: 2 -->
<!-- ### output_tensor_quantised: 1 -->
<!-- ### convert_type: hf -->
<!-- ### vocab_type: -->
<!-- ### tags: -->
static quants of https://huggingface.co/tokyotech-llm/Llama-3-Swallow-70B-v0.1
<!-- provided-files -->
weighted/imatrix quants are available at https://huggingface.co/mradermacher/Llama-3-Swallow-70B-v0.1-i1-GGUF
## Usage
If you are unsure how to use GGUF files, refer to one of [TheBloke's
READMEs](https://huggingface.co/TheBloke/KafkaLM-70B-German-V0.1-GGUF) for
more details, including on how to concatenate multi-part files.
## Provided Quants
(sorted by size, not necessarily quality. IQ-quants are often preferable over similar sized non-IQ quants)
| Link | Type | Size/GB | Notes |
|:-----|:-----|--------:|:------|
| [GGUF](https://huggingface.co/mradermacher/Llama-3-Swallow-70B-v0.1-GGUF/resolve/main/Llama-3-Swallow-70B-v0.1.Q2_K.gguf) | Q2_K | 26.5 | |
| [GGUF](https://huggingface.co/mradermacher/Llama-3-Swallow-70B-v0.1-GGUF/resolve/main/Llama-3-Swallow-70B-v0.1.IQ3_XS.gguf) | IQ3_XS | 29.4 | |
| [GGUF](https://huggingface.co/mradermacher/Llama-3-Swallow-70B-v0.1-GGUF/resolve/main/Llama-3-Swallow-70B-v0.1.IQ3_S.gguf) | IQ3_S | 31.0 | beats Q3_K* |
| [GGUF](https://huggingface.co/mradermacher/Llama-3-Swallow-70B-v0.1-GGUF/resolve/main/Llama-3-Swallow-70B-v0.1.Q3_K_S.gguf) | Q3_K_S | 31.0 | |
| [GGUF](https://huggingface.co/mradermacher/Llama-3-Swallow-70B-v0.1-GGUF/resolve/main/Llama-3-Swallow-70B-v0.1.IQ3_M.gguf) | IQ3_M | 32.0 | |
| [GGUF](https://huggingface.co/mradermacher/Llama-3-Swallow-70B-v0.1-GGUF/resolve/main/Llama-3-Swallow-70B-v0.1.Q3_K_M.gguf) | Q3_K_M | 34.4 | lower quality |
| [GGUF](https://huggingface.co/mradermacher/Llama-3-Swallow-70B-v0.1-GGUF/resolve/main/Llama-3-Swallow-70B-v0.1.Q3_K_L.gguf) | Q3_K_L | 37.2 | |
| [GGUF](https://huggingface.co/mradermacher/Llama-3-Swallow-70B-v0.1-GGUF/resolve/main/Llama-3-Swallow-70B-v0.1.IQ4_XS.gguf) | IQ4_XS | 38.4 | |
| [GGUF](https://huggingface.co/mradermacher/Llama-3-Swallow-70B-v0.1-GGUF/resolve/main/Llama-3-Swallow-70B-v0.1.Q4_K_S.gguf) | Q4_K_S | 40.4 | fast, recommended |
| [GGUF](https://huggingface.co/mradermacher/Llama-3-Swallow-70B-v0.1-GGUF/resolve/main/Llama-3-Swallow-70B-v0.1.Q4_K_M.gguf) | Q4_K_M | 42.6 | fast, recommended |
| [GGUF](https://huggingface.co/mradermacher/Llama-3-Swallow-70B-v0.1-GGUF/resolve/main/Llama-3-Swallow-70B-v0.1.Q5_K_S.gguf) | Q5_K_S | 48.8 | |
| [GGUF](https://huggingface.co/mradermacher/Llama-3-Swallow-70B-v0.1-GGUF/resolve/main/Llama-3-Swallow-70B-v0.1.Q5_K_M.gguf) | Q5_K_M | 50.0 | |
| [PART 1](https://huggingface.co/mradermacher/Llama-3-Swallow-70B-v0.1-GGUF/resolve/main/Llama-3-Swallow-70B-v0.1.Q6_K.gguf.part1of2) [PART 2](https://huggingface.co/mradermacher/Llama-3-Swallow-70B-v0.1-GGUF/resolve/main/Llama-3-Swallow-70B-v0.1.Q6_K.gguf.part2of2) | Q6_K | 58.0 | very good quality |
| [PART 1](https://huggingface.co/mradermacher/Llama-3-Swallow-70B-v0.1-GGUF/resolve/main/Llama-3-Swallow-70B-v0.1.Q8_0.gguf.part1of2) [PART 2](https://huggingface.co/mradermacher/Llama-3-Swallow-70B-v0.1-GGUF/resolve/main/Llama-3-Swallow-70B-v0.1.Q8_0.gguf.part2of2) | Q8_0 | 75.1 | fast, best quality |
Here is a handy graph by ikawrakow comparing some lower-quality quant
types (lower is better):

And here are Artefact2's thoughts on the matter:
https://gist.github.com/Artefact2/b5f810600771265fc1e39442288e8ec9
## FAQ / Model Request
See https://huggingface.co/mradermacher/model_requests for some answers to
questions you might have and/or if you want some other model quantized.
## Thanks
I thank my company, [nethype GmbH](https://www.nethype.de/), for letting
me use its servers and providing upgrades to my workstation to enable
this work in my free time.
<!-- end -->
|
monologg/kobigbird-bert-base | monologg | "2023-06-12T12:30:09Z" | 97,144 | 19 | transformers | [
"transformers",
"pytorch",
"safetensors",
"big_bird",
"fill-mask",
"korean",
"ko",
"autotrain_compatible",
"endpoints_compatible",
"region:us"
] | fill-mask | "2022-03-02T23:29:05Z" | ---
language: ko
tags:
- korean
mask_token: "[MASK]"
widget:
- text: 대한민국의 수도는 [MASK] 입니다.
---
# KoBigBird
<img src="https://user-images.githubusercontent.com/28896432/140442206-e34b02d5-e279-47e5-9c2a-db1278b1c14d.png" width="200"/>
Pretrained BigBird Model for Korean (**kobigbird-bert-base**)
## About
BigBird, is a sparse-attention based transformer which extends Transformer based models, such as BERT to much longer sequences.
BigBird relies on **block sparse attention** instead of normal attention (i.e. BERT's attention) and can handle sequences up to a length of 4096 at a much lower compute cost compared to BERT.
Model is warm started from Korean BERT’s checkpoint.
## How to use
*NOTE:* Use `BertTokenizer` instead of BigBirdTokenizer. (`AutoTokenizer` will load `BertTokenizer`)
```python
from transformers import AutoModel, AutoTokenizer
# by default its in `block_sparse` mode with num_random_blocks=3, block_size=64
model = AutoModel.from_pretrained("monologg/kobigbird-bert-base")
# you can change `attention_type` to full attention like this:
model = AutoModel.from_pretrained("monologg/kobigbird-bert-base", attention_type="original_full")
# you can change `block_size` & `num_random_blocks` like this:
model = AutoModel.from_pretrained("monologg/kobigbird-bert-base", block_size=16, num_random_blocks=2)
tokenizer = AutoTokenizer.from_pretrained("monologg/kobigbird-bert-base")
text = "한국어 BigBird 모델을 공개합니다!"
encoded_input = tokenizer(text, return_tensors='pt')
output = model(**encoded_input)
```
|
SpanBERT/spanbert-large-cased | SpanBERT | "2021-05-19T11:31:33Z" | 96,820 | 11 | transformers | [
"transformers",
"pytorch",
"jax",
"bert",
"endpoints_compatible",
"region:us"
] | null | "2022-03-02T23:29:05Z" | Entry not found |
facebook/sam-vit-large | facebook | "2024-01-11T19:23:46Z" | 96,595 | 21 | transformers | [
"transformers",
"pytorch",
"tf",
"safetensors",
"sam",
"mask-generation",
"vision",
"license:apache-2.0",
"endpoints_compatible",
"region:us"
] | mask-generation | "2023-04-19T14:17:03Z" | ---
license: apache-2.0
tags:
- vision
---
# Model Card for Segment Anything Model (SAM) - ViT Large (ViT-L) version
<p>
<img src="https://huggingface.co/datasets/huggingface/documentation-images/resolve/main/transformers/model_doc/sam-architecture.png" alt="Model architecture">
<em> Detailed architecture of Segment Anything Model (SAM).</em>
</p>
# Table of Contents
0. [TL;DR](#TL;DR)
1. [Model Details](#model-details)
2. [Usage](#usage)
3. [Citation](#citation)
# TL;DR
[Link to original repository](https://github.com/facebookresearch/segment-anything)
| <img src="https://huggingface.co/datasets/huggingface/documentation-images/resolve/main/transformers/model_doc/sam-beancans.png" alt="Snow" width="600" height="600"> | <img src="https://huggingface.co/facebook/sam-vit-huge/discussions/7" alt="Forest" width="600" height="600"> | <img src="https://huggingface.co/datasets/huggingface/documentation-images/resolve/main/transformers/model_doc/sam-car-seg.png" alt="Mountains" width="600" height="600"> |
|---------------------------------------------------------------------------------------------------------------------------------------|--------------------------------------------------------------------------------------------------------------------------------|--------------------------------------------------------------------------------------------------------------------------------------------------|
The **Segment Anything Model (SAM)** produces high quality object masks from input prompts such as points or boxes, and it can be used to generate masks for all objects in an image. It has been trained on a [dataset](https://segment-anything.com/dataset/index.html) of 11 million images and 1.1 billion masks, and has strong zero-shot performance on a variety of segmentation tasks.
The abstract of the paper states:
> We introduce the Segment Anything (SA) project: a new task, model, and dataset for image segmentation. Using our efficient model in a data collection loop, we built the largest segmentation dataset to date (by far), with over 1 billion masks on 11M licensed and privacy respecting images. The model is designed and trained to be promptable, so it can transfer zero-shot to new image distributions and tasks. We evaluate its capabilities on numerous tasks and find that its zero-shot performance is impressive -- often competitive with or even superior to prior fully supervised results. We are releasing the Segment Anything Model (SAM) and corresponding dataset (SA-1B) of 1B masks and 11M images at [https://segment-anything.com](https://segment-anything.com) to foster research into foundation models for computer vision.
**Disclaimer**: Content from **this** model card has been written by the Hugging Face team, and parts of it were copy pasted from the original [SAM model card](https://github.com/facebookresearch/segment-anything).
# Model Details
The SAM model is made up of 3 modules:
- The `VisionEncoder`: a VIT based image encoder. It computes the image embeddings using attention on patches of the image. Relative Positional Embedding is used.
- The `PromptEncoder`: generates embeddings for points and bounding boxes
- The `MaskDecoder`: a two-ways transformer which performs cross attention between the image embedding and the point embeddings (->) and between the point embeddings and the image embeddings. The outputs are fed
- The `Neck`: predicts the output masks based on the contextualized masks produced by the `MaskDecoder`.
# Usage
## Prompted-Mask-Generation
```python
from PIL import Image
import requests
from transformers import SamModel, SamProcessor
model = SamModel.from_pretrained("facebook/sam-vit-large")
processor = SamProcessor.from_pretrained("facebook/sam-vit-large")
img_url = "https://huggingface.co/ybelkada/segment-anything/resolve/main/assets/car.png"
raw_image = Image.open(requests.get(img_url, stream=True).raw).convert("RGB")
input_points = [[[450, 600]]] # 2D localization of a window
```
```python
inputs = processor(raw_image, input_points=input_points, return_tensors="pt").to("cuda")
outputs = model(**inputs)
masks = processor.image_processor.post_process_masks(outputs.pred_masks.cpu(), inputs["original_sizes"].cpu(), inputs["reshaped_input_sizes"].cpu())
scores = outputs.iou_scores
```
Among other arguments to generate masks, you can pass 2D locations on the approximate position of your object of interest, a bounding box wrapping the object of interest (the format should be x, y coordinate of the top right and bottom left point of the bounding box), a segmentation mask. At this time of writing, passing a text as input is not supported by the official model according to [the official repository](https://github.com/facebookresearch/segment-anything/issues/4#issuecomment-1497626844).
For more details, refer to this notebook, which shows a walk throught of how to use the model, with a visual example!
## Automatic-Mask-Generation
The model can be used for generating segmentation masks in a "zero-shot" fashion, given an input image. The model is automatically prompt with a grid of `1024` points
which are all fed to the model.
The pipeline is made for automatic mask generation. The following snippet demonstrates how easy you can run it (on any device! Simply feed the appropriate `points_per_batch` argument)
```python
from transformers import pipeline
generator = pipeline("mask-generation", device = 0, points_per_batch = 256)
image_url = "https://huggingface.co/ybelkada/segment-anything/resolve/main/assets/car.png"
outputs = generator(image_url, points_per_batch = 256)
```
Now to display the image:
```python
import matplotlib.pyplot as plt
from PIL import Image
import numpy as np
def show_mask(mask, ax, random_color=False):
if random_color:
color = np.concatenate([np.random.random(3), np.array([0.6])], axis=0)
else:
color = np.array([30 / 255, 144 / 255, 255 / 255, 0.6])
h, w = mask.shape[-2:]
mask_image = mask.reshape(h, w, 1) * color.reshape(1, 1, -1)
ax.imshow(mask_image)
plt.imshow(np.array(raw_image))
ax = plt.gca()
for mask in outputs["masks"]:
show_mask(mask, ax=ax, random_color=True)
plt.axis("off")
plt.show()
```
# Citation
If you use this model, please use the following BibTeX entry.
