Spaces:
Running
on
Zero
Running
on
Zero
import gradio as gr | |
import requests | |
import random | |
import os | |
import zipfile | |
import librosa | |
import time | |
from infer_rvc_python import BaseLoader | |
from pydub import AudioSegment | |
from tts_voice import tts_order_voice | |
import edge_tts | |
import tempfile | |
from audio_separator.separator import Separator | |
import model_handler | |
import psutil | |
import cpuinfo | |
language_dict = tts_order_voice | |
async def text_to_speech_edge(text, language_code): | |
voice = language_dict[language_code] | |
communicate = edge_tts.Communicate(text, voice) | |
with tempfile.NamedTemporaryFile(delete=False, suffix=".mp3") as tmp_file: | |
tmp_path = tmp_file.name | |
await communicate.save(tmp_path) | |
return tmp_path | |
try: | |
import spaces | |
spaces_status = True | |
except ImportError: | |
spaces_status = False | |
separator = Separator() | |
converter = BaseLoader(only_cpu=False, hubert_path=None, rmvpe_path=None) | |
global pth_file | |
global index_file | |
pth_file = "model.pth" | |
index_file = "model.index" | |
#CONFIGS | |
TEMP_DIR = "temp" | |
MODEL_PREFIX = "model" | |
PITCH_ALGO_OPT = [ | |
"pm", | |
"harvest", | |
"crepe", | |
"rmvpe", | |
"rmvpe+", | |
] | |
UVR_5_MODELS = [ | |
{"model_name": "BS-Roformer-Viperx-1297", "checkpoint": "model_bs_roformer_ep_317_sdr_12.9755.ckpt"}, | |
{"model_name": "MDX23C-InstVoc HQ 2", "checkpoint": "MDX23C-8KFFT-InstVoc_HQ_2.ckpt"}, | |
{"model_name": "Kim Vocal 2", "checkpoint": "Kim_Vocal_2.onnx"}, | |
{"model_name": "5_HP-Karaoke", "checkpoint": "5_HP-Karaoke-UVR.pth"}, | |
{"model_name": "UVR-DeNoise by FoxJoy", "checkpoint": "UVR-DeNoise.pth"}, | |
{"model_name": "UVR-DeEcho-DeReverb by FoxJoy", "checkpoint": "UVR-DeEcho-DeReverb.pth"}, | |
] | |
MODELS = [ | |
{"model": "model.pth", "index": "model.index", "model_name": "Test Model"}, | |
] | |
os.makedirs(TEMP_DIR, exist_ok=True) | |
def unzip_file(file): | |
filename = os.path.basename(file).split(".")[0] | |
with zipfile.ZipFile(file, 'r') as zip_ref: | |
zip_ref.extractall(os.path.join(TEMP_DIR, filename)) | |
return True | |
def progress_bar(total, current): | |
return "[" + "=" * int(current / total * 20) + ">" + " " * (20 - int(current / total * 20)) + "] " + str(int(current / total * 100)) + "%" | |
def contains_bad_word(text, bad_words): | |
text_lower = text.lower() | |
for word in bad_words: | |
if word.lower() in text_lower: | |
return True | |
return False | |
bad_words = ['puttana', 'whore', 'badword3', 'badword4'] | |
class BadWordError(Exception): | |
def __init__(self, msg): | |
super().__init__(msg) | |
self.word = word | |
def download_from_url(url, name=None): | |
if name is None: | |
raise ValueError("The model name must be provided") | |
if "/blob/" in url: | |
url = url.replace("/blob/", "/resolve/") | |
if "huggingface" not in url: | |
return ["The URL must be from huggingface", "Failed", "Failed"] | |
filename = os.path.join(TEMP_DIR, MODEL_PREFIX + str(random.randint(1, 1000)) + ".zip") | |
