Spaces:
Sleeping
Sleeping
Commit
•
339f0eb
1
Parent(s):
d7ea51a
Upload 457 files
Browse filesThis view is limited to 50 files because it contains too many changes.
See raw diff
- ultralytics/.github/ISSUE_TEMPLATE/bug-report.yml +96 -0
- ultralytics/.github/ISSUE_TEMPLATE/config.yml +11 -0
- ultralytics/.github/ISSUE_TEMPLATE/feature-request.yml +50 -0
- ultralytics/.github/ISSUE_TEMPLATE/question.yml +33 -0
- ultralytics/.github/dependabot.yml +27 -0
- ultralytics/.github/translate-readme.yml +26 -0
- ultralytics/.github/workflows/ci.yaml +320 -0
- ultralytics/.github/workflows/cla.yml +37 -0
- ultralytics/.github/workflows/codeql.yaml +42 -0
- ultralytics/.github/workflows/docker.yaml +148 -0
- ultralytics/.github/workflows/greetings.yml +58 -0
- ultralytics/.github/workflows/links.yml +45 -0
- ultralytics/.github/workflows/publish.yml +109 -0
- ultralytics/.github/workflows/stale.yml +47 -0
- ultralytics/.gitignore +165 -0
- ultralytics/.pre-commit-config.yaml +88 -0
- ultralytics/CITATION.cff +20 -0
- ultralytics/CONTRIBUTING.md +115 -0
- ultralytics/LICENSE +661 -0
- ultralytics/MANIFEST.in +8 -0
- ultralytics/README.md +276 -0
- ultralytics/README.zh-CN.md +275 -0
- ultralytics/docker/Dockerfile +82 -0
- ultralytics/docker/Dockerfile-arm64 +44 -0
- ultralytics/docker/Dockerfile-conda +38 -0
- ultralytics/docker/Dockerfile-cpu +55 -0
- ultralytics/docker/Dockerfile-jetson +48 -0
- ultralytics/docker/Dockerfile-python +52 -0
- ultralytics/docker/Dockerfile-runner +38 -0
- ultralytics/docs/CNAME +1 -0
- ultralytics/docs/README.md +73 -0
- ultralytics/docs/SECURITY.md +26 -0
- ultralytics/docs/assets/favicon.ico +0 -0
- ultralytics/docs/build_reference.py +127 -0
- ultralytics/docs/datasets/classify/caltech101.md +81 -0
- ultralytics/docs/datasets/classify/caltech256.md +78 -0
- ultralytics/docs/datasets/classify/cifar10.md +80 -0
- ultralytics/docs/datasets/classify/cifar100.md +80 -0
- ultralytics/docs/datasets/classify/fashion-mnist.md +79 -0
- ultralytics/docs/datasets/classify/imagenet.md +83 -0
- ultralytics/docs/datasets/classify/imagenet10.md +78 -0
- ultralytics/docs/datasets/classify/imagenette.md +113 -0
- ultralytics/docs/datasets/classify/imagewoof.md +84 -0
- ultralytics/docs/datasets/classify/index.md +120 -0
- ultralytics/docs/datasets/classify/mnist.md +86 -0
- ultralytics/docs/datasets/detect/argoverse.md +97 -0
- ultralytics/docs/datasets/detect/coco.md +94 -0
- ultralytics/docs/datasets/detect/coco8.md +80 -0
- ultralytics/docs/datasets/detect/globalwheat2020.md +91 -0
- ultralytics/docs/datasets/detect/index.md +108 -0
ultralytics/.github/ISSUE_TEMPLATE/bug-report.yml
ADDED
@@ -0,0 +1,96 @@
|
|
|
|
|
|
|
|
|
|
|
|
|
|
|
|
|
|
|
|
|
|
|
|
|
|
|
|
|
|
|
|
|
|
|
|
|
|
|
|
|
|
|
|
|
|
|
|
|
|
|
|
|
|
|
|
|
|
|
|
|
|
|
|
|
|
|
|
|
|
|
|
|
|
|
|
|
|
|
|
|
|
|
|
|
|
|
|
|
|
|
|
|
|
|
|
|
|
|
|
|
|
|
|
|
|
|
|
|
|
|
|
|
|
|
|
|
|
|
|
|
|
|
|
|
|
|
|
|
|
|
|
|
|
|
|
|
|
|
|
|
|
|
|
|
|
|
|
|
|
|
|
|
|
|
|
|
|
|
|
|
|
|
|
|
|
|
|
|
|
|
|
|
|
|
|
|
|
|
|
|
|
|
|
|
|
|
|
|
|
|
|
|
|
|
1 |
+
name: 🐛 Bug Report
|
2 |
+
# title: " "
|
3 |
+
description: Problems with YOLOv8
|
4 |
+
labels: [bug, triage]
|
5 |
+
body:
|
6 |
+
- type: markdown
|
7 |
+
attributes:
|
8 |
+
value: |
|
9 |
+
Thank you for submitting a YOLOv8 🐛 Bug Report!
|
10 |
+
|
11 |
+
- type: checkboxes
|
12 |
+
attributes:
|
13 |
+
label: Search before asking
|
14 |
+
description: >
|
15 |
+
Please search the [issues](https://github.com/ultralytics/ultralytics/issues) to see if a similar bug report already exists.
|
16 |
+
options:
|
17 |
+
- label: >
|
18 |
+
I have searched the YOLOv8 [issues](https://github.com/ultralytics/ultralytics/issues) and found no similar bug report.
|
19 |
+
required: true
|
20 |
+
|
21 |
+
- type: dropdown
|
22 |
+
attributes:
|
23 |
+
label: YOLOv8 Component
|
24 |
+
description: |
|
25 |
+
Please select the part of YOLOv8 where you found the bug.
|
26 |
+
multiple: true
|
27 |
+
options:
|
28 |
+
- "Install"
|
29 |
+
- "Train"
|
30 |
+
- "Val"
|
31 |
+
- "Predict"
|
32 |
+
- "Export"
|
33 |
+
- "Multi-GPU"
|
34 |
+
- "Augmentation"
|
35 |
+
- "Hyperparameter Tuning"
|
36 |
+
- "Integrations"
|
37 |
+
- "Other"
|
38 |
+
validations:
|
39 |
+
required: false
|
40 |
+
|
41 |
+
- type: textarea
|
42 |
+
attributes:
|
43 |
+
label: Bug
|
44 |
+
description: Provide console output with error messages and/or screenshots of the bug.
|
45 |
+
placeholder: |
|
46 |
+
💡 ProTip! Include as much information as possible (screenshots, logs, tracebacks etc.) to receive the most helpful response.
|
47 |
+
validations:
|
48 |
+
required: true
|
49 |
+
|
50 |
+
- type: textarea
|
51 |
+
attributes:
|
52 |
+
label: Environment
|
53 |
+
description: Please specify the software and hardware you used to produce the bug.
|
54 |
+
placeholder: |
|
55 |
+
Paste output of `yolo checks` or `ultralytics.checks()` command, i.e.:
|
56 |
+
```
|
57 |
+
Ultralytics YOLOv8.0.181 🚀 Python-3.11.2 torch-2.0.1 CPU (Apple M2)
|
58 |
+
Setup complete ✅ (8 CPUs, 16.0 GB RAM, 266.5/460.4 GB disk)
|
59 |
+
|
60 |
+
OS macOS-13.5.2
|
61 |
+
Environment Jupyter
|
62 |
+
Python 3.11.2
|
63 |
+
Install git
|
64 |
+
RAM 16.00 GB
|
65 |
+
CPU Apple M2
|
66 |
+
CUDA None
|
67 |
+
```
|
68 |
+
validations:
|
69 |
+
required: false
|
70 |
+
|
71 |
+
- type: textarea
|
72 |
+
attributes:
|
73 |
+
label: Minimal Reproducible Example
|
74 |
+
description: >
|
75 |
+
When asking a question, people will be better able to provide help if you provide code that they can easily understand and use to **reproduce** the problem.
|
76 |
+
This is referred to by community members as creating a [minimal reproducible example](https://docs.ultralytics.com/help/minimum_reproducible_example/).
|
77 |
+
placeholder: |
|
78 |
+
```
|
79 |
+
# Code to reproduce your issue here
|
80 |
+
```
|
81 |
+
validations:
|
82 |
+
required: false
|
83 |
+
|
84 |
+
- type: textarea
|
85 |
+
attributes:
|
86 |
+
label: Additional
|
87 |
+
description: Anything else you would like to share?
|
88 |
+
|
89 |
+
- type: checkboxes
|
90 |
+
attributes:
|
91 |
+
label: Are you willing to submit a PR?
|
92 |
+
description: >
|
93 |
+
(Optional) We encourage you to submit a [Pull Request](https://github.com/ultralytics/ultralytics/pulls) (PR) to help improve YOLOv8 for everyone, especially if you have a good understanding of how to implement a fix or feature.