```
@article{kirillov2023segany,
title={Segment Anything},
author={Kirillov, Alexander and Mintun, Eric and Ravi, Nikhila and Mao, Hanzi and Rolland, Chloe and Gustafson, Laura and Xiao, Tete and Whitehead, Spencer and Berg, Alexander C. and Lo, Wan-Yen and Doll{\'a}r, Piotr and Girshick, Ross},
journal={arXiv:2304.02643},
year={2023}
}
``` |
facebook/rag-sequence-nq | facebook | "2021-03-12T11:04:28Z" | 96,224 | 27 | transformers | [
"transformers",
"pytorch",
"tf",
"rag",
"en",
"dataset:wiki_dpr",
"arxiv:2005.11401",
"license:apache-2.0",
"endpoints_compatible",
"region:us"
] | null | "2022-03-02T23:29:05Z" | ---
language: en
license: apache-2.0
datasets:
- wiki_dpr
thumbnail: https://huggingface.co/front/thumbnails/facebook.png
---
## RAG
This is the RAG-Sequence Model of the the paper [Retrieval-Augmented Generation for Knowledge-Intensive NLP Tasks](https://arxiv.org/pdf/2005.11401.pdf)
by Patrick Lewis, Ethan Perez, Aleksandara Piktus et al.
The model is a *uncased* model, which means that capital letters are simply converted to lower-case letters.
The model consits of a *question_encoder*, *retriever* and a *generator*. The retriever extracts relevant passages from the *wiki_dpr* `train` datasets, which is linked above.
The question_encoder and retriever are based on `facebook/dpr-question_encoder-single-nq-base` and `facebook/bart-large`, which were jointly finetuned on
on the *wiki_dpr* QA dataset in an end-to-end fashion.
## Usage:
**Note**: In the usage example below only the *dummy* retriever of *wiki_dpr* is used because the complete *lecagy* index requires over 75 GB of RAM.
The model can generate answers to any factoid question as follows:
```python
from transformers import RagTokenizer, RagRetriever, RagSequenceForGeneration
tokenizer = RagTokenizer.from_pretrained("facebook/rag-sequence-nq")
retriever = RagRetriever.from_pretrained("facebook/rag-sequence-nq", index_name="exact", use_dummy_dataset=True)
model = RagSequenceForGeneration.from_pretrained("facebook/rag-sequence-nq", retriever=retriever)
input_dict = tokenizer.prepare_seq2seq_batch("how many countries are in europe", return_tensors="pt")
generated = model.generate(input_ids=input_dict["input_ids"])
print(tokenizer.batch_decode(generated, skip_special_tokens=True)[0])
# should give 54 => google says either 44 or 51
```
|
RichardErkhov/TheBloke_-_Llama-2-70B-fp16-gguf | RichardErkhov | "2024-06-30T18:43:00Z" | 95,749 | 0 | null | [
"gguf",
"region:us"
] | null | "2024-06-29T21:17:01Z" | Quantization made by Richard Erkhov.
[Github](https://github.com/RichardErkhov)
[Discord](https://discord.gg/pvy7H8DZMG)
[Request more models](https://github.com/RichardErkhov/quant_request)
Llama-2-70B-fp16 - GGUF
- Model creator: https://huggingface.co/TheBloke/
- Original model: https://huggingface.co/TheBloke/Llama-2-70B-fp16/
| Name | Quant method | Size |
| ---- | ---- | ---- |
| [Llama-2-70B-fp16.Q2_K.gguf](https://huggingface.co/RichardErkhov/TheBloke_-_Llama-2-70B-fp16-gguf/blob/main/Llama-2-70B-fp16.Q2_K.gguf) | Q2_K | 23.71GB |
| [Llama-2-70B-fp16.IQ3_XS.gguf](https://huggingface.co/RichardErkhov/TheBloke_-_Llama-2-70B-fp16-gguf/blob/main/Llama-2-70B-fp16.IQ3_XS.gguf) | IQ3_XS | 26.37GB |
| [Llama-2-70B-fp16.IQ3_S.gguf](https://huggingface.co/RichardErkhov/TheBloke_-_Llama-2-70B-fp16-gguf/blob/main/Llama-2-70B-fp16.IQ3_S.gguf) | IQ3_S | 27.86GB |
| [Llama-2-70B-fp16.Q3_K_S.gguf](https://huggingface.co/RichardErkhov/TheBloke_-_Llama-2-70B-fp16-gguf/blob/main/Llama-2-70B-fp16.Q3_K_S.gguf) | Q3_K_S | 27.86GB |
| [Llama-2-70B-fp16.IQ3_M.gguf](https://huggingface.co/RichardErkhov/TheBloke_-_Llama-2-70B-fp16-gguf/blob/main/Llama-2-70B-fp16.IQ3_M.gguf) | IQ3_M | 28.82GB |
| [Llama-2-70B-fp16.Q3_K.gguf](https://huggingface.co/RichardErkhov/TheBloke_-_Llama-2-70B-fp16-gguf/blob/main/Llama-2-70B-fp16.Q3_K.gguf) | Q3_K | 30.99GB |
| [Llama-2-70B-fp16.Q3_K_M.gguf](https://huggingface.co/RichardErkhov/TheBloke_-_Llama-2-70B-fp16-gguf/blob/main/Llama-2-70B-fp16.Q3_K_M.gguf) | Q3_K_M | 30.99GB |
| [Llama-2-70B-fp16.Q3_K_L.gguf](https://huggingface.co/RichardErkhov/TheBloke_-_Llama-2-70B-fp16-gguf/blob/main/Llama-2-70B-fp16.Q3_K_L.gguf) | Q3_K_L | 33.67GB |
| [Llama-2-70B-fp16.IQ4_XS.gguf](https://huggingface.co/RichardErkhov/TheBloke_-_Llama-2-70B-fp16-gguf/blob/main/Llama-2-70B-fp16.IQ4_XS.gguf) | IQ4_XS | 34.64GB |
| [Llama-2-70B-fp16.Q4_0.gguf](https://huggingface.co/RichardErkhov/TheBloke_-_Llama-2-70B-fp16-gguf/blob/main/Llama-2-70B-fp16.Q4_0.gguf) | Q4_0 | 36.2GB |
| [Llama-2-70B-fp16.IQ4_NL.gguf](https://huggingface.co/RichardErkhov/TheBloke_-_Llama-2-70B-fp16-gguf/blob/main/Llama-2-70B-fp16.IQ4_NL.gguf) | IQ4_NL | 36.55GB |
| [Llama-2-70B-fp16.Q4_K_S.gguf](https://huggingface.co/RichardErkhov/TheBloke_-_Llama-2-70B-fp16-gguf/blob/main/Llama-2-70B-fp16.Q4_K_S.gguf) | Q4_K_S | 36.55GB |
| [Llama-2-70B-fp16.Q4_K.gguf](https://huggingface.co/RichardErkhov/TheBloke_-_Llama-2-70B-fp16-gguf/tree/main/) | Q4_K | 38.58GB |
| [Llama-2-70B-fp16.Q4_K_M.gguf](https://huggingface.co/RichardErkhov/TheBloke_-_Llama-2-70B-fp16-gguf/tree/main/) | Q4_K_M | 38.58GB |
| [Llama-2-70B-fp16.Q4_1.gguf](https://huggingface.co/RichardErkhov/TheBloke_-_Llama-2-70B-fp16-gguf/tree/main/) | Q4_1 | 40.2GB |
| [Llama-2-70B-fp16.Q5_0.gguf](https://huggingface.co/RichardErkhov/TheBloke_-_Llama-2-70B-fp16-gguf/tree/main/) | Q5_0 | 44.2GB |
| [Llama-2-70B-fp16.Q5_K_S.gguf](https://huggingface.co/RichardErkhov/TheBloke_-_Llama-2-70B-fp16-gguf/tree/main/) | Q5_K_S | 44.2GB |
| [Llama-2-70B-fp16.Q5_K.gguf](https://huggingface.co/RichardErkhov/TheBloke_-_Llama-2-70B-fp16-gguf/tree/main/) | Q5_K | 45.41GB |
| [Llama-2-70B-fp16.Q5_K_M.gguf](https://huggingface.co/RichardErkhov/TheBloke_-_Llama-2-70B-fp16-gguf/tree/main/) | Q5_K_M | 45.41GB |
| [Llama-2-70B-fp16.Q5_1.gguf](https://huggingface.co/RichardErkhov/TheBloke_-_Llama-2-70B-fp16-gguf/tree/main/) | Q5_1 | 48.2GB |
| [Llama-2-70B-fp16.Q6_K.gguf](https://huggingface.co/RichardErkhov/TheBloke_-_Llama-2-70B-fp16-gguf/tree/main/) | Q6_K | 52.7GB |
| [Llama-2-70B-fp16.Q8_0.gguf](https://huggingface.co/RichardErkhov/TheBloke_-_Llama-2-70B-fp16-gguf/tree/main/) | Q8_0 | 68.26GB |
Original model description:
---
inference: false
language:
- en
license: llama2
model_type: llama
pipeline_tag: text-generation
tags:
- facebook
- meta
- pytorch
- llama
- llama-2
---
<!-- header start -->
<div style="width: 100%;">
<img src="https://i.imgur.com/EBdldam.jpg" alt="TheBlokeAI" style="width: 100%; min-width: 400px; display: block; margin: auto;">
</div>
<div style="display: flex; justify-content: space-between; width: 100%;">
<div style="display: flex; flex-direction: column; align-items: flex-start;">
<p><a href="https://discord.gg/theblokeai">Chat & support: my new Discord server</a></p>
</div>
<div style="display: flex; flex-direction: column; align-items: flex-end;">
<p><a href="https://www.patreon.com/TheBlokeAI">Want to contribute? TheBloke's Patreon page</a></p>
</div>
</div>
<!-- header end -->
# Meta's Llama 2 70B fp16
These files are fp16 format model files for [Meta's Llama 2 70B](https://huggingface.co/meta-llama/Llama-2-70b-hf).
They were produced by downloading the PTH files from Meta, and then converting to HF format using the latest Transformers 4.32.0.dev0, from Git, with the Llama 2 PR included: https://github.com/huggingface/transformers/pull/24891.
Command to convert was:
```
python3 /workspace/venv/pytorch2/lib/python3.10/site-packages/transformers/models/llama/convert_llama_weights_to_hf.py --input_dir /workspace/git/llama/download --model_size 70B --output_dir /workspace/process/llama-2-70b-chat/source --safe_serialization true
```
The files were saved in Safetensors format.
I am uploading this repo because I initially tried to create GPTQs using the [MetaLlama 2 70B HF repo](https://huggingface.co/meta-llama/Llama-2-70b-hf), but got strange errors that suggested the weights were not correct. But converting from the PTH files using the latest `convert_llama_weights_to_hf.py` script worked fine.
Many thanks to William Beauchamp from [Chai](https://chai-research.com/) for providing the hardware for merging and uploading these files!
## Repositories available
* [GPTQ models for GPU inference, with multiple quantisation parameter options.](https://huggingface.co/TheBloke/Llama-2-70B-GPTQ)
* [Original unquantised fp16 model in pytorch format, for GPU inference and for further conversions](https://huggingface.co/meta-llama/Llama-2-70b-hf)
* [My fp16 conversion of the unquantised PTH model files](https://huggingface.co/TheBloke/Llama-2-70B-fp16)
## Prompt template: None
```
{prompt}
```
<!-- footer start -->
## Discord
For further support, and discussions on these models and AI in general, join us at:
[TheBloke AI's Discord server](https://discord.gg/theblokeai)
## Thanks, and how to contribute.
Thanks to the [chirper.ai](https://chirper.ai) team!
I've had a lot of people ask if they can contribute. I enjoy providing models and helping people, and would love to be able to spend even more time doing it, as well as expanding into new projects like fine tuning/training.
If you're able and willing to contribute it will be most gratefully received and will help me to keep providing more models, and to start work on new AI projects.
Donaters will get priority support on any and all AI/LLM/model questions and requests, access to a private Discord room, plus other benefits.
* Patreon: https://patreon.com/TheBlokeAI
* Ko-Fi: https://ko-fi.com/TheBlokeAI
**Special thanks to**: Luke from CarbonQuill, Aemon Algiz.
**Patreon special mentions**: Space Cruiser, Nikolai Manek, Sam, Chris McCloskey, Rishabh Srivastava, Kalila, Spiking Neurons AB, Khalefa Al-Ahmad, WelcomeToTheClub, Chadd, Lone Striker, Viktor Bowallius, Edmond Seymore, Ai Maven, Chris Smitley, Dave, Alexandros Triantafyllidis, Luke @flexchar, Elle, ya boyyy, Talal Aujan, Alex , Jonathan Leane, Deep Realms, Randy H, subjectnull, Preetika Verma, Joseph William Delisle, Michael Levine, chris gileta, K, Oscar Rangel, LangChain4j, Trenton Dambrowitz, Eugene Pentland, Johann-Peter Hartmann, Femi Adebogun, Illia Dulskyi, senxiiz, Daniel P. Andersen, Sean Connelly, Artur Olbinski, RoA, Mano Prime, Derek Yates, Raven Klaugh, David Flickinger, Willem Michiel, Pieter, Willian Hasse, vamX, Luke Pendergrass, webtim, Ghost , Rainer Wilmers, Nathan LeClaire, Will Dee, Cory Kujawski, John Detwiler, Fred von Graf, biorpg, Iucharbius , Imad Khwaja, Pierre Kircher, terasurfer , Asp the Wyvern, John Villwock, theTransient, zynix , Gabriel Tamborski, Fen Risland, Gabriel Puliatti, Matthew Berman, Pyrater, SuperWojo, Stephen Murray, Karl Bernard, Ajan Kanaga, Greatston Gnanesh, Junyu Yang.
Thank you to all my generous patrons and donaters!
