Julien Chaumond PRO
AI & ML interests
Articles
Organizations
julien-c's activity
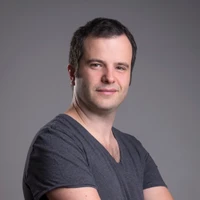
very cool, @yunusserhat !
cool! let's do more visibility about this? @Jofthomas @radames
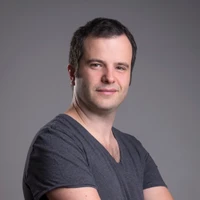
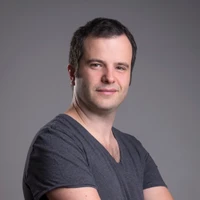
thanks @mishig for setting this up
Let's make the Hub as useful as possible for the community ❤️
and what about sign in with hf? WDYT?
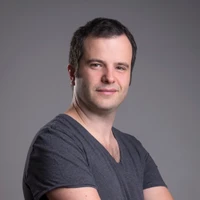
potential idea: plug "sign in with HF" as an authentication provider and persist interactions in a dataset or something
this is working quite well!
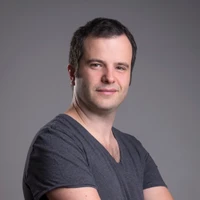
is there a dadjoke org on HF?
welcome!
very cool!
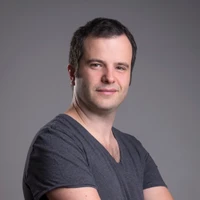
BOOOM
very cool!
Thanks for your great contributions @WizardLM , you are advancing AI for all of us 🦾
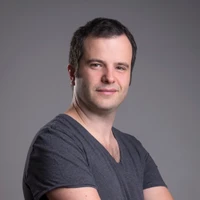
Along with text-embeddings-inference.
We just switched both of those repos' license back to Apache 2. 🔥
That is awesome! GG @bartowski and @yagilb and team 🔥
This could work quite well inside a Space too!
The UX could be really nice imo
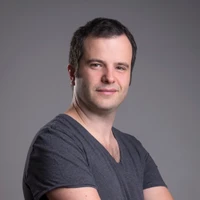
AI applied to 3D printing could be big.
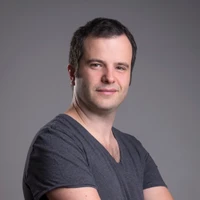
not yet! but soon:)
LaVague is awesome 🔥
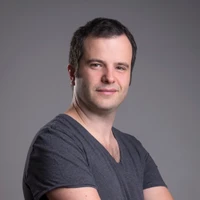
e/acc 😁
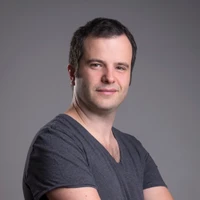
really nice blog
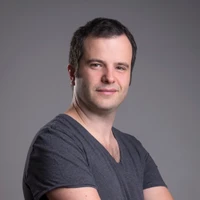
Gemini 1.5: Unlocking multimodal understanding across millions of tokens of context (2403.05530) Gemini 1.5: Unlocking multimodal understanding across millions of tokens of context
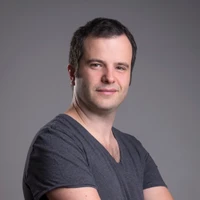
This is awesome. FSDP+QLoRA support is incredible 🔥
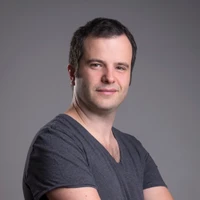
hello! welcome 👋
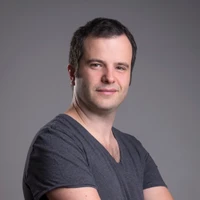
currently in private beta / undergoing some testing. Hopefully available soon!
