File size: 10,103 Bytes
70860f8 50e0acf 70860f8 3560a96 3e0831f 2d4ceff 16eb4c5 d478a77 828d428 5e4e554 dbd0ed7 9fddd0e befb6c9 6b91936 445e83e 14068ab befb6c9 34f2784 98e6327 34f2784 14068ab d1858c9 2eed696 de95105 2153e83 385926e d1858c9 71f69a9 d1858c9 0b01beb d1858c9 19053f7 d1858c9 de95105 71f69a9 de95105 d1858c9 2153e83 2eed696 fd864f7 2153e83 befb6c9 e9ec569 befb6c9 d4ebb5c befb6c9 d1858c9 5772277 cda02a7 2153e83 c83ff03 2eed696 c83ff03 2153e83 c83ff03 2153e83 fc74874 c83ff03 2d4ceff a121d01 5993af4 a121d01 2d4ceff 5993af4 1f17719 9975451 1f17719 2d4ceff |
1 2 3 4 5 6 7 8 9 10 11 12 13 14 15 16 17 18 19 20 21 22 23 24 25 26 27 28 29 30 31 32 33 34 35 36 37 38 39 40 41 42 43 44 45 46 47 48 49 50 51 52 53 54 55 56 57 58 59 60 61 62 63 64 65 66 67 68 69 70 71 72 73 74 75 76 77 78 79 80 81 82 83 84 85 86 87 88 89 90 91 92 93 94 95 96 97 98 99 100 101 102 103 104 105 106 107 108 109 110 111 112 113 114 115 116 117 118 119 120 121 122 123 124 125 126 127 128 129 130 131 132 133 134 135 136 137 138 139 140 141 142 143 144 145 146 147 148 149 150 151 152 153 154 155 156 157 158 159 160 161 162 163 164 165 166 167 168 169 170 171 172 173 174 175 176 177 178 179 180 181 182 183 184 185 186 187 188 189 190 191 192 193 194 195 196 197 198 199 200 201 202 203 204 205 206 207 208 209 210 211 212 213 214 215 216 217 218 219 220 221 222 223 224 225 226 227 228 229 230 231 232 233 234 235 236 237 238 239 240 241 242 243 244 245 246 |
---
pipeline_tag: sentence-similarity
tags:
- feature-extraction
- sentence-similarity
language: en
license: apache-2.0
---
# **m**utual **i**nformation **C**ontrastive **S**entence **E**mbedding (**miCSE**) for Low-shot Sentence Embeddings
Paper accepted at [ACL 2023](https://2023.aclweb.org/)[](https://arxiv.org/abs/2211.04928)[](https://github.com/SAP-samples/acl2023-micse/)
# Brief Model Description
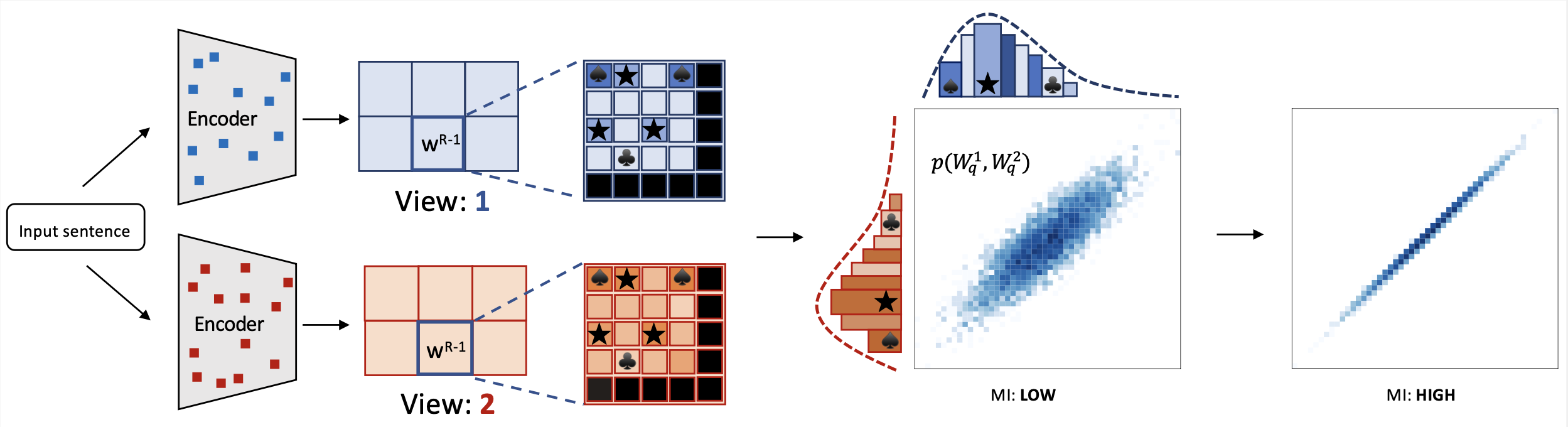
The **miCSE** language model is trained for sentence similarity computation. Training the model imposes alignment between the attention pattern of different views (embeddings of augmentations) during contrastive learning. Intuitively, learning sentence embeddings with miCSE entails enforcing __syntactic consistency across dropout augmented views__. Practically, this is achieved by regularizing the self-attention distribution. By regularizing self-attention during training, representation learning becomes much more sample efficient. Hence, self-supervised learning becomes tractable even when the training set is limited in size. This property makes miCSE particularly interesting for __real-world applications__, where training data is typically limited.