|
94 |
+
See the YOLOv8 [Contributing Guide](https://docs.ultralytics.com/help/contributing) to get started.
|
95 |
+
options:
|
96 |
+
- label: Yes I'd like to help by submitting a PR!
|
ultralytics/.github/ISSUE_TEMPLATE/config.yml
ADDED
@@ -0,0 +1,11 @@
|
|
|
|
|
|
|
|
|
|
|
|
|
|
|
|
|
|
|
|
|
|
|
|
|
1 |
+
blank_issues_enabled: true
|
2 |
+
contact_links:
|
3 |
+
- name: 📄 Docs
|
4 |
+
url: https://docs.ultralytics.com/
|
5 |
+
about: Full Ultralytics YOLOv8 Documentation
|
6 |
+
- name: 💬 Forum
|
7 |
+
url: https://community.ultralytics.com/
|
8 |
+
about: Ask on Ultralytics Community Forum
|
9 |
+
- name: 🎧 Discord
|
10 |
+
url: https://ultralytics.com/discord
|
11 |
+
about: Ask on Ultralytics Discord
|
ultralytics/.github/ISSUE_TEMPLATE/feature-request.yml
ADDED
@@ -0,0 +1,50 @@
|
|
|
|
|
|
|
|
|
|
|
|
|
|
|
|
|
|
|
|
|
|
|
|
|
|
|
|
|
|
|
|
|
|
|
|
|
|
|
|
|
|
|
|
|
|
|
|
|
|
|
|
|
|
|
|
|
|
|
|
|
|
|
|
|
|
|
|
|
|
|
|
|
|
|
|
|
|
|
|
|
|
|
|
|
|
|
|
|
|
|
|
|
|
|
|
|
|
|
|
|
|
|
1 |
+
name: 🚀 Feature Request
|
2 |
+
description: Suggest a YOLOv8 idea
|
3 |
+
# title: " "
|
4 |
+
labels: [enhancement]
|
5 |
+
body:
|
6 |
+
- type: markdown
|
7 |
+
attributes:
|
8 |
+
value: |
|
9 |
+
Thank you for submitting a YOLOv8 🚀 Feature Request!
|
10 |
+
|
11 |
+
- type: checkboxes
|
12 |
+
attributes:
|
13 |
+
label: Search before asking
|
14 |
+
description: >
|
15 |
+
Please search the [issues](https://github.com/ultralytics/ultralytics/issues) to see if a similar feature request already exists.
|
16 |
+
options:
|
17 |
+
- label: >
|
18 |
+
I have searched the YOLOv8 [issues](https://github.com/ultralytics/ultralytics/issues) and found no similar feature requests.
|
19 |
+
required: true
|
20 |
+
|
21 |
+
- type: textarea
|
22 |
+
attributes:
|
23 |
+
label: Description
|
24 |
+
description: A short description of your feature.
|
25 |
+
placeholder: |
|
26 |
+
What new feature would you like to see in YOLOv8?
|
27 |
+
validations:
|
28 |
+
required: true
|
29 |
+
|
30 |
+
- type: textarea
|
31 |
+
attributes:
|
32 |
+
label: Use case
|
33 |
+
description: |
|
34 |
+
Describe the use case of your feature request. It will help us understand and prioritize the feature request.
|
35 |
+
placeholder: |
|
36 |
+
How would this feature be used, and who would use it?
|
37 |
+
|
38 |
+
- type: textarea
|
39 |
+
attributes:
|
40 |
+
label: Additional
|
41 |
+
description: Anything else you would like to share?
|
42 |
+
|
43 |
+
- type: checkboxes
|
44 |
+
attributes:
|
45 |
+
label: Are you willing to submit a PR?
|
46 |
+
description: >
|
47 |
+
(Optional) We encourage you to submit a [Pull Request](https://github.com/ultralytics/ultralytics/pulls) (PR) to help improve YOLOv8 for everyone, especially if you have a good understanding of how to implement a fix or feature.
|
48 |
+
See the YOLOv8 [Contributing Guide](https://docs.ultralytics.com/help/contributing) to get started.
|
49 |
+
options:
|
50 |
+
- label: Yes I'd like to help by submitting a PR!
|
ultralytics/.github/ISSUE_TEMPLATE/question.yml
ADDED
@@ -0,0 +1,33 @@
|
|
|
|
|
|
|
|
|
|
|
|
|
|
|
|
|
|
|
|
|
|
|
|
|
|
|
|
|
|
|
|
|
|
|
|
|
|
|
|
|
|
|
|
|
|
|
|
|
|
|
|
|
|
|
|
|
|
|
|
|
|
|
|
|
|
|
|
|
1 |
+
name: ❓ Question
|
2 |
+
description: Ask a YOLOv8 question
|
3 |
+
# title: " "
|
4 |
+
labels: [question]
|
5 |
+
body:
|
6 |
+
- type: markdown
|
7 |
+
attributes:
|
8 |
+
value: |
|
9 |
+
Thank you for asking a YOLOv8 ❓ Question!
|
10 |
+
|
11 |
+
- type: checkboxes
|
12 |
+
attributes:
|
13 |
+
label: Search before asking
|
14 |
+
description: >
|
15 |
+
Please search the [issues](https://github.com/ultralytics/ultralytics/issues) and [discussions](https://github.com/ultralytics/ultralytics/discussions) to see if a similar question already exists.
|
16 |
+
options:
|
17 |
+
- label: >
|
18 |
+
I have searched the YOLOv8 [issues](https://github.com/ultralytics/ultralytics/issues) and [discussions](https://github.com/ultralytics/ultralytics/discussions) and found no similar questions.
|
19 |
+
required: true
|
20 |
+
|
21 |
+
- type: textarea
|
22 |
+
attributes:
|
23 |
+
label: Question
|
24 |
+
description: What is your question?
|
25 |
+
placeholder: |
|
26 |
+
💡 ProTip! Include as much information as possible (screenshots, logs, tracebacks etc.) to receive the most helpful response.
|
27 |
+
validations:
|
28 |
+
required: true
|
29 |
+
|
30 |
+
- type: textarea
|
31 |
+
attributes:
|
32 |
+
label: Additional
|
33 |
+
description: Anything else you would like to share?
