Commit
•
5719859
1
Parent(s):
da140f8
Create README.md
Browse files
README.md
ADDED
@@ -0,0 +1,83 @@
|
|
|
|
|
|
|
|
|
|
|
|
|
|
|
|
|
|
|
|
|
|
|
|
|
|
|
|
|
|
|
|
|
|
|
|
|
|
|
|
|
|
|
|
|
|
|
|
|
|
|
|
|
|
|
|
|
|
|
|
|
|
|
|
|
|
|
|
|
|
|
|
|
|
|
|
|
|
|
|
|
|
|
|
|
|
|
|
|
|
|
|
|
|
|
|
|
|
|
|
|
|
|
|
|
|
|
|
|
|
|
|
|
|
|
|
|
|
|
|
|
|
|
|
|
|
|
|
|
|
|
|
|
|
|
|
|
|
|
|
|
|
|
|
|
|
|
|
|
|
|
|
|
|
|
|
|
|
|
|
|
|
|
|
|
|
|
|
|
1 |
+
---
|
2 |
+
language: de
|
3 |
+
datasets:
|
4 |
+
- deepset/germandpr
|
5 |
+
license: mit
|
6 |
+
---
|
7 |
+
|
8 |
+
## Overview
|
9 |
+
**Language model:** gbert-base-germandpr-reranking
|
10 |
+
**Language:** German
|
11 |
+
**Training data:** GermanDPR train set (~ 56MB)
|
12 |
+
**Eval data:** GermanDPR test set (~ 6MB)
|
13 |
+
**Infrastructure**: 1x V100 GPU
|
14 |
+
**Published**: June 3rd, 2021
|
15 |
+
|
16 |
+
## Details
|
17 |
+
- We trained a text pair classification model in FARM, which can be used for reranking in document retrieval tasks. To this end, the classifier calculates the similarity of the query and each retrieved top k document (e.g., k=10). The top k documents are then sorted by their similarity scores. The document most similar to the query is the best.
|
18 |
+
|
19 |
+
## Hyperparameters
|
20 |
+
```
|
21 |
+
batch_size = 64
|
22 |
+
n_epochs = 2
|
23 |
+
max_seq_len = 512 tokens for question and passage concatenated
|
24 |
+
learning_rate = 2e-5
|
25 |
+
lr_schedule = LinearWarmup
|
26 |
+
embeds_dropout_prob = 0.1
|
27 |
+
```
|
28 |
+
## Performance
|
29 |
+
We use the GermanDPR test dataset as ground truth labels and run two experiments to compare how a BM25 retriever performs with or without reranking with our model. The first experiment runs retrieval on the full German Wikipedia (>2million passages) and second experiment runs retrieval on the GermanDPR dataset only (<5000 passages). Both experiments use 1025 queries. Note that the second experiment is evaluating on a much simpler task because of the smaller dataset size, which explains strong BM25 retrieval performance.
|
30 |
+
|
31 |
+
Full German Wikipedia:
|
32 |
+
BM25 Retriever without Reranking
|
33 |
+
-----------------
|
34 |
+
recall@3: 0.4088 (419 / 1025)
|
35 |
+
mean_reciprocal_rank@3: 0.3322
|
36 |
+
|
37 |
+
BM25 Retriever with Reranking Top 10 Documents
|
38 |
+
-----------------
|
39 |
+
recall@3: 0.5200 (533 / 1025)
|
40 |
+
mean_reciprocal_rank@3: 0.4800
|
41 |
+
|
42 |
+
Germandpr only:
|
43 |
+
BM25 Retriever without Reranking
|
44 |
+
-----------------
|
45 |
+
recall@3: 0.9102 (933 / 1025)
|
46 |
+
mean_reciprocal_rank@3: 0.8528
|
47 |
+
|
48 |
+
BM25 Retriever with Reranking Top 10 Documents
|
49 |
+
-----------------
|
50 |
+
recall@3: 0.9298 (953 / 1025)
|
51 |
+
mean_reciprocal_rank@3: 0.8813
|
52 |
+
|
53 |
+
|
54 |
+
|
55 |
+
## Usage
|
56 |
+
### In haystack
|
57 |
+
You can load the model in [haystack](https://github.com/deepset-ai/haystack/) for reranking the documents returned by a Retriever:
|
58 |
+
```python
|
59 |
+
...
|
60 |
+
retriever = ElasticsearchRetriever(document_store=document_store)
|
61 |
+
ranker = FARMRanker(model_name_or_path="deepset/gbert-base-germandpr-reranking")
|
62 |
+
...
|
63 |
+
p = Pipeline()
|
64 |
+
p.add_node(component=retriever, name="ESRetriever", inputs=["Query"])
|
65 |
+
p.add_node(component=ranker, name="Ranker", inputs=["ESRetriever"])
|
66 |
+
)
|
67 |
+
```
|
68 |
+
|
69 |
+
## About us
|
70 |
+
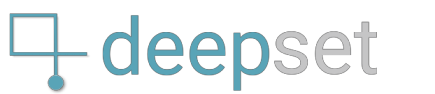
|
71 |
+
We bring NLP to the industry via open source!
|
72 |
+
Our focus: Industry specific language models & large scale QA systems.
|
73 |
+
|
74 |
+
Some of our work:
|
75 |
+
- [German BERT (aka "bert-base-german-cased")](https://deepset.ai/german-bert)
|
76 |
+
- [GermanQuAD and GermanDPR datasets and models (aka "gelectra-base-germanquad", "gbert-base-germandpr")](https://deepset.ai/germanquad)
|
77 |
+
- [FARM](https://github.com/deepset-ai/FARM)
|
78 |
+
- [Haystack](https://github.com/deepset-ai/haystack/)
|
79 |
+
|
80 |
+
Get in touch:
|
81 |
+
[Twitter](https://twitter.com/deepset_ai) | [LinkedIn](https://www.linkedin.com/company/deepset-ai/) | [Website](https://deepset.ai)
|
82 |
+
|
83 |
+
By the way: [we're hiring!](https://apply.workable.com/deepset/)
|