Spaces:
Runtime error
Runtime error
Update app.py
Browse files
app.py
CHANGED
@@ -1,146 +1,1169 @@
|
|
1 |
import gradio as gr
|
2 |
-
|
3 |
-
|
4 |
-
|
5 |
import torch
|
|
|
|
|
|
|
|
|
|
|
|
|
|
|
|
|
|
|
|
|
|
|
|
|
|
|
|
|
|
|
|
|
|
|
|
|
|
|
|
|
|
|
|
|
|
|
|
|
|
|
|
|
|
|
|
|
|
|
|
|
|
|
|
|
|
|
|
|
|
|
|
|
|
|
|
|
|
|
|
|
|
|
|
|
|
|
|
|
|
|
|
|
|
|
|
|
|
|
|
|
|
|
|
|
|
|
|
|
|
|
|
|
|
|
|
|
|
|
|
|
|
|
|
|
|
|
|
|
|
|
|
|
|
|
|
|
|
|
|
|
|
|
|
|
|
|
|
|
|
|
|
|
|
|
|
|
|
|
|
|
|
|
|
|
|
|
|
|
|
|
|
|
|
|
|
|
|
|
|
|
|
|
|
|
|
|
|
|
|
|
|
|
|
|
|
|
|
|
|
|
|
|
|
|
|
|
|
|
|
|
|
|
|
|
|
|
|
|
|
|
|
|
|
|
|
|
|
|
|
|
|
|
|
|
|
|
|
|
|
|
|
|
|
|
|
|
|
|
|
|
|
|
|
|
|
|
|
|
|
|
|
|
|
|
|
|
|
|
|
|
|
|
|
|
|
|
|
|
|
|
|
|
|
|
|
|
|
|
|
|
|
|
|
|
|
|
|
|
|
|
|
|
|
|
|
|
|
|
|
|
|
|
|
|
|
|
|
|
|
|
|
|
|
|
|
|
|
|
|
|
|
|
|
|
|
|
|
|
|
|
|
|
|
|
|
|
|
|
|
|
|
|
|
|
|
|
|
|
|
|
|
|
|
|
|
|
|
|
|
|
|
|
|
|
|
|
|
|
|
|
|
|
|
|
|
|
|
|
|
|
|
|
|
|
|
|
|
|
|
|
|
|
|
|
|
|
|
|
|
|
|
|
|
|
|
|
|
|
|
|
|
|
|
|
|
|
|
|
|
|
|
|
|
|
|
|
|
|
|
|
|
|
|
|
|
|
|
|
|
|
|
|
|
|
|
|
|
|
|
|
|
|
|
|
|
|
|
|
|
|
|
|
|
|
|
|
|
|
|
|
|
|
|
|
|
|
|
|
|
|
|
|
|
|
|
|
|
|
|
|
|
|
|
|
|
|
|
|
|
|
|
|
|
|
|
|
|
|
|
|
|
|
|
|
|
|
|
|
|
|
|
|
|
|
|
|
|
|
|
|
|
|
|
|
|
|
|
|
|
|
|
|
|
|
|
|
|
|
|
|
|
|
|
|
|
|
|
|
|
|
|
|
|
|
|
|
|
|
|
|
|
|
|
|
|
|
|
|
|
|
|
|
|
|
|
|
|
|
|
|
|
|
|
|
|
|
|
|
|
|
|
|
|
|
|
|
|
|
|
|
|
|
|
|
|
|
|
|
|
|
|
|
|
|
|
|
|
|
|
|
|
|
|
|
|
|
|
|
|
|
|
|
|
|
|
|
|
|
|
|
|
|
|
|
|
|
|
|
|
|
|
|
|
|
|
|
|
|
|
|
|
|
|
|
|
|
|
|
|
|
|
|
|
|
|
|
|
|
|
|
|
|
|
|
|
|
|
|
|
|
|
|
|
|
|
|
|
|
|
|
|
|
|
|
|
|
|
|
|
|
|
|
|
|
|
|
|
|
|
|
|
|
|
|
|
|
|
|
|
|
|
|
|
|
|
|
|
|
|
|
|
|
|
|
|
|
|
|
|
|
|
|
|
|
|
|
|
|
|
|
|
|
|
|
|
|
|
|
|
|
|
|
|
|
|
|
|
|
|
|
|
|
|
|
|
|
|
|
|
|
|
|
|
|
|
|
|
|
|
|
|
|
|
|
|
|
|
|
|
|
|
|
|
|
|
|
|
|
|
|
|
|
|
|
|
|
|
|
|
|
|
|
|
|
|
|
|
|
|
|
|
|
|
|
|
|
|
|
|
|
|
|
|
|
|
|
|
|
|
|
|
|
|
|
|
|
|
|
|
|
|
|
|
|
|
|
|
|
|
|
|
|
|
|
|
|
|
|
|
|
|
|
|
|
|
|
|
|
|
|
|
|
|
|
|
|
|
|
|
|
|
|
|
|
|
|
|
|
|
|
|
|
|
|
|
|
|
|
|
|
|
|
|
|
|
|
|
|
|
|
|
|
|
|
|
|
|
|
|
|
|
|
|
|
|
|
|
|
|
|
|
|
|
|
|
|
|
|
|
|
|
|
|
|
|
|
|
|
|
|
|
|
|
|
|
|
|
|
|
|
|
|
|
|
|
|
|
|
|
|
|
|
|
|
|
|
|
|
|
|
|
|
|
|
|
|
6 |
|
7 |
-
|
8 |
-
|
9 |
-
|
10 |
-
|
11 |
-
|
12 |
-
|
13 |
-
|
14 |
-
|
15 |
-
|
16 |
-
|
17 |
-
|
18 |
-
|
19 |
-
|
20 |
-
|
21 |
-
|
22 |
-
|
23 |
-
|
24 |
-
|
25 |
-
|
26 |
-
|
27 |
-
|
28 |
-
|
29 |
-
|
30 |
-
|
31 |
-
|
32 |
-
|
33 |
-
|
34 |
-
|
35 |
-
|
36 |
-
|
37 |
-
|
38 |
-
|
39 |
-
|
40 |
-
|
41 |
-
|
42 |
-
|
43 |
-
|
44 |
-
]
|
45 |
-
|
46 |
-
|
47 |
-
|
48 |
-
|
49 |
-
|
50 |
-
|
51 |
-
|
52 |
-
|
53 |
-
|
54 |
-
|
55 |
-
|
56 |
-
|
57 |
-
|
58 |
-
|
59 |
-
|
60 |
-
|
61 |
-
|
62 |
-
|
63 |
-
|
64 |
-
|
65 |
-
|
66 |
-
|
67 |
-
|
68 |
-
|
69 |
-
|
70 |
-
|
71 |
-
|
72 |
-
|
73 |
-
|
74 |
-
|
75 |
-
|
76 |
-
|
77 |
-
|
78 |
-
|
79 |
-
|
80 |
-
|
81 |
-
|
82 |
-
|
83 |
-
|
84 |
-
|
85 |
-
|
86 |
-
|
87 |
-
|
88 |
-
|
89 |
-
|
90 |
-
|
91 |
-
|
92 |
-
|
93 |
-
|
94 |
-
|
95 |
-
|
96 |
-
|
97 |
-
|
98 |
-
|
99 |
-
|
100 |
-
|
101 |
-
|
102 |
-
|
103 |
-
|
104 |
-
|
105 |
-
|
106 |
-
|
107 |
-
|
108 |
-
|
109 |
-
|
110 |
-
|
111 |
-
|
112 |
-
|
113 |
-
|
114 |
-
|
115 |
-
|
116 |
-
|
117 |
-
|
118 |
-
|
119 |
-
|
120 |
-
|
121 |
-
|
122 |
-
|
123 |
-
|
124 |
-
|
125 |
-
|
126 |
-
|
127 |
-
|
128 |
-
|
129 |
-
|
130 |
-
|
131 |
-
|
132 |
-
|
133 |
-
|
134 |
-
|
135 |
-
|
136 |
-
|
137 |
-
|
138 |
-
|
139 |
-
|
140 |
-
|
141 |
-
|
142 |
-
|
143 |
-
|
|
|
|
|
|
|
|
|
|
|
|
|
|
|
|
|
|
|
|
|
|
|
|
|
|
|
|
|
|
|
|
|
|
|
|
|
|
|
|
|
|
|
|
|
|
|
|
|
|
|
|
|
|
|
|
|
|
|
|
|
|
|
|
|
|
|
|
|
|
|
|
|
|
|
|
|
|
|
|
|
|
|
|
|
|
|
|
|
|
|
|
|
|
|
|
|
|
|
|
|
|
|
|
|
|
|
|
|
|
|
|
|
|
|
|
|
|
|
|
|
|
|
|
|
|
|
|
|
|
|
|
|
|
|
|
|
|
|
|
|
|
|
|
|
|
|
|
|
|
|
|
|
|
|
|
|
|
|
|
|
|
|
|
|
|
|
|
|
|
|
|
|
|
|
|
|
|
|
|
|
|
|
|
|
|
|
|
|
|
|
|
|
|
|
|
|
|
|
|
|
|
|
|
|
|
|
|
|
|
|
|
|
|
|
|
|
|
|
|
|
|
|
|
|
|
|
|
|
|
|
|
|
|
|
|
|
|
|
|
|
|
|
|
|
|
|
|
|
|
|
|
|
|
|
|
|
|
|
|
|
|
|
|
|
|
|
|
|
|
|
|
|
|
|
|
|
|
|
|
|
|
|
|
|
|
|
|
|
|
|
|
|
|
|
|
|
|
|
|
|
|
|
|
|
|
|
|
|
|
|
|
|
|
|
|
|
|
|
|
|
|
|
|
|
|
|
|
|
|
|
|
|
|
|
|
|
|
|
|
|
|
|
|
|
|
|
|
|
|
|
|
|
|
|
|
|
|
|
|
|
|
|
|
|
|
|
|
|
|
|
|
|
|
|
|
|
|
|
|
|
|
|
|
|
|
|
|
|
|
|
|
|
|
|
|
|
|
|
|
|
|
|
|
|
|
|
|
|
|
|
|
|
|
|
|
|
|
|
|
|
|
|
|
|
|
|
|
|
|
|
|
|
|
|
|
|
|
|
|
|
|
|
|
|
|
|
|
|
|
|
|
|
|
|
|
|
|
|
|
|
|
|
|
|
|
|
|
|
|
|
|
|
|
|
|
|
|
|
|
|
|
|
|
|
|
|
|
|
|
|
|
|
|
|
|
|
|
|
|
|
|
|
|
|
|
|
|
|
|
|
|
|
|
|
|
|
|
|
|
|
|
|
|
|
|
|
|
|
|
|
|
|
|
|
|
|
|
|
|
|
|
|
|
|
|
|
|
|
|
|
|
|
|
|
|
|
|
|
|
|
|
|
|
|
|
|
|
|
|
|
|
|
|
|
|
|
|
|
|
|
|
|
|
|
|
|
|
|
|
|
|
|
|
|
|
|
|
|
|
|
|
|
|
|
|
|
|
|
|
|
|
|
|
|
|
|
|
|
|
|
|
|
|
|
|
|
|
|
|
|
|
|
|
|
|
|
|
|
|
|
|
|
|
|
|
|
|
|
|
|
|
|
|
|
|
|
|
|
|
|
|
|
|
|
|
|
|
|
|
|
|
|
|
|
144 |
)
|
|
|
|
|
|
|
|
|
|
|
|
|
|
|
|
|
|
|
|
|
|
|
|
|
|
|
|
|
|
|
|
|
|
|
|
|
|
|
|
|
|
|
|
|
|
|
|
|
|
|
|
|
|
|
|
|
|
|
|
|
|
|
|
|
|
|
|
|
|
|
|
|
|
|
|
|
|
|
|
|
|
|
|
|
|
|
|
|
|
|
|
|
|
|
|
|
|
|
|
|
|
|
|
|
|
|
|
|
|
|
|
|
|
|
|
|
|
|
|
|
|
|
|
|
|
|
|
|
|
|
|
|
|
|
|
|
|
|
|
|
|
|
|
|
|
|
|
|
|
|
|
|
|
|
|
|
|
|
|
|
|
|
|
|
|
|
|
|
|
|
|
|
|
|
|
|
|
|
|
|
|
|
|
|
|
|
|
|
|
|
|
|
|
|
|
|
|
|
|
|
|
|
|
|
|
|
|
|
|
|
|
|
|
|
|
|
|
|
|
|
|
|
|
|
|
|
|
|
|
|
|
|
|
|
|
|
|
|
|
|
|
|
|
|
|
|
|
|
|
|
|
|
|
|
|
|
|
|
|
|
|
|
|
|
|
|
|
|
|
|
|
|
|
|
|
|
|
|
|
|
|
|
|
|
|
|
|
|
|
|
|
|
|
|
|
|
|
|
|
|
|
|
|
|
|
|
|
|
|
|
|
|
|
|
|
|
|
|
|
|
|
|
|
|
|
|
|
|
|
|
|
|
|
|
|
|
145 |
|
146 |
-
|
|
|
1 |
import gradio as gr
|
2 |
+
from base64 import b64encode
|
3 |
+
|
4 |
+
import numpy
|
5 |
import torch
|
6 |
+
from diffusers import AutoencoderKL, LMSDiscreteScheduler, UNet2DConditionModel
|
7 |
+
|
8 |
+
|
9 |
+
|
10 |
+
from PIL import Image
|
11 |
+
from torch import autocast
|
12 |
+
from torchvision import transforms as tfms
|
13 |
+
from tqdm.auto import tqdm
|
14 |
+
from transformers import CLIPTextModel, CLIPTokenizer, logging
|
15 |
+
import torchvision.transforms as T
|
16 |
+
|
17 |
+
torch.manual_seed(1)
|
18 |
+
|
19 |
+
|
20 |
+
# Supress some unnecessary warnings when loading the CLIPTextModel
|
21 |
+
logging.set_verbosity_error()
|
22 |
+
|
23 |
+
torch_device = "cpu"
