File size: 3,901 Bytes
38fef2e 251bba3 38fef2e |
1 2 3 4 5 6 7 8 9 10 11 12 13 14 15 16 17 18 19 20 21 22 23 24 25 26 27 28 29 30 31 32 33 34 35 36 37 38 39 40 41 42 43 44 45 46 47 48 49 50 51 52 53 54 55 56 57 58 59 60 61 62 63 64 65 66 67 68 69 70 71 72 73 74 75 76 77 78 79 80 81 82 83 84 85 86 |
---
license: apache-2.0
datasets:
- saucam/sans_data
language:
- sa
---
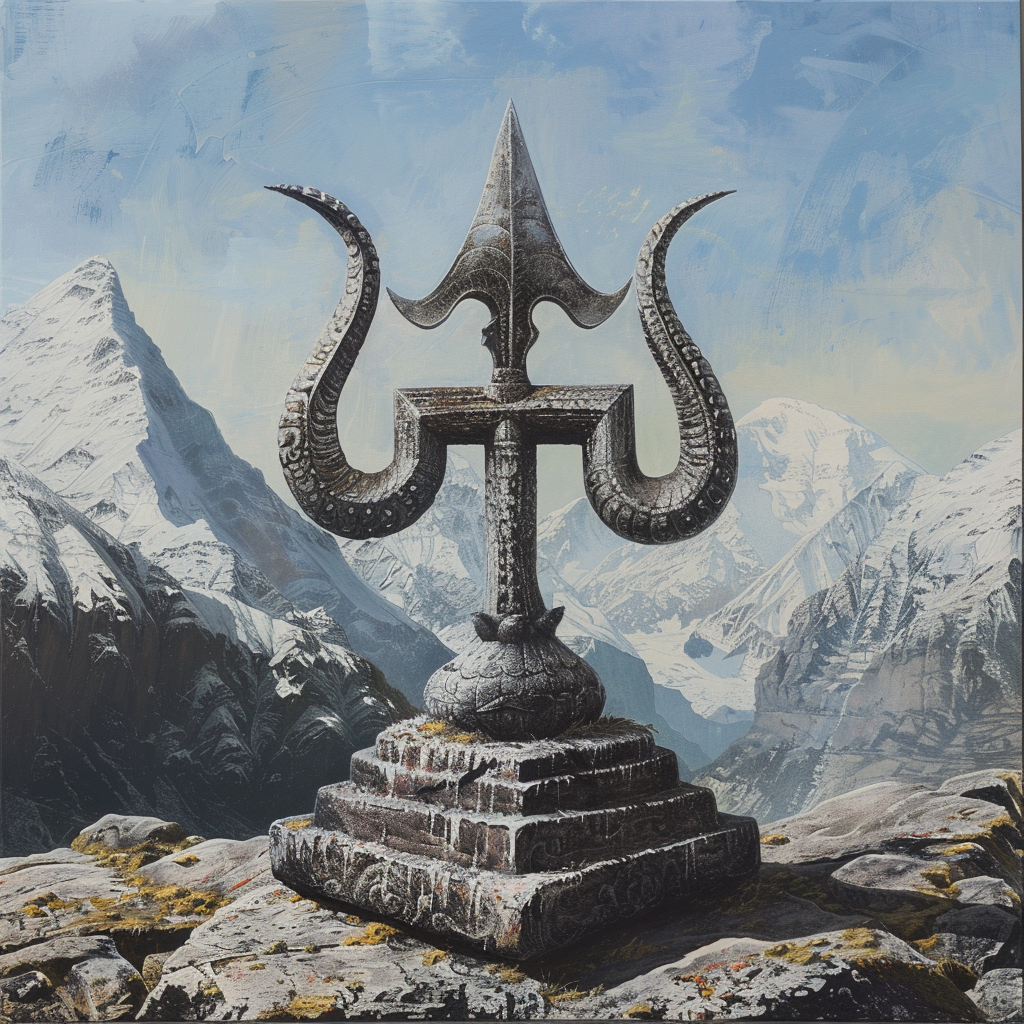
# 🔱 Rudra-7b
**Rudra-7b is a LoRA fine-tune of [gemma-7b](https://huggingface.co/google/gemma-7b) on sanskrit data**
This is a text-completion model for Sanskrit language. The model was finetuned using unsloth library.
I hope this paves the way for future work for Sanskrit models.