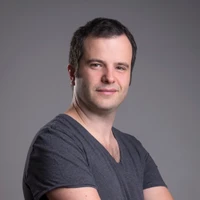
PS: did I mention we have an insane infra team at HF?
welcome here @chiphuyen 🤗
this is incredible 🤯
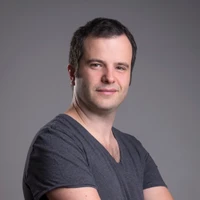
having more Earth Observation datasets in the open for the whole community is simple awesome. Thanks for sharing and looking forward to the cool things we can build with this 🌎🌏❤️
only tried it now, very nice space
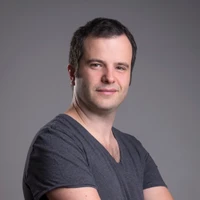
kudos for your awesome work on this @mrfakename
will try this ASAP!
❤️❤️
ja möglicherweise
Very excited about AMD next gen hardware 🔥
do you know if https://www.linkedin.com/in/eliovp is on the HF Hub?
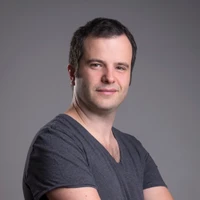
very cool! This is activated on a new /chat/completions
endpoint in text-generation-inference (in addition to the existing endpoint), correct?
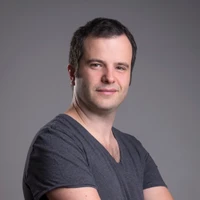
the Dataset Viewer is now available on *private datasets* too
You need to be a PRO or a Enterprise Hub user. 🔥
Great work from our Datasets team 🥰: @lhoestq @severo @polinaeterna @asoria @albertvillanova and the whole team 🥰
This is great, i want to repost this @jimfan even though we don't have the feature yet 🤣
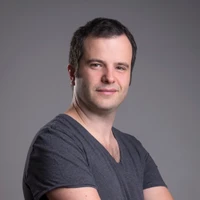
mysterious!
Flutter looks very cool!
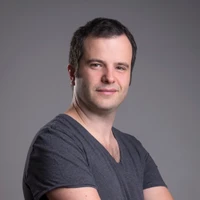
gonna do a collection of merve avatars 🔥
hat/tip @soldni 🎉
very cool! link to model checkpoint on the hub: https://huggingface.co/google/siglip-base-patch16-256-multilingual
very cool @chansung !
dstack looks very cool – @peterschmidt85 and team are on this platform btw: https://huggingface.co/dstackai – hi!
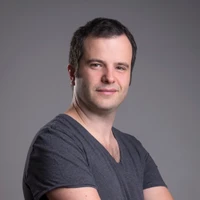
welcome. Post good stuff!
i would do the LLM in the cloud and the ASR+TTS on device
wdyt @victor ?
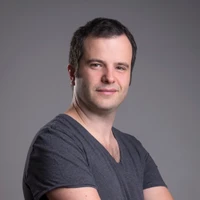
i was expecting dataset + dataset-viewer but this also works 😁
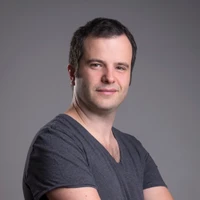
paris is too cold for @merve these days 🙉
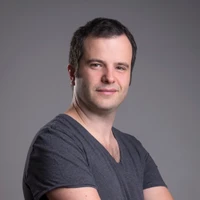
100%
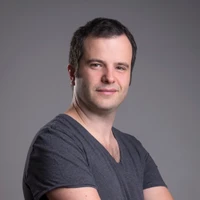
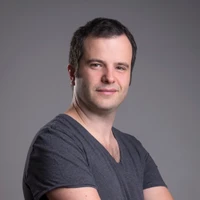
might be of interest to @merve @osanseviero too
would be awesome to link to the Paper pages 🔥
looks cool 🔥
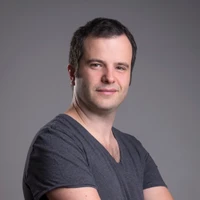
the Space was down, i rebooted it! great Space!