|
24 |
+
t
|
25 |
+
|
26 |
+
# Load the autoencoder model which will be used to decode the latents into image space.
|
27 |
+
vae = AutoencoderKL.from_pretrained("CompVis/stable-diffusion-v1-4", subfolder="vae")
|
28 |
+
|
29 |
+
# Load the tokenizer and text encoder to tokenize and encode the text.
|
30 |
+
tokenizer = CLIPTokenizer.from_pretrained("openai/clip-vit-large-patch14")
|
31 |
+
text_encoder = CLIPTextModel.from_pretrained("openai/clip-vit-large-patch14")
|
32 |
+
|
33 |
+
# The UNet model for generating the latents.
|
34 |
+
unet = UNet2DConditionModel.from_pretrained("CompVis/stable-diffusion-v1-4", subfolder="unet")
|
35 |
+
|
36 |
+
# The noise scheduler
|
37 |
+
scheduler = LMSDiscreteScheduler(beta_start=0.00085, beta_end=0.012, beta_schedule="scaled_linear", num_train_timesteps=1000)
|
38 |
+
|
39 |
+
# To the GPU we go!
|
40 |
+
vae = vae.to(torch_device)
|
41 |
+
text_encoder = text_encoder.to(torch_device)
|
42 |
+
unet = unet.to(torch_device);
|
43 |
+
|
44 |
+
"""## A diffusion loop
|
45 |
+
|
46 |
+
If all you want is to make a picture with some text, you could ignore this notebook and use one of the existing tools (such as [DreamStudio](https://beta.dreamstudio.ai/)) or use the simplified pipeline from huggingface, as documented [here](https://huggingface.co/blog/stable_diffusion).
|
47 |
+
|
48 |
+
What we want to do in this notebook is dig a little deeper into how this works, so we'll start by checking that the example code runs. Again, this is adapted from the [HF notebook](https://colab.research.google.com/github/huggingface/notebooks/blob/main/diffusers/stable_diffusion.ipynb) and looks very similar to what you'll find if you inspect [the `__call__()` method of the stable diffusion pipeline](https://github.com/huggingface/diffusers/blob/main/src/diffusers/pipelines/stable_diffusion/pipeline_stable_diffusion.py#L200).
|
49 |
+
"""
|
50 |
+
|
51 |
+
# Some settings
|
52 |
+
prompt = ["A watercolor painting of an otter"]
|
53 |
+
height = 512 # default height of Stable Diffusion
|
54 |
+
width = 512 # default width of Stable Diffusion
|
55 |
+
num_inference_steps = 30 # Number of denoising steps
|
56 |
+
guidance_scale = 7.5 # Scale for classifier-free guidance
|
57 |
+
generator = torch.manual_seed(32) # Seed generator to create the inital latent noise
|
58 |
+
batch_size = 1
|
59 |
+
|
60 |
+
# Prep text
|
61 |
+
text_input = tokenizer(prompt, padding="max_length", max_length=tokenizer.model_max_length, truncation=True, return_tensors="pt")
|
62 |
+
with torch.no_grad():
|
63 |
+
text_embeddings = text_encoder(text_input.input_ids.to(torch_device))[0]
|
64 |
+
max_length = text_input.input_ids.shape[-1]
|
65 |
+
uncond_input = tokenizer(
|
66 |
+
[""] * batch_size, padding="max_length", max_length=max_length, return_tensors="pt"
|
67 |
+
)
|
68 |
+
with torch.no_grad():
|
69 |
+
uncond_embeddings = text_encoder(uncond_input.input_ids.to(torch_device))[0]
|
70 |
+
text_embeddings = torch.cat([uncond_embeddings, text_embeddings])
|
71 |
+
|
72 |
+
# Prep Scheduler
|
73 |
+
def set_timesteps(scheduler, num_inference_steps):
|
74 |
+
scheduler.set_timesteps(num_inference_steps)
|
75 |
+
scheduler.timesteps = scheduler.timesteps.to(torch.float32) # minor fix to ensure MPS compatibility, fixed in diffusers PR 3925
|
76 |
+
|
77 |
+
set_timesteps(scheduler,num_inference_steps)
|
78 |
+
|
79 |
+
# Prep latents
|
80 |
+
latents = torch.randn(
|
81 |
+
(batch_size, unet.in_channels, height // 8, width // 8),
|
82 |
+
generator=generator,
|
83 |
+
)
|
84 |
+
latents = latents.to(torch_device)
|
85 |
+
latents = latents * scheduler.init_noise_sigma # Scaling (previous versions did latents = latents * self.scheduler.sigmas[0]
|
86 |
+
|
87 |
+
# Loop
|
88 |
+
with autocast("cuda"): # will fallback to CPU if no CUDA; no autocast for MPS
|
89 |
+
for i, t in tqdm(enumerate(scheduler.timesteps), total=len(scheduler.timesteps)):
|
90 |
+
# expand the latents if we are doing classifier-free guidance to avoid doing two forward passes.
|
91 |
+
latent_model_input = torch.cat([latents] * 2)
|
92 |
+
sigma = scheduler.sigmas[i]
|
93 |
+
# Scale the latents (preconditioning):
|
94 |
+
# latent_model_input = latent_model_input / ((sigma**2 + 1) ** 0.5) # Diffusers 0.3 and below
|
95 |
+
latent_model_input = scheduler.scale_model_input(latent_model_input, t)
|
96 |
+
|
97 |
+
# predict the noise residual
|
98 |
+
with torch.no_grad():
|
99 |
+
noise_pred = unet(latent_model_input, t, encoder_hidden_states=text_embeddings).sample
|
100 |
+
|
101 |
+
# perform guidance
|
102 |
+
noise_pred_uncond, noise_pred_text = noise_pred.chunk(2)
|
103 |
+
noise_pred = noise_pred_uncond + guidance_scale * (noise_pred_text - noise_pred_uncond)
|
104 |
+
|
105 |
+
# compute the previous noisy sample x_t -> x_t-1
|
106 |
+
# latents = scheduler.step(noise_pred, i, latents)["prev_sample"] # Diffusers 0.3 and below
|
107 |
+
latents = scheduler.step(noise_pred, t, latents).prev_sample
|
108 |
+
|
109 |
+
# scale and decode the image latents with vae
|
110 |
+
latents = 1 / 0.18215 * latents
|
111 |
+
with torch.no_grad():
|
112 |
+
image = vae.decode(latents).sample
|
113 |
+
|
114 |
+
# Display
|
115 |
+
image = (image / 2 + 0.5).clamp(0, 1)
|
116 |
+
image = image.detach().cpu().permute(0, 2, 3, 1).numpy()
|
117 |
+
images = (image * 255).round().astype("uint8")
|
118 |
+
pil_images = [Image.fromarray(image) for image in images]
|
119 |
+
pil_images[0]
|
120 |
+
|
121 |
+
"""It's working, but that's quite a bit of code! Let's look at the components one by one.
|
122 |
+
|
123 |
+
## The Autoencoder (AE)
|
124 |
+
|
125 |
+
The AE can 'encode' an image into some sort of latent representation, and decode this back into an image. I've wrapped the code for this into a couple of functions here so we can see what this looks like in action:
|
126 |
+
"""
|
127 |
+
|
128 |
+
def pil_to_latent(input_im):
|
129 |
+
# Single image -> single latent in a batch (so size 1, 4, 64, 64)
|
130 |
+
with torch.no_grad():
|
131 |
+
latent = vae.encode(tfms.ToTensor()(input_im).unsqueeze(0).to(torch_device)*2-1) # Note scaling
|
132 |
+
return 0.18215 * latent.latent_dist.sample()
|
133 |
+
|
134 |
+
def latents_to_pil(latents):
|
135 |
+
# bath of latents -> list of images
|
136 |
+
latents = (1 / 0.18215) * latents
|
137 |
+
with torch.no_grad():
|
138 |
+
image = vae.decode(latents).sample
|
139 |
+
image = (image / 2 + 0.5).clamp(0, 1)
|
140 |
+
image = image.detach().cpu().permute(0, 2, 3, 1).numpy()
|
141 |
+
images = (image * 255).round().astype("uint8")
|
142 |
+
pil_images = [Image.fromarray(image) for image in images]
|
143 |
+
return pil_images
|
144 |
+
|
145 |
+
"""We'll use a pic from the web here, but you can load your own instead by uploading it and editing the filename in the next cell."""