|
ultralytics/.github/dependabot.yml
ADDED
@@ -0,0 +1,27 @@
|
|
|
|
|
|
|
|
|
|
|
|
|
|
|
|
|
|
|
|
|
|
|
|
|
|
|
|
|
|
|
|
|
|
|
|
|
|
|
|
|
|
|
|
|
|
|
|
|
|
|
|
|
|
|
|
|
1 |
+
# Ultralytics YOLO 🚀, AGPL-3.0 license
|
2 |
+
# Dependabot for package version updates
|
3 |
+
# https://docs.github.com/github/administering-a-repository/configuration-options-for-dependency-updates
|
4 |
+
|
5 |
+
version: 2
|
6 |
+
updates:
|
7 |
+
- package-ecosystem: pip
|
8 |
+
directory: "/"
|
9 |
+
schedule:
|
10 |
+
interval: weekly
|
11 |
+
time: "04:00"
|
12 |
+
open-pull-requests-limit: 10
|
13 |
+
reviewers:
|
14 |
+
- glenn-jocher
|
15 |
+
labels:
|
16 |
+
- dependencies
|
17 |
+
|
18 |
+
- package-ecosystem: github-actions
|
19 |
+
directory: "/"
|
20 |
+
schedule:
|
21 |
+
interval: weekly
|
22 |
+
time: "04:00"
|
23 |
+
open-pull-requests-limit: 5
|
24 |
+
reviewers:
|
25 |
+
- glenn-jocher
|
26 |
+
labels:
|
27 |
+
- dependencies
|
ultralytics/.github/translate-readme.yml
ADDED
@@ -0,0 +1,26 @@
|
|
|
|
|
|
|
|
|
|
|
|
|
|
|
|
|
|
|
|
|
|
|
|
|
|
|
|
|
|
|
|
|
|
|
|
|
|
|
|
|
|
|
|
|
|
|
|
|
|
|
|
|
|
|
1 |
+
# Ultralytics YOLO 🚀, AGPL-3.0 license
|
2 |
+
# README translation action to translate README.md to Chinese as README.zh-CN.md on any change to README.md
|
3 |
+
|
4 |
+
name: Translate README
|
5 |
+
|
6 |
+
on:
|
7 |
+
push:
|
8 |
+
branches:
|
9 |
+
- translate_readme # replace with 'main' to enable action
|
10 |
+
paths:
|
11 |
+
- README.md
|
12 |
+
|
13 |
+
jobs:
|
14 |
+
Translate:
|
15 |
+
runs-on: ubuntu-latest
|
16 |
+
steps:
|
17 |
+
- uses: actions/checkout@v3
|
18 |
+
- name: Setup Node.js
|
19 |
+
uses: actions/setup-node@v3
|
20 |
+
with:
|
21 |
+
node-version: 16
|
22 |
+
# ISO Language Codes: https://cloud.google.com/translate/docs/languages
|
23 |
+
- name: Adding README - Chinese Simplified
|
24 |
+
uses: dephraiim/translate-readme@main
|
25 |
+
with:
|
26 |
+
LANG: zh-CN
|
ultralytics/.github/workflows/ci.yaml
ADDED
@@ -0,0 +1,320 @@
|
|
|
|
|
|
|
|
|
|
|
|
|
|
|
|
|
|
|
|
|
|
|
|
|
|
|
|
|
|
|
|
|
|
|
|
|
|
|
|
|
|
|
|
|
|
|
|
|
|
|
|
|
|
|
|
|
|
|
|
|
|
|
|
|
|
|
|
|
|
|
|
|
|
|
|
|
|
|
|
|
|
|
|
|
|
|
|
|
|
|
|
|
|
|
|
|
|
|
|
|
|
|
|
|
|
|
|
|
|
|
|
|
|
|
|
|
|
|
|
|
|
|
|
|
|
|
|
|
|
|
|
|
|
|
|
|
|
|
|
|
|
|
|
|
|
|
|
|
|
|
|
|
|
|
|
|
|
|
|
|
|
|
|
|
|
|
|
|
|
|
|
|
|
|
|
|
|
|
|
|
|
|
|
|
|
|
|
|
|
|
|
|
|
|
|
|
|
|
|
|
|
|
|
|
|
|
|
|
|
|
|
|
|
|
|
|
|
|
|
|
|
|
|
|
|
|
|
|
|
|
|
|
|
|
|
|
|
|
|
|
|
|
|
|
|
|
|
|
|
|
|
|
|
|
|
|
|
|
|
|
|
|
|
|
|
|
|
|
|
|
|
|
|
|
|
|
|
|
|
|
|
|
|
|
|
|
|
|
|
|
|
|
|
|
|
|
|
|
|
|
|
|
|
|
|
|
|
|
|
|
|
|
|
|
|
|
|
|
|
|
|
|
|
|
|
|
|
|
|
|
|
|
|
|
|
|
|
|
|
|
|
|
|
|
|
|
|
|
|
|
|
|
|
|
|
|
|
|
|
|
|
|
|
|
|
|
|
|
|
|
|
|
|
|
|
|
|
|
|
|
|
|
|
|
|
|
|
|
|
|
|
|
|
|
|
|
|
|
|
|
|
|
|
|
|
|
|
|
|
|
|
|
|
|
|
|
|
|
|
|
|
|
|
|
|
|
|
|
|
|
|
|
|
|
|
|
|
|
|
|
|
|
|
|
|
|
|
|
|
|
|
|
|
|
|
|
|
|
|
|
|
|
|
|
|
|
|
|
|
|
|
|
|
|
|
|
|
|
|
|
|
|
|
|
|
|
|
|
|
|
|
|
|
|
|
|
|
|
|
|
|
|
|
|
|
|
|
|
|
|
|
|
|
|
|
|
|
|
|
|
|
|
|
|
|
|
|
|
|
|
|
|
|
|
|
|
|
|
|
|
|
|
|
|
|
|
|
|
|
|
|
|
|
|
|
|
|
|
|
|
|
|
|
|
|
|
|
|
|
|
|
|
|
|
|
|
|
|
|
|
|
|
|
|
|
|
|
|
|
|
|
|
|
|
|
|
|
|
|
|
|
|
|
|
|
|
|
|
|
|
|
|
|
|
|
|
|
|
|
|
|
|
|
|
|
|
|
|
|
|
|
|
|
|
|
|
|
|
|
|
|
|
1 |
+
# Ultralytics YOLO 🚀, AGPL-3.0 license
|
2 |
+
# YOLO Continuous Integration (CI) GitHub Actions tests
|
3 |
+
|
4 |
+
name: Ultralytics CI
|
5 |
+
|
6 |
+
on:
|
7 |
+
push:
|
8 |
+
branches: [main]
|
9 |
+
pull_request:
|
10 |
+
branches: [main]
|
11 |
+
schedule:
|
12 |
+
- cron: '0 0 * * *' # runs at 00:00 UTC every day
|
13 |
+
workflow_dispatch:
|
14 |
+
inputs:
|
15 |
+
hub:
|
16 |
+
description: 'Run HUB'
|
17 |
+
default: false
|
18 |
+
type: boolean
|
19 |
+
benchmarks:
|
20 |
+
description: 'Run Benchmarks'
|
21 |
+
default: false
|
22 |
+
type: boolean
|
23 |
+
tests:
|
24 |
+
description: 'Run Tests'
|
25 |
+
default: false
|
26 |
+
type: boolean
|
27 |
+
gpu:
|
28 |
+
description: 'Run GPU'
|
29 |
+
default: false
|
30 |
+
type: boolean
|
31 |
+
conda:
|
32 |
+
description: 'Run Conda'
|
33 |
+
default: false
|
34 |
+
type: boolean
|
35 |
+
|
36 |
+
jobs:
|
37 |
+
HUB:
|
38 |
+
if: github.repository == 'ultralytics/ultralytics' && (github.event_name == 'schedule' || github.event_name == 'push' || (github.event_name == 'workflow_dispatch' && github.event.inputs.hub == 'true'))
|
39 |
+
runs-on: ${{ matrix.os }}
|
40 |
+
strategy:
|
41 |
+
fail-fast: false
|
42 |
+
matrix:
|
43 |
+
os: [ubuntu-latest]
|
44 |
+
python-version: ['3.11']
|
45 |
+
steps:
|
46 |
+
- uses: actions/checkout@v4
|
47 |
+
- uses: actions/setup-python@v4
|
48 |
+
with:
|
49 |
+
python-version: ${{ matrix.python-version }}
|
50 |
+
cache: 'pip' # caching pip dependencies
|
51 |
+
- name: Install requirements
|
52 |
+
shell: bash # for Windows compatibility
|
53 |
+
run: |
|
54 |
+
python -m pip install --upgrade pip wheel
|
55 |
+
pip install -e . --extra-index-url https://download.pytorch.org/whl/cpu
|
56 |
+
- name: Check environment
|
57 |
+
run: |
|
58 |
+
yolo checks
|
59 |
+
echo "RUNNER_OS is ${{ runner.os }}"
|
60 |
+
echo "GITHUB_EVENT_NAME is ${{ github.event_name }}"
|
61 |
+
echo "GITHUB_WORKFLOW is ${{ github.workflow }}"
|
62 |
+
echo "GITHUB_ACTOR is ${{ github.actor }}"
|
63 |
+
echo "GITHUB_REPOSITORY is ${{ github.repository }}"
|
64 |
+
echo "GITHUB_REPOSITORY_OWNER is ${{ github.repository_owner }}"
|
65 |
+
python --version
|
66 |
+
pip --version
|
67 |
+
pip list
|
68 |
+
- name: Test HUB training
|
69 |
+
shell: python
|
70 |
+
env:
|
71 |
+
API_KEY: ${{ secrets.ULTRALYTICS_HUB_API_KEY }}
|
72 |
+
MODEL_ID: ${{ secrets.ULTRALYTICS_HUB_MODEL_ID }}
|
73 |
+
run: |
|
74 |
+
import os
|
75 |
+
from ultralytics import YOLO, hub
|
76 |
+
api_key, model_id = os.environ['API_KEY'], os.environ['MODEL_ID']
|
77 |
+
hub.login(api_key)
|
78 |
+
hub.reset_model(model_id)
|
79 |
+
model = YOLO('https://hub.ultralytics.com/models/' + model_id)
|
80 |
+
model.train()
|
81 |
+
- name: Test HUB inference API
|
82 |
+
shell: python
|
83 |
+
env:
|
84 |
+
API_KEY: ${{ secrets.ULTRALYTICS_HUB_API_KEY }}
|
85 |
+
MODEL_ID: ${{ secrets.ULTRALYTICS_HUB_MODEL_ID }}
|
86 |
+
run: |
|
87 |
+
import os
|
88 |
+
import requests
|
89 |
+
import json
|
90 |
+
api_key, model_id = os.environ['API_KEY'], os.environ['MODEL_ID']
|
91 |
+
url = f"https://api.ultralytics.com/v1/predict/{model_id}"