if contains_bad_word(url, bad_words): | |
return BadWordError("The filename has a bad word.") | |
response = requests.get(url) | |
total = int(response.headers.get('content-length', 0)) | |
if total > 500000000: | |
return ["The file is too large. You can only download files up to 500 MB in size.", "Failed", "Failed"] | |
current = 0 | |
with open(filename, "wb") as f: | |
for data in response.iter_content(chunk_size=4096): | |
f.write(data) | |
current += len(data) | |
print(progress_bar(total, current), end="\r") # | |
try: | |
unzip_file(filename) | |
except Exception as e: | |
return ["Failed to unzip the file", "Failed", "Failed"] | |
unzipped_dir = os.path.join(TEMP_DIR, os.path.basename(filename).split(".")[0]) | |
pth_files = [] | |
index_files = [] | |
for root, dirs, files in os.walk(unzipped_dir): | |
for file in files: | |
if file.endswith(".pth"): | |
pth_files.append(os.path.join(root, file)) | |
elif file.endswith(".index"): | |
index_files.append(os.path.join(root, file)) | |
print(pth_files, index_files) | |
global pth_file | |
global index_file | |
pth_file = pth_files[0] | |
index_file = index_files[0] | |
print(pth_file) | |
print(index_file) | |
if name == "": | |
name = pth_file.split(".")[0] | |
MODELS.append({"model": pth_file, "index": index_file, "model_name": name}) | |
return ["Downloaded as " + name, pth_files[0], index_files[0]] | |
def inference(audio, model_name): | |
output_data = inf_handler(audio, model_name) | |
vocals = output_data[0] | |
inst = output_data[1] | |
return vocals, inst | |
if spaces_status: | |
def convert_now(audio_files, random_tag, converter): | |
return converter( | |
audio_files, | |
random_tag, | |
overwrite=False, | |
parallel_workers=8 | |
) | |
else: | |
def convert_now(audio_files, random_tag, converter): | |
return converter( | |
audio_files, | |
random_tag, | |
overwrite=False, | |
parallel_workers=8 | |
) | |
def calculate_remaining_time(epochs, seconds_per_epoch): | |
total_seconds = epochs * seconds_per_epoch | |
hours = total_seconds // 3600 | |
minutes = (total_seconds % 3600) // 60 | |
seconds = total_seconds % 60 | |
if hours == 0: | |
return f"{int(minutes)} minutes" | |
elif hours == 1: | |
return f"{int(hours)} hour and {int(minutes)} minutes" | |
else: | |
return f"{int(hours)} hours and {int(minutes)} minutes" | |
def inf_handler(audio, model_name): | |
model_found = False | |
for model_info in UVR_5_MODELS: | |
if model_info["model_name"] == model_name: | |
separator.load_model(model_info["checkpoint"]) | |
model_found = True | |
break | |
if not model_found: | |
separator.load_model() | |
output_files = separator.separate(audio) | |
vocals = output_files[0] | |
inst = output_files[1] | |
return vocals, inst | |
def run( | |
model, | |
audio_files, | |
pitch_alg, | |
pitch_lvl, | |
index_inf, | |
r_m_f, | |
e_r, | |
c_b_p, | |
): | |
if not audio_files: | |
raise ValueError("The audio pls") | |
if isinstance(audio_files, str): | |
audio_files = [audio_files] | |
try: | |
duration_base = librosa.get_duration(filename=audio_files[0]) | |