|
146 |
+
|
147 |
+
# Download a demo Image
|
148 |
+
!curl --output macaw.jpg 'https://lafeber.com/pet-birds/wp-content/uploads/2018/06/Scarlet-Macaw-2.jpg'
|
149 |
+
|
150 |
+
# Load the image with PIL
|
151 |
+
input_image = Image.open('macaw.jpg').resize((512, 512))
|
152 |
+
input_image
|
153 |
+
|
154 |
+
"""Encoding this into the latent space of the AE with the function defined above looks like this:"""
|
155 |
+
|
156 |
+
# Encode to the latent space
|
157 |
+
encoded = pil_to_latent(input_image)
|
158 |
+
encoded.shape
|
159 |
+
|
160 |
+
# Let's visualize the four channels of this latent representation:
|
161 |
+
fig, axs = plt.subplots(1, 4, figsize=(16, 4))
|
162 |
+
for c in range(4):
|
163 |
+
axs[c].imshow(encoded[0][c].cpu(), cmap='Greys')
|
164 |
+
|
165 |
+
"""This 4x64x64 tensor captures lots of information about the image, hopefully enough that when we feed it through the decoder we get back something very close to our input image:"""
|
166 |
+
|
167 |
+
# Decode this latent representation back into an image
|
168 |
+
decoded = latents_to_pil(encoded)[0]
|
169 |
+
decoded
|
170 |
+
|
171 |
+
"""You'll see some small differences if you squint! Forcus on the eye if you can't see anything obvious. This is pretty impressive - that 4x64x64 latent seems to hold a lot more information that a 64px image...
|
172 |
+
|
173 |
+
This autoencoder has been trained to squish down an image to a smaller representation and then re-create the image back from this compressed version again.
|
174 |
+
|
175 |
+
In this particular case the compression factor is 48, we start with a 3x512x512(chxhtxwd) image and it get compressed to a latent vector 4x64x64. Each 3x8x8 pixel volume in the input image gets compressed down to just 4 numbers(4x1x1). You can find AEs with a higher compression ratio (eg f16 like some popular VQGAN models) but at some point they begin to introduce artifacts that we don't want.
|
176 |
+
|
177 |
+
Why do we even use an autoencoder? We can do diffusion in pixel space - where the model gets all the image data as inputs and produces an output prediction of the same shape. But this means processing a LOT of data, and make high-resolution generation very computationally expensive. Some solutions to this involve doing diffusion at low resolution (64px for eg) and then training a separate model to upscale repeatedly (as with D2/Imagen). But latent diffusion instead does the diffusion process in this 'latent space', using the compressed representations from our AE rather than raw images. These representations are information rich, and can be small enough to handle manageably on consumer hardware. Once we've generated a new 'image' as a latent representation, the autoencoder can take those final latent outputs and turn them into actual pixels.
|
178 |
+
|
179 |
+
# The Scheduler
|
180 |
+
Now we need to talk about adding noise...
|
181 |
+
|
182 |
+
During training, we add some noise to an image an then have the model try to predict the noise. If we always added a ton of noise, the model might not have much to work with. If we only add a tiny amount, the model won't be able to do much with the random starting points we use for sampling. So during training the amount is varied, according to some distribution.
|
183 |
+
|
184 |
+
During sampling, we want to 'denoise' over a number of steps. How many steps and how much noise we should aim for at each step are going to affect the final result.
|
185 |
+
|
186 |
+
The scheduler is in charge of handling all of these details. For example: `scheduler = LMSDiscreteScheduler(beta_start=0.00085, beta_end=0.012, beta_schedule="scaled_linear", num_train_timesteps=1000)` sets up a scheduler that matches the one used to train this model. When we want to sample over a smaller number of steps, we set this up with `scheduler.set_timesteps`:
|
187 |
+
"""
|
188 |
+
|
189 |
+
# Setting the number of sampling steps:
|
190 |
+
set_timesteps(scheduler, 15)
|
191 |
+
|
192 |
+
"""You can see how our new set of steps corresponds to those used in training:"""
|
193 |
+
|
194 |
+
# See these in terms of the original 1000 steps used for training:
|
195 |
+
print(scheduler.timesteps)
|
196 |
+
|
197 |
+
"""And how much noise is present at each:"""
|
198 |
+
|
199 |
+
# Look at the equivalent noise levels:
|
200 |
+
print(scheduler.sigmas)
|
201 |
+
|
202 |
+
"""During sampling, we'll start at a high noise level (in fact, our input will be pure noise) and gradually 'denoise' down to an image, according to this schedule."""
|
203 |
+
|
204 |
+
# Plotting this noise schedule:
|
205 |
+
plt.plot(scheduler.sigmas)
|
206 |
+
plt.title('Noise Schedule')
|
207 |
+
plt.xlabel('Sampling step')
|
208 |
+
plt.ylabel('sigma')
|
209 |
+
plt.show()
|
210 |
+
|
211 |
+
# TODO maybe show timestep as well
|
212 |
+
|
213 |
+
"""This 'sigma' is the amount of noise added to the latent representation. Let's visualize what this looks like by adding a bit of noise to our encoded image and then decoding this noised version:"""
|
214 |
+
|
215 |
+
noise = torch.randn_like(encoded) # Random noise
|
216 |
+
sampling_step = 10 # Equivalent to step 10 out of 15 in the schedule above
|
217 |
+
# encoded_and_noised = scheduler.add_noise(encoded, noise, timestep) # Diffusers 0.3 and below
|
218 |
+
encoded_and_noised = scheduler.add_noise(encoded, noise, timesteps=torch.tensor([scheduler.timesteps[sampling_step]]))
|
219 |
+
latents_to_pil(encoded_and_noised.float())[0] # Display
|
220 |
+
|
221 |
+
"""What does this look like at different timesteps? Experiment and see for yourself!
|
222 |
+
|
223 |
+
If you uncomment the cell below you'll see that in this case the `scheduler.add_noise` function literally just adds noise scaled by sigma: `noisy_samples = original_samples + noise * sigmas`
|
224 |
+
"""
|
225 |
+
|
226 |
+
# ??scheduler.add_noise
|
227 |
+
|
228 |
+
"""Other diffusion models may be trained with different noising and scheduling approaches, some of which keep the variance fairly constant across noise levels ('variance preserving') with different scaling and mixing tricks instead of having noisy latents with higher and higher variance as more noise is added ('variance exploding').
|
229 |
+
|
230 |
+
If we want to start from random noise instead of a noised image, we need to scale it by the largest sigma value used during training, ~14 in this case. And before these noisy latents are fed to the model they are scaled again in the so-called pre-conditioning step:
|
231 |
+
`latent_model_input = latent_model_input / ((sigma**2 + 1) ** 0.5)` (now handled by `latent_model_input = scheduler.scale_model_input(latent_model_input, t)`).
|
232 |
+
|
233 |
+
Again, this scaling/pre-conditioning differs between papers and implementations, so keep an eye out for this if you work with a different type of diffusion model.
|
234 |
+
|
235 |
+
## Loop starting from noised version of input (AKA image2image)
|
236 |
+
|
237 |
+
Let's see what happens when we use our image as a starting point, adding some noise and then doing the final few denoising steps in the loop with a new prompt.
|
238 |
+
|
239 |
+
We'll use a similar loop to the first demo, but we'll skip the first `start_step` steps.
|
240 |
+
|
241 |
+
To noise our image we'll use code like that shown above, using the scheduler to noise it to a level equivalent to step 10 (`start_step`).
|
242 |
+
"""
|
243 |
+
|
244 |
+
# Settings (same as before except for the new prompt)
|
245 |
+
prompt = ["A colorful dancer, nat geo photo"]
|
246 |
+
height = 512 # default height of Stable Diffusion
|
247 |
+
width = 512 # default width of Stable Diffusion
|
248 |
+
num_inference_steps = 50 # Number of denoising steps
|
249 |
+
guidance_scale = 8 # Scale for classifier-free guidance
|
250 |
+
generator = torch.manual_seed(32) # Seed generator to create the inital latent noise
|
251 |
+
batch_size = 1
|
252 |
+
|
253 |
+
# Prep text (same as before)
|
254 |
+
text_input = tokenizer(prompt, padding="max_length", max_length=tokenizer.model_max_length, truncation=True, return_tensors="pt")
|
255 |
+
with torch.no_grad():
|
256 |
+
text_embeddings = text_encoder(text_input.input_ids.to(torch_device))[0]
|
257 |
+
max_length = text_input.input_ids.shape[-1]
|
258 |
+
uncond_input = tokenizer(
|
259 |
+
[""] * batch_size, padding="max_length", max_length=max_length, return_tensors="pt"
|
260 |
+
)
|
261 |
+
with torch.no_grad():
|
262 |
+
uncond_embeddings = text_encoder(uncond_input.input_ids.to(torch_device))[0]
|
263 |
+
text_embeddings = torch.cat([uncond_embeddings, text_embeddings])
|
264 |
+
|
265 |
+
# Prep Scheduler (setting the number of inference steps)
|
266 |
+
set_timesteps(scheduler, num_inference_steps)
|
267 |
+
|
268 |
+
# Prep latents (noising appropriately for start_step)
|
269 |
+
start_step = 10
|
270 |
+
start_sigma = scheduler.sigmas[start_step]
|
271 |
+
noise = torch.randn_like(encoded)
|
272 |
+
latents = scheduler.add_noise(encoded, noise, timesteps=torch.tensor([scheduler.timesteps[start_step]]))
|
273 |
+
latents = latents.to(torch_device).float()
|
274 |
+
|
275 |
+
# Loop
|
276 |
+
for i, t in tqdm(enumerate(scheduler.timesteps), total=len(scheduler.timesteps)):
|
277 |
+
if i >= start_step: # << This is the only modification to the loop we do
|
278 |
+
|
279 |
+
# expand the latents if we are doing classifier-free guidance to avoid doing two forward passes.
|
280 |
+
latent_model_input = torch.cat([latents] * 2)
|
281 |
+
sigma = scheduler.sigmas[i]
|
282 |
+
latent_model_input = scheduler.scale_model_input(latent_model_input, t)
|
283 |
+
|
284 |
+
# predict the noise residual
|
285 |
+
with torch.no_grad():
|
286 |
+
noise_pred = unet(latent_model_input, t, encoder_hidden_states=text_embeddings)["sample"]
|
287 |
+
|
288 |
+
# perform guidance
|
289 |
+
noise_pred_uncond, noise_pred_text = noise_pred.chunk(2)
|
290 |
+
noise_pred = noise_pred_uncond + guidance_scale * (noise_pred_text - noise_pred_uncond)
|
291 |
+
|
292 |
+
# compute the previous noisy sample x_t -> x_t-1
|
293 |
+
latents = scheduler.step(noise_pred, t, latents).prev_sample
|
294 |
+
|
295 |
+
latents_to_pil(latents)[0]
|
296 |
+
|
297 |
+
"""You can see that some colours and structure from the image are kept, but we now have a new picture! The more noise you add and the more steps you do, the further away it gets from the input image.
|
298 |
+
|
299 |
+
This is how the popular img2img pipeline works. Again, if this is your end goal there are tools to make this easy!
|
300 |
+
|
301 |
+
But you can see that under the hood this is the same as the generation loop just skipping the first few steps and starting from a noised image rather than pure noise.
|
302 |
+
|
303 |
+
Explore changing how many steps are skipped and see how this affects the amount the image changes from the input.
|
304 |
+
|
305 |
+
## Exploring the text -> embedding pipeline
|
306 |
+
|
307 |
+
We use a text encoder model to turn our text into a set of 'embeddings' which are fed to the diffusion model as conditioning. Let's follow a piece of text through this process and see how it works.