|
92 |
+
headers = {"x-api-key": api_key}
|
93 |
+
data = {"size": 320, "confidence": 0.25, "iou": 0.45}
|
94 |
+
with open("ultralytics/assets/zidane.jpg", "rb") as f:
|
95 |
+
response = requests.post(url, headers=headers, data=data, files={"image": f})
|
96 |
+
assert response.status_code == 200, f'Status code {response.status_code}, Reason {response.reason}'
|
97 |
+
print(json.dumps(response.json(), indent=2))
|
98 |
+
|
99 |
+
Benchmarks:
|
100 |
+
if: github.event_name != 'workflow_dispatch' || github.event.inputs.benchmarks == 'true'
|
101 |
+
runs-on: ${{ matrix.os }}
|
102 |
+
strategy:
|
103 |
+
fail-fast: false
|
104 |
+
matrix:
|
105 |
+
os: [ubuntu-latest]
|
106 |
+
python-version: ['3.10']
|
107 |
+
model: [yolov8n]
|
108 |
+
steps:
|
109 |
+
- uses: actions/checkout@v4
|
110 |
+
- uses: actions/setup-python@v4
|
111 |
+
with:
|
112 |
+
python-version: ${{ matrix.python-version }}
|
113 |
+
cache: 'pip' # caching pip dependencies
|
114 |
+
- name: Install requirements
|
115 |
+
shell: bash # for Windows compatibility
|
116 |
+
run: |
|
117 |
+
python -m pip install --upgrade pip wheel
|
118 |
+
pip install -e ".[export]" coverage --extra-index-url https://download.pytorch.org/whl/cpu
|
119 |
+
# Fix SavedModel issue "partially initialized module 'jax' has no attribute 'version' (most likely due to a circular import)" in https://github.com/google/jax/discussions/14036
|
120 |
+
# pip install -U 'jax!=0.4.15' 'jaxlib!=0.4.15'
|
121 |
+
# yolo settings tensorboard=False
|
122 |
+
yolo export format=tflite imgsz=32 || true
|
123 |
+
- name: Check environment
|
124 |
+
run: |
|
125 |
+
yolo checks
|
126 |
+
echo "RUNNER_OS is ${{ runner.os }}"
|
127 |
+
echo "GITHUB_EVENT_NAME is ${{ github.event_name }}"
|
128 |
+
echo "GITHUB_WORKFLOW is ${{ github.workflow }}"
|
129 |
+
echo "GITHUB_ACTOR is ${{ github.actor }}"
|
130 |
+
echo "GITHUB_REPOSITORY is ${{ github.repository }}"
|
131 |
+
echo "GITHUB_REPOSITORY_OWNER is ${{ github.repository_owner }}"
|
132 |
+
python --version
|
133 |
+
pip --version
|
134 |
+
pip list
|
135 |
+
# - name: Benchmark DetectionModel
|
136 |
+
# shell: bash
|
137 |
+
# run: coverage run -a --source=ultralytics -m ultralytics.cfg.__init__ benchmark model='path with spaces/${{ matrix.model }}.pt' imgsz=160 verbose=0.318
|
138 |
+
- name: Benchmark SegmentationModel
|
139 |
+
shell: bash
|
140 |
+
run: coverage run -a --source=ultralytics -m ultralytics.cfg.__init__ benchmark model='path with spaces/${{ matrix.model }}-seg.pt' imgsz=160 verbose=0.286
|
141 |
+
- name: Benchmark ClassificationModel
|
142 |
+
shell: bash
|
143 |
+
run: coverage run -a --source=ultralytics -m ultralytics.cfg.__init__ benchmark model='path with spaces/${{ matrix.model }}-cls.pt' imgsz=160 verbose=0.166
|
144 |
+
- name: Benchmark PoseModel
|
145 |
+
shell: bash
|
146 |
+
run: coverage run -a --source=ultralytics -m ultralytics.cfg.__init__ benchmark model='path with spaces/${{ matrix.model }}-pose.pt' imgsz=160 verbose=0.185
|
147 |
+
- name: Merge Coverage Reports
|
148 |
+
run: |
|
149 |
+
coverage xml -o coverage-benchmarks.xml
|
150 |
+
- name: Upload Coverage Reports to CodeCov
|
151 |
+
if: github.repository == 'ultralytics/ultralytics'
|
152 |
+
uses: codecov/codecov-action@v3
|
153 |
+
with:
|
154 |
+
flags: Benchmarks
|
155 |
+
env:
|
156 |
+
CODECOV_TOKEN: ${{ secrets.CODECOV_TOKEN }}
|
157 |
+
- name: Benchmark Summary
|
158 |
+
run: |
|
159 |
+
cat benchmarks.log
|
160 |
+
echo "$(cat benchmarks.log)" >> $GITHUB_STEP_SUMMARY
|
161 |
+
|
162 |
+
Tests:
|
163 |
+
if: github.event_name != 'workflow_dispatch' || github.event.inputs.tests == 'true'
|
164 |
+
timeout-minutes: 60
|
165 |
+
runs-on: ${{ matrix.os }}
|
166 |
+
strategy:
|
167 |
+
fail-fast: false
|
168 |
+
matrix:
|
169 |
+
os: [ubuntu-latest]
|
170 |
+
python-version: ['3.11']
|
171 |
+
torch: [latest]
|
172 |
+
include:
|
173 |
+
- os: ubuntu-latest
|
174 |
+
python-version: '3.8' # torch 1.8.0 requires python >=3.6, <=3.8
|
175 |
+
torch: '1.8.0' # min torch version CI https://pypi.org/project/torchvision/
|
176 |
+
steps:
|
177 |
+
- uses: actions/checkout@v4
|
178 |
+
- uses: actions/setup-python@v4
|
179 |
+
with:
|
180 |
+
python-version: ${{ matrix.python-version }}
|
181 |
+
cache: 'pip' # caching pip dependencies
|
182 |
+
- name: Install requirements
|
183 |
+
shell: bash # for Windows compatibility
|
184 |
+
run: | # CoreML must be installed before export due to protobuf error from AutoInstall
|
185 |
+
python -m pip install --upgrade pip wheel
|
186 |
+
torch=""
|
187 |
+
if [ "${{ matrix.torch }}" == "1.8.0" ]; then
|
188 |
+
torch="torch==1.8.0 torchvision==0.9.0"
|
189 |
+
fi
|
190 |
+
pip install -e . $torch pytest-cov "coremltools>=7.0" --extra-index-url https://download.pytorch.org/whl/cpu
|
191 |
+
- name: Check environment
|
192 |
+
run: |
|
193 |
+
yolo checks
|
194 |
+
echo "RUNNER_OS is ${{ runner.os }}"
|
195 |
+
echo "GITHUB_EVENT_NAME is ${{ github.event_name }}"
|
196 |
+
echo "GITHUB_WORKFLOW is ${{ github.workflow }}"
|
197 |
+
echo "GITHUB_ACTOR is ${{ github.actor }}"
|
198 |
+
echo "GITHUB_REPOSITORY is ${{ github.repository }}"
|
199 |
+
echo "GITHUB_REPOSITORY_OWNER is ${{ github.repository_owner }}"
|
200 |
+
python --version
|
201 |
+
pip --version
|
202 |
+
pip list
|
203 |
+
- name: Pytest tests
|
204 |
+
shell: bash # for Windows compatibility
|
205 |
+
run: |
|
206 |
+
slow=""
|
207 |
+
if [[ "${{ github.event_name }}" == "schedule" ]] || [[ "${{ github.event_name }}" == "workflow_dispatch" ]]; then
|
208 |
+
pip install mlflow pycocotools 'ray[tune]'
|
209 |
+
slow="--slow"
|
210 |
+
fi
|
211 |
+
pytest $slow --cov=ultralytics/ --cov-report xml tests/
|
212 |
+
- name: Upload Coverage Reports to CodeCov
|
213 |
+
if: github.repository == 'ultralytics/ultralytics' # && matrix.os == 'ubuntu-latest' && matrix.python-version == '3.11'
|
214 |
+
uses: codecov/codecov-action@v3
|
215 |
+
with:
|
216 |
+
flags: Tests
|
217 |
+
env:
|
218 |
+
CODECOV_TOKEN: ${{ secrets.CODECOV_TOKEN }}
|
219 |
+
|
220 |
+
GPU:
|
221 |
+
if: github.repository == 'ultralytics/ultralytics' && (github.event_name != 'workflow_dispatch' || github.event.inputs.gpu == 'true')
|
222 |
+
timeout-minutes: 60
|
223 |
+
runs-on: gpu-latest
|
224 |
+
steps:
|
225 |
+
- uses: actions/checkout@v4
|
226 |
+
- name: Install requirements
|
227 |
+
run: pip install -e .