print("Duration:", duration_base) | |
except Exception as e: | |
print(e) | |
random_tag = "USER_"+str(random.randint(10000000, 99999999)) | |
file_m = model | |
print("File model:", file_m) | |
# get from MODELS | |
for model in MODELS: | |
if model["model_name"] == file_m: | |
print(model) | |
file_m = model["model"] | |
file_index = model["index"] | |
break | |
if not file_m.endswith(".pth"): | |
raise ValueError("The model file must be a .pth file") | |
print("Random tag:", random_tag) | |
print("File model:", file_m) | |
print("Pitch algorithm:", pitch_alg) | |
print("Pitch level:", pitch_lvl) | |
print("File index:", file_index) | |
print("Index influence:", index_inf) | |
print("Respiration median filtering:", r_m_f) | |
print("Envelope ratio:", e_r) | |
converter.apply_conf( | |
tag=random_tag, | |
file_model=file_m, | |
pitch_algo=pitch_alg, | |
pitch_lvl=pitch_lvl, | |
file_index=file_index, | |
index_influence=index_inf, | |
respiration_median_filtering=r_m_f, | |
envelope_ratio=e_r, | |
consonant_breath_protection=c_b_p, | |
resample_sr=44100 if audio_files[0].endswith('.mp3') else 0, | |
) | |
time.sleep(0.1) | |
result = convert_now(audio_files, random_tag, converter) | |
print("Result:", result) | |
return result[0] | |
def upload_model(index_file, pth_file, model_name): | |
pth_file = pth_file.name | |
index_file = index_file.name | |
MODELS.append({"model": pth_file, "index": index_file, "model_name": model_name}) | |
return "Uploaded!" | |
with gr.Blocks(theme=gr.themes.Default(primary_hue="pink", secondary_hue="rose"), title="Ilaria RVC 💖") as demo: | |
gr.Markdown("## Ilaria RVC 💖") | |
with gr.Tab("Inference"): | |
sound_gui = gr.Audio(value=None,type="filepath",autoplay=False,visible=True,) | |
def update(): | |
print(MODELS) | |
return gr.Dropdown(label="Model",choices=[model["model_name"] for model in MODELS],visible=True,interactive=True, value=MODELS[0]["model_name"],) | |
with gr.Row(): | |
models_dropdown = gr.Dropdown(label="Model",choices=[model["model_name"] for model in MODELS],visible=True,interactive=True, value=MODELS[0]["model_name"],) | |
refresh_button = gr.Button("Refresh Models") | |
refresh_button.click(update, outputs=[models_dropdown]) | |
with gr.Accordion("Ilaria TTS", open=False): | |
text_tts = gr.Textbox(label="Text", placeholder="Hello!", lines=3, interactive=True,) | |
dropdown_tts = gr.Dropdown(label="Language and Model",choices=list(language_dict.keys()),interactive=True, value=list(language_dict.keys())[0]) | |
button_tts = gr.Button("Speak", variant="primary",) | |
button_tts.click(text_to_speech_edge, inputs=[text_tts, dropdown_tts], outputs=[sound_gui]) | |
with gr.Accordion("Settings", open=False): | |
pitch_algo_conf = gr.Dropdown(PITCH_ALGO_OPT,value=PITCH_ALGO_OPT[4],label="Pitch algorithm",visible=True,interactive=True,) | |
pitch_lvl_conf = gr.Slider(label="Pitch level (lower -> 'male' while higher -> 'female')",minimum=-24,maximum=24,step=1,value=0,visible=True,interactive=True,) | |
index_inf_conf = gr.Slider(minimum=0,maximum=1,label="Index influence -> How much accent is applied",value=0.75,) | |