|
308 |
+
"""
|
309 |
+
|
310 |
+
# Our text prompt
|
311 |
+
prompt = 'A picture of a puppy'
|
312 |
+
|
313 |
+
"""We begin with tokenization:"""
|
314 |
+
|
315 |
+
# Turn the text into a sequnce of tokens:
|
316 |
+
text_input = tokenizer(prompt, padding="max_length", max_length=tokenizer.model_max_length, truncation=True, return_tensors="pt")
|
317 |
+
text_input['input_ids'][0] # View the tokens
|
318 |
+
|
319 |
+
# See the individual tokens
|
320 |
+
for t in text_input['input_ids'][0][:8]: # We'll just look at the first 7 to save you from a wall of '<|endoftext|>'
|
321 |
+
print(t, tokenizer.decoder.get(int(t)))
|
322 |
+
|
323 |
+
# TODO call out that 6829 is puppy
|
324 |
+
|
325 |
+
"""We can jump straight to the final (output) embeddings like so:"""
|
326 |
+
|
327 |
+
# Grab the output embeddings
|
328 |
+
output_embeddings = text_encoder(text_input.input_ids.to(torch_device))[0]
|
329 |
+
print('Shape:', output_embeddings.shape)
|
330 |
+
output_embeddings
|
331 |
+
|
332 |
+
"""We pass our tokens through the text_encoder and we magically get some numbers we can feed to the model.
|
333 |
+
|
334 |
+
How are these generated? The tokens are transformed into a set of input embeddings, which are then fed through the transformer model to get the final output embeddings.
|
335 |
+
|
336 |
+
To get these input embeddings, there are actually two steps - as revealed by inspecting `text_encoder.text_model.embeddings`:
|
337 |
+
"""
|
338 |
+
|
339 |
+
text_encoder.text_model.embeddings
|
340 |
+
|
341 |
+
"""### Token embeddings
|
342 |
+
|
343 |
+
The token is fed to the `token_embedding` to transform it into a vector. The function name `get_input_embeddings` here is misleading since these token embeddings need to be combined with the position embeddings before they are actually used as inputs to the model! Anyway, let's look at just the token embedding part first
|
344 |
+
|
345 |
+
We can look at the embedding layer:
|
346 |
+
"""
|
347 |
+
|
348 |
+
# Access the embedding layer
|
349 |
+
token_emb_layer = text_encoder.text_model.embeddings.token_embedding
|
350 |
+
token_emb_layer # Vocab size 49408, emb_dim 768
|
351 |
+
|
352 |
+
"""And embed a token like so:"""
|
353 |
+
|
354 |
+
# Embed a token - in this case the one for 'puppy'
|
355 |
+
embedding = token_emb_layer(torch.tensor(6829, device=torch_device))
|
356 |
+
embedding.shape # 768-dim representation
|
357 |
+
|
358 |
+
"""This single token has been mapped to a 768-dimensional vector - the token embedding.
|
359 |
+
|
360 |
+
We can do the same with all of the tokens in the prompt to get all the token embeddings:
|
361 |
+
"""
|
362 |
+
|
363 |
+
token_embeddings = token_emb_layer(text_input.input_ids.to(torch_device))
|
364 |
+
print(token_embeddings.shape) # batch size 1, 77 tokens, 768 values for each
|
365 |
+
token_embeddings
|
366 |
+
|
367 |
+
"""### Positional Embeddings
|
368 |
+
|
369 |
+
Positional embeddings tell the model where in a sequence a token is. Much like the token embedding, this is a set of (optionally learnable) parameters. But now instead of dealing with ~50k tokens we just need one for each position (77 total):
|
370 |
+
"""
|
371 |
+
|
372 |
+
pos_emb_layer = text_encoder.text_model.embeddings.position_embedding
|
373 |
+
pos_emb_layer
|
374 |
+
|
375 |
+
"""We can get the positional embedding for each position:"""
|
376 |
+
|
377 |
+
position_ids = text_encoder.text_model.embeddings.position_ids[:, :77]
|
378 |
+
position_embeddings = pos_emb_layer(position_ids)
|
379 |
+
print(position_embeddings.shape)
|
380 |
+
position_embeddings
|
381 |
+
|
382 |
+
"""### Combining token and position embeddings
|
383 |
+
|
384 |
+
Time to combine the two. How do we do this? Just add them! Other approaches are possible but for this model this is how it is done.
|
385 |
+
|
386 |
+
Combining them in this way gives us the final input embeddings ready to feed through the transformer model:
|
387 |
+
"""
|
388 |
+
|
389 |
+
# And combining them we get the final input embeddings
|
390 |
+
input_embeddings = token_embeddings + position_embeddings
|
391 |
+
print(input_embeddings.shape)
|
392 |
+
input_embeddings
|
393 |
+
|
394 |
+
"""We can check that these are the same as the result we'd get from `text_encoder.text_model.embeddings`:"""
|
395 |
+
|
396 |
+
# The following combines all the above steps (but doesn't let us fiddle with them!)
|
397 |
+
text_encoder.text_model.embeddings(text_input.input_ids.to(torch_device))
|
398 |
+
|
399 |
+
"""### Feeding these through the transformer model
|
400 |
+
|
401 |
+

|
402 |
+
|
403 |
+
We want to mess with these input embeddings (specifically the token embeddings) before we send them through the rest of the model, but first we should check that we know how to do that. I read the code of the text_encoders `forward` method, and based on that the code for the `forward` method of the text_model that the text_encoder wraps. To inspect it yourself, type `??text_encoder.text_model.forward` and you'll get the function info and source code - a useful debugging trick!
|
404 |
+
|
405 |
+
Anyway, based on that we can copy in the bits we need to get the so-called 'last hidden state' and thus generate our final embeddings:
|
406 |
+
"""
|
407 |
+
|
408 |
+
def get_output_embeds(input_embeddings):
|
409 |
+
# CLIP's text model uses causal mask, so we prepare it here:
|
410 |
+
bsz, seq_len = input_embeddings.shape[:2]
|
411 |
+
causal_attention_mask = text_encoder.text_model._build_causal_attention_mask(bsz, seq_len, dtype=input_embeddings.dtype)
|
412 |
+
|
413 |
+
# Getting the output embeddings involves calling the model with passing output_hidden_states=True
|
414 |
+
# so that it doesn't just return the pooled final predictions:
|
415 |
+
encoder_outputs = text_encoder.text_model.encoder(
|
416 |
+
inputs_embeds=input_embeddings,
|
417 |
+
attention_mask=None, # We aren't using an attention mask so that can be None
|
418 |
+
causal_attention_mask=causal_attention_mask.to(torch_device),
|
419 |
+
output_attentions=None,
|
420 |
+
output_hidden_states=True, # We want the output embs not the final output
|
421 |
+
return_dict=None,
|
422 |
+
)
|
423 |
+
|
424 |
+
# We're interested in the output hidden state only
|
425 |
+
output = encoder_outputs[0]
|
426 |
+
|
427 |
+
# There is a final layer norm we need to pass these through
|
428 |
+
output = text_encoder.text_model.final_layer_norm(output)
|
429 |
+
|
430 |
+
# And now they're ready!
|
431 |
+
return output
|
432 |
+
|
433 |
+
out_embs_test = get_output_embeds(input_embeddings) # Feed through the model with our new function
|
434 |
+
print(out_embs_test.shape) # Check the output shape
|
435 |
+
out_embs_test # Inspect the output
|
436 |
+
|
437 |
+
"""Note that these match the `output_embeddings` we saw near the start - we've figured out how to split up that one step ("get the text embeddings") into multiple sub-steps ready for us to modify.
|
438 |
+
|
439 |
+
Now that we have this process in place, we can replace the input embedding of a token with a new one of our choice - which in our final use-case will be something we learn. To demonstrate the concept though, let's replace the input embedding for 'puppy' in the prompt we've been playing with with the embedding for token 2368, get a new set of output embeddings based on this, and use these to generate an image to see what we get:
|
440 |
+
"""
|
441 |
+
|
442 |
+
prompt = 'A picture of a puppy'
|
443 |
+
|
444 |
+
# Tokenize
|
445 |
+
text_input = tokenizer(prompt, padding="max_length", max_length=tokenizer.model_max_length, truncation=True, return_tensors="pt")
|
446 |
+
input_ids = text_input.input_ids.to(torch_device)
|
447 |
+
|
448 |
+
# Get token embeddings
|
449 |
+
token_embeddings = token_emb_layer(input_ids)
|
450 |
+
|
451 |
+
# The new embedding. In this case just the input embedding of token 2368...
|
452 |
+
replacement_token_embedding = text_encoder.get_input_embeddings()(torch.tensor(2368, device=torch_device))
|
453 |
+
|
454 |
+
# Insert this into the token embeddings (
|
455 |
+
token_embeddings[0, torch.where(input_ids[0]==6829)] = replacement_token_embedding.to(torch_device)
|
456 |
+
|
457 |
+
# Combine with pos embs
|
458 |
+
input_embeddings = token_embeddings + position_embeddings
|
459 |
+
|
460 |
+
# Feed through to get final output embs
|
461 |
+
modified_output_embeddings = get_output_embeds(input_embeddings)
|
462 |
+
|
463 |
+
print(modified_output_embeddings.shape)
|
464 |
+
modified_output_embeddings
|
465 |
+
|
466 |
+
"""The first few are the same, the last aren't. Everything at and after the position of the token we're replacing will be affected.
|
467 |
+
|
468 |
+
If all went well, we should see something other than a puppy when we use these to generate an image. And sure enough, we do!
|
469 |
+
"""
|
470 |
+
|
471 |
+
#Generating an image with these modified embeddings
|
472 |
+
|
473 |
+
def generate_with_embs(text_embeddings):
|
474 |
+
height = 512 # default height of Stable Diffusion
|
475 |
+
width = 512 # default width of Stable Diffusion
|
476 |
+
num_inference_steps = 30 # Number of denoising steps
|
477 |
+
guidance_scale = 7.5 # Scale for classifier-free guidance
|
478 |
+
generator = torch.manual_seed(32) # Seed generator to create the inital latent noise
|
479 |
+
batch_size = 1
|
480 |
+
|
481 |
+
max_length = text_input.input_ids.shape[-1]
|
482 |
+
uncond_input = tokenizer(
|
483 |
+
[""] * batch_size, padding="max_length", max_length=max_length, return_tensors="pt"
|
484 |
+
)
|
485 |
+
with torch.no_grad():
|
486 |
+
uncond_embeddings = text_encoder(uncond_input.input_ids.to(torch_device))[0]
|
487 |
+
text_embeddings = torch.cat([uncond_embeddings, text_embeddings])
|
488 |
+
|
489 |
+
# Prep Scheduler
|
490 |
+
set_timesteps(scheduler, num_inference_steps)
|
491 |
+
|
492 |
+
# Prep latents
|
493 |
+
latents = torch.randn(
|
494 |
+
(batch_size, unet.in_channels, height // 8, width // 8),
|
495 |
+
generator=generator,
|
496 |
+
)
|
497 |
+
latents = latents.to(torch_device)
|
498 |
+
latents = latents * scheduler.init_noise_sigma
|
499 |
+
|
500 |
+
# Loop
|
501 |
+
for i, t in tqdm(enumerate(scheduler.timesteps), total=len(scheduler.timesteps)):