|
228 |
+
- name: Check environment
|
229 |
+
run: |
|
230 |
+
yolo checks
|
231 |
+
echo "RUNNER_OS is ${{ runner.os }}"
|
232 |
+
echo "GITHUB_EVENT_NAME is ${{ github.event_name }}"
|
233 |
+
echo "GITHUB_WORKFLOW is ${{ github.workflow }}"
|
234 |
+
echo "GITHUB_ACTOR is ${{ github.actor }}"
|
235 |
+
echo "GITHUB_REPOSITORY is ${{ github.repository }}"
|
236 |
+
echo "GITHUB_REPOSITORY_OWNER is ${{ github.repository_owner }}"
|
237 |
+
python --version
|
238 |
+
pip --version
|
239 |
+
pip list
|
240 |
+
- name: Pytest tests
|
241 |
+
run: pytest --cov=ultralytics/ --cov-report xml tests/test_cuda.py
|
242 |
+
- name: Upload Coverage Reports to CodeCov
|
243 |
+
uses: codecov/codecov-action@v3
|
244 |
+
with:
|
245 |
+
flags: GPU
|
246 |
+
env:
|
247 |
+
CODECOV_TOKEN: ${{ secrets.CODECOV_TOKEN }}
|
248 |
+
|
249 |
+
Conda:
|
250 |
+
if: github.repository == 'ultralytics/ultralytics' && (github.event_name == 'schedule_disabled' || github.event.inputs.conda == 'true')
|
251 |
+
runs-on: ${{ matrix.os }}
|
252 |
+
strategy:
|
253 |
+
fail-fast: false
|
254 |
+
matrix:
|
255 |
+
os: [ubuntu-latest]
|
256 |
+
python-version: ['3.11']
|
257 |
+
defaults:
|
258 |
+
run:
|
259 |
+
shell: bash -el {0}
|
260 |
+
steps:
|
261 |
+
- uses: conda-incubator/setup-miniconda@v2
|
262 |
+
with:
|
263 |
+
python-version: ${{ matrix.python-version }}
|
264 |
+
mamba-version: "*"
|
265 |
+
channels: conda-forge,defaults
|
266 |
+
channel-priority: true
|
267 |
+
activate-environment: anaconda-client-env
|
268 |
+
- name: Install Libmamba
|
269 |
+
run: |
|
270 |
+
conda config --set solver libmamba
|
271 |
+
- name: Install Ultralytics package from conda-forge
|
272 |
+
run: |
|
273 |
+
conda install -c pytorch -c conda-forge pytorch torchvision ultralytics openvino
|
274 |
+
- name: Install pip packages
|
275 |
+
run: |
|
276 |
+
pip install pytest 'coremltools>=7.0'
|
277 |
+
- name: Check environment
|
278 |
+
run: |
|
279 |
+
echo "RUNNER_OS is ${{ runner.os }}"
|
280 |
+
echo "GITHUB_EVENT_NAME is ${{ github.event_name }}"
|
281 |
+
echo "GITHUB_WORKFLOW is ${{ github.workflow }}"
|
282 |
+
echo "GITHUB_ACTOR is ${{ github.actor }}"
|
283 |
+
echo "GITHUB_REPOSITORY is ${{ github.repository }}"
|
284 |
+
echo "GITHUB_REPOSITORY_OWNER is ${{ github.repository_owner }}"
|
285 |
+
python --version
|
286 |
+
conda list
|
287 |
+
- name: Test CLI
|
288 |
+
run: |
|
289 |
+
yolo predict model=yolov8n.pt imgsz=320
|
290 |
+
yolo train model=yolov8n.pt data=coco8.yaml epochs=1 imgsz=32
|
291 |
+
yolo val model=yolov8n.pt data=coco8.yaml imgsz=32
|
292 |
+
yolo export model=yolov8n.pt format=torchscript imgsz=160
|
293 |
+
- name: Test Python
|
294 |
+
run: |
|
295 |
+
python -c "
|
296 |
+
from ultralytics import YOLO
|
297 |
+
model = YOLO('yolov8n.pt')
|
298 |
+
results = model.train(data='coco8.yaml', epochs=3, imgsz=160)
|
299 |
+
results = model.val(imgsz=160)
|
300 |
+
results = model.predict(imgsz=160)
|
301 |
+
results = model.export(format='onnx', imgsz=160)
|
302 |
+
"
|
303 |
+
- name: PyTest
|
304 |
+
run: |
|
305 |
+
git clone https://github.com/ultralytics/ultralytics
|
306 |
+
pytest ultralytics/tests
|
307 |
+
|
308 |
+
Summary:
|
309 |
+
runs-on: ubuntu-latest
|
310 |
+
needs: [HUB, Benchmarks, Tests, GPU, Conda] # Add job names that you want to check for failure
|
311 |
+
if: always() # This ensures the job runs even if previous jobs fail
|
312 |
+
steps:
|
313 |
+
- name: Check for failure and notify
|
314 |
+
if: (needs.HUB.result == 'failure' || needs.Benchmarks.result == 'failure' || needs.Tests.result == 'failure' || needs.GPU.result == 'failure' || needs.Conda.result == 'failure') && github.repository == 'ultralytics/ultralytics' && (github.event_name == 'schedule' || github.event_name == 'push')
|
315 |
+
uses: slackapi/slack-github-action@v1.24.0
|
316 |
+
with:
|
317 |
+
payload: |
|
318 |
+
{"text": "<!channel> GitHub Actions error for ${{ github.workflow }} ❌\n\n\n*Repository:* https://github.com/${{ github.repository }}\n*Action:* https://github.com/${{ github.repository }}/actions/runs/${{ github.run_id }}\n*Author:* ${{ github.actor }}\n*Event:* ${{ github.event_name }}\n"}
|
319 |
+
env:
|
320 |
+
SLACK_WEBHOOK_URL: ${{ secrets.SLACK_WEBHOOK_URL_YOLO }}
|
ultralytics/.github/workflows/cla.yml
ADDED
@@ -0,0 +1,37 @@
|
|
|
|
|
|
|
|
|
|
|
|
|
|
|
|
|
|
|
|
|
|
|
|
|
|
|
|
|
|
|
|
|
|
|
|
|
|
|
|
|
|
|
|
|
|
|
|
|
|
|
|
|
|
|
|
|
|
|
|
|
|
|
|
|
|
|
|
|
|
|
|
|
|
|
|
|
1 |
+
# Ultralytics YOLO 🚀, AGPL-3.0 license
|
2 |
+
|
3 |
+
name: CLA Assistant
|
4 |
+
on:
|
5 |
+
issue_comment:
|
6 |
+
types:
|
7 |
+
- created
|
8 |
+
pull_request_target:
|
9 |
+
types:
|
10 |
+
- reopened
|
11 |
+
- opened
|
12 |
+
- synchronize
|
13 |
+
|
14 |
+
jobs:
|
15 |
+
CLA:
|
16 |
+
if: github.repository == 'ultralytics/ultralytics'
|
17 |
+
runs-on: ubuntu-latest
|
18 |
+
steps:
|
19 |
+
- name: CLA Assistant
|
20 |
+
if: (github.event.comment.body == 'recheck' || github.event.comment.body == 'I have read the CLA Document and I sign the CLA') || github.event_name == 'pull_request_target'
|
21 |
+
uses: contributor-assistant/github-action@v2.3.1
|
22 |
+
env:
|
23 |
+
GITHUB_TOKEN: ${{ secrets.GITHUB_TOKEN }}
|
24 |
+
# must be repository secret token
|
25 |
+
PERSONAL_ACCESS_TOKEN: ${{ secrets.PERSONAL_ACCESS_TOKEN }}
|
26 |
+
with:
|
27 |
+
path-to-signatures: 'signatures/version1/cla.json'
|
28 |
+
path-to-document: 'https://docs.ultralytics.com/help/CLA' # CLA document
|
29 |
+
# branch should not be protected
|
30 |
+
branch: 'main'
|
31 |
+
allowlist: dependabot[bot],github-actions,[pre-commit*,pre-commit*,bot*
|
32 |
+
|
33 |
+
remote-organization-name: ultralytics
|
34 |
+
remote-repository-name: cla
|
35 |
+
custom-pr-sign-comment: 'I have read the CLA Document and I sign the CLA'
|
36 |
+
custom-allsigned-prcomment: All Contributors have signed the CLA. ✅
|
37 |
+
#custom-notsigned-prcomment: 'pull request comment with Introductory message to ask new contributors to sign'
|
ultralytics/.github/workflows/codeql.yaml
ADDED
@@ -0,0 +1,42 @@
|
|
|
|
|
|
|
|
|
|
|
|
|
|
|
|
|
|
|
|
|
|
|
|
|
|
|
|
|
|
|
|
|
|
|
|
|
|
|
|
|
|
|
|
|
|
|
|
|
|
|
|
|
|
|
|
|
|
|
|
|
|
|
|
|
|
|
|
|
|
|
|
|
|
|
|
|
|
|
|
|
|
|
|
|
|
|
1 |
+
# Ultralytics YOLO 🚀, AGPL-3.0 license
|
2 |
+
|
3 |
+
name: "CodeQL"
|
4 |
+
|
5 |
+
on:
|
6 |
+
schedule:
|
7 |
+
- cron: '0 0 1 * *'
|
8 |
+
workflow_dispatch:
|
9 |
+
|
10 |
+
jobs:
|
11 |
+
analyze:
|
12 |
+
name: Analyze
|
13 |
+
runs-on: ${{ 'ubuntu-latest' }}
|
14 |
+
permissions:
|
15 |
+
actions: read
|
16 |
+
contents: read
|
17 |
+
security-events: write
|
18 |
+
|
19 |
+
strategy:
|
20 |
+
fail-fast: false
|
21 |
+
matrix:
|
22 |
+
language: [ 'python' ]
|
23 |
+
# CodeQL supports [ 'cpp', 'csharp', 'go', 'java', 'javascript', 'python', 'ruby' ]
|
24 |
+
|
25 |
+
steps:
|
26 |
+
- name: Checkout repository
|
27 |
+
uses: actions/checkout@v4
|
28 |
+
|
29 |
+
# Initializes the CodeQL tools for scanning.
|
30 |
+
- name: Initialize CodeQL
|
31 |
+
uses: github/codeql-action/init@v2
|
32 |
+
with:
|
33 |
+
languages: ${{ matrix.language }}
|
34 |
+
# If you wish to specify custom queries, you can do so here or in a config file.
|
35 |
+
# By default, queries listed here will override any specified in a config file.
|
36 |
+
# Prefix the list here with "+" to use these queries and those in the config file.
|
37 |
+
# queries: security-extended,security-and-quality
|
38 |
+
|
39 |
+
- name: Perform CodeQL Analysis
|
40 |
+
uses: github/codeql-action/analyze@v2
|
41 |
+
with:
|
42 |
+
category: "/language:${{matrix.language}}"
|
ultralytics/.github/workflows/docker.yaml
ADDED
@@ -0,0 +1,148 @@
|
|
|
|
|
|
|
|
|
|
|
|
|
|
|
|
|
|
|
|
|
|
|
|
|
|
|
|
|
|
|
|
|
|
|
|
|
|
|
|
|
|
|
|
|
|
|
|
|
|
|
|
|
|
|
|
|
|
|
|
|
|
|
|
|
|
|
|
|
|
|
|
|
|
|
|
|
|
|
|
|
|
|
|
|
|
|
|
|
|
|
|
|
|
|
|
|
|
|
|
|
|
|
|
|
|
|
|
|
|
|
|
|
|
|
|
|
|
|
|
|
|
|
|
|
|
|
|
|
|
|
|
|
|
|
|
|
|
|
|
|
|
|
|
|
|
|
|
|
|
|
|
|
|
|
|
|
|
|
|
|
|
|
|
|
|
|
|
|
|
|
|
|
|
|
|
|
|
|
|
|
|
|
|
|
|
|
|
|
|
|
|
|
|
|
|
|
|
|
|
|
|
|
|
|
|
|
|
|
|
|
|
|
|
|
|
|
|
|
|
|
|
|
|
|
|
|
|
|
|
|
|
|
|
|
|
|
|
|
|
|
|
|
|
|
|
|
|
|
|
|
|
|
|
|
|
|
|
|
|
|
|
|
|
|
|
|
|
|
|
|
|
|
|
|
|
|
|
|
|
|
|
|
|
|
|
|
|
|
|
|
|
|
|
|
|
|
|
|
1 |
+
# Ultralytics YOLO 🚀, AGPL-3.0 license
|
2 |
+
# Builds ultralytics/ultralytics:latest images on DockerHub https://hub.docker.com/r/ultralytics
|
3 |
+
|
4 |
+
name: Publish Docker Images
|
5 |
+
|
6 |
+
on:
|
7 |
+
push:
|
8 |
+
branches: [main]
|
9 |
+
workflow_dispatch:
|
10 |
+
inputs:
|
11 |
+
Dockerfile:
|
12 |
+
type: boolean
|
13 |
+
description: Use Dockerfile
|
14 |
+
default: true
|
15 |
+
Dockerfile-cpu:
|
16 |
+
type: boolean
|
17 |
+
description: Use Dockerfile-cpu
|
18 |
+
Dockerfile-arm64:
|
19 |
+
type: boolean
|
20 |
+
description: Use Dockerfile-arm64
|
21 |
+
Dockerfile-jetson:
|
22 |
+
type: boolean
|
23 |
+
description: Use Dockerfile-jetson
|
24 |
+
Dockerfile-python:
|
25 |
+
type: boolean
|
26 |
+
description: Use Dockerfile-python
|
27 |
+
Dockerfile-conda:
|
28 |
+
type: boolean
|
29 |
+
description: Use Dockerfile-conda
|
30 |
+
push:
|
31 |
+
type: boolean
|
32 |
+
description: Push images to Docker Hub
|
33 |
+
default: true
|
34 |
+
|
35 |
+
jobs:
|
36 |
+
docker:
|
37 |
+
if: github.repository == 'ultralytics/ultralytics'
|
38 |
+
name: Push
|
39 |
+
runs-on: ubuntu-latest
|
40 |
+
strategy:
|
41 |
+
fail-fast: false
|
42 |
+
max-parallel: 6
|
43 |
+
matrix:
|
44 |
+
include:
|
45 |
+
- dockerfile: "Dockerfile"
|
46 |
+
tags: "latest"
|
47 |
+
platforms: "linux/amd64"
|
48 |
+
- dockerfile: "Dockerfile-cpu"
|
49 |
+
tags: "latest-cpu"
|
50 |
+
platforms: "linux/amd64"
|
51 |
+
- dockerfile: "Dockerfile-arm64"
|
52 |
+
tags: "latest-arm64"
|
53 |
+
platforms: "linux/arm64"
|
54 |
+
- dockerfile: "Dockerfile-jetson"
|
55 |
+
tags: "latest-jetson"
|
56 |
+
platforms: "linux/arm64"
|
57 |
+
- dockerfile: "Dockerfile-python"
|
58 |
+
tags: "latest-python"
|
59 |
+
platforms: "linux/amd64"
|
60 |
+
# - dockerfile: "Dockerfile-conda"
|
61 |
+
# tags: "latest-conda"
|
62 |
+
# platforms: "linux/amd64"
|
63 |
+
steps:
|
64 |
+
- name: Checkout repo
|
65 |
+
uses: actions/checkout@v4
|
66 |
+
with:
|
67 |
+
fetch-depth: 0 # copy full .git directory to access full git history in Docker images
|
68 |
+
|
69 |
+
- name: Set up QEMU
|
70 |
+
uses: docker/setup-qemu-action@v3
|
71 |
+
|
72 |
+
- name: Set up Docker Buildx
|
73 |
+
uses: docker/setup-buildx-action@v3
|
74 |
+
|
75 |
+
- name: Login to Docker Hub
|
76 |
+
uses: docker/login-action@v3
|
77 |
+
with:
|
78 |
+
username: ${{ secrets.DOCKERHUB_USERNAME }}
|
79 |
+
password: ${{ secrets.DOCKERHUB_TOKEN }}
|
80 |
+
|
81 |
+
- name: Retrieve Ultralytics version
|
82 |
+
id: get_version
|
83 |
+
run: |
|
84 |
+
VERSION=$(grep "^__version__ =" ultralytics/__init__.py | awk -F"'" '{print $2}')
|
85 |
+
echo "Retrieved Ultralytics version: $VERSION"
|
86 |
+
echo "version=$VERSION" >> $GITHUB_OUTPUT
|
87 |
+
|
88 |
+
VERSION_TAG=$(echo "${{ matrix.tags }}" | sed "s/latest/${VERSION}/")
|
89 |
+
echo "Intended version tag: $VERSION_TAG"
|
90 |
+
echo "version_tag=$VERSION_TAG" >> $GITHUB_OUTPUT
|
91 |
+
|
92 |
+
- name: Check if version tag exists on DockerHub
|
93 |
+
id: check_tag
|
94 |
+
run: |
|
95 |
+
RESPONSE=$(curl -s https://hub.docker.com/v2/repositories/ultralytics/ultralytics/tags/$VERSION_TAG)
|
96 |
+
MESSAGE=$(echo $RESPONSE | jq -r '.message')
|
97 |
+
if [[ "$MESSAGE" == "null" ]]; then
|
98 |
+
echo "Tag $VERSION_TAG already exists on DockerHub."
|
99 |
+
echo "exists=true" >> $GITHUB_OUTPUT
|
100 |
+
elif [[ "$MESSAGE" == *"404"* ]]; then
|
101 |
+
echo "Tag $VERSION_TAG does not exist on DockerHub."
|
102 |
+
echo "exists=false" >> $GITHUB_OUTPUT
|
103 |
+
else
|
104 |
+
echo "Unexpected response from DockerHub. Please check manually."