respiration_filter_conf = gr.Slider(minimum=0,maximum=7,label="Respiration median filtering",value=3,step=1,interactive=True,) | |
envelope_ratio_conf = gr.Slider(minimum=0,maximum=1,label="Envelope ratio",value=0.25,interactive=True,) | |
consonant_protec_conf = gr.Slider(minimum=0,maximum=0.5,label="Consonant breath protection",value=0.5,interactive=True,) | |
button_conf = gr.Button("Convert",variant="primary",) | |
output_conf = gr.Audio(type="filepath",label="Output",) | |
button_conf.click(lambda :None, None, output_conf) | |
button_conf.click( | |
run, | |
inputs=[ | |
models_dropdown, | |
sound_gui, | |
pitch_algo_conf, | |
pitch_lvl_conf, | |
index_inf_conf, | |
respiration_filter_conf, | |
envelope_ratio_conf, | |
consonant_protec_conf, | |
], | |
outputs=[output_conf], | |
) | |
with gr.Tab("Model Loader (Download and Upload)"): | |
with gr.Accordion("Model Downloader", open=False): | |
gr.Markdown( | |
"Download the model from the following URL and upload it here. (Huggingface RVC model)" | |
) | |
model = gr.Textbox(lines=1, label="Model URL") | |
name = gr.Textbox(lines=1, label="Model Name", placeholder="Model Name") | |
download_button = gr.Button("Download Model") | |
status = gr.Textbox(lines=1, label="Status", placeholder="Waiting....", interactive=False) | |
model_pth = gr.Textbox(lines=1, label="Model pth file", placeholder="Waiting....", interactive=False) | |
index_pth = gr.Textbox(lines=1, label="Index pth file", placeholder="Waiting....", interactive=False) | |
download_button.click(download_from_url, [model, name], outputs=[status, model_pth, index_pth]) | |
with gr.Accordion("Upload A Model", open=False): | |
index_file_upload = gr.File(label="Index File (.index)") | |
pth_file_upload = gr.File(label="Model File (.pth)") | |
model_name = gr.Textbox(label="Model Name", placeholder="Model Name") | |
upload_button = gr.Button("Upload Model") | |
upload_status = gr.Textbox(lines=1, label="Status", placeholder="Waiting....", interactive=False) | |
upload_button.click(upload_model, [index_file_upload, pth_file_upload, model_name], upload_status) | |
with gr.Tab("Vocal Separator (UVR)"): | |
gr.Markdown("Separate vocals and instruments from an audio file using UVR models. - This is only on CPU due to ZeroGPU being ZeroGPU :(") | |
uvr5_audio_file = gr.Audio(label="Audio File",type="filepath") | |
with gr.Row(): | |
uvr5_model = gr.Dropdown(label="Model", choices=[model["model_name"] for model in UVR_5_MODELS]) | |
uvr5_button = gr.Button("Separate Vocals", variant="primary",) | |
uvr5_output_voc = gr.Audio(type="filepath", label="Output 1",) | |
uvr5_output_inst = gr.Audio(type="filepath", label="Output 2",) | |
uvr5_button.click(inference, [uvr5_audio_file, uvr5_model], [uvr5_output_voc, uvr5_output_inst]) | |
with gr.Tab("Extra"): | |
with gr.Accordion("Model Information", open=False): | |
def json_to_markdown_table(json_data): | |
table = "| Key | Value |\n| --- | --- |\n" | |
for key, value in json_data.items(): | |
table += f"| {key} | {value} |\n" | |