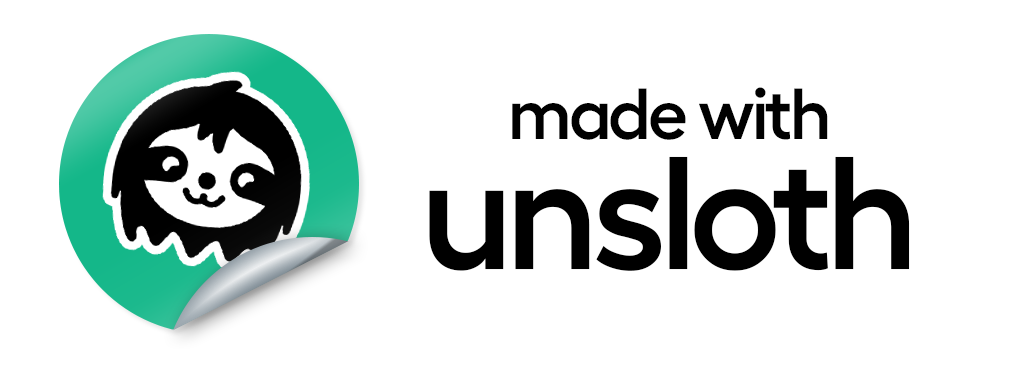
## Training
Qlora finetuning was used.
### Details
- GPU: 1 H100
- Time: ~ 29 hours
### Data
https://huggingface.co/datasets/saucam/sans_data/blob/main/README.md
## 💻 Usage
### Unsloth
```
from unsloth import FastLanguageModel
model, tokenizer = FastLanguageModel.from_pretrained(
model_name = "saucam/Rudra-7b", # YOUR MODEL YOU USED FOR TRAINING
max_seq_length = 2048,
dtype = None,
load_in_4bit = False,
)
FastLanguageModel.for_inference(model) # Enable native 2x faster inference
inputs = tokenizer(
[
"संस्कृतम्"
], return_tensors = "pt").to("cuda")
outputs = model.generate(**inputs, max_new_tokens = 256, use_cache = True, repetition_penalty=1.0, temperature=1.0, )
out = tokenizer.batch_decode(outputs)
print(out)
```
### Transformers
```python
!pip install -qU transformers accelerate
from transformers import AutoTokenizer, AutoModelForCausalLM
import transformers
import torch
model_name = "saucam/Rudra-7b"
tokenizer = AutoTokenizer.from_pretrained(model_name)
model = AutoModelForCausalLM.from_pretrained(model_name)
inputs = tokenizer("संस्कृतम्", return_tensors = "pt")#.to("cuda")
outputs = model.generate(**inputs, max_new_tokens=256, do_sample=True, temperature=0.7, top_k=50, top_p=0.95)
print(tokenizer.decode(outputs[0]))
```
Sample output from above script
```
Gemma's activation function should be approximate GeLU and not exact GeLU.
Changing the activation function to `gelu_pytorch_tanh`.if you want to use the legacy `gelu`, edit the `model.config` to set `hidden_activation=gelu` instead of `hidden_act`. See https://github.com/huggingface/transformers/pull/29402 for more details.
Loading checkpoint shards: 100%|████████████████████████████| 4/4 [00:01<00:00, 2.54it/s]
<bos>संस्कृतम् भारतस्य राष्ट्रभाषा इति भारतसर्वकारस्य 1987तमे वर्षे निर्णयः । प्रायः 125 कोटि जनाः संस्कृतम् एव पठन्ति इति अनुमानम् । संस्कृतम् भारतस्य ध्रुवम् आङ्ग्लानुभाष्यम् । संस्कृतम् अत्यन्तम् प्राचीनम् । संस्कृतम् शैथिल्यात् यदा यदा बहिर्निर्याति तदा तदा एव साम्प्रतकाले संस्कृतेन सह तस्य देशस्य संस्कृतिः सह जगतः संस्कृतिः सह सङ्गच्छति इति । संस्कृतेन सह देशस्य संस्कृतिः सह नगरस्य संस्कृतिः सह क्रीडायाः संस्कृतिः सह राजकीयः, सामाजिकः, सांस्कृतिकः, आर्थिकः, सांविभागिकः, नैतिकः, शिक्षणम्, आवासीयः, साम्प्रदायिकः, धार्मिकः, आध्यात्मिकः, विनोदः, प्रौद्योगिकी, विद्यार्थ
``` |