|
105 |
+
echo "exists=false" >> $GITHUB_OUTPUT
|
106 |
+
fi
|
107 |
+
env:
|
108 |
+
VERSION_TAG: ${{ steps.get_version.outputs.version_tag }}
|
109 |
+
|
110 |
+
- name: Build Image
|
111 |
+
if: github.event_name == 'push' || github.event.inputs[matrix.dockerfile] == 'true'
|
112 |
+
run: |
|
113 |
+
docker build --platform ${{ matrix.platforms }} -f docker/${{ matrix.dockerfile }} \
|
114 |
+
-t ultralytics/ultralytics:${{ matrix.tags }} \
|
115 |
+
-t ultralytics/ultralytics:${{ steps.get_version.outputs.version_tag }} .
|
116 |
+
|
117 |
+
- name: Run Tests
|
118 |
+
if: (github.event_name == 'push' || github.event.inputs[matrix.dockerfile] == 'true') && matrix.platforms == 'linux/amd64' && matrix.dockerfile != 'Dockerfile-conda' # arm64 images not supported on GitHub CI runners
|
119 |
+
run: docker run ultralytics/ultralytics:${{ matrix.tags }} /bin/bash -c "pip install pytest && pytest tests"
|
120 |
+
|
121 |
+
- name: Run Benchmarks
|
122 |
+
# WARNING: Dockerfile (GPU) error on TF.js export 'module 'numpy' has no attribute 'object'.
|
123 |
+
if: (github.event_name == 'push' || github.event.inputs[matrix.dockerfile] == 'true') && matrix.platforms == 'linux/amd64' && matrix.dockerfile != 'Dockerfile' && matrix.dockerfile != 'Dockerfile-conda' # arm64 images not supported on GitHub CI runners
|
124 |
+
run: docker run ultralytics/ultralytics:${{ matrix.tags }} yolo benchmark model=yolov8n.pt imgsz=160 verbose=0.318
|
125 |
+
|
126 |
+
- name: Push Docker Image with Ultralytics version tag
|
127 |
+
if: (github.event_name == 'push' || (github.event.inputs[matrix.dockerfile] == 'true' && github.event.inputs.push == 'true')) && steps.check_tag.outputs.exists == 'false' && matrix.dockerfile != 'Dockerfile-conda'
|
128 |
+
run: |
|
129 |
+
docker push ultralytics/ultralytics:${{ steps.get_version.outputs.version_tag }}
|
130 |
+
|
131 |
+
- name: Push Docker Image with latest tag
|
132 |
+
if: github.event_name == 'push' || (github.event.inputs[matrix.dockerfile] == 'true' && github.event.inputs.push == 'true')
|
133 |
+
run: |
|
134 |
+
docker push ultralytics/ultralytics:${{ matrix.tags }}
|
135 |
+
if [[ "${{ matrix.tags }}" == "latest" ]]; then
|
136 |
+
t=ultralytics/ultralytics:latest-runner
|
137 |
+
docker build -f docker/Dockerfile-runner -t $t .
|
138 |
+
docker push $t
|
139 |
+
fi
|
140 |
+
|
141 |
+
- name: Notify on failure
|
142 |
+
if: github.event_name == 'push' && failure() # do not notify on cancelled() as cancelling is performed by hand
|
143 |
+
uses: slackapi/slack-github-action@v1.24.0
|
144 |
+
with:
|
145 |
+
payload: |
|
146 |
+
{"text": "<!channel> GitHub Actions error for ${{ github.workflow }} ❌\n\n\n*Repository:* https://github.com/${{ github.repository }}\n*Action:* https://github.com/${{ github.repository }}/actions/runs/${{ github.run_id }}\n*Author:* ${{ github.actor }}\n*Event:* ${{ github.event_name }}\n"}
|
147 |
+
env:
|
148 |
+
SLACK_WEBHOOK_URL: ${{ secrets.SLACK_WEBHOOK_URL_YOLO }}
|
ultralytics/.github/workflows/greetings.yml
ADDED
@@ -0,0 +1,58 @@
|
|
|
|
|
|
|
|
|
|
|
|
|
|
|
|
|
|
|
|
|
|
|
|
|
|
|
|
|
|
|
|
|
|
|
|
|
|
|
|
|
|
|
|
|
|
|
|
|
|
|
|
|
|
|
|
|
|
|
|
|
|
|
|
|
|
|
|
|
|
|
|
|
|
|
|
|
|
|
|
|
|
|
|
|
|
|
|
|
|
|
|
|
|
|
|
|
|
|
|
|
|
|
|
|
|
|
|
|
|
|
|
|
|
|
|
|
|
|
1 |
+
# Ultralytics YOLO 🚀, AGPL-3.0 license
|
2 |
+
|
3 |
+
name: Greetings
|
4 |
+
|
5 |
+
on:
|
6 |
+
pull_request_target:
|
7 |
+
types: [opened]
|
8 |
+
issues:
|
9 |
+
types: [opened]
|
10 |
+
|
11 |
+
jobs:
|
12 |
+
greeting:
|
13 |
+
runs-on: ubuntu-latest
|
14 |
+
steps:
|
15 |
+
- uses: actions/first-interaction@v1
|
16 |
+
with:
|
17 |
+
repo-token: ${{ secrets.GITHUB_TOKEN }}
|
18 |
+
pr-message: |
|
19 |
+
👋 Hello @${{ github.actor }}, thank you for submitting a YOLOv8 🚀 PR! To allow your work to be integrated as seamlessly as possible, we advise you to:
|
20 |
+
|
21 |
+
- ✅ Verify your PR is **up-to-date** with `ultralytics/ultralytics` `main` branch. If your PR is behind you can update your code by clicking the 'Update branch' button or by running `git pull` and `git merge main` locally.
|
22 |
+
- ✅ Verify all YOLOv8 Continuous Integration (CI) **checks are passing**.
|
23 |
+
- ✅ Update YOLOv8 [Docs](https://docs.ultralytics.com) for any new or updated features.
|
24 |
+
- ✅ Reduce changes to the absolute **minimum** required for your bug fix or feature addition. _"It is not daily increase but daily decrease, hack away the unessential. The closer to the source, the less wastage there is."_ — Bruce Lee
|
25 |
+
|
26 |
+
See our [Contributing Guide](https://docs.ultralytics.com/help/contributing) for details and let us know if you have any questions!
|
27 |
+
|
28 |
+
issue-message: |
|
29 |
+
👋 Hello @${{ github.actor }}, thank you for your interest in YOLOv8 🚀! We recommend a visit to the [YOLOv8 Docs](https://docs.ultralytics.com) for new users where you can find many [Python](https://docs.ultralytics.com/usage/python/) and [CLI](https://docs.ultralytics.com/usage/cli/) usage examples and where many of the most common questions may already be answered.
|
30 |
+
|
31 |
+
If this is a 🐛 Bug Report, please provide a [minimum reproducible example](https://docs.ultralytics.com/help/minimum_reproducible_example/) to help us debug it.
|
32 |
+
|
33 |
+
If this is a custom training ❓ Question, please provide as much information as possible, including dataset image examples and training logs, and verify you are following our [Tips for Best Training Results](https://docs.ultralytics.com/yolov5/tutorials/tips_for_best_training_results/).
|
34 |
+
|
35 |
+
Join the vibrant [Ultralytics Discord](https://ultralytics.com/discord) 🎧 community for real-time conversations and collaborations. This platform offers a perfect space to inquire, showcase your work, and connect with fellow Ultralytics users.
|
36 |
+
|
37 |
+
## Install
|
38 |
+
|
39 |
+
Pip install the `ultralytics` package including all [requirements](https://github.com/ultralytics/ultralytics/blob/main/requirements.txt) in a [**Python>=3.8**](https://www.python.org/) environment with [**PyTorch>=1.8**](https://pytorch.org/get-started/locally/).
