# Embodied acting and speaking
This code was based on these repositories:
[`gym-minigrid`](https://github.com/maximecb/gym-minigrid)
[`torch-ac`](https://github.com/lcswillems/torch-ac)
[`rl-starter-files`](add_url)
## Features
- **Script to train**, including:
- Log in txt, CSV and Tensorboard
- Save model
- Stop and restart training
- Use A2C or PPO algorithms
- **Script to visualize**, including:
- Act by sampling or argmax
- Save as Gif
- **Script to evaluate**, including:
- Act by sampling or argmax
- List the worst performed episodes
## Installation
### Option 1
[comment]: <> todo: add this part
[comment]: <> (Clone the repo)
[comment]: <> (```)
[comment]: <> (git clone https://gitlab.inria.fr/gkovac/act-and-speak.git)
[comment]: <> (```)
Create and activate your conda env
```
conda create --name act_and_speak python=3.6
conda activate act_and_speak
```
Install the required packages
```
pip install -r requirements.txt
pip install -e torch-ac
pip install -e gym-minigrid --use-feature=2020-resolver
```
### Option 2
Alternative use the conda yaml file:
```
TODO:
```
## Example of use
Train, visualize and evaluate an agent on the `MiniGrid-DoorKey-5x5-v0` environment:
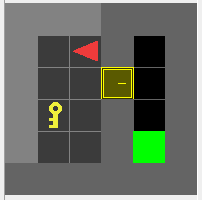
1. Train the agent on the `MiniGrid-DoorKey-5x5-v0` environment with PPO algorithm:
```
python3 -m scripts.train --algo ppo --env MiniGrid-DoorKey-5x5-v0 --model DoorKey --save-interval 10 --frames 80000
```

2. Visualize agent's behavior:
```
python3 -m scripts.visualize --env MiniGrid-DoorKey-5x5-v0 --model DoorKey
```

3. Evaluate agent's performance:
```
python3 -m scripts.evaluate --env MiniGrid-DoorKey-5x5-v0 --model DoorKey
```

**Note:** More details on the commands are given below.
## Other examples
### Handle textual instructions
In the `GoToDoor` environment, the agent receives an image along with a textual instruction. To handle the latter, add `--text` to the command:
```
python3 -m scripts.train --algo ppo --env MiniGrid-GoToDoor-5x5-v0 --model GoToDoor --text --save-interval 10 --frames 1000000
```

### Handle dialogue with multi a multi headed agent
In the `GoToDoorTalk` environment, the agent receives an image along with the dialogue. To handle the latter, add `--dialogue` and, to use the multi headed agent, add `--multi-headed-agent` to the command:
```
python3 -m scripts.train --algo ppo --env MiniGrid-GoToDoorTalk-5x5-v0 --model GoToDoorMultiHead --dialogue --multi-headed-agent --save-interval 10 --frames 1000000
```
### Add memory
In the `RedBlueDoors` environment, the agent has to open the red door then the blue one. To solve it efficiently, when it opens the red door, it has to remember it. To add memory to the agent, add `--recurrence X` to the command:
```
python3 -m scripts.train --algo ppo --env MiniGrid-RedBlueDoors-6x6-v0 --model RedBlueDoors --recurrence 4 --save-interval 10 --frames 1000000
```

## Files
This package contains:
- scripts to:
- train an agent \
in `script/train.py` ([more details](#scripts-train))
- visualize agent's behavior \
in `script/visualize.py` ([more details](#scripts-visualize))
- evaluate agent's performances \
in `script/evaluate.py` ([more details](#scripts-evaluate))
- a default agent's model \
in `model.py` ([more details](#model))
- utilitarian classes and functions used by the scripts \
in `utils`
These files are suited for [`gym-minigrid`](https://github.com/maximecb/gym-minigrid) environments and [`torch-ac`](https://github.com/lcswillems/torch-ac) RL algorithms. They are easy to adapt to other environments and RL algorithms by modifying:
- `model.py`
- `utils/format.py`
scripts/train.py
An example of use:
```bash
python3 -m scripts.train --algo ppo --env MiniGrid-DoorKey-5x5-v0 --model DoorKey --save-interval 10 --frames 80000
```
The script loads the model in `storage/DoorKey` or creates it if it doesn't exist, then trains it with the PPO algorithm on the MiniGrid DoorKey environment, and saves it every 10 updates in `storage/DoorKey`. It stops after 80 000 frames.
**Note:** You can define a different storage location in the environment variable `PROJECT_STORAGE`.
More generally, the script has 2 required arguments:
- `--algo ALGO`: name of the RL algorithm used to train
- `--env ENV`: name of the environment to train on
and a bunch of optional arguments among which:
- `--recurrence N`: gradient will be backpropagated over N timesteps. By default, N = 1. If N > 1, a LSTM is added to the model to have memory.
- `--text`: a GRU is added to the model to handle text input.
- ... (see more using `--help`)
During training, logs are printed in your terminal (and saved in text and CSV format):