|
502 |
+
# expand the latents if we are doing classifier-free guidance to avoid doing two forward passes.
|
503 |
+
latent_model_input = torch.cat([latents] * 2)
|
504 |
+
sigma = scheduler.sigmas[i]
|
505 |
+
latent_model_input = scheduler.scale_model_input(latent_model_input, t)
|
506 |
+
|
507 |
+
# predict the noise residual
|
508 |
+
with torch.no_grad():
|
509 |
+
noise_pred = unet(latent_model_input, t, encoder_hidden_states=text_embeddings)["sample"]
|
510 |
+
|
511 |
+
# perform guidance
|
512 |
+
noise_pred_uncond, noise_pred_text = noise_pred.chunk(2)
|
513 |
+
noise_pred = noise_pred_uncond + guidance_scale * (noise_pred_text - noise_pred_uncond)
|
514 |
+
|
515 |
+
# compute the previous noisy sample x_t -> x_t-1
|
516 |
+
latents = scheduler.step(noise_pred, t, latents).prev_sample
|
517 |
+
|
518 |
+
return latents_to_pil(latents)[0]
|
519 |
+
|
520 |
+
#Generating an image with these modified embeddings
|
521 |
+
|
522 |
+
def generate_with_embs_seed(text_embeddings, seed, max_length):
|
523 |
+
"""
|
524 |
|
525 |
+
Args:
|
526 |
+
text_embeddings:
|
527 |
+
seed:
|
528 |
+
max_length:
|
529 |
+
|
530 |
+
Returns:
|
531 |
+
|
532 |
+
"""
|
533 |
+
height = 512 # default height of Stable Diffusion
|
534 |
+
width = 512 # default width of Stable Diffusion
|
535 |
+
num_inference_steps = 30 # Number of denoising steps
|
536 |
+
guidance_scale = 7.5 # Scale for classifier-free guidance
|
537 |
+
generator = torch.manual_seed(32) # Seed generator to create the inital latent noise
|
538 |
+
batch_size = 1
|
539 |
+
|
540 |
+
# max_length = text_input.input_ids.shape[-1]
|
541 |
+
uncond_input = tokenizer(
|
542 |
+
[""] * batch_size, padding="max_length", max_length=max_length, return_tensors="pt"
|
543 |
+
)
|
544 |
+
with torch.no_grad():
|
545 |
+
uncond_embeddings = text_encoder(uncond_input.input_ids.to(torch_device))[0]
|
546 |
+
text_embeddings = torch.cat([uncond_embeddings, text_embeddings])
|
547 |
+
|
548 |
+
# Prep Scheduler
|
549 |
+
set_timesteps(scheduler, num_inference_steps)
|
550 |
+
|
551 |
+
# Prep latents
|
552 |
+
latents = torch.randn(
|
553 |
+
(batch_size, unet.in_channels, height // 8, width // 8),
|
554 |
+
generator=generator,
|
555 |
+
)
|
556 |
+
latents = latents.to(torch_device)
|
557 |
+
latents = latents * scheduler.init_noise_sigma
|
558 |
+
|
559 |
+
# Loop
|
560 |
+
for i, t in tqdm(enumerate(scheduler.timesteps), total=len(scheduler.timesteps)):
|
561 |
+
# expand the latents if we are doing classifier-free guidance to avoid doing two forward passes.
|
562 |
+
latent_model_input = torch.cat([latents] * 2)
|
563 |
+
sigma = scheduler.sigmas[i]
|
564 |
+
latent_model_input = scheduler.scale_model_input(latent_model_input, t)
|
565 |
+
|
566 |
+
# predict the noise residual
|
567 |
+
with torch.no_grad():
|
568 |
+
noise_pred = unet(latent_model_input, t, encoder_hidden_states=text_embeddings)["sample"]
|
569 |
+
|
570 |
+
# perform guidance
|
571 |
+
noise_pred_uncond, noise_pred_text = noise_pred.chunk(2)
|
572 |
+
noise_pred = noise_pred_uncond + guidance_scale * (noise_pred_text - noise_pred_uncond)
|
573 |
+
|
574 |
+
# compute the previous noisy sample x_t -> x_t-1
|
575 |
+
latents = scheduler.step(noise_pred, t, latents).prev_sample
|
576 |
+
|
577 |
+
return latents_to_pil(latents)[0]
|
578 |
+
|
579 |
+
generate_with_embs(modified_output_embeddings)
|
580 |
+
|
581 |
+
"""Suprise! Now you know what token 2368 means ;)
|
582 |
+
|
583 |
+
**What can we do with this?** Why did we go to all of this trouble? Well, we'll see a more compelling use-case shortly but the tl;dr is that once we can access and modify the token embeddings we can do tricks like replacing them with something else. In the example we just did, that was just another token embedding from the model's vocabulary, equivalent to just editing the prompt. But we can also mix tokens - for example, here's a half-puppy-half-skunk:
|
584 |
+
"""
|
585 |
+
|
586 |
+
# In case you're wondering how to get the token for a word, or the embedding for a token:
|
587 |
+
prompt = 'skunk'
|
588 |
+
print('tokenizer(prompt):', tokenizer(prompt))
|
589 |
+
print('token_emb_layer([token_id]) shape:', token_emb_layer(torch.tensor([8797], device=torch_device)).shape)
|
590 |
+
|
591 |
+
prompt = 'A picture of a puppy'
|
592 |
+
|
593 |
+
# Tokenize
|
594 |
+
text_input = tokenizer(prompt, padding="max_length", max_length=tokenizer.model_max_length, truncation=True, return_tensors="pt")
|
595 |
+
input_ids = text_input.input_ids.to(torch_device)
|
596 |
+
|
597 |
+
# Get token embeddings
|
598 |
+
token_embeddings = token_emb_layer(input_ids)
|
599 |
+
|
600 |
+
# The new embedding. Which is now a mixture of the token embeddings for 'puppy' and 'skunk'
|
601 |
+
puppy_token_embedding = token_emb_layer(torch.tensor(6829, device=torch_device))
|
602 |
+
skunk_token_embedding = token_emb_layer(torch.tensor(42194, device=torch_device))
|
603 |
+
replacement_token_embedding = 0.5*puppy_token_embedding + 0.5*skunk_token_embedding
|
604 |
+
|
605 |
+
# Insert this into the token embeddings (
|
606 |
+
token_embeddings[0, torch.where(input_ids[0]==6829)] = replacement_token_embedding.to(torch_device)
|
607 |
+
|
608 |
+
# Combine with pos embs
|
609 |
+
input_embeddings = token_embeddings + position_embeddings
|
610 |
+
|
611 |
+
# Feed through to get final output embs
|
612 |
+
modified_output_embeddings = get_output_embeds(input_embeddings)
|
613 |
+
|
614 |
+
# Generate an image with these
|
615 |
+
generate_with_embs(modified_output_embeddings)
|
616 |
+
|
617 |
+
"""### Textual Inversion
|
618 |
+
|
619 |
+
OK, so we can slip in a modified token embedding, and use this to generate an image. We used the token embedding for 'cat' in the above example, but what if instead could 'learn' a new token embedding for a specific concept? This is the idea behind 'Textual Inversion', in which a few example images are used to create a new token embedding:
|
620 |
+
|
621 |
+
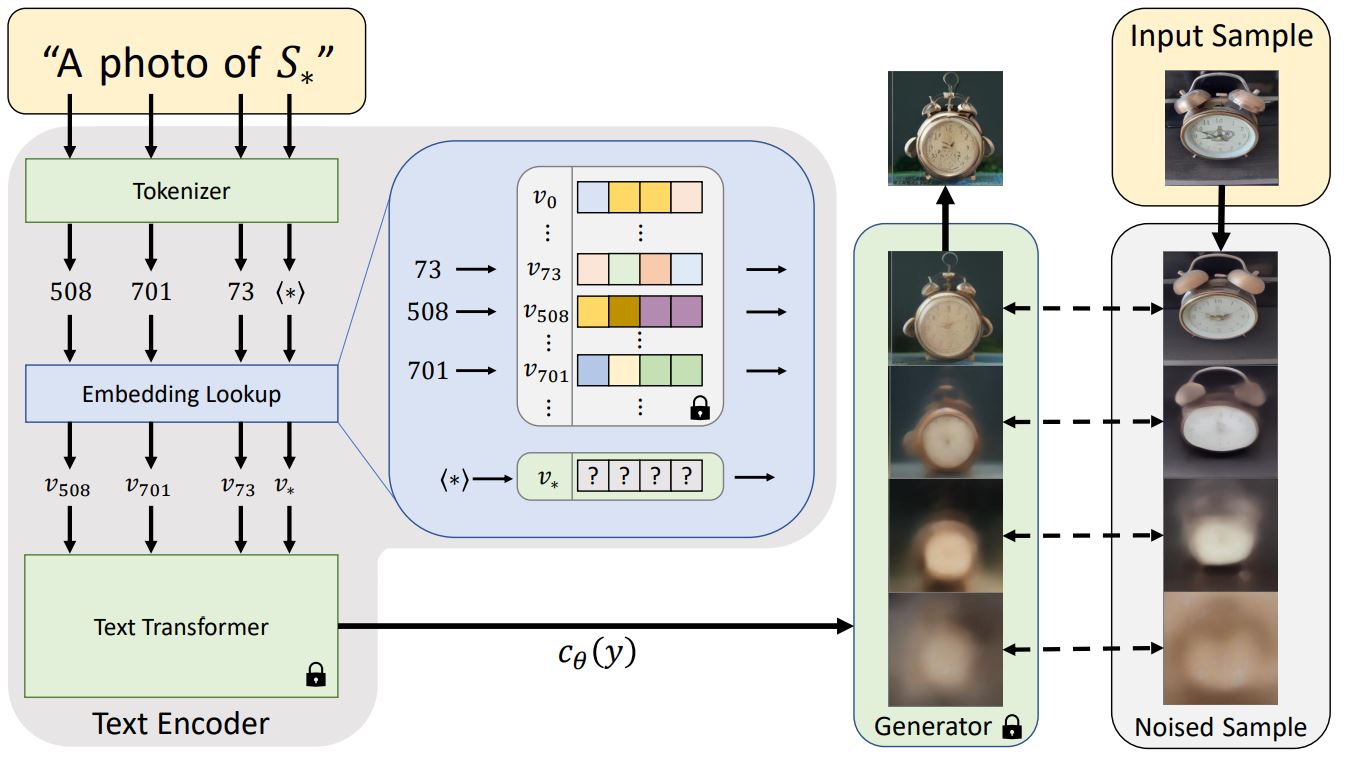
|
622 |
+
_Diagram from the [textual inversion blog post](https://textual-inversion.github.io/static/images/training/training.JPG) - note it doesn't show the positional embeddings step for simplicity_
|
623 |
+
|
624 |
+
We won't cover how this training works, but we can try loading one of these new 'concepts' from the [community-created SD concepts library](https://huggingface.co/sd-concepts-library) and see how it fits in with our example above. I'll use https://huggingface.co/sd-concepts-library/birb-style since it was the first one I made :) Download the learned_embeds.bin file from there and upload the file to wherever this notebook is before running this next cell:
|
625 |
+
"""
|
626 |
+
|
627 |
+
birb_embed = torch.load('learned_embeds.bin')
|
628 |
+
birb_embed.keys(), birb_embed['<birb-style>'].shape
|
629 |
+
|
630 |
+
"""We get a dictionary with a key (the special placeholder I used, <birb-style>) and the corresponding token embedding. As in the previous example, let's replace the 'puppy' token embedding with this and see what happens:"""
|
631 |
+
|
632 |
+
prompt = 'A mouse in the style of puppy'
|
633 |
+
|
634 |
+
# Tokenize
|
635 |
+
text_input = tokenizer(prompt, padding="max_length", max_length=tokenizer.model_max_length, truncation=True, return_tensors="pt")
|
636 |
+
input_ids = text_input.input_ids.to(torch_device)
|
637 |
+
|
638 |
+
# Get token embeddings
|
639 |
+
token_embeddings = token_emb_layer(input_ids)
|
640 |
+
|
641 |
+
# The new embedding - our special birb word
|
642 |
+
replacement_token_embedding = birb_embed['<birb-style>'].to(torch_device)
|
643 |
+
|
644 |
+
# Insert this into the token embeddings
|
645 |
+
token_embeddings[0, torch.where(input_ids[0]==6829)] = replacement_token_embedding.to(torch_device)
|
646 |
+
|
647 |
+
# Combine with pos embs
|
648 |
+
input_embeddings = token_embeddings + position_embeddings
|
649 |
+
|
650 |
+
# Feed through to get final output embs
|
651 |
+
modified_output_embeddings = get_output_embeds(input_embeddings)
|
652 |
+
|
653 |
+
# And generate an image with this:
|
654 |
+
generate_with_embs(modified_output_embeddings)
|
655 |
+
|
656 |
+
"""The token for 'puppy' was replaced with one that captures a particular style of painting, but it could just as easily represent a specific object or class of objects.