<!-- footer end -->
# Original model card: Meta's Llama 2 70B
# **Llama 2**
Llama 2 is a collection of pretrained and fine-tuned generative text models ranging in scale from 7 billion to 70 billion parameters. This is the repository for the 70B pretrained model, converted for the Hugging Face Transformers format. Links to other models can be found in the index at the bottom.
## Model Details
*Note: Use of this model is governed by the Meta license. In order to download the model weights and tokenizer, please visit the [website](https://ai.meta.com/resources/models-and-libraries/llama-downloads/) and accept our License before requesting access here.*
Meta developed and publicly released the Llama 2 family of large language models (LLMs), a collection of pretrained and fine-tuned generative text models ranging in scale from 7 billion to 70 billion parameters. Our fine-tuned LLMs, called Llama-2-Chat, are optimized for dialogue use cases. Llama-2-Chat models outperform open-source chat models on most benchmarks we tested, and in our human evaluations for helpfulness and safety, are on par with some popular closed-source models like ChatGPT and PaLM.
**Model Developers** Meta
**Variations** Llama 2 comes in a range of parameter sizes — 7B, 13B, and 70B — as well as pretrained and fine-tuned variations.
**Input** Models input text only.
**Output** Models generate text only.
**Model Architecture** Llama 2 is an auto-regressive language model that uses an optimized transformer architecture. The tuned versions use supervised fine-tuning (SFT) and reinforcement learning with human feedback (RLHF) to align to human preferences for helpfulness and safety.
||Training Data|Params|Content Length|GQA|Tokens|LR|
|---|---|---|---|---|---|---|
|Llama 2|*A new mix of publicly available online data*|7B|4k|✗|2.0T|3.0 x 10<sup>-4</sup>|
|Llama 2|*A new mix of publicly available online data*|13B|4k|✗|2.0T|3.0 x 10<sup>-4</sup>|
|Llama 2|*A new mix of publicly available online data*|70B|4k|✔|2.0T|1.5 x 10<sup>-4</sup>|
*Llama 2 family of models.* Token counts refer to pretraining data only. All models are trained with a global batch-size of 4M tokens. Bigger models - 70B -- use Grouped-Query Attention (GQA) for improved inference scalability.
**Model Dates** Llama 2 was trained between January 2023 and July 2023.
**Status** This is a static model trained on an offline dataset. Future versions of the tuned models will be released as we improve model safety with community feedback.
**License** A custom commercial license is available at: [https://ai.meta.com/resources/models-and-libraries/llama-downloads/](https://ai.meta.com/resources/models-and-libraries/llama-downloads/)
## Intended Use
**Intended Use Cases** Llama 2 is intended for commercial and research use in English. Tuned models are intended for assistant-like chat, whereas pretrained models can be adapted for a variety of natural language generation tasks.
To get the expected features and performance for the chat versions, a specific formatting needs to be followed, including the `INST` and `<<SYS>>` tags, `BOS` and `EOS` tokens, and the whitespaces and breaklines in between (we recommend calling `strip()` on inputs to avoid double-spaces). See our reference code in github for details: [`chat_completion`](https://github.com/facebookresearch/llama/blob/main/llama/generation.py#L212).
**Out-of-scope Uses** Use in any manner that violates applicable laws or regulations (including trade compliance laws).Use in languages other than English. Use in any other way that is prohibited by the Acceptable Use Policy and Licensing Agreement for Llama 2.
## Hardware and Software
**Training Factors** We used custom training libraries, Meta's Research Super Cluster, and production clusters for pretraining. Fine-tuning, annotation, and evaluation were also performed on third-party cloud compute.
**Carbon Footprint** Pretraining utilized a cumulative 3.3M GPU hours of computation on hardware of type A100-80GB (TDP of 350-400W). Estimated total emissions were 539 tCO2eq, 100% of which were offset by Meta’s sustainability program.
||Time (GPU hours)|Power Consumption (W)|Carbon Emitted(tCO<sub>2</sub>eq)|
|---|---|---|---|
|Llama 2 7B|184320|400|31.22|
|Llama 2 13B|368640|400|62.44|
|Llama 2 70B|1720320|400|291.42|
|Total|3311616||539.00|
**CO<sub>2</sub> emissions during pretraining.** Time: total GPU time required for training each model. Power Consumption: peak power capacity per GPU device for the GPUs used adjusted for power usage efficiency. 100% of the emissions are directly offset by Meta's sustainability program, and because we are openly releasing these models, the pretraining costs do not need to be incurred by others.
## Training Data
**Overview** Llama 2 was pretrained on 2 trillion tokens of data from publicly available sources. The fine-tuning data includes publicly available instruction datasets, as well as over one million new human-annotated examples. Neither the pretraining nor the fine-tuning datasets include Meta user data.
**Data Freshness** The pretraining data has a cutoff of September 2022, but some tuning data is more recent, up to July 2023.
## Evaluation Results
In this section, we report the results for the Llama 1 and Llama 2 models on standard academic benchmarks.For all the evaluations, we use our internal evaluations library.
|Model|Size|Code|Commonsense Reasoning|World Knowledge|Reading Comprehension|Math|MMLU|BBH|AGI Eval|
|---|---|---|---|---|---|---|---|---|---|
|Llama 1|7B|14.1|60.8|46.2|58.5|6.95|35.1|30.3|23.9|
|Llama 1|13B|18.9|66.1|52.6|62.3|10.9|46.9|37.0|33.9|
|Llama 1|33B|26.0|70.0|58.4|67.6|21.4|57.8|39.8|41.7|
|Llama 1|65B|30.7|70.7|60.5|68.6|30.8|63.4|43.5|47.6|
|Llama 2|7B|16.8|63.9|48.9|61.3|14.6|45.3|32.6|29.3|
|Llama 2|13B|24.5|66.9|55.4|65.8|28.7|54.8|39.4|39.1|
|Llama 2|70B|**37.5**|**71.9**|**63.6**|**69.4**|**35.2**|**68.9**|**51.2**|**54.2**|
**Overall performance on grouped academic benchmarks.** *Code:* We report the average pass@1 scores of our models on HumanEval and MBPP. *Commonsense Reasoning:* We report the average of PIQA, SIQA, HellaSwag, WinoGrande, ARC easy and challenge, OpenBookQA, and CommonsenseQA. We report 7-shot results for CommonSenseQA and 0-shot results for all other benchmarks. *World Knowledge:* We evaluate the 5-shot performance on NaturalQuestions and TriviaQA and report the average. *Reading Comprehension:* For reading comprehension, we report the 0-shot average on SQuAD, QuAC, and BoolQ. *MATH:* We report the average of the GSM8K (8 shot) and MATH (4 shot) benchmarks at top 1.
|||TruthfulQA|Toxigen|
|---|---|---|---|
|Llama 1|7B|27.42|23.00|
|Llama 1|13B|41.74|23.08|
|Llama 1|33B|44.19|22.57|
|Llama 1|65B|48.71|21.77|
|Llama 2|7B|33.29|**21.25**|
|Llama 2|13B|41.86|26.10|
|Llama 2|70B|**50.18**|24.60|
**Evaluation of pretrained LLMs on automatic safety benchmarks.** For TruthfulQA, we present the percentage of generations that are both truthful and informative (the higher the better). For ToxiGen, we present the percentage of toxic generations (the smaller the better).
|||TruthfulQA|Toxigen|
|---|---|---|---|
|Llama-2-Chat|7B|57.04|**0.00**|
|Llama-2-Chat|13B|62.18|**0.00**|
|Llama-2-Chat|70B|**64.14**|0.01|
**Evaluation of fine-tuned LLMs on different safety datasets.** Same metric definitions as above.
## Ethical Considerations and Limitations
Llama 2 is a new technology that carries risks with use. Testing conducted to date has been in English, and has not covered, nor could it cover all scenarios. For these reasons, as with all LLMs, Llama 2’s potential outputs cannot be predicted in advance, and the model may in some instances produce inaccurate, biased or other objectionable responses to user prompts. Therefore, before deploying any applications of Llama 2, developers should perform safety testing and tuning tailored to their specific applications of the model.
Please see the Responsible Use Guide available at [https://ai.meta.com/llama/responsible-use-guide/](https://ai.meta.com/llama/responsible-use-guide)
## Reporting Issues
Please report any software “bug,” or other problems with the models through one of the following means:
- Reporting issues with the model: [github.com/facebookresearch/llama](http://github.com/facebookresearch/llama)
- Reporting problematic content generated by the model: [developers.facebook.com/llama_output_feedback](http://developers.facebook.com/llama_output_feedback)
- Reporting bugs and security concerns: [facebook.com/whitehat/info](http://facebook.com/whitehat/info)
## Llama Model Index
|Model|Llama2|Llama2-hf|Llama2-chat|Llama2-chat-hf|
|---|---|---|---|---|
|7B| [Link](https://huggingface.co/llamaste/Llama-2-7b) | [Link](https://huggingface.co/llamaste/Llama-2-7b-hf) | [Link](https://huggingface.co/llamaste/Llama-2-7b-chat) | [Link](https://huggingface.co/llamaste/Llama-2-7b-chat-hf)|
|13B| [Link](https://huggingface.co/llamaste/Llama-2-13b) | [Link](https://huggingface.co/llamaste/Llama-2-13b-hf) | [Link](https://huggingface.co/llamaste/Llama-2-13b-chat) | [Link](https://huggingface.co/llamaste/Llama-2-13b-hf)|
|70B| [Link](https://huggingface.co/llamaste/Llama-2-70b) | [Link](https://huggingface.co/llamaste/Llama-2-70b-hf) | [Link](https://huggingface.co/llamaste/Llama-2-70b-chat) | [Link](https://huggingface.co/llamaste/Llama-2-70b-hf)|
|
facebook/wav2vec2-xlsr-53-espeak-cv-ft | facebook | "2021-12-10T17:18:39Z" | 95,711 | 20 | transformers | [
"transformers",
"pytorch",
"wav2vec2",
"automatic-speech-recognition",
"speech",
"audio",
"phoneme-recognition",
"dataset:common_voice",
"arxiv:2109.11680",
"license:apache-2.0",
"endpoints_compatible",
"region:us"
] | automatic-speech-recognition | "2022-03-02T23:29:05Z" | ---
language: multi-lingual
datasets:
- common_voice
tags:
- speech
- audio
- automatic-speech-recognition
- phoneme-recognition
widget:
- example_title: Librispeech sample 1
src: https://cdn-media.huggingface.co/speech_samples/sample1.flac
- example_title: Librispeech sample 2
src: https://cdn-media.huggingface.co/speech_samples/sample2.flac
license: apache-2.0
---
# Wav2Vec2-Large-XLSR-53 finetuned on multi-lingual Common Voice
This checkpoint leverages the pretrained checkpoint [wav2vec2-large-xlsr-53](https://huggingface.co/facebook/wav2vec2-large-xlsr-53)
and is fine-tuned on [CommonVoice](https://huggingface.co/datasets/common_voice) to recognize phonetic labels in multiple languages.
When using the model make sure that your speech input is sampled at 16kHz.
Note that the model outputs a string of phonetic labels. A dictionary mapping phonetic labels to words
has to be used to map the phonetic output labels to output words.
[Paper: Simple and Effective Zero-shot Cross-lingual Phoneme Recognition](https://arxiv.org/abs/2109.11680)
Authors: Qiantong Xu, Alexei Baevski, Michael Auli
**Abstract**
Recent progress in self-training, self-supervised pretraining and unsupervised learning enabled well performing speech recognition systems without any labeled data. However, in many cases there is labeled data available for related languages which is not utilized by these methods. This paper extends previous work on zero-shot cross-lingual transfer learning by fine-tuning a multilingually pretrained wav2vec 2.0 model to transcribe unseen languages. This is done by mapping phonemes of the training languages to the target language using articulatory features. Experiments show that this simple method significantly outperforms prior work which introduced task-specific architectures and used only part of a monolingually pretrained model.
The original model can be found under https://github.com/pytorch/fairseq/tree/master/examples/wav2vec#wav2vec-20.
# Usage
To transcribe audio files the model can be used as a standalone acoustic model as follows:
```python
from transformers import Wav2Vec2Processor, Wav2Vec2ForCTC
from datasets import load_dataset
import torch
# load model and processor
processor = Wav2Vec2Processor.from_pretrained("facebook/wav2vec2-xlsr-53-espeak-cv-ft")
model = Wav2Vec2ForCTC.from_pretrained("facebook/wav2vec2-xlsr-53-espeak-cv-ft")
# load dummy dataset and read soundfiles
ds = load_dataset("patrickvonplaten/librispeech_asr_dummy", "clean", split="validation")
# tokenize
input_values = processor(ds[0]["audio"]["array"], return_tensors="pt").input_values
# retrieve logits
with torch.no_grad():
logits = model(input_values).logits
# take argmax and decode
predicted_ids = torch.argmax(logits, dim=-1)
transcription = processor.batch_decode(predicted_ids)
# => should give ['m ɪ s t ɚ k w ɪ l t ɚ ɪ z ð ɪ ɐ p ɑː s əl l ʌ v ð ə m ɪ d əl k l æ s ɪ z æ n d w iː aʊ ɡ l æ d t ə w ɛ l k ə m h ɪ z ɡ ɑː s p ə']
``` |
Qwen/Qwen2-72B-Instruct | Qwen | "2024-06-06T14:40:05Z" | 95,683 | 466 | transformers | [
"transformers",
"safetensors",
"qwen2",
"text-generation",
"chat",
"conversational",
"en",
"arxiv:2309.00071",
"license:other",
"autotrain_compatible",
"endpoints_compatible",
"text-generation-inference",
"region:us"
] | text-generation | "2024-05-28T03:48:49Z" | ---
license: other
license_name: tongyi-qianwen
license_link: https://huggingface.co/Qwen/Qwen2-72B-Instruct/blob/main/LICENSE
language:
- en
pipeline_tag: text-generation
tags:
- chat
---
# Qwen2-72B-Instruct
## Introduction
Qwen2 is the new series of Qwen large language models. For Qwen2, we release a number of base language models and instruction-tuned language models ranging from 0.5 to 72 billion parameters, including a Mixture-of-Experts model. This repo contains the instruction-tuned 72B Qwen2 model.