# Model Use Cases
The model intended to be used for encoding sentences or short paragraphs. Given an input text, the model produces a vector embedding capturing the semantics. Sentence representations correspond to embedding of the _**[CLS]**_ token. The embedding can be used for numerous tasks such as **retrieval**,**sentence similarity** comparison (see example 1) or **clustering** (see example 2).
# Training data
The model was trained on a random collection of **English** sentences from Wikipedia. The *full-shot* training file is available [here](https://huggingface.co/datasets/princeton-nlp/datasets-for-simcse/resolve/main/wiki1m_for_simcse.txt).
Low-shot training data consists of data splits of different sizes (from 10% to 0.0064%) of the [SimCSE](https://github.com/princeton-nlp/SimCSE) training corpus. Each split size comprises 5 files, created with a different seed indicated with filename postfix.
Data can be downloaded [here](https://huggingface.co/datasets/sap-ai-research/datasets-for-micse).
# Model Training
In order to make use of the **few-shot** capability of **miCSE**, the mode needs to be trained on your data. The source code and data splits used in the paper are available [here](https://github.com/SAP-samples/acl2023-micse).
## Training Data
# Model Usage
### Example 1) - Sentence Similarity
<details>
<summary> Click to expand </summary>
```python
from transformers import AutoTokenizer, AutoModel
import torch.nn as nn
tokenizer = AutoTokenizer.from_pretrained("sap-ai-research/miCSE")
model = AutoModel.from_pretrained("sap-ai-research/miCSE")
# Encoding of sentences in a list with a predefined maximum lengths of tokens (max_length)
max_length = 32
sentences = [
"This is a sentence for testing miCSE.",
"This is yet another test sentence for the mutual information Contrastive Sentence Embeddings model."
]
batch = tokenizer.batch_encode_plus(
sentences,
return_tensors='pt',
padding=True,
max_length=max_length,
truncation=True
)
# Compute the embeddings and keep only the _**[CLS]**_ embedding (the first token)
# Get raw embeddings (no gradients)
with torch.no_grad():
outputs = model(**batch, output_hidden_states=True, return_dict=True)
embeddings = outputs.last_hidden_state[:,0]
# Define similarity metric, e.g., cosine similarity
sim = nn.CosineSimilarity(dim=-1)
# Compute similarity between the **first** and the **second** sentence
cos_sim = sim(embeddings.unsqueeze(1),
embeddings.unsqueeze(0))
print(f"Distance: {cos_sim[0,1].detach().item()}")
```
</details>
### Example 2) - Clustering
<details>
<summary> Click to expand </summary>
```python
from transformers import AutoTokenizer, AutoModel
import torch.nn as nn
import torch
import numpy as np
import tqdm
from datasets import load_dataset
import umap
import umap.plot as umap_plot
# Determine available hardware
if torch.backends.mps.is_available():
device = torch.device("mps")
elif torch.cuda.is_available():
device = torch.device("cuda")
else:
device = torch.device("cpu")
# Load tokenizer and model
tokenizer = AutoTokenizer.from_pretrained("/Users/d065243/miCSE")
model = AutoModel.from_pretrained("/Users/d065243/miCSE")
model.to(device);
# Load Twitter data for sentiment clustering
dataset = load_dataset("tweet_eval", "sentiment")
# Compute embeddings of the tweets
# set batch size and maxium tweet token length
batch_size = 50
max_length = 128
iterations = int(np.floor(len(dataset['train'])/batch_size))*batch_size
embedding_stack = []
classes = []
for i in tqdm.notebook.tqdm(range(0,iterations,batch_size)):
# create batch
batch = tokenizer.batch_encode_plus(
dataset['train'][i:i+batch_size]['text'],
return_tensors='pt',
padding=True,
max_length=max_length,
truncation=True
).to(device)
classes = classes + dataset['train'][i:i+batch_size]['label']
# model inference without gradient
with torch.no_grad():
outputs = model(**batch, output_hidden_states=True, return_dict=True)