|
657 |
+
|
658 |
+
Again, there is [a nice inference notebook ](https://colab.research.google.com/github/huggingface/notebooks/blob/main/diffusers/stable_conceptualizer_inference.ipynb) from hf to make it easy to use the different concepts, that properly handles using the names in prompts ("A \<cat-toy> in the style of \<birb-style>") without worrying about all this manual stuff. The goal of this notebook is to pull back the curtain a bit so you know what is going on behind the scenes :)
|
659 |
+
|
660 |
+
## Messing with Embeddings
|
661 |
+
|
662 |
+
Besides just replacing the token embedding of a single word, there are various other tricks we can try. For example, what if we create a 'chimera' by averaging the embeddings of two different prompts?
|
663 |
+
"""
|
664 |
+
|
665 |
+
# Embed two prompts
|
666 |
+
text_input1 = tokenizer(["A mouse"], padding="max_length", max_length=tokenizer.model_max_length, truncation=True, return_tensors="pt")
|
667 |
+
text_input2 = tokenizer(["A leopard"], padding="max_length", max_length=tokenizer.model_max_length, truncation=True, return_tensors="pt")
|
668 |
+
with torch.no_grad():
|
669 |
+
text_embeddings1 = text_encoder(text_input1.input_ids.to(torch_device))[0]
|
670 |
+
text_embeddings2 = text_encoder(text_input2.input_ids.to(torch_device))[0]
|
671 |
+
|
672 |
+
# Mix them together
|
673 |
+
mix_factor = 0.35
|
674 |
+
mixed_embeddings = (text_embeddings1*mix_factor + \
|
675 |
+
text_embeddings2*(1-mix_factor))
|
676 |
+
|
677 |
+
# Generate!
|
678 |
+
generate_with_embs(mixed_embeddings)
|
679 |
+
|
680 |
+
"""## The UNET and CFG
|
681 |
+
|
682 |
+
Now it's time we looked at the actual diffusion model. This is typically a Unet that takes in the noisy latents (x) and predicts the noise. We use a conditional model that also takes in the timestep (t) and our text embedding (aka encoder_hidden_states) as conditioning. Feeding all of these into the model looks like this:
|
683 |
+
`noise_pred = unet(latents, t, encoder_hidden_states=text_embeddings)["sample"]`
|
684 |
+
|
685 |
+
We can try it out and see what the output looks like:
|
686 |
+
"""
|
687 |
+
|
688 |
+
# Prep Scheduler
|
689 |
+
set_timesteps(scheduler, num_inference_steps)
|
690 |
+
|
691 |
+
# What is our timestep
|
692 |
+
t = scheduler.timesteps[0]
|
693 |
+
sigma = scheduler.sigmas[0]
|
694 |
+
|
695 |
+
# A noisy latent
|
696 |
+
latents = torch.randn(
|
697 |
+
(batch_size, unet.in_channels, height // 8, width // 8),
|
698 |
+
generator=generator,
|
699 |
+
)
|
700 |
+
latents = latents.to(torch_device)
|
701 |
+
latents = latents * scheduler.init_noise_sigma
|
702 |
+
|
703 |
+
# Text embedding
|
704 |
+
text_input = tokenizer(['A macaw'], padding="max_length", max_length=tokenizer.model_max_length, truncation=True, return_tensors="pt")
|
705 |
+
with torch.no_grad():
|
706 |
+
text_embeddings = text_encoder(text_input.input_ids.to(torch_device))[0]
|
707 |
+
|
708 |
+
# Run this through the unet to predict the noise residual
|
709 |
+
with torch.no_grad():
|
710 |
+
noise_pred = unet(latents, t, encoder_hidden_states=text_embeddings)["sample"]
|
711 |
+
|
712 |
+
latents.shape, noise_pred.shape # We get preds in the same shape as the input
|
713 |
+
|
714 |
+
"""Given a set of noisy latents, the model predicts the noise component. We can remove this noise from the noisy latents to see what the output image looks like (`latents_x0 = latents - sigma * noise_pred`). And we can add most of the noise back to this predicted output to get the (slightly less noisy hopefully) input for the next diffusion step. To visualize this let's generate another image, saving both the predicted output (x0) and the next step (xt-1) after every step:"""
|
715 |
+
|
716 |
+
prompt = 'Oil painting of an otter in a top hat'
|
717 |
+
height = 512
|
718 |
+
width = 512
|
719 |
+
num_inference_steps = 50
|
720 |
+
guidance_scale = 8
|
721 |
+
generator = torch.manual_seed(32)
|
722 |
+
batch_size = 1
|
723 |
+
|
724 |
+
# Make a folder to store results
|
725 |
+
!rm -rf steps/
|
726 |
+
!mkdir -p steps/
|
727 |
+
|
728 |
+
# Prep text
|
729 |
+
text_input = tokenizer([prompt], padding="max_length", max_length=tokenizer.model_max_length, truncation=True, return_tensors="pt")
|
730 |
+
with torch.no_grad():
|
731 |
+
text_embeddings = text_encoder(text_input.input_ids.to(torch_device))[0]
|
732 |
+
max_length = text_input.input_ids.shape[-1]
|
733 |
+
uncond_input = tokenizer(
|
734 |
+
[""] * batch_size, padding="max_length", max_length=max_length, return_tensors="pt"
|
735 |
+
)
|
736 |
+
with torch.no_grad():
|
737 |
+
uncond_embeddings = text_encoder(uncond_input.input_ids.to(torch_device))[0]
|
738 |
+
text_embeddings = torch.cat([uncond_embeddings, text_embeddings])
|
739 |
+
|
740 |
+
# Prep Scheduler
|
741 |
+
set_timesteps(scheduler, num_inference_steps)
|
742 |
+
|
743 |
+
# Prep latents
|
744 |
+
latents = torch.randn(
|
745 |
+
(batch_size, unet.in_channels, height // 8, width // 8),
|
746 |
+
generator=generator,
|
747 |
+
)
|
748 |
+
latents = latents.to(torch_device)
|
749 |
+
latents = latents * scheduler.init_noise_sigma
|
750 |
+
|
751 |
+
# Loop
|
752 |
+
for i, t in tqdm(enumerate(scheduler.timesteps), total=len(scheduler.timesteps)):
|
753 |
+
# expand the latents if we are doing classifier-free guidance to avoid doing two forward passes.
|
754 |
+
latent_model_input = torch.cat([latents] * 2)
|
755 |
+
sigma = scheduler.sigmas[i]
|
756 |
+
latent_model_input = scheduler.scale_model_input(latent_model_input, t)
|
757 |
+
|
758 |
+
# predict the noise residual
|
759 |
+
with torch.no_grad():
|
760 |
+
noise_pred = unet(latent_model_input, t, encoder_hidden_states=text_embeddings)["sample"]
|
761 |
+
|
762 |
+
# perform guidance
|
763 |
+
noise_pred_uncond, noise_pred_text = noise_pred.chunk(2)
|
764 |
+
noise_pred = noise_pred_uncond + guidance_scale * (noise_pred_text - noise_pred_uncond)
|
765 |
+
|
766 |
+
# Get the predicted x0:
|
767 |
+
# latents_x0 = latents - sigma * noise_pred # Calculating ourselves
|
768 |
+
scheduler_step = scheduler.step(noise_pred, t, latents)
|
769 |
+
latents_x0 = scheduler_step.pred_original_sample # Using the scheduler (Diffusers 0.4 and above)
|
770 |
+
|
771 |
+
# compute the previous noisy sample x_t -> x_t-1
|
772 |
+
latents = scheduler_step.prev_sample
|
773 |
+
|
774 |
+
# To PIL Images
|
775 |
+
im_t0 = latents_to_pil(latents_x0)[0]
|
776 |
+
im_next = latents_to_pil(latents)[0]
|
777 |
+
|
778 |
+
# Combine the two images and save for later viewing
|
779 |
+
im = Image.new('RGB', (1024, 512))
|
780 |
+
im.paste(im_next, (0, 0))
|
781 |
+
im.paste(im_t0, (512, 0))
|
782 |
+
im.save(f'steps/{i:04}.jpeg')
|
783 |
+
|
784 |
+
# Make and show the progress video (change width to 1024 for full res)
|
785 |
+
!ffmpeg -v 1 -y -f image2 -framerate 12 -i steps/%04d.jpeg -c:v libx264 -preset slow -qp 18 -pix_fmt yuv420p out.mp4
|
786 |
+
mp4 = open('out.mp4','rb').read()
|
787 |
+
data_url = "data:video/mp4;base64," + b64encode(mp4).decode()
|
788 |
+
HTML("""
|
789 |
+
<video width=600 controls>
|
790 |
+
<source src="%s" type="video/mp4">
|
791 |
+
</video>
|
792 |
+
""" % data_url)
|
793 |
+
|
794 |
+
"""The version on the right shows the predicted 'final output' (x0) at each step, and this is what is usually used for progress videos etc. The version on the left is the 'next step'. I found it interesteing to compare the two - watching the progress videos only you'd think drastic changes are happening expecially at early stages, but since the changes made per-step are relatively small the actual process is much more gradual.
|
795 |
+
|
796 |
+
### Classifier Free Guidance
|
797 |
+
|
798 |
+
By default, the model doesn't often do what we ask. If we want it to follow the prompt better, we use a hack called CFG. There's a good explanation in this video (AI coffee break GLIDE).
|
799 |
+
|
800 |
+
In the code, this comes down to us doing:
|
801 |
+
|
802 |
+
`noise_pred = noise_pred_uncond + guidance_scale * (noise_pred_text - noise_pred_uncond)`
|
803 |
+
|
804 |
+
This works suprisingly well :) Explore changing the guidance_scale in the code above and see how this affects the results. How high can you push it before the results get worse?