Compared with the state-of-the-art opensource language models, including the previous released Qwen1.5, Qwen2 has generally surpassed most opensource models and demonstrated competitiveness against proprietary models across a series of benchmarks targeting for language understanding, language generation, multilingual capability, coding, mathematics, reasoning, etc.
Qwen2-72B-Instruct supports a context length of up to 131,072 tokens, enabling the processing of extensive inputs. Please refer to [this section](#processing-long-texts) for detailed instructions on how to deploy Qwen2 for handling long texts.
For more details, please refer to our [blog](https://qwenlm.github.io/blog/qwen2/), [GitHub](https://github.com/QwenLM/Qwen2), and [Documentation](https://qwen.readthedocs.io/en/latest/).
<br>
## Model Details
Qwen2 is a language model series including decoder language models of different model sizes. For each size, we release the base language model and the aligned chat model. It is based on the Transformer architecture with SwiGLU activation, attention QKV bias, group query attention, etc. Additionally, we have an improved tokenizer adaptive to multiple natural languages and codes.
## Training details
We pretrained the models with a large amount of data, and we post-trained the models with both supervised finetuning and direct preference optimization.
## Requirements
The code of Qwen2 has been in the latest Hugging face transformers and we advise you to install `transformers>=4.37.0`, or you might encounter the following error:
```
KeyError: 'qwen2'
```
## Quickstart
Here provides a code snippet with `apply_chat_template` to show you how to load the tokenizer and model and how to generate contents.
```python
from transformers import AutoModelForCausalLM, AutoTokenizer
device = "cuda" # the device to load the model onto
model = AutoModelForCausalLM.from_pretrained(
"Qwen/Qwen2-72B-Instruct",
torch_dtype="auto",
device_map="auto"
)
tokenizer = AutoTokenizer.from_pretrained("Qwen/Qwen2-72B-Instruct")
prompt = "Give me a short introduction to large language model."
messages = [
{"role": "system", "content": "You are a helpful assistant."},
{"role": "user", "content": prompt}
]
text = tokenizer.apply_chat_template(
messages,
tokenize=False,
add_generation_prompt=True
)
model_inputs = tokenizer([text], return_tensors="pt").to(device)
generated_ids = model.generate(
model_inputs.input_ids,
max_new_tokens=512
)
generated_ids = [
output_ids[len(input_ids):] for input_ids, output_ids in zip(model_inputs.input_ids, generated_ids)
]
response = tokenizer.batch_decode(generated_ids, skip_special_tokens=True)[0]
```
### Processing Long Texts
To handle extensive inputs exceeding 32,768 tokens, we utilize [YARN](https://arxiv.org/abs/2309.00071), a technique for enhancing model length extrapolation, ensuring optimal performance on lengthy texts.
For deployment, we recommend using vLLM. You can enable the long-context capabilities by following these steps:
1. **Install vLLM**: You can install vLLM by running the following command.
```bash
pip install "vllm>=0.4.3"
```
Or you can install vLLM from [source](https://github.com/vllm-project/vllm/).
2. **Configure Model Settings**: After downloading the model weights, modify the `config.json` file by including the below snippet:
```json
{
"architectures": [
"Qwen2ForCausalLM"
],
// ...
"vocab_size": 152064,
// adding the following snippets
"rope_scaling": {
"factor": 4.0,
"original_max_position_embeddings": 32768,
"type": "yarn"
}
}
```
This snippet enable YARN to support longer contexts.
3. **Model Deployment**: Utilize vLLM to deploy your model. For instance, you can set up an openAI-like server using the command:
```bash
python -m vllm.entrypoints.openai.api_server --served-model-name Qwen2-72B-Instruct --model path/to/weights
```
Then you can access the Chat API by:
```bash
curl http://localhost:8000/v1/chat/completions \
-H "Content-Type: application/json" \
-d '{
"model": "Qwen2-72B-Instruct",
"messages": [
{"role": "system", "content": "You are a helpful assistant."},
{"role": "user", "content": "Your Long Input Here."}
]
}'
```
For further usage instructions of vLLM, please refer to our [Github](https://github.com/QwenLM/Qwen2).
**Note**: Presently, vLLM only supports static YARN, which means the scaling factor remains constant regardless of input length, **potentially impacting performance on shorter texts**. We advise adding the `rope_scaling` configuration only when processing long contexts is required.
## Evaluation
We briefly compare Qwen2-72B-Instruct with similar-sized instruction-tuned LLMs, including our previous Qwen1.5-72B-Chat. The results are shown as follows:
| Datasets | Llama-3-70B-Instruct | Qwen1.5-72B-Chat | **Qwen2-72B-Instruct** |
| :--- | :---: | :---: | :---: |
| _**English**_ | | | |
| MMLU | 82.0 | 75.6 | **82.3** |
| MMLU-Pro | 56.2 | 51.7 | **64.4** |
| GPQA | 41.9 | 39.4 | **42.4** |
| TheroemQA | 42.5 | 28.8 | **44.4** |
| MT-Bench | 8.95 | 8.61 | **9.12** |
| Arena-Hard | 41.1 | 36.1 | **48.1** |
| IFEval (Prompt Strict-Acc.) | 77.3 | 55.8 | **77.6** |
| _**Coding**_ | | | |
| HumanEval | 81.7 | 71.3 | **86.0** |
| MBPP | **82.3** | 71.9 | 80.2 |
| MultiPL-E | 63.4 | 48.1 | **69.2** |
| EvalPlus | 75.2 | 66.9 | **79.0** |
| LiveCodeBench | 29.3 | 17.9 | **35.7** |
| _**Mathematics**_ | | | |
| GSM8K | **93.0** | 82.7 | 91.1 |
| MATH | 50.4 | 42.5 | **59.7** |
| _**Chinese**_ | | | |
| C-Eval | 61.6 | 76.1 | **83.8** |
| AlignBench | 7.42 | 7.28 | **8.27** |
## Citation
If you find our work helpful, feel free to give us a cite.
```
@article{qwen2,
title={Qwen2 Technical Report},
year={2024}
}
``` |
mohitsha/tiny-random-testing-bert2gpt2 | mohitsha | "2023-09-01T12:59:38Z" | 95,664 | 1 | transformers | [
"transformers",
"pytorch",
"encoder-decoder",
"text2text-generation",
"autotrain_compatible",
"endpoints_compatible",
"region:us"
] | text2text-generation | "2023-09-01T12:56:21Z" | Entry not found |
mradermacher/Hermes-2-Theta-Llama-3-70B-32k-i1-GGUF | mradermacher | "2024-06-22T06:19:18Z" | 95,211 | 0 | transformers | [
"transformers",
"gguf",
"distillation",
"synthetic data",
"function calling",
"structured outputs",
"json mode",
"en",
"base_model:OpenPipe/Hermes-2-Theta-Llama-3-70B-32k",
"license:llama3",
"endpoints_compatible",
"region:us"
] | null | "2024-06-21T18:16:39Z" | ---
base_model: OpenPipe/Hermes-2-Theta-Llama-3-70B-32k
language:
- en
library_name: transformers
license: llama3
quantized_by: mradermacher
tags:
- distillation
- synthetic data
- function calling
- structured outputs
- json mode
---
## About
<!-- ### quantize_version: 2 -->
<!-- ### output_tensor_quantised: 1 -->
<!-- ### convert_type: hf -->
<!-- ### vocab_type: -->
<!-- ### tags: nicoboss -->
weighted/imatrix quants of https://huggingface.co/OpenPipe/Hermes-2-Theta-Llama-3-70B-32k
<!-- provided-files -->
static quants are available at https://huggingface.co/mradermacher/Hermes-2-Theta-Llama-3-70B-32k-GGUF
## Usage
If you are unsure how to use GGUF files, refer to one of [TheBloke's
READMEs](https://huggingface.co/TheBloke/KafkaLM-70B-German-V0.1-GGUF) for
more details, including on how to concatenate multi-part files.
## Provided Quants
(sorted by size, not necessarily quality. IQ-quants are often preferable over similar sized non-IQ quants)
| Link | Type | Size/GB | Notes |
|:-----|:-----|--------:|:------|
| [GGUF](https://huggingface.co/mradermacher/Hermes-2-Theta-Llama-3-70B-32k-i1-GGUF/resolve/main/Hermes-2-Theta-Llama-3-70B-32k.i1-IQ1_S.gguf) | i1-IQ1_S | 15.4 | for the desperate |
| [GGUF](https://huggingface.co/mradermacher/Hermes-2-Theta-Llama-3-70B-32k-i1-GGUF/resolve/main/Hermes-2-Theta-Llama-3-70B-32k.i1-IQ1_M.gguf) | i1-IQ1_M | 16.9 | mostly desperate |
| [GGUF](https://huggingface.co/mradermacher/Hermes-2-Theta-Llama-3-70B-32k-i1-GGUF/resolve/main/Hermes-2-Theta-Llama-3-70B-32k.i1-IQ2_XXS.gguf) | i1-IQ2_XXS | 19.2 | |
| [GGUF](https://huggingface.co/mradermacher/Hermes-2-Theta-Llama-3-70B-32k-i1-GGUF/resolve/main/Hermes-2-Theta-Llama-3-70B-32k.i1-IQ2_XS.gguf) | i1-IQ2_XS | 21.2 | |
| [GGUF](https://huggingface.co/mradermacher/Hermes-2-Theta-Llama-3-70B-32k-i1-GGUF/resolve/main/Hermes-2-Theta-Llama-3-70B-32k.i1-IQ2_S.gguf) | i1-IQ2_S | 22.3 | |
| [GGUF](https://huggingface.co/mradermacher/Hermes-2-Theta-Llama-3-70B-32k-i1-GGUF/resolve/main/Hermes-2-Theta-Llama-3-70B-32k.i1-IQ2_M.gguf) | i1-IQ2_M | 24.2 | |
| [GGUF](https://huggingface.co/mradermacher/Hermes-2-Theta-Llama-3-70B-32k-i1-GGUF/resolve/main/Hermes-2-Theta-Llama-3-70B-32k.i1-Q2_K.gguf) | i1-Q2_K | 26.5 | IQ3_XXS probably better |
| [GGUF](https://huggingface.co/mradermacher/Hermes-2-Theta-Llama-3-70B-32k-i1-GGUF/resolve/main/Hermes-2-Theta-Llama-3-70B-32k.i1-IQ3_XXS.gguf) | i1-IQ3_XXS | 27.6 | lower quality |
| [GGUF](https://huggingface.co/mradermacher/Hermes-2-Theta-Llama-3-70B-32k-i1-GGUF/resolve/main/Hermes-2-Theta-Llama-3-70B-32k.i1-IQ3_XS.gguf) | i1-IQ3_XS | 29.4 | |
| [GGUF](https://huggingface.co/mradermacher/Hermes-2-Theta-Llama-3-70B-32k-i1-GGUF/resolve/main/Hermes-2-Theta-Llama-3-70B-32k.i1-IQ3_S.gguf) | i1-IQ3_S | 31.0 | beats Q3_K* |
| [GGUF](https://huggingface.co/mradermacher/Hermes-2-Theta-Llama-3-70B-32k-i1-GGUF/resolve/main/Hermes-2-Theta-Llama-3-70B-32k.i1-Q3_K_S.gguf) | i1-Q3_K_S | 31.0 | IQ3_XS probably better |
| [GGUF](https://huggingface.co/mradermacher/Hermes-2-Theta-Llama-3-70B-32k-i1-GGUF/resolve/main/Hermes-2-Theta-Llama-3-70B-32k.i1-IQ3_M.gguf) | i1-IQ3_M | 32.0 | |
| [GGUF](https://huggingface.co/mradermacher/Hermes-2-Theta-Llama-3-70B-32k-i1-GGUF/resolve/main/Hermes-2-Theta-Llama-3-70B-32k.i1-Q3_K_M.gguf) | i1-Q3_K_M | 34.4 | IQ3_S probably better |
| [GGUF](https://huggingface.co/mradermacher/Hermes-2-Theta-Llama-3-70B-32k-i1-GGUF/resolve/main/Hermes-2-Theta-Llama-3-70B-32k.i1-Q3_K_L.gguf) | i1-Q3_K_L | 37.2 | IQ3_M probably better |
| [GGUF](https://huggingface.co/mradermacher/Hermes-2-Theta-Llama-3-70B-32k-i1-GGUF/resolve/main/Hermes-2-Theta-Llama-3-70B-32k.i1-IQ4_XS.gguf) | i1-IQ4_XS | 38.0 | |
| [GGUF](https://huggingface.co/mradermacher/Hermes-2-Theta-Llama-3-70B-32k-i1-GGUF/resolve/main/Hermes-2-Theta-Llama-3-70B-32k.i1-Q4_0.gguf) | i1-Q4_0 | 40.2 | fast, low quality |
| [GGUF](https://huggingface.co/mradermacher/Hermes-2-Theta-Llama-3-70B-32k-i1-GGUF/resolve/main/Hermes-2-Theta-Llama-3-70B-32k.i1-Q4_K_S.gguf) | i1-Q4_K_S | 40.4 | optimal size/speed/quality |
| [GGUF](https://huggingface.co/mradermacher/Hermes-2-Theta-Llama-3-70B-32k-i1-GGUF/resolve/main/Hermes-2-Theta-Llama-3-70B-32k.i1-Q4_K_M.gguf) | i1-Q4_K_M | 42.6 | fast, recommended |
| [GGUF](https://huggingface.co/mradermacher/Hermes-2-Theta-Llama-3-70B-32k-i1-GGUF/resolve/main/Hermes-2-Theta-Llama-3-70B-32k.i1-Q5_K_S.gguf) | i1-Q5_K_S | 48.8 | |
| [GGUF](https://huggingface.co/mradermacher/Hermes-2-Theta-Llama-3-70B-32k-i1-GGUF/resolve/main/Hermes-2-Theta-Llama-3-70B-32k.i1-Q5_K_M.gguf) | i1-Q5_K_M | 50.0 | |
| [PART 1](https://huggingface.co/mradermacher/Hermes-2-Theta-Llama-3-70B-32k-i1-GGUF/resolve/main/Hermes-2-Theta-Llama-3-70B-32k.i1-Q6_K.gguf.part1of2) [PART 2](https://huggingface.co/mradermacher/Hermes-2-Theta-Llama-3-70B-32k-i1-GGUF/resolve/main/Hermes-2-Theta-Llama-3-70B-32k.i1-Q6_K.gguf.part2of2) | i1-Q6_K | 58.0 | practically like static Q6_K |
Here is a handy graph by ikawrakow comparing some lower-quality quant
types (lower is better):

And here are Artefact2's thoughts on the matter:
https://gist.github.com/Artefact2/b5f810600771265fc1e39442288e8ec9
## FAQ / Model Request
See https://huggingface.co/mradermacher/model_requests for some answers to
questions you might have and/or if you want some other model quantized.