return table | |
def model_info(name): | |
for model in MODELS: | |
if model["model_name"] == name: | |
print(model["model"]) | |
info = model_handler.model_info(model["model"]) | |
info2 = { | |
"Model Name": model["model_name"], | |
"Model Config": info['config'], | |
"Epochs Trained": info['epochs'], | |
"Sample Rate": info['sr'], | |
"Pitch Guidance": info['f0'], | |
"Model Precision": info['size'], | |
} | |
return gr.Markdown(json_to_markdown_table(info2)) | |
return "Model not found" | |
def update(): | |
print(MODELS) | |
return gr.Dropdown(label="Model", choices=[model["model_name"] for model in MODELS]) | |
with gr.Row(): | |
model_info_dropdown = gr.Dropdown(label="Model", choices=[model["model_name"] for model in MODELS]) | |
refresh_button = gr.Button("Refresh Models") | |
refresh_button.click(update, outputs=[model_info_dropdown]) | |
model_info_button = gr.Button("Get Model Information") | |
model_info_output = gr.Textbox(value="Waiting...",label="Output", interactive=False) | |
model_info_button.click(model_info, [model_info_dropdown], [model_info_output]) | |
with gr.Accordion("Training Time Calculator", open=False): | |
with gr.Column(): | |
epochs_input = gr.Number(label="Number of Epochs") | |
seconds_input = gr.Number(label="Seconds per Epoch") | |
calculate_button = gr.Button("Calculate Time Remaining") | |
remaining_time_output = gr.Textbox(label="Remaining Time", interactive=False) | |
calculate_button.click(calculate_remaining_time,inputs=[epochs_input, seconds_input],outputs=[remaining_time_output]) | |
with gr.Accordion("Model Fusion", open=False): | |
with gr.Group(): | |
def merge(ckpt_a, ckpt_b, alpha_a, sr_, if_f0_, info__, name_to_save0, version_2): | |
for model in MODELS: | |
if model["model_name"] == ckpt_a: | |
ckpt_a = model["model"] | |
if model["model_name"] == ckpt_b: | |
ckpt_b = model["model"] | |
path = model_handler.merge(ckpt_a, ckpt_b, alpha_a, sr_, if_f0_, info__, name_to_save0, version_2) | |
if path == "Fail to merge the models. The model architectures are not the same.": | |
return "Fail to merge the models. The model architectures are not the same." | |
else: | |
MODELS.append({"model": path, "index": None, "model_name": name_to_save0}) | |
return "Merged, saved as " + name_to_save0 | |
gr.Markdown(value="Strongly suggested to use only very clean models.") | |
with gr.Row(): | |
def update(): | |
print(MODELS) | |
return gr.Dropdown(label="Model A", choices=[model["model_name"] for model in MODELS]), gr.Dropdown(label="Model B", choices=[model["model_name"] for model in MODELS]) | |
refresh_button_fusion = gr.Button("Refresh Models") | |
ckpt_a = gr.Dropdown(label="Model A", choices=[model["model_name"] for model in MODELS]) | |
ckpt_b = gr.Dropdown(label="Model B", choices=[model["model_name"] for model in MODELS]) | |
refresh_button_fusion.click(update, outputs=[ckpt_a, ckpt_b]) | |
alpha_a = gr.Slider( | |
minimum=0, | |
maximum=1, | |
label="Weight of the first model over the second", | |
value=0.5, | |
interactive=True, | |
) | |
with gr.Group(): | |