|
805 |
+
|
806 |
+
## Sampling
|
807 |
+
|
808 |
+
There is still some complexity hidden from us inside `latents = scheduler.step(noise_pred, i, latents)["prev_sample"]`. How exactly does the sampler go from the current noisy latents to a slightly less noisy version? Why don't we just use the model in a single step? Are there other ways to view this?
|
809 |
+
|
810 |
+
The model tries to predict the noise in an image. For low noise values, we assume it does a pretty good job. For higher noise levels, it has a hard task! So instead of producing a perfect image, the results tend to look like a blurry mess - see the start of the video above for a visual! So, samplers use the model predictions to move a small amount towards the model prediction (removing some of the noise) and then get another prediction based on this marginally-less-rubbish input, and hope that this iteratively improves the result.
|
811 |
+
|
812 |
+
Different samplers do this in different ways. You can try to inspect the code for the default LMS sampler with:
|
813 |
+
"""
|
814 |
+
|
815 |
+
# ??scheduler.step
|
816 |
+
|
817 |
+
"""**Time to draw some diagrams!** (Whiteboard/paper interlude)
|
818 |
+
|
819 |
+
# Guidance
|
820 |
+
|
821 |
+
|
822 |
+
OK, final trick! How can we add some extra control to this generation process?
|
823 |
+
|
824 |
+
At each step, we're going to use our model as before to predict the noise component of x. Then we'll use this to produce a predicted output image, and apply some loss function to this image.
|
825 |
+
|
826 |
+
This function can be anything, but let's demo with a super simple example. If we want images that have a lot of blue, we can craft a loss function that gives a high loss if pixels have a low blue component:
|
827 |
+
"""
|
828 |
+
|
829 |
+
def blue_loss(images):
|
830 |
+
# How far are the blue channel values to 0.9:
|
831 |
+
error = torch.abs(images[:,2] - 0.9).mean() # [:,2] -> all images in batch, only the blue channel
|
832 |
+
return error
|
833 |
+
|
834 |
+
def orange_loss(images):
|
835 |
+
"""
|
836 |
+
Calculate the mean absolute error between the RGB values of the images and the target orange color.
|
837 |
+
|
838 |
+
Parameters:
|
839 |
+
- images (torch.Tensor): A batch of images with shape (batch_size, channels, height, width).
|
840 |
+
The images are assumed to be in RGB format.
|
841 |
+
|
842 |
+
Returns:
|
843 |
+
- torch.Tensor: The mean absolute error for the orange color.
|
844 |
+
"""
|
845 |
+
# Define the target RGB values for the color orange
|
846 |
+
target_orange = torch.tensor([255/255, 200/255, 0/255]).view(1, 3, 1, 1).to(images.device) # (R, G, B)
|
847 |
+
|
848 |
+
# Normalize images to [0, 1] range if not already normalized
|
849 |
+
images = images / 255.0 if images.max() > 1.0 else images
|
850 |
+
|
851 |
+
# Calculate the mean absolute error between the RGB values and the target orange values
|
852 |
+
error = torch.abs(images - target_orange).mean()
|
853 |
+
|
854 |
+
return error
|
855 |
+
|
856 |
+
"""During each update step, we find the gradient of the loss with respect to the current noisy latents, and tweak them in the direction that reduces this loss as well as performing the normal update step:"""
|
857 |
+
|
858 |
+
prompt = 'A campfire (oil on canvas)' #@param
|
859 |
+
height = 512 # default height of Stable Diffusion
|
860 |
+
width = 512 # default width of Stable Diffusion
|
861 |
+
num_inference_steps = 50 #@param # Number of denoising steps
|
862 |
+
guidance_scale = 8 #@param # Scale for classifier-free guidance
|
863 |
+
generator = torch.manual_seed(32) # Seed generator to create the inital latent noise
|
864 |
+
batch_size = 1
|
865 |
+
orange_loss_scale = 200 #@param
|
866 |
+
|
867 |
+
# Prep text
|
868 |
+
text_input = tokenizer([prompt], padding="max_length", max_length=tokenizer.model_max_length, truncation=True, return_tensors="pt")
|
869 |
+
with torch.no_grad():
|
870 |
+
text_embeddings = text_encoder(text_input.input_ids.to(torch_device))[0]
|
871 |
+
|
872 |
+
# And the uncond. input as before:
|
873 |
+
max_length = text_input.input_ids.shape[-1]
|
874 |
+
uncond_input = tokenizer(
|
875 |
+
[""] * batch_size, padding="max_length", max_length=max_length, return_tensors="pt"
|
876 |
+
)
|
877 |
+
with torch.no_grad():
|
878 |
+
uncond_embeddings = text_encoder(uncond_input.input_ids.to(torch_device))[0]
|
879 |
+
text_embeddings = torch.cat([uncond_embeddings, text_embeddings])
|
880 |
+
|
881 |
+
# Prep Scheduler
|
882 |
+
set_timesteps(scheduler, num_inference_steps)
|
883 |
+
|
884 |
+
# Prep latents
|
885 |
+
latents = torch.randn(
|
886 |
+
(batch_size, unet.in_channels, height // 8, width // 8),
|
887 |
+
generator=generator,
|
888 |
+
)
|
889 |
+
latents = latents.to(torch_device)
|
890 |
+
latents = latents * scheduler.init_noise_sigma
|
891 |
+
|
892 |
+
# Loop
|
893 |
+
for i, t in tqdm(enumerate(scheduler.timesteps), total=len(scheduler.timesteps)):
|
894 |
+
# expand the latents if we are doing classifier-free guidance to avoid doing two forward passes.
|
895 |
+
latent_model_input = torch.cat([latents] * 2)
|
896 |
+
sigma = scheduler.sigmas[i]
|
897 |
+
latent_model_input = scheduler.scale_model_input(latent_model_input, t)
|
898 |
+
|
899 |
+
# predict the noise residual
|
900 |
+
with torch.no_grad():
|
901 |
+
noise_pred = unet(latent_model_input, t, encoder_hidden_states=text_embeddings)["sample"]
|
902 |
+
|
903 |
+
# perform CFG
|
904 |
+
noise_pred_uncond, noise_pred_text = noise_pred.chunk(2)
|
905 |
+
noise_pred = noise_pred_uncond + guidance_scale * (noise_pred_text - noise_pred_uncond)
|
906 |
+
|
907 |
+
#### ADDITIONAL GUIDANCE ###
|
908 |
+
if i%5 == 0:
|
909 |
+
# Requires grad on the latents
|
910 |
+
latents = latents.detach().requires_grad_()
|
911 |
+
|
912 |
+
# Get the predicted x0:
|
913 |
+
latents_x0 = latents - sigma * noise_pred
|
914 |
+
# latents_x0 = scheduler.step(noise_pred, t, latents).pred_original_sample
|
915 |
+
|
916 |
+
# Decode to image space
|
917 |
+
denoised_images = vae.decode((1 / 0.18215) * latents_x0).sample / 2 + 0.5 # range (0, 1)
|
918 |
+
|
919 |
+
# Calculate loss
|
920 |
+
loss = blue_loss(denoised_images) * orange_loss_scale
|
921 |
+
|
922 |
+
# Occasionally print it out
|
923 |
+
if i%10==0:
|
924 |
+
print(i, 'loss:', loss.item())
|
925 |
+
|
926 |
+
# Get gradient
|
927 |
+
cond_grad = torch.autograd.grad(loss, latents)[0]
|
928 |
+
|
929 |
+
# Modify the latents based on this gradient
|
930 |
+
latents = latents.detach() - cond_grad * sigma**2
|
931 |
+
|
932 |
+
# Now step with scheduler
|
933 |
+
latents = scheduler.step(noise_pred, t, latents).prev_sample
|
934 |
+
|
935 |
+
|
936 |
+
latents_to_pil(latents)[0]
|
937 |
+
|
938 |
+
prompt = 'A mouse in the style of puppy'
|
939 |
+
|
940 |
+
# Tokenize
|
941 |
+
text_input = tokenizer(prompt, padding="max_length", max_length=tokenizer.model_max_length, truncation=True, return_tensors="pt")
|
942 |
+
text_input
|
943 |
+
input_ids = text_input.input_ids.to(torch_device)
|
944 |
+
|
945 |
+
# Get token embeddings
|
946 |
+
token_embeddings = token_emb_layer(input_ids)
|
947 |
+
|
948 |
+
# The new embedding - our special birb word
|
949 |
+
replacement_token_embedding = birb_embed['<birb-style>'].to(torch_device)
|
950 |
+
|
951 |
+
# Insert this into the token embeddings
|
952 |
+
token_embeddings[0, torch.where(input_ids[0]==6829)] = replacement_token_embedding.to(torch_device)
|
953 |
+
|
954 |
+
# Combine with pos embs
|
955 |
+
input_embeddings = token_embeddings + position_embeddings
|
956 |
+
|
957 |
+
# Feed through to get final output embs
|
958 |
+
modified_output_embeddings = get_output_embeds(input_embeddings)
|
959 |
+
|
960 |
+
# And generate an image with this:
|
961 |
+
generate_with_embs(modified_output_embeddings)
|
962 |
+
|
963 |
+
text_input, input_ids,token_embeddings
|
964 |
+
|
965 |
+
def generate_loss(modified_output_embeddings, seed, max_length):
|
966 |
+
# prompt = 'A campfire (oil on canvas)' #@param
|
967 |
+
height = 512 # default height of Stable Diffusion
|
968 |
+
width = 512 # default width of Stable Diffusion
|
969 |
+
num_inference_steps = 50 #@param # Number of denoising steps
|
970 |
+
guidance_scale = 8 #@param # Scale for classifier-free guidance
|
971 |
+
generator = torch.manual_seed(32) # Seed generator to create the initial latent noise
|
972 |
+
batch_size = 1
|
973 |
+
blue_loss_scale = 200 #@param
|
974 |
+
|
975 |
+
# Prep text
|
976 |
+
# text_input = tokenizer([""] * batch_size, padding="max_length", max_length=tokenizer.model_max_length, truncation=True, return_tensors="pt")
|
977 |
+
|
978 |
+
#input_ids = text_input.input_ids.to(torch_device)
|
979 |
+
# Get token embeddings
|
980 |
+
#token_embeddings = token_emb_layer(input_ids)
|
981 |
+
|
982 |
+
# The new embedding - our special birb word
|
983 |
+
#replacement_token_embedding = birb_embed['<birb-style>'].to(torch_device)
|
984 |
+
# Insert this into the token embeddings
|
985 |
+
#indices = torch.where(input_ids[0] == 6829)[0]
|
986 |
+
#token_embeddings[0, indices] = replacement_token_embedding.expand_as(token_embeddings[0, indices])
|
987 |
+
|
988 |
+
# Combine with pos embs
|
989 |
+
#input_embeddings = token_embeddings + position_embeddings
|
990 |
+
|
991 |
+
# Pass the modified embeddings to the text encoder
|
992 |
+
#with torch.no_grad():
|
993 |
+
# text_embeddings = text_encoder(inputs_embeds=input_embeddings)[0]
|
994 |
+
|
995 |
+
# And the uncond. input as before:
|
996 |
+
# max_length = input_ids.shape[-1]
|
997 |
+
uncond_input = tokenizer(
|
998 |
+
[""] * batch_size, padding="max_length", max_length=max_length, return_tensors="pt"
|
999 |
)
|
1000 |
+
with torch.no_grad():
|
1001 |
+
uncond_embeddings = text_encoder(uncond_input.input_ids.to(torch_device))[0]
|
1002 |
+
# Ensure both embeddings have the same shape
|
1003 |
+
if uncond_embeddings.shape != modified_output_embeddings.shape:
|
1004 |
+
raise ValueError(f"Shape mismatch: uncond_embeddings {uncond_embeddings.shape} vs modified_output_embeddings {modified_output_embeddings.shape}")
|
1005 |
+
|
1006 |
+
text_embeddings = torch.cat([uncond_embeddings, modified_output_embeddings])
|
1007 |
+
|
1008 |
+
# Prep Scheduler
|
1009 |
+
set_timesteps(scheduler, num_inference_steps)
|
1010 |
+
|
1011 |
+
# Prep latents
|
1012 |
+
latents = torch.randn(
|
1013 |
+
(batch_size, unet.in_channels, height // 8, width // 8),
|
1014 |
+
generator=generator,
|
1015 |
+
)
|
1016 |
+
latents = latents.to(torch_device)
|
1017 |
+
latents = latents * scheduler.init_noise_sigma
|
1018 |
+
|
1019 |
+
# Loop
|
1020 |
+
for i, t in tqdm(enumerate(scheduler.timesteps), total=len(scheduler.timesteps)):