## Thanks
I thank my company, [nethype GmbH](https://www.nethype.de/), for letting
me use its servers and providing upgrades to my workstation to enable
this work in my free time. Additional thanks to [@nicoboss](https://huggingface.co/nicoboss) for giving me access to his hardware for calculating the imatrix for these quants.
<!-- end -->
|
mradermacher/Swallow-70b-NVE-hf-i1-GGUF | mradermacher | "2024-06-30T22:09:24Z" | 95,199 | 0 | transformers | [
"transformers",
"gguf",
"en",
"ja",
"base_model:tokyotech-llm/Swallow-70b-NVE-hf",
"license:llama2",
"endpoints_compatible",
"region:us"
] | null | "2024-06-30T10:22:13Z" | ---
base_model: tokyotech-llm/Swallow-70b-NVE-hf
language:
- en
- ja
library_name: transformers
license: llama2
model_type: llama
quantized_by: mradermacher
---
## About
<!-- ### quantize_version: 2 -->
<!-- ### output_tensor_quantised: 1 -->
<!-- ### convert_type: hf -->
<!-- ### vocab_type: -->
<!-- ### tags: nicoboss -->
weighted/imatrix quants of https://huggingface.co/tokyotech-llm/Swallow-70b-NVE-hf
<!-- provided-files -->
static quants are available at https://huggingface.co/mradermacher/Swallow-70b-NVE-hf-GGUF
## Usage
If you are unsure how to use GGUF files, refer to one of [TheBloke's
READMEs](https://huggingface.co/TheBloke/KafkaLM-70B-German-V0.1-GGUF) for
more details, including on how to concatenate multi-part files.
## Provided Quants
(sorted by size, not necessarily quality. IQ-quants are often preferable over similar sized non-IQ quants)
| Link | Type | Size/GB | Notes |
|:-----|:-----|--------:|:------|
| [GGUF](https://huggingface.co/mradermacher/Swallow-70b-NVE-hf-i1-GGUF/resolve/main/Swallow-70b-NVE-hf.i1-IQ1_S.gguf) | i1-IQ1_S | 14.6 | for the desperate |
| [GGUF](https://huggingface.co/mradermacher/Swallow-70b-NVE-hf-i1-GGUF/resolve/main/Swallow-70b-NVE-hf.i1-IQ1_M.gguf) | i1-IQ1_M | 16.0 | mostly desperate |
| [GGUF](https://huggingface.co/mradermacher/Swallow-70b-NVE-hf-i1-GGUF/resolve/main/Swallow-70b-NVE-hf.i1-IQ2_XXS.gguf) | i1-IQ2_XXS | 18.4 | |
| [GGUF](https://huggingface.co/mradermacher/Swallow-70b-NVE-hf-i1-GGUF/resolve/main/Swallow-70b-NVE-hf.i1-IQ2_XS.gguf) | i1-IQ2_XS | 20.4 | |
| [GGUF](https://huggingface.co/mradermacher/Swallow-70b-NVE-hf-i1-GGUF/resolve/main/Swallow-70b-NVE-hf.i1-IQ2_S.gguf) | i1-IQ2_S | 21.5 | |
| [GGUF](https://huggingface.co/mradermacher/Swallow-70b-NVE-hf-i1-GGUF/resolve/main/Swallow-70b-NVE-hf.i1-IQ2_M.gguf) | i1-IQ2_M | 23.3 | |
| [GGUF](https://huggingface.co/mradermacher/Swallow-70b-NVE-hf-i1-GGUF/resolve/main/Swallow-70b-NVE-hf.i1-Q2_K.gguf) | i1-Q2_K | 25.6 | IQ3_XXS probably better |
| [GGUF](https://huggingface.co/mradermacher/Swallow-70b-NVE-hf-i1-GGUF/resolve/main/Swallow-70b-NVE-hf.i1-IQ3_XXS.gguf) | i1-IQ3_XXS | 26.7 | lower quality |
| [GGUF](https://huggingface.co/mradermacher/Swallow-70b-NVE-hf-i1-GGUF/resolve/main/Swallow-70b-NVE-hf.i1-IQ3_XS.gguf) | i1-IQ3_XS | 28.4 | |
| [GGUF](https://huggingface.co/mradermacher/Swallow-70b-NVE-hf-i1-GGUF/resolve/main/Swallow-70b-NVE-hf.i1-IQ3_S.gguf) | i1-IQ3_S | 30.0 | beats Q3_K* |
| [GGUF](https://huggingface.co/mradermacher/Swallow-70b-NVE-hf-i1-GGUF/resolve/main/Swallow-70b-NVE-hf.i1-Q3_K_S.gguf) | i1-Q3_K_S | 30.0 | IQ3_XS probably better |
| [GGUF](https://huggingface.co/mradermacher/Swallow-70b-NVE-hf-i1-GGUF/resolve/main/Swallow-70b-NVE-hf.i1-IQ3_M.gguf) | i1-IQ3_M | 31.0 | |
| [GGUF](https://huggingface.co/mradermacher/Swallow-70b-NVE-hf-i1-GGUF/resolve/main/Swallow-70b-NVE-hf.i1-Q3_K_M.gguf) | i1-Q3_K_M | 33.4 | IQ3_S probably better |
| [GGUF](https://huggingface.co/mradermacher/Swallow-70b-NVE-hf-i1-GGUF/resolve/main/Swallow-70b-NVE-hf.i1-Q3_K_L.gguf) | i1-Q3_K_L | 36.2 | IQ3_M probably better |
| [GGUF](https://huggingface.co/mradermacher/Swallow-70b-NVE-hf-i1-GGUF/resolve/main/Swallow-70b-NVE-hf.i1-IQ4_XS.gguf) | i1-IQ4_XS | 36.9 | |
| [GGUF](https://huggingface.co/mradermacher/Swallow-70b-NVE-hf-i1-GGUF/resolve/main/Swallow-70b-NVE-hf.i1-Q4_0.gguf) | i1-Q4_0 | 39.1 | fast, low quality |
| [GGUF](https://huggingface.co/mradermacher/Swallow-70b-NVE-hf-i1-GGUF/resolve/main/Swallow-70b-NVE-hf.i1-Q4_K_S.gguf) | i1-Q4_K_S | 39.3 | optimal size/speed/quality |
| [GGUF](https://huggingface.co/mradermacher/Swallow-70b-NVE-hf-i1-GGUF/resolve/main/Swallow-70b-NVE-hf.i1-Q4_K_M.gguf) | i1-Q4_K_M | 41.5 | fast, recommended |
| [GGUF](https://huggingface.co/mradermacher/Swallow-70b-NVE-hf-i1-GGUF/resolve/main/Swallow-70b-NVE-hf.i1-Q5_K_S.gguf) | i1-Q5_K_S | 47.6 | |
| [GGUF](https://huggingface.co/mradermacher/Swallow-70b-NVE-hf-i1-GGUF/resolve/main/Swallow-70b-NVE-hf.i1-Q5_K_M.gguf) | i1-Q5_K_M | 48.9 | |
| [PART 1](https://huggingface.co/mradermacher/Swallow-70b-NVE-hf-i1-GGUF/resolve/main/Swallow-70b-NVE-hf.i1-Q6_K.gguf.part1of2) [PART 2](https://huggingface.co/mradermacher/Swallow-70b-NVE-hf-i1-GGUF/resolve/main/Swallow-70b-NVE-hf.i1-Q6_K.gguf.part2of2) | i1-Q6_K | 56.7 | practically like static Q6_K |
Here is a handy graph by ikawrakow comparing some lower-quality quant
types (lower is better):

And here are Artefact2's thoughts on the matter:
https://gist.github.com/Artefact2/b5f810600771265fc1e39442288e8ec9
## FAQ / Model Request
See https://huggingface.co/mradermacher/model_requests for some answers to
questions you might have and/or if you want some other model quantized.
## Thanks
I thank my company, [nethype GmbH](https://www.nethype.de/), for letting
me use its servers and providing upgrades to my workstation to enable
this work in my free time. Additional thanks to [@nicoboss](https://huggingface.co/nicoboss) for giving me access to his hardware for calculating the imatrix for these quants.
<!-- end -->
|
mradermacher/Smaug-Qwen2-72B-Instruct-i1-GGUF | mradermacher | "2024-06-28T19:06:21Z" | 94,511 | 0 | transformers | [
"transformers",
"gguf",
"chat",
"en",
"base_model:abacusai/Smaug-Qwen2-72B-Instruct",
"license:other",
"endpoints_compatible",
"region:us"
] | null | "2024-06-27T16:21:55Z" | ---
base_model: abacusai/Smaug-Qwen2-72B-Instruct
language:
- en
library_name: transformers
license: other
license_link: https://huggingface.co/Qwen/Qwen2-72B-Instruct/blob/main/LICENSE
license_name: tongyi-qianwen
quantized_by: mradermacher
tags:
- chat
---
## About
<!-- ### quantize_version: 2 -->
<!-- ### output_tensor_quantised: 1 -->
<!-- ### convert_type: hf -->
<!-- ### vocab_type: -->
<!-- ### tags: nicoboss -->
weighted/imatrix quants of https://huggingface.co/abacusai/Smaug-Qwen2-72B-Instruct
<!-- provided-files -->
static quants are available at https://huggingface.co/mradermacher/Smaug-Qwen2-72B-Instruct-GGUF
## Usage
If you are unsure how to use GGUF files, refer to one of [TheBloke's
READMEs](https://huggingface.co/TheBloke/KafkaLM-70B-German-V0.1-GGUF) for
more details, including on how to concatenate multi-part files.