embeddings = outputs.last_hidden_state[:,0]
embedding_stack.append( embeddings.cpu().clone() )
embeddings = torch.vstack(embedding_stack)
# Cluster embeddings in 2D with UMAP
umap_model = umap.UMAP(n_neighbors=250,
n_components=2,
min_dist=1.0e-9,
low_memory=True,
angular_rp_forest=True,
metric='cosine')
umap_model.fit(embeddings)
# Plot result
umap_plot.points(umap_model, labels = np.array(classes),theme='fire')
```
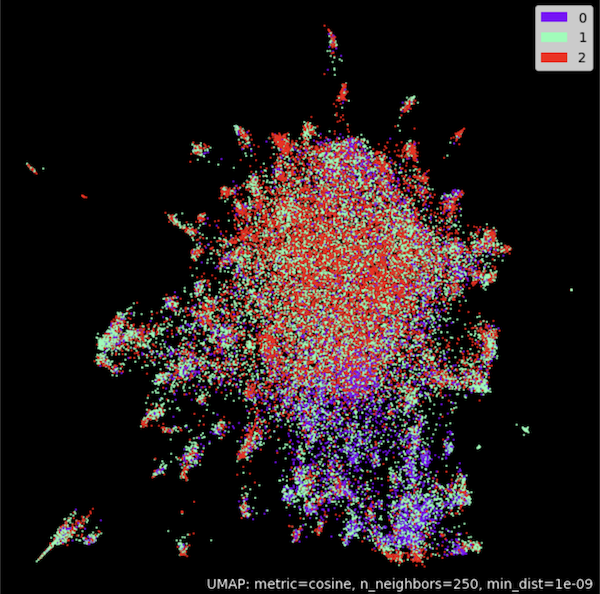
</details>
### Example 3) - Using [SentenceTransformers](https://www.sbert.net/)
<details>
<summary> Click to expand </summary>
```python
from sentence_transformers import SentenceTransformer, util
from sentence_transformers import models
import torch.nn as nn
# Using the model with [CLS] embeddings
model_name = 'sap-ai-research/miCSE'
word_embedding_model = models.Transformer(model_name, max_seq_length=32)
pooling_model = models.Pooling(word_embedding_model.get_word_embedding_dimension())
model = SentenceTransformer(modules=[word_embedding_model, pooling_model])
# Using cosine similarity as metric
cos_sim = nn.CosineSimilarity(dim=-1)
# List of sentences for comparison
sentences_1 = ["This is a sentence for testing miCSE.",
"This is using mutual information Contrastive Sentence Embeddings model."]
sentences_2 = ["This is testing miCSE.",
"Similarity with miCSE"]
# Compute embedding for both lists
embeddings_1 = model.encode(sentences_1, convert_to_tensor=True)
embeddings_2 = model.encode(sentences_2, convert_to_tensor=True)
# Compute cosine similarities
cosine_sim_scores = cos_sim(embeddings_1, embeddings_2)
#Output of results
for i in range(len(sentences1)):
print(f"Similarity {cosine_scores[i][i]:.2f}: {sentences1[i]} << vs. >> {sentences2[i]}")
```
</details>
<br/>
# Benchmark
Model results on SentEval Benchmark:
<details>
<summary> Click to expand </summary>
```shell
+-------+-------+-------+-------+-------+--------------+-----------------+--------+
| STS12 | STS13 | STS14 | STS15 | STS16 | STSBenchmark | SICKRelatedness | S.Avg. |
+-------+-------+-------+-------+-------+--------------+-----------------+--------+
| 71.71 | 83.09 | 75.46 | 83.13 | 80.22 | 79.70 | 73.62 | 78.13 |
+-------+-------+-------+-------+-------+--------------+-----------------+--------+
```
</details>
## Citations
If you use this code in your research or want to refer to our work, please cite:
```
@inproceedings{klein-nabi-2023-micse,
title = "mi{CSE}: Mutual Information Contrastive Learning for Low-shot Sentence Embeddings",
author = "Klein, Tassilo and
Nabi, Moin",
booktitle = "Proceedings of the 61st Annual Meeting of the Association for Computational Linguistics (Volume 1: Long Papers)",
month = jul,
year = "2023",
address = "Toronto, Canada",
publisher = "Association for Computational Linguistics",
url = "https://aclanthology.org/2023.acl-long.339",
pages = "6159--6177",
abstract = "This paper presents miCSE, a mutual information-based contrastive learning framework that significantly advances the state-of-the-art in few-shot sentence embedding.The proposed approach imposes alignment between the attention pattern of different views during contrastive learning. Learning sentence embeddings with miCSE entails enforcing the structural consistency across augmented views for every sentence, making contrastive self-supervised learning more sample efficient. As a result, the proposed approach shows strong performance in the few-shot learning domain. While it achieves superior results compared to state-of-the-art methods on multiple benchmarks in few-shot learning, it is comparable in the full-shot scenario. This study opens up avenues for efficient self-supervised learning methods that are more robust than current contrastive methods for sentence embedding.",
}
```
#### Authors:
- [Tassilo Klein](https://tjklein.github.io/)
- [Moin Nabi](https://moinnabi.github.io/) |