with gr.Row(): | |
sr_ = gr.Radio( | |
label="Sample rate of both models", | |
choices=["32k","40k", "48k"], | |
value="32k", | |
interactive=True, | |
) | |
if_f0_ = gr.Radio( | |
label="Pitch Guidance", | |
choices=["Yes", "Nah"], | |
value="Yes", | |
interactive=True, | |
) | |
info__ = gr.Textbox( | |
label="Add informations to the model", | |
value="", | |
max_lines=8, | |
interactive=True, | |
visible=False | |
) | |
name_to_save0 = gr.Textbox( | |
label="Final Model name", | |
value="", | |
max_lines=1, | |
interactive=True, | |
) | |
version_2 = gr.Radio( | |
label="Versions of the models", | |
choices=["v1", "v2"], | |
value="v2", | |
interactive=True, | |
) | |
with gr.Group(): | |
with gr.Row(): | |
but6 = gr.Button("Fuse the two models", variant="primary") | |
info4 = gr.Textbox(label="Output", value="", max_lines=8) | |
but6.click( | |
merge, | |
[ckpt_a,ckpt_b,alpha_a,sr_,if_f0_,info__,name_to_save0,version_2,],info4,api_name="ckpt_merge",) | |
with gr.Accordion("Model Quantization", open=False): | |
gr.Markdown("Quantize the model to a lower precision. - soon™ or never™ 😎") | |
with gr.Accordion("Debug", open=False): | |
def json_to_markdown_table(json_data): | |
table = "| Key | Value |\n| --- | --- |\n" | |
for key, value in json_data.items(): | |
table += f"| {key} | {value} |\n" | |
return table | |
gr.Markdown("View the models that are currently loaded in the instance.") | |
gr.Markdown(json_to_markdown_table({"Models": len(MODELS), "UVR Models": len(UVR_5_MODELS)})) | |
gr.Markdown("View the current status of the instance.") | |
status = { | |
"Status": "Running", # duh lol | |
"Models": len(MODELS), | |
"UVR Models": len(UVR_5_MODELS), | |
"CPU Usage": f"{psutil.cpu_percent()}%", | |
"RAM Usage": f"{psutil.virtual_memory().percent}%", | |
"CPU": f"{cpuinfo.get_cpu_info()['brand_raw']}", | |
"System Uptime": f"{round(time.time() - psutil.boot_time(), 2)} seconds", | |
"System Load Average": f"{psutil.getloadavg()}", | |
"====================": "====================", | |
"CPU Cores": psutil.cpu_count(), | |
"CPU Threads": psutil.cpu_count(logical=True), | |
"RAM Total": f"{round(psutil.virtual_memory().total / 1024**3, 2)} GB", | |
"RAM Used": f"{round(psutil.virtual_memory().used / 1024**3, 2)} GB", | |
"CPU Frequency": f"{psutil.cpu_freq().current} MHz", | |
"====================": "====================", | |
"GPU": "A100 - Do a request (Inference, you won't see it either way)", | |
} | |
gr.Markdown(json_to_markdown_table(status)) | |
with gr.Tab("Credits"): | |
gr.Markdown( | |
""" | |
Ilaria RVC made by [Ilaria](https://huggingface.co/TheStinger) suport her on [ko-fi](https://ko-fi.com/ilariaowo) | |
The Inference code is made by [r3gm](https://huggingface.co/r3gm) (his module helped form this space 💖) | |
made with ❤️ by [mikus](https://github.com/cappuch) - made the ui! | |
## In loving memory of JLabDX 🕊️ | |
""" | |
) | |
with gr.Tab(("")): | |
gr.Markdown(''' | |
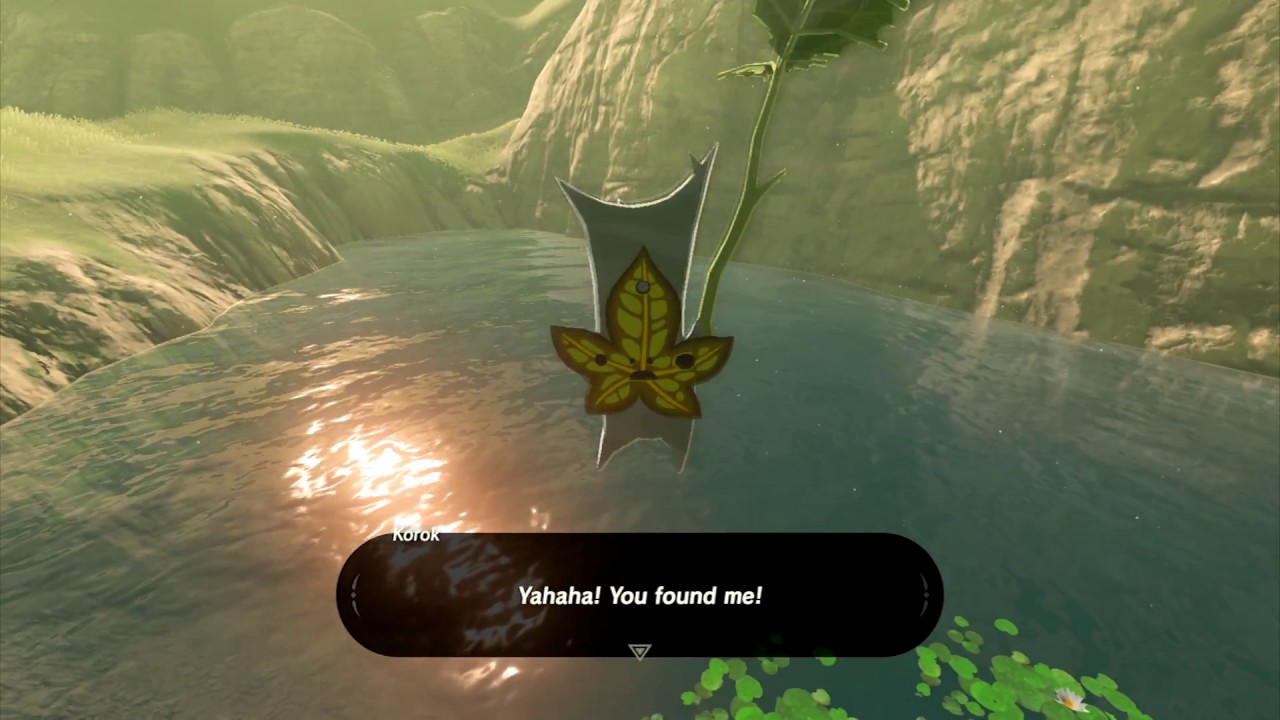 | |
''') | |
demo.queue(api_open=False).launch(show_api=False) | |