## Provided Quants
(sorted by size, not necessarily quality. IQ-quants are often preferable over similar sized non-IQ quants)
| Link | Type | Size/GB | Notes |
|:-----|:-----|--------:|:------|
| [GGUF](https://huggingface.co/mradermacher/Smaug-Qwen2-72B-Instruct-i1-GGUF/resolve/main/Smaug-Qwen2-72B-Instruct.i1-IQ1_S.gguf) | i1-IQ1_S | 22.8 | for the desperate |
| [GGUF](https://huggingface.co/mradermacher/Smaug-Qwen2-72B-Instruct-i1-GGUF/resolve/main/Smaug-Qwen2-72B-Instruct.i1-IQ1_M.gguf) | i1-IQ1_M | 23.8 | mostly desperate |
| [GGUF](https://huggingface.co/mradermacher/Smaug-Qwen2-72B-Instruct-i1-GGUF/resolve/main/Smaug-Qwen2-72B-Instruct.i1-IQ2_XXS.gguf) | i1-IQ2_XXS | 25.6 | |
| [GGUF](https://huggingface.co/mradermacher/Smaug-Qwen2-72B-Instruct-i1-GGUF/resolve/main/Smaug-Qwen2-72B-Instruct.i1-IQ2_XS.gguf) | i1-IQ2_XS | 27.2 | |
| [GGUF](https://huggingface.co/mradermacher/Smaug-Qwen2-72B-Instruct-i1-GGUF/resolve/main/Smaug-Qwen2-72B-Instruct.i1-IQ2_S.gguf) | i1-IQ2_S | 28.0 | |
| [GGUF](https://huggingface.co/mradermacher/Smaug-Qwen2-72B-Instruct-i1-GGUF/resolve/main/Smaug-Qwen2-72B-Instruct.i1-IQ2_M.gguf) | i1-IQ2_M | 29.4 | |
| [GGUF](https://huggingface.co/mradermacher/Smaug-Qwen2-72B-Instruct-i1-GGUF/resolve/main/Smaug-Qwen2-72B-Instruct.i1-Q2_K.gguf) | i1-Q2_K | 29.9 | IQ3_XXS probably better |
| [GGUF](https://huggingface.co/mradermacher/Smaug-Qwen2-72B-Instruct-i1-GGUF/resolve/main/Smaug-Qwen2-72B-Instruct.i1-IQ3_XXS.gguf) | i1-IQ3_XXS | 31.9 | lower quality |
| [GGUF](https://huggingface.co/mradermacher/Smaug-Qwen2-72B-Instruct-i1-GGUF/resolve/main/Smaug-Qwen2-72B-Instruct.i1-IQ3_XS.gguf) | i1-IQ3_XS | 32.9 | |
| [GGUF](https://huggingface.co/mradermacher/Smaug-Qwen2-72B-Instruct-i1-GGUF/resolve/main/Smaug-Qwen2-72B-Instruct.i1-IQ3_S.gguf) | i1-IQ3_S | 34.6 | beats Q3_K* |
| [GGUF](https://huggingface.co/mradermacher/Smaug-Qwen2-72B-Instruct-i1-GGUF/resolve/main/Smaug-Qwen2-72B-Instruct.i1-Q3_K_S.gguf) | i1-Q3_K_S | 34.6 | IQ3_XS probably better |
| [GGUF](https://huggingface.co/mradermacher/Smaug-Qwen2-72B-Instruct-i1-GGUF/resolve/main/Smaug-Qwen2-72B-Instruct.i1-IQ3_M.gguf) | i1-IQ3_M | 35.6 | |
| [GGUF](https://huggingface.co/mradermacher/Smaug-Qwen2-72B-Instruct-i1-GGUF/resolve/main/Smaug-Qwen2-72B-Instruct.i1-Q3_K_M.gguf) | i1-Q3_K_M | 37.8 | IQ3_S probably better |
| [GGUF](https://huggingface.co/mradermacher/Smaug-Qwen2-72B-Instruct-i1-GGUF/resolve/main/Smaug-Qwen2-72B-Instruct.i1-Q3_K_L.gguf) | i1-Q3_K_L | 39.6 | IQ3_M probably better |
| [GGUF](https://huggingface.co/mradermacher/Smaug-Qwen2-72B-Instruct-i1-GGUF/resolve/main/Smaug-Qwen2-72B-Instruct.i1-IQ4_XS.gguf) | i1-IQ4_XS | 39.8 | |
| [GGUF](https://huggingface.co/mradermacher/Smaug-Qwen2-72B-Instruct-i1-GGUF/resolve/main/Smaug-Qwen2-72B-Instruct.i1-Q4_0.gguf) | i1-Q4_0 | 41.5 | fast, low quality |
| [GGUF](https://huggingface.co/mradermacher/Smaug-Qwen2-72B-Instruct-i1-GGUF/resolve/main/Smaug-Qwen2-72B-Instruct.i1-Q4_K_S.gguf) | i1-Q4_K_S | 44.0 | optimal size/speed/quality |
| [GGUF](https://huggingface.co/mradermacher/Smaug-Qwen2-72B-Instruct-i1-GGUF/resolve/main/Smaug-Qwen2-72B-Instruct.i1-Q4_K_M.gguf) | i1-Q4_K_M | 47.5 | fast, recommended |
| [PART 1](https://huggingface.co/mradermacher/Smaug-Qwen2-72B-Instruct-i1-GGUF/resolve/main/Smaug-Qwen2-72B-Instruct.i1-Q5_K_S.gguf.part1of2) [PART 2](https://huggingface.co/mradermacher/Smaug-Qwen2-72B-Instruct-i1-GGUF/resolve/main/Smaug-Qwen2-72B-Instruct.i1-Q5_K_S.gguf.part2of2) | i1-Q5_K_S | 51.5 | |
| [PART 1](https://huggingface.co/mradermacher/Smaug-Qwen2-72B-Instruct-i1-GGUF/resolve/main/Smaug-Qwen2-72B-Instruct.i1-Q5_K_M.gguf.part1of2) [PART 2](https://huggingface.co/mradermacher/Smaug-Qwen2-72B-Instruct-i1-GGUF/resolve/main/Smaug-Qwen2-72B-Instruct.i1-Q5_K_M.gguf.part2of2) | i1-Q5_K_M | 54.5 | |
| [PART 1](https://huggingface.co/mradermacher/Smaug-Qwen2-72B-Instruct-i1-GGUF/resolve/main/Smaug-Qwen2-72B-Instruct.i1-Q6_K.gguf.part1of2) [PART 2](https://huggingface.co/mradermacher/Smaug-Qwen2-72B-Instruct-i1-GGUF/resolve/main/Smaug-Qwen2-72B-Instruct.i1-Q6_K.gguf.part2of2) | i1-Q6_K | 64.4 | practically like static Q6_K |
Here is a handy graph by ikawrakow comparing some lower-quality quant
types (lower is better):

And here are Artefact2's thoughts on the matter:
https://gist.github.com/Artefact2/b5f810600771265fc1e39442288e8ec9
## FAQ / Model Request
See https://huggingface.co/mradermacher/model_requests for some answers to
questions you might have and/or if you want some other model quantized.
## Thanks
I thank my company, [nethype GmbH](https://www.nethype.de/), for letting
me use its servers and providing upgrades to my workstation to enable
this work in my free time. Additional thanks to [@nicoboss](https://huggingface.co/nicoboss) for giving me access to his hardware for calculating the imatrix for these quants.
<!-- end -->
|
bartowski/firefunction-v2-GGUF | bartowski | "2024-06-22T19:30:10Z" | 94,332 | 2 | null | [
"gguf",
"function-calling",
"text-generation",
"license:llama3",
"region:us"
] | text-generation | "2024-06-22T06:03:38Z" | ---
license: llama3
tags:
- function-calling
quantized_by: bartowski
pipeline_tag: text-generation
---
## Llamacpp imatrix Quantizations of firefunction-v2
Using <a href="https://github.com/ggerganov/llama.cpp/">llama.cpp</a> release <a href="https://github.com/ggerganov/llama.cpp/releases/tag/b3197">b3197</a> for quantization.
Original model: https://huggingface.co/fireworks-ai/firefunction-v2
All quants made using imatrix option with dataset from [here](https://gist.github.com/bartowski1182/eb213dccb3571f863da82e99418f81e8)
## Prompt format
```
<|begin_of_text|><|start_header_id|>system<|end_header_id|>
You are a helpful assistant with access to functions.
In addition to plain text responses, you can chose to call one or more of the provided functions.
Use the following rule to decide when to call a function:
* if the response can be generated from your internal knowledge (e.g., as in the case of queries like "What is the capital of Poland?"), do so
* if you need external information that can be obtained by calling one or more of the provided functions, generate a function calls
If you decide to call functions:
* prefix function calls with functools marker (no closing marker required)
* all function calls should be generated in a single JSON list formatted as functools[{"name": [function name], "arguments": [function arguments as JSON]},...]
* follow the provided JSON schema. Do not hallucinate arguments or values. Do to blindly copy values from the provided samples
* respect the argument type formatting. E.g., if the type if number and format is float, write value 7 as 7.0
* make sure you pick the right functions that match the user intent
Available functions as JSON spec:
[
{functions}
]
Today is {datetime}.<|eot_id|><|start_header_id|>user<|end_header_id|>
{prompt}<|eot_id|><|start_header_id|>assistant<|end_header_id|>
```
## Download a file (not the whole branch) from below:
| Filename | Quant type | File Size | Description |
| -------- | ---------- | --------- | ----------- |
| [firefunction-v2-Q8_0.gguf](https://huggingface.co/bartowski/firefunction-v2-GGUF//main/firefunction-v2-Q8_0.gguf) | Q8_0 | 0GB | Extremely high quality, generally unneeded but max available quant. |
| [firefunction-v2-Q6_K.gguf](https://huggingface.co/bartowski/firefunction-v2-GGUF//main/firefunction-v2-Q6_K.gguf) | Q6_K | 0GB | Very high quality, near perfect, *recommended*. |
| [firefunction-v2-Q5_K_L.gguf](https://huggingface.co/bartowski/firefunction-v2-GGUF//main/firefunction-v2-Q5_K_L.gguf) | Q5_K_L | 0GB | *Experimental*, uses f16 for embed and output weights. Please provide any feedback of differences. High quality, *recommended*. |
| [firefunction-v2-Q5_K_M.gguf](https://huggingface.co/bartowski/firefunction-v2-GGUF/blob/main/firefunction-v2-Q5_K_M.gguf) | Q5_K_M | 49.94GB | High quality, *recommended*. |
| [firefunction-v2-Q4_K_L.gguf](https://huggingface.co/bartowski/firefunction-v2-GGUF/blob/main/firefunction-v2-Q4_K_L.gguf) | Q4_K_L | 45.27GB | *Experimental*, uses f16 for embed and output weights. Please provide any feedback of differences. Good quality, uses about 4.83 bits per weight, *recommended*. |
| [firefunction-v2-Q4_K_M.gguf](https://huggingface.co/bartowski/firefunction-v2-GGUF/blob/main/firefunction-v2-Q4_K_M.gguf) | Q4_K_M | 42.52GB | Good quality, uses about 4.83 bits per weight, *recommended*. |
| [firefunction-v2-IQ4_XS.gguf](https://huggingface.co/bartowski/firefunction-v2-GGUF/blob/main/firefunction-v2-IQ4_XS.gguf) | IQ4_XS | 37.90GB | Decent quality, smaller than Q4_K_S with similar performance, *recommended*. |
| [firefunction-v2-Q3_K_M.gguf](https://huggingface.co/bartowski/firefunction-v2-GGUF/blob/main/firefunction-v2-Q3_K_M.gguf) | Q3_K_M | 34.26GB | Even lower quality. |
| [firefunction-v2-IQ3_M.gguf](https://huggingface.co/bartowski/firefunction-v2-GGUF/blob/main/firefunction-v2-IQ3_M.gguf) | IQ3_M | 31.93GB | Medium-low quality, new method with decent performance comparable to Q3_K_M. |
| [firefunction-v2-Q3_K_S.gguf](https://huggingface.co/bartowski/firefunction-v2-GGUF/blob/main/firefunction-v2-Q3_K_S.gguf) | Q3_K_S | 30.91GB | Low quality, not recommended. |
| [firefunction-v2-IQ3_XXS.gguf](https://huggingface.co/bartowski/firefunction-v2-GGUF/blob/main/firefunction-v2-IQ3_XXS.gguf) | IQ3_XXS | 27.46GB | Lower quality, new method with decent performance, comparable to Q3 quants. |
| [firefunction-v2-Q2_K.gguf](https://huggingface.co/bartowski/firefunction-v2-GGUF/blob/main/firefunction-v2-Q2_K.gguf) | Q2_K | 26.37GB | Very low quality but surprisingly usable. |
| [firefunction-v2-IQ2_M.gguf](https://huggingface.co/bartowski/firefunction-v2-GGUF/blob/main/firefunction-v2-IQ2_M.gguf) | IQ2_M | 24.11GB | Very low quality, uses SOTA techniques to also be surprisingly usable. |
| [firefunction-v2-IQ2_XS.gguf](https://huggingface.co/bartowski/firefunction-v2-GGUF/blob/main/firefunction-v2-IQ2_XS.gguf) | IQ2_XS | 21.14GB | Lower quality, uses SOTA techniques to be usable. |
| [firefunction-v2-IQ2_XXS.gguf](https://huggingface.co/bartowski/firefunction-v2-GGUF/blob/main/firefunction-v2-IQ2_XXS.gguf) | IQ2_XXS | 19.09GB | Lower quality, uses SOTA techniques to be usable. |
| [firefunction-v2-IQ1_M.gguf](https://huggingface.co/bartowski/firefunction-v2-GGUF/blob/main/firefunction-v2-IQ1_M.gguf) | IQ1_M | 16.75GB | Extremely low quality, *not* recommended. |
## Downloading using huggingface-cli
First, make sure you have hugginface-cli installed:
```
pip install -U "huggingface_hub[cli]"
```
Then, you can target the specific file you want:
```
huggingface-cli download bartowski/firefunction-v2-GGUF --include "firefunction-v2-Q4_K_M.gguf" --local-dir ./
```
If the model is bigger than 50GB, it will have been split into multiple files. In order to download them all to a local folder, run:
```
huggingface-cli download bartowski/firefunction-v2-GGUF --include "firefunction-v2-Q8_0.gguf/*" --local-dir firefunction-v2-Q8_0
```
You can either specify a new local-dir (firefunction-v2-Q8_0) or download them all in place (./)
## Which file should I choose?
A great write up with charts showing various performances is provided by Artefact2 [here](https://gist.github.com/Artefact2/b5f810600771265fc1e39442288e8ec9)
The first thing to figure out is how big a model you can run. To do this, you'll need to figure out how much RAM and/or VRAM you have.
If you want your model running as FAST as possible, you'll want to fit the whole thing on your GPU's VRAM. Aim for a quant with a file size 1-2GB smaller than your GPU's total VRAM.
If you want the absolute maximum quality, add both your system RAM and your GPU's VRAM together, then similarly grab a quant with a file size 1-2GB Smaller than that total.
Next, you'll need to decide if you want to use an 'I-quant' or a 'K-quant'.
If you don't want to think too much, grab one of the K-quants. These are in format 'QX_K_X', like Q5_K_M.
If you want to get more into the weeds, you can check out this extremely useful feature chart:
[llama.cpp feature matrix](https://github.com/ggerganov/llama.cpp/wiki/Feature-matrix)
But basically, if you're aiming for below Q4, and you're running cuBLAS (Nvidia) or rocBLAS (AMD), you should look towards the I-quants. These are in format IQX_X, like IQ3_M. These are newer and offer better performance for their size.
These I-quants can also be used on CPU and Apple Metal, but will be slower than their K-quant equivalent, so speed vs performance is a tradeoff you'll have to decide.
The I-quants are *not* compatible with Vulcan, which is also AMD, so if you have an AMD card double check if you're using the rocBLAS build or the Vulcan build. At the time of writing this, LM Studio has a preview with ROCm support, and other inference engines have specific builds for ROCm.
