Spaces:
Build error
Build error
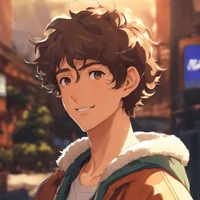
chore: Update import statement for InpaintingRequest in painting.py and refactor code to use shared BaseModel for Painting and InpaintingRequest classes
47b9b86
import gradio as gr | |
import requests | |
from pydantic import BaseModel | |
from diffusers.utils import load_image | |
from io import BytesIO | |
sdxl_inference_endpoint = 'http://127.0.0.1:8000/api/v1/product-diffusion/sdxl_v0_lora_inference' | |
sdxl_batch_inference_endpoint = 'http://127.0.0.1:8000/api/v1/product-diffusion/sdxl_v0_lora_inference/batch' | |
kandinsky_inpainting_inference = 'http://127.0.0.1:8000/api/v1/product-diffusion/kandinskyv2.2_inpainting' | |
# Define the InpaintingRequest model | |
class InputRequest(BaseModel): | |
prompt: str | |
num_inference_steps: int | |
guidance_scale: float | |
negative_prompt: str | |
num_images: int | |
mode: str | |
class InpaintingRequest(BaseModel): | |
prompt: str | |
negative_prompt: str | |
num_inference_steps: int | |
strength: float | |
guidance_scale: float | |
mode: str | |
async def generate_sdxl_lora_image(prompt, negative_prompt, num_inference_steps, guidance_scale, num_images, mode): | |
# Prepare the payload for SDXL LORA API | |
payload = InputRequest( | |
prompt=prompt, | |
negative_prompt=negative_prompt, | |
num_inference_steps=num_inference_steps, | |
guidance_scale=guidance_scale, | |
num_images=num_images, | |
mode=mode | |
).dict() | |
response = requests.post(sdxl_inference_endpoint, json=payload) | |
response = response.json() | |
url = response['url'] | |
image = load_image(url) | |
return image | |
def generate_outpainting(prompt, negative_prompt, num_inference_steps, strength, guidance_scale, mode, image): | |
# Convert the image to bytes | |
img_byte_arr = BytesIO() | |
image.save(img_byte_arr, format='PNG') | |
img_byte_arr = img_byte_arr.getvalue() | |
# Prepare the payload for multipart/form-data | |
files = { | |
'image': ('image.png', img_byte_arr, 'image/png'), | |
'prompt': (None, prompt), | |
'negative_prompt': (None, negative_prompt), | |
'num_inference_steps': (None, str(num_inference_steps)), | |
'strength': (None, str(strength)), | |
'guidance_scale': (None, str(guidance_scale)), | |
'mode': (None, mode) | |
} | |
response = requests.post(kandinsky_inpainting_inference, files=files) | |
response.raise_for_status() | |
response = response.json() | |
url = response['url'] | |
image = load_image(url) | |
return image | |
with gr.Blocks(theme='VikramSingh178/Webui-Theme') as demo: | |
with gr.Tab("SdxL-Lora"): | |
with gr.Row(): | |
with gr.Column(): | |
with gr.Group(): | |
prompt = gr.Textbox(label="Prompt", placeholder="Enter your prompt here") | |
negative_prompt = gr.Textbox(label="Negative Prompt", placeholder="Enter negative prompt here") | |
num_inference_steps = gr.Slider(minimum=1, maximum=1000, step=1, value=20, label="Inference Steps") | |
guidance_scale = gr.Slider(minimum=1.0, maximum=10.0, step=0.1, value=7.5, label="Guidance Scale") | |
num_images = gr.Slider(minimum=1, maximum=10, step=1, value=1, label="Number of Images") | |
mode = gr.Dropdown(choices=["s3_json", "b64_json"], value="s3_json", label="Mode") | |
generate_button = gr.Button("Generate Image",variant='primary') | |
with gr.Column(scale=1): | |
image_preview = gr.Image(label="Generated Image",show_download_button=True,show_share_button=True,container=True) | |
generate_button.click(generate_sdxl_lora_image, inputs=[prompt, negative_prompt, num_inference_steps, guidance_scale, num_images, mode], outputs=[image_preview]) | |
with gr.Tab("Generate AI Background"): | |
with gr.Row(): | |
with gr.Column(): | |
with gr.Group(): | |
image_input = gr.Image(type="pil", label="Upload Image") | |
prompt = gr.Textbox(label="Prompt", placeholder="Enter your prompt here") | |
negative_prompt = gr.Textbox(label="Negative Prompt", placeholder="Enter negative prompt here") | |
num_inference_steps = gr.Slider(minimum=1, maximum=500, step=1, value=20, label="Inference Steps") | |
guidance_scale = gr.Slider(minimum=1.0, maximum=10.0, step=0.1, value=7.5, label="Guidance Scale") | |
strength = gr.Slider(minimum=0.1, maximum=1, step=0.1, value=1, label="Strength") | |
mode = gr.Dropdown(choices=["s3_json", "b64_json"], value="s3_json", label="Mode") | |
generate_button = gr.Button("Generate Background", variant='primary') | |
with gr.Column(scale=1): | |
image_preview = gr.Image(label="Image", show_download_button=True, show_share_button=True, container=True) | |
generate_button.click(generate_outpainting, inputs=[prompt, negative_prompt, num_inference_steps, strength, guidance_scale, mode, image_input], outputs=[image_preview]) | |
demo.launch() | |