|
1021 |
+
# expand the latents if we are doing classifier-free guidance to avoid doing two forward passes.
|
1022 |
+
latent_model_input = torch.cat([latents] * 2)
|
1023 |
+
sigma = scheduler.sigmas[i]
|
1024 |
+
latent_model_input = scheduler.scale_model_input(latent_model_input, t)
|
1025 |
+
|
1026 |
+
# predict the noise residual
|
1027 |
+
with torch.no_grad():
|
1028 |
+
noise_pred = unet(latent_model_input, t, encoder_hidden_states=text_embeddings)["sample"]
|
1029 |
+
|
1030 |
+
# perform CFG
|
1031 |
+
noise_pred_uncond, noise_pred_text = noise_pred.chunk(2)
|
1032 |
+
noise_pred = noise_pred_uncond + guidance_scale * (noise_pred_text - noise_pred_uncond)
|
1033 |
+
|
1034 |
+
#### ADDITIONAL GUIDANCE ###
|
1035 |
+
if i % 5 == 0:
|
1036 |
+
# Requires grad on the latents
|
1037 |
+
latents = latents.detach().requires_grad_()
|
1038 |
+
|
1039 |
+
# Get the predicted x0:
|
1040 |
+
latents_x0 = latents - sigma * noise_pred
|
1041 |
+
# latents_x0 = scheduler.step(noise_pred, t, latents).pred_original_sample
|
1042 |
+
|
1043 |
+
# Decode to image space
|
1044 |
+
denoised_images = vae.decode((1 / 0.18215) * latents_x0).sample / 2 + 0.5 # range (0, 1)
|
1045 |
+
|
1046 |
+
# Calculate loss
|
1047 |
+
loss = orange_loss(denoised_images) * blue_loss_scale
|
1048 |
+
|
1049 |
+
# Occasionally print it out
|
1050 |
+
if i % 10 == 0:
|
1051 |
+
print(i, 'loss:', loss.item())
|
1052 |
+
|
1053 |
+
# Get gradient
|
1054 |
+
cond_grad = torch.autograd.grad(loss, latents)[0]
|
1055 |
+
|
1056 |
+
# Modify the latents based on this gradient
|
1057 |
+
latents = latents.detach() - cond_grad * sigma ** 2
|
1058 |
+
|
1059 |
+
# Now step with scheduler
|
1060 |
+
latents = scheduler.step(noise_pred, t, latents).prev_sample
|
1061 |
+
|
1062 |
+
# Convert the final latents to an image and display it
|
1063 |
+
image = latents_to_pil(latents)[0]
|
1064 |
+
image.show()
|
1065 |
+
return image
|
1066 |
+
|
1067 |
+
def generate_loss_style(prompt, style_embed, style_seed):
|
1068 |
+
# Tokenize
|
1069 |
+
text_input = tokenizer(prompt, padding="max_length", max_length=tokenizer.model_max_length, truncation=True, return_tensors="pt")
|
1070 |
+
input_ids = text_input.input_ids.to(torch_device)
|
1071 |
+
|
1072 |
+
# Get token embeddings
|
1073 |
+
token_embeddings = token_emb_layer(input_ids)
|
1074 |
+
if isinstance(style_embed, dict):
|
1075 |
+
style_embed = style_embed['<gartic-phone>']
|
1076 |
+
|
1077 |
+
# The new embedding - our special birb word
|
1078 |
+
replacement_token_embedding = style_embed.to(torch_device)
|
1079 |
+
# Assuming token_embeddings has shape [batch_size, seq_length, embedding_dim]
|
1080 |
+
replacement_token_embedding = replacement_token_embedding[:768] # Adjust the size
|
1081 |
+
replacement_token_embedding = replacement_token_embedding.unsqueeze(0) # Make it [1, 768] if necessary
|
1082 |
+
indices = torch.where(input_ids[0] == 6829)[0] # Extract indices where the condition is True
|
1083 |
+
print(f"indices: {indices}") # Debug print
|
1084 |
+
for index in indices:
|
1085 |
+
print(f"index: {index}") # Debug print
|
1086 |
+
token_embeddings[0, index] = replacement_token_embedding.to(torch_device) # Update each index
|
1087 |
+
|
1088 |
+
# Insert this into the token embeddings
|
1089 |
+
# token_embeddings[0, torch.where(input_ids[0]==6829)] = replacement_token_embedding.to(torch_device)
|
1090 |
+
|
1091 |
+
# Combine with pos embs
|
1092 |
+
input_embeddings = token_embeddings + position_embeddings
|
1093 |
+
|
1094 |
+
# Feed through to get final output embs
|
1095 |
+
modified_output_embeddings = get_output_embeds(input_embeddings)
|
1096 |
+
|
1097 |
+
# And generate an image with this:
|
1098 |
+
max_length = text_input.input_ids.shape[-1]
|
1099 |
+
return generate_loss(modified_output_embeddings, style_seed,max_length)
|
1100 |
+
|
1101 |
+
def generate_embed_style(prompt, learned_style, seed):
|
1102 |
+
# prompt = 'A campfire (oil on canvas)' #@param
|
1103 |
+
height = 512 # default height of Stable Diffusion
|
1104 |
+
width = 512 # default width of Stable Diffusion
|
1105 |
+
num_inference_steps = 50 #@param # Number of denoising steps
|
1106 |
+
guidance_scale = 8 #@param # Scale for classifier-free guidance
|
1107 |
+
generator = torch.manual_seed(32) # Seed generator to create the initial latent noise
|
1108 |
+
batch_size = 1
|
1109 |
+
blue_loss_scale = 200 #@param
|
1110 |
+
if isinstance(learned_style, dict):
|
1111 |
+
learned_style = learned_style['<gartic-phone>']
|
1112 |
+
|
1113 |
+
# Prep text
|
1114 |
+
text_input = tokenizer([prompt], padding="max_length", max_length=tokenizer.model_max_length, truncation=True, return_tensors="pt")
|
1115 |
+
|
1116 |
+
input_ids = text_input.input_ids.to(torch_device)
|
1117 |
+
# Get token embeddings
|
1118 |
+
token_embeddings = text_encoder.get_input_embeddings()(input_ids)
|
1119 |
+
|
1120 |
+
# The new embedding - our special birb word
|
1121 |
+
replacement_token_embedding = learned_style.to(torch_device)
|
1122 |
+
replacement_token_embedding = replacement_token_embedding[:768] # Adjust the size
|
1123 |
+
replacement_token_embedding = replacement_token_embedding.unsqueeze(0) # Make it [1, 768] if necessary
|
1124 |
+
# Insert this into the token embeddings
|
1125 |
+
indices = torch.where(input_ids[0] == 6829)[0]
|
1126 |
+
for index in indices:
|
1127 |
+
token_embeddings[0, index] = replacement_token_embedding.to(torch_device)
|
1128 |
+
# Combine with pos embs
|
1129 |
+
position_ids = torch.arange(token_embeddings.shape[1], dtype=torch.long, device=torch_device)
|
1130 |
+
position_ids = position_ids.unsqueeze(0).expand_as(input_ids)
|
1131 |
+
position_ids = text_encoder.text_model.embeddings.position_ids[:, :77]
|
1132 |
+
position_embeddings = pos_emb_layer(position_ids)
|
1133 |
+
#position_embeddings = text_encoder.get_position_embeddings()(position_ids)
|
1134 |
+
input_embeddings = token_embeddings + position_embeddings
|
1135 |
+
# Feed through to get final output embs
|
1136 |
+
modified_output_embeddings = get_output_embeds(input_embeddings)
|
1137 |
+
# And generate an image with this:
|
1138 |
+
max_length = text_input.input_ids.shape[-1]
|
1139 |
+
emb_seed = generate_with_embs_seed(modified_output_embeddings, seed, max_length)
|
1140 |
+
#generate_loss_details = generate_loss(modified_output_embeddings, seed, max_length)
|
1141 |
+
return emb_seed
|
1142 |
+
# And generate an , generateimage with this:
|
1143 |
+
|
1144 |
+
|
1145 |
+
|
1146 |
+
def generate_image_from_prompt(text_in, style_in):
|
1147 |
+
|
1148 |
+
prompt = 'A campfire (oil on canvas)'
|
1149 |
+
style_seed = 32
|
1150 |
+
dict_styles = {'<gartic-phone>':'learned_embeds_gartic-phone.bin',
|
1151 |
+
'<hawaiian shirt>':'learned_embeds_hawaiian-shirt.bin',
|
1152 |
+
'<gp>': 'learned_embeds_phone01.bin',
|
1153 |
+
'<style-spdmn>':'learned_embeds_style-spdmn.bin',
|
1154 |
+
'<yvmqznrm>': 'learned_embedssd_yvmqznrm.bin'}
|
1155 |
+
|
1156 |
+
learn_embed = ['learned_embeds_gartic-phone.bin', 'learned_embeds_hawaiian-shirt_style.bin', 'learned_embeds_phone01_style.bin', 'learned_embeds_style-spdmn_style.bin', 'learned_embedssd_yvmqznrm_style.bin']
|
1157 |
+
style = dict_styles # (learn_embed[0])
|
1158 |
+
birb_embed = torch.load(learn_embed[0])
|
1159 |
+
#birb_embed.keys(), dict_styles['<gartic-phone>'].shape
|
1160 |
+
#style_embed = torch.load(dict_styles)
|
1161 |
+
#birb_embed = torch.load('learned_embeds.bin')
|
1162 |
+
#birb_embed.keys(), birb_embed['<birb-style>'].shape
|
1163 |
+
generated_image = generate_embed_style(prompt,birb_embed, style_seed)
|
1164 |
+
generate_loss_details = (generate_loss_style(prompt, birb_embed, style_seed))
|
1165 |
+
#generate_loss_style(prompt, style_embed, style_seed):
|
1166 |
+
|
1167 |
+
#loss_generated_img = (loss_style(prompt, style_embed[0], style_seed))
|
1168 |
|
1169 |
+
return [generated_image]
|