Want to support my work? Visit my ko-fi page here: https://ko-fi.com/bartowski
|
jbochi/madlad400-3b-mt | jbochi | "2024-01-10T15:00:28Z" | 94,144 | 116 | transformers | [
"transformers",
"safetensors",
"gguf",
"t5",
"text2text-generation",
"text-generation-inference",
"translation",
"multilingual",
"en",
"ru",
"es",
"fr",
"de",
"it",
"pt",
"pl",
"nl",
"vi",
"tr",
"sv",
"id",
"ro",
"cs",
"zh",
"hu",
"ja",
"th",
"fi",
"fa",
"uk",
"da",
"el",
"no",
"bg",
"sk",
"ko",
"ar",
"lt",
"ca",
"sl",
"he",
"et",
"lv",
"hi",
"sq",
"ms",
"az",
"sr",
"ta",
"hr",
"kk",
"is",
"ml",
"mr",
"te",
"af",
"gl",
"fil",
"be",
"mk",
"eu",
"bn",
"ka",
"mn",
"bs",
"uz",
"ur",
"sw",
"yue",
"ne",
"kn",
"kaa",
"gu",
"si",
"cy",
"eo",
"la",
"hy",
"ky",
"tg",
"ga",
"mt",
"my",
"km",
"tt",
"so",
"ku",
"ps",
"pa",
"rw",
"lo",
"ha",
"dv",
"fy",
"lb",
"ckb",
"mg",
"gd",
"am",
"ug",
"ht",
"grc",
"hmn",
"sd",
"jv",
"mi",
"tk",
"ceb",
"yi",
"ba",
"fo",
"or",
"xh",
"su",
"kl",
"ny",
"sm",
"sn",
"co",
"zu",
"ig",
"yo",
"pap",
"st",
"haw",
"as",
"oc",
"cv",
"lus",
"tet",
"gsw",
"sah",
"br",
"rm",
"sa",
"bo",
"om",
"se",
"ce",
"cnh",
"ilo",
"hil",
"udm",
"os",
"lg",
"ti",
"vec",
"ts",
"tyv",
"kbd",
"ee",
"iba",
"av",
"kha",
"to",
"tn",
"nso",
"fj",
"zza",
"ak",
"ada",
"otq",
"dz",
"bua",
"cfm",
"ln",
"chm",
"gn",
"krc",
"wa",
"hif",
"yua",
"srn",
"war",
"rom",
"bik",
"pam",
"sg",
"lu",
"ady",
"kbp",
"syr",
"ltg",
"myv",
"iso",
"kac",
"bho",
"ay",
"kum",
"qu",
"za",
"pag",
"ngu",
"ve",
"pck",
"zap",
"tyz",
"hui",
"bbc",
"tzo",
"tiv",
"ksd",
"gom",
"min",
"ang",
"nhe",
"bgp",
"nzi",
"nnb",
"nv",
"zxx",
"bci",
"kv",
"new",
"mps",
"alt",
"meu",
"bew",
"fon",
"iu",
"abt",
"mgh",
"mnw",
"tvl",
"dov",
"tlh",
"ho",
"kw",
"mrj",
"meo",
"crh",
"mbt",
"emp",
"ace",
"ium",
"mam",
"gym",
"mai",
"crs",
"pon",
"ubu",
"fip",
"quc",
"gv",
"kj",
"btx",
"ape",
"chk",
"rcf",
"shn",
"tzh",
"mdf",
"ppk",
"ss",
"gag",
"cab",
"kri",
"seh",
"ibb",
"tbz",
"bru",
"enq",
"ach",
"cuk",
"kmb",
"wo",
"kek",
"qub",
"tab",
"bts",
"kos",
"rwo",
"cak",
"tuc",
"bum",
"cjk",
"gil",
"stq",
"tsg",
"quh",
"mak",
"arn",
"ban",
"jiv",
"sja",
"yap",
"tcy",
"toj",
"twu",
"xal",
"amu",
"rmc",
"hus",
"nia",
"kjh",
"bm",
"guh",
"mas",
"acf",
"dtp",
"ksw",
"bzj",
"din",
"zne",
"mad",
"msi",
"mag",
"mkn",
"kg",
"lhu",
"ch",
"qvi",
"mh",
"djk",
"sus",
"mfe",
"srm",
"dyu",
"ctu",
"gui",
"pau",
"inb",
"bi",
"mni",
"guc",
"jam",
"wal",
"jac",
"bas",
"gor",
"skr",
"nyu",
"noa",
"sda",
"gub",
"nog",
"cni",
"teo",
"tdx",
"sxn",
"rki",
"nr",
"frp",
"alz",
"taj",
"lrc",
"cce",
"rn",
"jvn",
"hvn",
"nij",
"dwr",
"izz",
"msm",
"bus",
"ktu",
"chr",
"maz",
"tzj",
"suz",
"knj",
"bim",
"gvl",
"bqc",
"tca",
"pis",
"prk",
"laj",
"mel",
"qxr",
"niq",
"ahk",
"shp",
"hne",
"spp",
"koi",
"krj",
"quf",
"luz",
"agr",
"tsc",
"mqy",
"gof",
"gbm",
"miq",
"dje",
"awa",
"bjj",
"qvz",
"sjp",
"tll",
"raj",
"kjg",
"bgz",
"quy",
"cbk",
"akb",
"oj",
"ify",
"mey",
"ks",
"cac",
"brx",
"qup",
"syl",
"jax",
"ff",
"ber",
"tks",
"trp",
"mrw",
"adh",
"smt",
"srr",
"ffm",
"qvc",
"mtr",
"ann",
"aa",
"noe",
"nut",
"gyn",
"kwi",
"xmm",
"msb",
"dataset:allenai/MADLAD-400",
"arxiv:2309.04662",
"license:apache-2.0",
"autotrain_compatible",
"endpoints_compatible",
"region:us"
] | translation | "2023-09-21T00:28:38Z" | ---
license: apache-2.0
language:
- multilingual
- en
- ru
- es
- fr
- de
- it
- pt
- pl
- nl
- vi
- tr
- sv
- id
- ro
- cs
- zh
- hu
- ja
- th
- fi
- fa
- uk
- da
- el
- "no"
- bg
- sk
- ko
- ar
- lt
- ca
- sl
- he
- et
- lv
- hi
- sq
- ms
- az
- sr
- ta
- hr
- kk
- is
- ml
- mr
- te
- af
- gl
- fil
- be
- mk
- eu
- bn
- ka
- mn
- bs
- uz
- ur
- sw
- yue
- ne
- kn
- kaa
- gu
- si
- cy
- eo
- la
- hy
- ky
- tg
- ga
- mt
- my
- km
- tt
- so
- ku
- ps
- pa
- rw
- lo
- ha
- dv
- fy
- lb
- ckb
- mg
- gd
- am
- ug
- ht
- grc
- hmn
- sd
- jv
- mi
- tk
- ceb
- yi
- ba
- fo
- or
- xh
- su
- kl
- ny
- sm
- sn
- co
- zu
- ig
- yo
- pap
- st
- haw
- as
- oc
- cv
- lus
- tet
- gsw
- sah
- br
- rm
- sa
- bo
- om
- se
- ce
- cnh
- ilo
- hil
- udm
- os
- lg
- ti
- vec
- ts
- tyv
- kbd
- ee
- iba
- av
- kha
- to
- tn
- nso
- fj
- zza
- ak
- ada
- otq
- dz
- bua
- cfm
- ln
- chm
- gn
- krc
- wa
- hif
- yua
- srn
- war
- rom
- bik
- pam
- sg
- lu
- ady
- kbp
- syr
- ltg
- myv
- iso
- kac
- bho
- ay
- kum
- qu
- za
- pag
- ngu
- ve
- pck
- zap
- tyz
- hui
- bbc
- tzo
- tiv
- ksd
- gom
- min
- ang
- nhe
- bgp
- nzi
- nnb
- nv
- zxx
- bci
- kv
- new
- mps
- alt
- meu
- bew
- fon
- iu
- abt
- mgh
- mnw
- tvl
- dov
- tlh
- ho
- kw
- mrj
- meo
- crh
- mbt
- emp
- ace
- ium
- mam
- gym
- mai
- crs
- pon
- ubu
- fip
- quc
- gv
- kj
- btx
- ape
- chk
- rcf
- shn
- tzh
- mdf
- ppk
- ss
- gag
- cab
- kri
- seh
- ibb
- tbz
- bru
- enq
- ach
- cuk
- kmb
- wo
- kek
- qub
- tab
- bts
- kos
- rwo
- cak
- tuc
- bum
- cjk
- gil
- stq
- tsg
- quh
- mak
- arn
- ban
- jiv
- sja
- yap
- tcy
- toj
- twu
- xal
- amu
- rmc
- hus
- nia
- kjh
- bm
- guh
- mas
- acf
- dtp
- ksw
- bzj
- din
- zne
- mad
- msi
- mag
- mkn
- kg
- lhu
- ch
- qvi
- mh
- djk
- sus
- mfe
- srm
- dyu
- ctu
- gui
- pau
- inb
- bi
- mni
- guc
- jam
- wal
- jac
- bas
- gor
- skr
- nyu
- noa
- sda
- gub
- nog
- cni
- teo
- tdx
- sxn
- rki
- nr
- frp
- alz
- taj
- lrc
- cce
- rn
- jvn
- hvn
- nij
- dwr
- izz
- msm
- bus
- ktu
- chr
- maz
- tzj
- suz
- knj
- bim
- gvl
- bqc
- tca
- pis
- prk
- laj
- mel
- qxr
- niq
- ahk
- shp
- hne
- spp
- koi
- krj
- quf
- luz
- agr
- tsc
- mqy
- gof
- gbm
- miq
- dje
- awa
- bjj
- qvz
- sjp
- tll
- raj
- kjg
- bgz
- quy
- cbk
- akb
- oj
- ify
- mey
- ks
- cac
- brx
- qup
- syl
- jax
- ff
- ber
- tks
- trp
- mrw
- adh
- smt
- srr
- ffm
- qvc
- mtr
- ann
- kaa
- aa
- noe
- nut
- gyn
- kwi
- xmm
- msb
library_name: transformers
tags:
- text2text-generation
- text-generation-inference
datasets:
- allenai/MADLAD-400
pipeline_tag: translation
widget:
- text: "<2en> Como vai, amigo?"
example_title: "Translation to English"
- text: "<2de> Do you speak German?"
example_title: "Translation to German"
---
# Model Card for MADLAD-400-3B-MT
# Table of Contents
0. [TL;DR](#TL;DR)
1. [Model Details](#model-details)
2. [Usage](#usage)
3. [Uses](#uses)
4. [Bias, Risks, and Limitations](#bias-risks-and-limitations)
5. [Training Details](#training-details)
6. [Evaluation](#evaluation)
7. [Environmental Impact](#environmental-impact)
8. [Citation](#citation)
# TL;DR
MADLAD-400-3B-MT is a multilingual machine translation model based on the T5 architecture that was
trained on 1 trillion tokens covering over 450 languages using publicly available data.
It is competitive with models that are significantly larger.
**Disclaimer**: [Juarez Bochi](https://huggingface.co/jbochi), who was not involved in this research, converted
the original weights and wrote the contents of this model card based on the original paper and Flan-T5.
# Model Details
## Model Description
- **Model type:** Language model
- **Language(s) (NLP):** Multilingual (400+ languages)
- **License:** Apache 2.0
- **Related Models:** [All MADLAD-400 Checkpoints](https://huggingface.co/models?search=madlad)
- **Original Checkpoints:** [All Original MADLAD-400 Checkpoints](https://github.com/google-research/google-research/tree/master/madlad_400)
- **Resources for more information:**
- [Research paper](https://arxiv.org/abs/2309.04662)
- [GitHub Repo](https://github.com/google-research/t5x)
- [Hugging Face MADLAD-400 Docs (Similar to T5) ](https://huggingface.co/docs/transformers/model_doc/MADLAD-400) - [Pending PR](https://github.com/huggingface/transformers/pull/27471)
# Usage
Find below some example scripts on how to use the model:
## Using the Pytorch model with `transformers`
### Running the model on a CPU or GPU
<details>
<summary> Click to expand </summary>
First, install the Python packages that are required:
`pip install transformers accelerate sentencepiece protobuf`
```python
from transformers import T5ForConditionalGeneration, T5Tokenizer
model_name = 'jbochi/madlad400-3b-mt'
model = T5ForConditionalGeneration.from_pretrained(model_name, device_map="auto")
tokenizer = T5Tokenizer.from_pretrained(model_name)
text = "<2pt> I love pizza!"
input_ids = tokenizer(text, return_tensors="pt").input_ids.to(model.device)
outputs = model.generate(input_ids=input_ids)
tokenizer.decode(outputs[0], skip_special_tokens=True)
# Eu adoro pizza!
```
</details>
## Running the model with Candle
<details>
<summary> Click to expand </summary>
Usage with [candle](https://github.com/huggingface/candle):
```bash
$ cargo run --example t5 --release -- \
--model-id "jbochi/madlad400-3b-mt" \
--prompt "<2de> How are you, my friend?" \
--decode --temperature 0
```
We also provide a quantized model (1.65 GB vs the original 11.8 GB file):
```
cargo run --example quantized-t5 --release -- \
--model-id "jbochi/madlad400-3b-mt" --weight-file "model-q4k.gguf" \
--prompt "<2de> How are you, my friend?" \
--temperature 0
...
Wie geht es dir, mein Freund?
```
</details>
# Uses
## Direct Use and Downstream Use
> Primary intended uses: Machine Translation and multilingual NLP tasks on over 400 languages.
> Primary intended users: Research community.
## Out-of-Scope Use
> These models are trained on general domain data and are therefore not meant to
> work on domain-specific models out-of-the box. Moreover, these research models have not been assessed
> for production usecases.
# Bias, Risks, and Limitations
> We note that we evaluate on only 204 of the languages supported by these models and on machine translation
> and few-shot machine translation tasks. Users must consider use of this model carefully for their own
> usecase.
## Ethical considerations and risks
> We trained these models with MADLAD-400 and publicly available data to create baseline models that
> support NLP for over 400 languages, with a focus on languages underrepresented in large-scale corpora.