**Note:** `U` gives the update number, `F` the total number of frames, `FPS` the number of frames per second, `D` the total duration, `rR:μσmM` the mean, std, min and max reshaped return per episode, `F:μσmM` the mean, std, min and max number of frames per episode, `H` the entropy, `V` the value, `pL` the policy loss, `vL` the value loss and `∇` the gradient norm.
During training, logs are also plotted in Tensorboard:
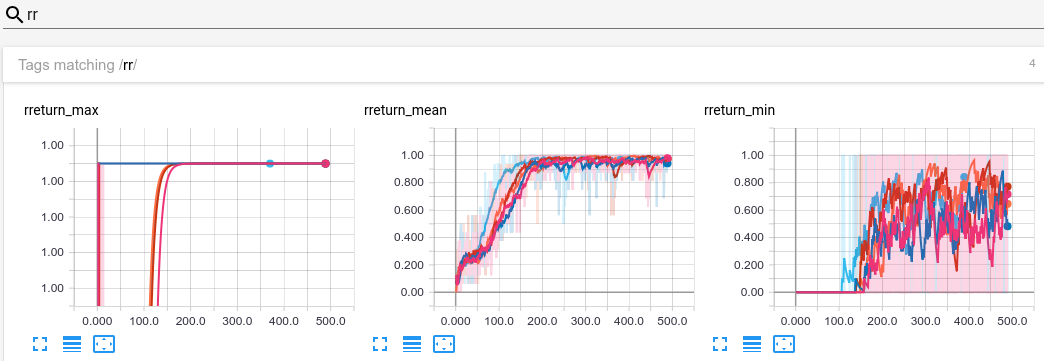
scripts/visualize.py
An example of use:
```
python3 -m scripts.visualize --env MiniGrid-DoorKey-5x5-v0 --model DoorKey
```

In this use case, the script displays how the model in `storage/DoorKey` behaves on the MiniGrid DoorKey environment.
More generally, the script has 2 required arguments:
- `--env ENV`: name of the environment to act on.
- `--model MODEL`: name of the trained model.
and a bunch of optional arguments among which:
- `--argmax`: select the action with highest probability
- ... (see more using `--help`)
scripts/evaluate.py
An example of use:
```
python3 -m scripts.evaluate --env MiniGrid-DoorKey-5x5-v0 --model DoorKey
```

In this use case, the script prints in the terminal the performance among 100 episodes of the model in `storage/DoorKey`.
More generally, the script has 2 required arguments:
- `--env ENV`: name of the environment to act on.
- `--model MODEL`: name of the trained model.
and a bunch of optional arguments among which:
- `--episodes N`: number of episodes of evaluation. By default, N = 100.
- ... (see more using `--help`)
model.py
The default model is discribed by the following schema:
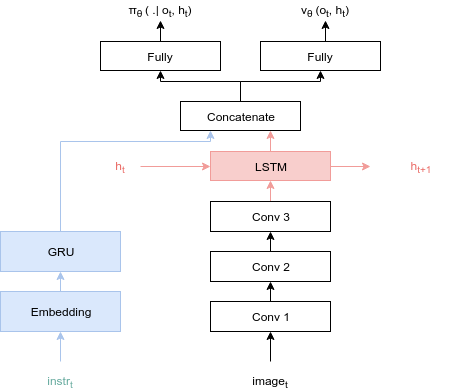
By default, the memory part (in red) and the langage part (in blue) are disabled. They can be enabled by setting to `True` the `use_memory` and `use_text` parameters of the model constructor.
This model can be easily adapted to your needs.