> Given that these models were trained with web-crawled datasets that may contain sensitive, offensive or
> otherwise low-quality content despite extensive preprocessing, it is still possible that these issues to the
> underlying training data may cause differences in model performance and toxic (or otherwise problematic)
> output for certain domains. Moreover, large models are dual use technologies that have specific risks
> associated with their use and development. We point the reader to surveys such as those written by
> Weidinger et al. or Bommasani et al. for a more detailed discussion of these risks, and to Liebling
> et al. for a thorough discussion of the risks of machine translation systems.
## Known Limitations
More information needed
## Sensitive Use:
More information needed
# Training Details
> We train models of various sizes: a 3B, 32-layer parameter model,
> a 7.2B 48-layer parameter model and a 10.7B 32-layer parameter model.
> We share all parameters of the model across language pairs,
> and use a Sentence Piece Model with 256k tokens shared on both the encoder and decoder
> side. Each input sentence has a <2xx> token prepended to the source sentence to indicate the target
> language.
See the [research paper](https://arxiv.org/pdf/2309.04662.pdf) for further details.
## Training Data
> For both the machine translation and language model, MADLAD-400 is used. For the machine translation
> model, a combination of parallel datasources covering 157 languages is also used. Further details are
> described in the [paper](https://arxiv.org/pdf/2309.04662.pdf).
## Training Procedure
See the [research paper](https://arxiv.org/pdf/2309.04662.pdf) for further details.
# Evaluation
## Testing Data, Factors & Metrics
> For evaluation, we used WMT, NTREX, Flores-200 and Gatones datasets as described in Section 4.3 in the [paper](https://arxiv.org/pdf/2309.04662.pdf).
> The translation quality of this model varies based on language, as seen in the paper, and likely varies on
> domain, though we have not assessed this.
## Results



See the [research paper](https://arxiv.org/pdf/2309.04662.pdf) for further details.
# Environmental Impact
More information needed
# Citation
**BibTeX:**
```bibtex
@misc{kudugunta2023madlad400,
title={MADLAD-400: A Multilingual And Document-Level Large Audited Dataset},
author={Sneha Kudugunta and Isaac Caswell and Biao Zhang and Xavier Garcia and Christopher A. Choquette-Choo and Katherine Lee and Derrick Xin and Aditya Kusupati and Romi Stella and Ankur Bapna and Orhan Firat},
year={2023},
eprint={2309.04662},
archivePrefix={arXiv},
primaryClass={cs.CL}
}
```
|
vikhyatk/moondream1 | vikhyatk | "2024-02-07T02:57:53Z" | 94,118 | 471 | transformers | [
"transformers",
"pytorch",
"safetensors",
"moondream1",
"text-generation",
"custom_code",
"en",
"autotrain_compatible",
"region:us"
] | text-generation | "2024-01-20T18:10:04Z" | ---
language:
- en
---
# 🌔 moondream1
1.6B parameter model built by [@vikhyatk](https://x.com/vikhyatk) using SigLIP, Phi-1.5 and the LLaVa training dataset.
The model is release for research purposes only, commercial use is not allowed.
Try it out on [Huggingface Spaces](https://huggingface.co/spaces/vikhyatk/moondream1)!
**Usage**
```
pip install transformers timm einops
```
```python
from transformers import AutoModelForCausalLM, CodeGenTokenizerFast as Tokenizer
from PIL import Image
model_id = "vikhyatk/moondream1"
model = AutoModelForCausalLM.from_pretrained(model_id, trust_remote_code=True)
tokenizer = Tokenizer.from_pretrained(model_id)
image = Image.open('<IMAGE_PATH>')
enc_image = model.encode_image(image)
print(model.answer_question(enc_image, "<QUESTION>", tokenizer))
```
## Benchmarks
| Model | Parameters | VQAv2 | GQA | TextVQA |
| --- | --- | --- | --- | --- |
| LLaVA-1.5 | 13.3B | 80.0 | 63.3 | 61.3 |
| LLaVA-1.5 | 7.3B | 78.5 | 62.0 | 58.2 |
| **moondream1** | 1.6B | 74.7 | 57.9 | 35.6 |
## Examples
| Image | Examples |
| --- | --- |
|  | **What is the title of this book?**<br>The Little Book of Deep Learning<br><br>**What can you tell me about this book?**<br>The book in the image is titled "The Little Book of Deep Learning." It appears to be a guide or manual that provides information and instructions on the subject of deep learning. The book is described as being small and thin, making it easy to carry and read. It is set in a room with a view outside, suggesting that the person holding the book might be in a living or working space with a view. The content of the book is likely to be complex, as the title implies a focus on advanced concepts in deep learning.<br><br>**Where is the person standing?**<br> The person is standing on a balcony or deck, which is outside. |
|  | **What type of food is the girl holding?**<br>The girl is holding a hamburger.<br><br>**What color is the woman's hair?**<br>The woman's hair is white.<br><br>**What is the girl doing in the image?**<br>The girl in the image is eating a hamburger. |
|  | **What color is the train?**<br>The train is red.<br><br>**What is the bus doing in the image?**<br> The bus in the image is driving down a city street, passing through an intersection.<br><br>**What kind of public transportation is in the image?**<br>The image features a red train or trolley as the public transportation option. |
|  | **What is the girl looking at?**<br>The girl is looking at her reflection in the mirror.<br><br>**What color is the woman's hair?**<br>The woman's hair is brown.<br><br>**What is the woman doing in the mirror?**<br>The woman is adjusting her hair in the mirror. |
|  | **What might be the reason for the dog's aggressive behavior?**<br>The dog's aggressive behavior, including its growling, baring its teeth, and showing its sharp teeth, could be due to various reasons. It is possible that the dog is feeling threatened, scared, or defensive about something in its environment, such as another animal, a loud noise, or a person nearby. Alternatively, the dog might be reacting to a command it received from its owner, or it could be experiencing discomfort or pain, which is causing it to display aggressive behavior. It is essential to observe the dog's body language and context to determine the underlying cause of its aggressive behavior and respond accordingly to address its needs or concerns.<br><br>**What kind of dog is in the picture?**<br>The dog in the picture is a small, black and white chihuahua. |
|
mradermacher/CabraLlama3-70b-v2-i1-GGUF | mradermacher | "2024-06-21T17:24:46Z" | 94,094 | 0 | transformers | [
"transformers",
"gguf",
"portuguese",
"llama",
"cabra",
"llama-3",
"pt",
"dataset:botbot-ai/Cabra3k",
"base_model:nicolasdec/CabraLlama3-70b-v2",
"license:llama3",
"endpoints_compatible",
"region:us"
] | null | "2024-06-20T14:12:15Z" | ---
base_model: nicolasdec/CabraLlama3-70b-v2
datasets:
- botbot-ai/Cabra3k
language:
- pt
library_name: transformers
license: llama3
quantized_by: mradermacher
tags:
- portuguese
- llama
- cabra
- llama-3
---
## About
<!-- ### quantize_version: 2 -->
<!-- ### output_tensor_quantised: 1 -->
<!-- ### convert_type: hf -->
<!-- ### vocab_type: -->
<!-- ### tags: nicoboss -->
weighted/imatrix quants of https://huggingface.co/nicolasdec/CabraLlama3-70b-v2
<!-- provided-files -->
static quants are available at https://huggingface.co/mradermacher/CabraLlama3-70b-v2-GGUF
## Usage
If you are unsure how to use GGUF files, refer to one of [TheBloke's
READMEs](https://huggingface.co/TheBloke/KafkaLM-70B-German-V0.1-GGUF) for
more details, including on how to concatenate multi-part files.
## Provided Quants
(sorted by size, not necessarily quality. IQ-quants are often preferable over similar sized non-IQ quants)
| Link | Type | Size/GB | Notes |
|:-----|:-----|--------:|:------|
| [GGUF](https://huggingface.co/mradermacher/CabraLlama3-70b-v2-i1-GGUF/resolve/main/CabraLlama3-70b-v2.i1-IQ1_S.gguf) | i1-IQ1_S | 15.4 | for the desperate |
| [GGUF](https://huggingface.co/mradermacher/CabraLlama3-70b-v2-i1-GGUF/resolve/main/CabraLlama3-70b-v2.i1-IQ1_M.gguf) | i1-IQ1_M | 16.9 | mostly desperate |
| [GGUF](https://huggingface.co/mradermacher/CabraLlama3-70b-v2-i1-GGUF/resolve/main/CabraLlama3-70b-v2.i1-IQ2_XXS.gguf) | i1-IQ2_XXS | 19.2 | |
| [GGUF](https://huggingface.co/mradermacher/CabraLlama3-70b-v2-i1-GGUF/resolve/main/CabraLlama3-70b-v2.i1-IQ2_XS.gguf) | i1-IQ2_XS | 21.2 | |
| [GGUF](https://huggingface.co/mradermacher/CabraLlama3-70b-v2-i1-GGUF/resolve/main/CabraLlama3-70b-v2.i1-IQ2_S.gguf) | i1-IQ2_S | 22.3 | |
| [GGUF](https://huggingface.co/mradermacher/CabraLlama3-70b-v2-i1-GGUF/resolve/main/CabraLlama3-70b-v2.i1-IQ2_M.gguf) | i1-IQ2_M | 24.2 | |
| [GGUF](https://huggingface.co/mradermacher/CabraLlama3-70b-v2-i1-GGUF/resolve/main/CabraLlama3-70b-v2.i1-Q2_K.gguf) | i1-Q2_K | 26.5 | IQ3_XXS probably better |
| [GGUF](https://huggingface.co/mradermacher/CabraLlama3-70b-v2-i1-GGUF/resolve/main/CabraLlama3-70b-v2.i1-IQ3_XXS.gguf) | i1-IQ3_XXS | 27.6 | lower quality |
| [GGUF](https://huggingface.co/mradermacher/CabraLlama3-70b-v2-i1-GGUF/resolve/main/CabraLlama3-70b-v2.i1-IQ3_XS.gguf) | i1-IQ3_XS | 29.4 | |
| [GGUF](https://huggingface.co/mradermacher/CabraLlama3-70b-v2-i1-GGUF/resolve/main/CabraLlama3-70b-v2.i1-IQ3_S.gguf) | i1-IQ3_S | 31.0 | beats Q3_K* |
| [GGUF](https://huggingface.co/mradermacher/CabraLlama3-70b-v2-i1-GGUF/resolve/main/CabraLlama3-70b-v2.i1-Q3_K_S.gguf) | i1-Q3_K_S | 31.0 | IQ3_XS probably better |
| [GGUF](https://huggingface.co/mradermacher/CabraLlama3-70b-v2-i1-GGUF/resolve/main/CabraLlama3-70b-v2.i1-IQ3_M.gguf) | i1-IQ3_M | 32.0 | |
| [GGUF](https://huggingface.co/mradermacher/CabraLlama3-70b-v2-i1-GGUF/resolve/main/CabraLlama3-70b-v2.i1-Q3_K_M.gguf) | i1-Q3_K_M | 34.4 | IQ3_S probably better |
| [GGUF](https://huggingface.co/mradermacher/CabraLlama3-70b-v2-i1-GGUF/resolve/main/CabraLlama3-70b-v2.i1-Q3_K_L.gguf) | i1-Q3_K_L | 37.2 | IQ3_M probably better |
| [GGUF](https://huggingface.co/mradermacher/CabraLlama3-70b-v2-i1-GGUF/resolve/main/CabraLlama3-70b-v2.i1-IQ4_XS.gguf) | i1-IQ4_XS | 38.0 | |
| [GGUF](https://huggingface.co/mradermacher/CabraLlama3-70b-v2-i1-GGUF/resolve/main/CabraLlama3-70b-v2.i1-Q4_0.gguf) | i1-Q4_0 | 40.2 | fast, low quality |
| [GGUF](https://huggingface.co/mradermacher/CabraLlama3-70b-v2-i1-GGUF/resolve/main/CabraLlama3-70b-v2.i1-Q4_K_S.gguf) | i1-Q4_K_S | 40.4 | optimal size/speed/quality |
| [GGUF](https://huggingface.co/mradermacher/CabraLlama3-70b-v2-i1-GGUF/resolve/main/CabraLlama3-70b-v2.i1-Q4_K_M.gguf) | i1-Q4_K_M | 42.6 | fast, recommended |
| [GGUF](https://huggingface.co/mradermacher/CabraLlama3-70b-v2-i1-GGUF/resolve/main/CabraLlama3-70b-v2.i1-Q5_K_S.gguf) | i1-Q5_K_S | 48.8 | |
| [GGUF](https://huggingface.co/mradermacher/CabraLlama3-70b-v2-i1-GGUF/resolve/main/CabraLlama3-70b-v2.i1-Q5_K_M.gguf) | i1-Q5_K_M | 50.0 | |
| [PART 1](https://huggingface.co/mradermacher/CabraLlama3-70b-v2-i1-GGUF/resolve/main/CabraLlama3-70b-v2.i1-Q6_K.gguf.part1of2) [PART 2](https://huggingface.co/mradermacher/CabraLlama3-70b-v2-i1-GGUF/resolve/main/CabraLlama3-70b-v2.i1-Q6_K.gguf.part2of2) | i1-Q6_K | 58.0 | practically like static Q6_K |
Here is a handy graph by ikawrakow comparing some lower-quality quant
types (lower is better):

And here are Artefact2's thoughts on the matter:
https://gist.github.com/Artefact2/b5f810600771265fc1e39442288e8ec9
## FAQ / Model Request
See https://huggingface.co/mradermacher/model_requests for some answers to
questions you might have and/or if you want some other model quantized.
## Thanks
I thank my company, [nethype GmbH](https://www.nethype.de/), for letting
me use its servers and providing upgrades to my workstation to enable
this work in my free time. Additional thanks to [@nicoboss](https://huggingface.co/nicoboss) for giving me access to his hardware for calculating the imatrix for these quants.
<!-- end -->
|