|
40 |
+
|
41 |
+
```bash
|
42 |
+
pip install ultralytics
|
43 |
+
```
|
44 |
+
|
45 |
+
## Environments
|
46 |
+
|
47 |
+
YOLOv8 may be run in any of the following up-to-date verified environments (with all dependencies including [CUDA](https://developer.nvidia.com/cuda)/[CUDNN](https://developer.nvidia.com/cudnn), [Python](https://www.python.org/) and [PyTorch](https://pytorch.org/) preinstalled):
|
48 |
+
|
49 |
+
- **Notebooks** with free GPU: <a href="https://console.paperspace.com/github/ultralytics/ultralytics"><img src="https://assets.paperspace.io/img/gradient-badge.svg" alt="Run on Gradient"/></a> <a href="https://colab.research.google.com/github/ultralytics/ultralytics/blob/main/examples/tutorial.ipynb"><img src="https://colab.research.google.com/assets/colab-badge.svg" alt="Open In Colab"></a> <a href="https://www.kaggle.com/ultralytics/yolov8"><img src="https://kaggle.com/static/images/open-in-kaggle.svg" alt="Open In Kaggle"></a>
|
50 |
+
- **Google Cloud** Deep Learning VM. See [GCP Quickstart Guide](https://docs.ultralytics.com/yolov5/environments/google_cloud_quickstart_tutorial/)
|
51 |
+
- **Amazon** Deep Learning AMI. See [AWS Quickstart Guide](https://docs.ultralytics.com/yolov5/environments/aws_quickstart_tutorial/)
|
52 |
+
- **Docker Image**. See [Docker Quickstart Guide](https://docs.ultralytics.com/yolov5/environments/docker_image_quickstart_tutorial/) <a href="https://hub.docker.com/r/ultralytics/ultralytics"><img src="https://img.shields.io/docker/pulls/ultralytics/ultralytics?logo=docker" alt="Docker Pulls"></a>
|
53 |
+
|
54 |
+
## Status
|
55 |
+
|
56 |
+
<a href="https://github.com/ultralytics/ultralytics/actions/workflows/ci.yaml?query=event%3Aschedule"><img src="https://github.com/ultralytics/ultralytics/actions/workflows/ci.yaml/badge.svg" alt="Ultralytics CI"></a>
|
57 |
+
|
58 |
+
If this badge is green, all [Ultralytics CI](https://github.com/ultralytics/ultralytics/actions/workflows/ci.yaml?query=event%3Aschedule) tests are currently passing. CI tests verify correct operation of all YOLOv8 [Modes](https://docs.ultralytics.com/modes/) and [Tasks](https://docs.ultralytics.com/tasks/) on macOS, Windows, and Ubuntu every 24 hours and on every commit.
|
ultralytics/.github/workflows/links.yml
ADDED
@@ -0,0 +1,45 @@
|
|
|
|
|
|
|
|
|
|
|
|
|
|
|
|
|
|
|
|
|
|
|
|
|
|
|
|
|
|
|
|
|
|
|
|
|
|
|
|
|
|
|
|
|
|
|
|
|
|
|
|
|
|
|
|
|
|
|
|
|
|
|
|
|
|
|
|
|
|
|
|
|
|
|
|
|
|
|
|
|
|
|
|
|
|
|
|
|
|
|
|
|
1 |
+
# Ultralytics YOLO 🚀, AGPL-3.0 license
|
2 |
+
# Continuous Integration (CI) GitHub Actions tests broken link checker using https://github.com/lycheeverse/lychee
|
3 |
+
# Ignores the following status codes to reduce false positives:
|
4 |
+
# - 403(OpenVINO, 'forbidden')
|
5 |
+
# - 429(Instagram, 'too many requests')
|
6 |
+
# - 500(Zenodo, 'cached')
|
7 |
+
# - 502(Zenodo, 'bad gateway')
|
8 |
+
# - 999(LinkedIn, 'unknown status code')
|
9 |
+
|
10 |
+
name: Check Broken links
|
11 |
+
|
12 |
+
on:
|
13 |
+
workflow_dispatch:
|
14 |
+
schedule:
|
15 |
+
- cron: '0 0 * * *' # runs at 00:00 UTC every day
|
16 |
+
|
17 |
+
jobs:
|
18 |
+
Links:
|
19 |
+
runs-on: ubuntu-latest
|
20 |
+
steps:
|
21 |
+
- uses: actions/checkout@v4
|
22 |
+
|
23 |
+
- name: Download and install lychee
|
24 |
+
run: |
|
25 |
+
LYCHEE_URL=$(curl -s https://api.github.com/repos/lycheeverse/lychee/releases/latest | grep "browser_download_url" | grep "x86_64-unknown-linux-gnu.tar.gz" | cut -d '"' -f 4)
|
26 |
+
curl -L $LYCHEE_URL -o lychee.tar.gz
|
27 |
+
tar xzf lychee.tar.gz
|
28 |
+
sudo mv lychee /usr/local/bin
|
29 |
+
|
30 |
+
- name: Test Markdown and HTML links with retry
|
31 |
+
uses: nick-invision/retry@v2
|
32 |
+
with:
|
33 |
+
timeout_minutes: 5
|
34 |
+
retry_wait_seconds: 60
|
35 |
+
max_attempts: 3
|
36 |
+
command: lychee --accept 403,429,500,502,999 --exclude-loopback --exclude 'https?://(www\.)?(linkedin\.com|twitter\.com|instagram\.com|kaggle\.com|fonts\.gstatic\.com|url\.com)' --exclude-path '**/ci.yaml' --exclude-mail --github-token ${{ secrets.GITHUB_TOKEN }} './**/*.md' './**/*.html'
|
37 |
+
|
38 |
+
- name: Test Markdown, HTML, YAML, Python and Notebook links with retry
|
39 |
+
if: github.event_name == 'workflow_dispatch'
|
40 |
+
uses: nick-invision/retry@v2
|
41 |
+
with:
|
42 |
+
timeout_minutes: 5
|
43 |
+
retry_wait_seconds: 60
|
44 |
+
max_attempts: 3
|
45 |
+
command: lychee --accept 429,999 --exclude-loopback --exclude 'https?://(www\.)?(linkedin\.com|twitter\.com|instagram\.com|kaggle\.com|fonts\.gstatic\.com|url\.com)' --exclude-path '**/ci.yaml' --exclude-mail --github-token ${{ secrets.GITHUB_TOKEN }} './**/*.md' './**/*.html' './**/*.yml' './**/*.yaml' './**/*.py' './**/*.ipynb'
|
ultralytics/.github/workflows/publish.yml
ADDED
@@ -0,0 +1,109 @@
|
|
|
|
|
|
|
|
|
|
|
|
|
|
|
|
|
|
|
|
|
|
|
|
|
|
|
|
|
|
|
|
|
|
|
|
|
|
|
|
|
|
|
|
|
|
|
|
|
|
|
|
|
|
|
|
|
|
|
|
|
|
|
|
|
|
|
|
|
|
|
|
|
|
|
|
|
|
|
|
|
|
|
|
|
|
|
|
|
|
|
|
|
|
|
|
|
|
|
|
|
|
|
|
|
|
|
|
|
|
|
|
|
|
|
|
|
|
|
|
|
|
|
|
|
|
|
|
|
|
|
|
|
|
|
|
|
|
|
|
|
|
|
|
|
|
|
|
|
|
|
|
|
|
|
|
|
|
|
|
|
|
|
|
|
|
|
|
|
|
|
|
|
|
|
|
|
|
|
|
|
|
|
|
|
|
|
|
|
|
|
|
|
|
|
|
|
|
|
|
|
|
|
|
|
|
|
|
|
|
|
|
|
|
|
|
|
|
|
|
|
1 |
+
# Ultralytics YOLO 🚀, AGPL-3.0 license
|
2 |
+
# Publish pip package to PyPI https://pypi.org/project/ultralytics/ and Docs to https://docs.ultralytics.com
|
3 |
+
|
4 |
+
name: Publish to PyPI and Deploy Docs
|
5 |
+
|
6 |
+
on:
|
7 |
+
push:
|
8 |
+
branches: [main]
|
9 |
+
workflow_dispatch:
|
10 |
+
inputs:
|
11 |
+
pypi:
|
12 |
+
type: boolean
|
13 |
+
description: Publish to PyPI
|
14 |
+
docs:
|
15 |
+
type: boolean
|
16 |
+
description: Deploy Docs
|
17 |
+
|
18 |
+
jobs:
|
19 |
+
publish:
|
20 |
+
if: github.repository == 'ultralytics/ultralytics' && github.actor == 'glenn-jocher'
|
21 |
+
name: Publish
|
22 |
+
runs-on: ubuntu-latest
|
23 |
+
steps:
|
24 |
+
- name: Checkout code
|
25 |
+
uses: actions/checkout@v4
|
26 |
+
with:
|
27 |
+
fetch-depth: "0" # pulls all commits (needed correct last updated dates in Docs)
|
28 |
+
- name: Set up Python environment
|
29 |
+
uses: actions/setup-python@v4
|
30 |
+
with:
|
31 |
+
python-version: '3.10'
|
32 |
+
cache: 'pip' # caching pip dependencies
|
33 |
+
- name: Install dependencies
|
34 |
+
run: |
|
35 |
+
python -m pip install --upgrade pip wheel build twine
|
36 |
+
pip install -e ".[dev]" --extra-index-url https://download.pytorch.org/whl/cpu
|
37 |
+
- name: Check PyPI version
|
38 |
+
shell: python
|
39 |
+
run: |
|
40 |
+
import os
|
41 |
+
import ultralytics
|
42 |
+
from ultralytics.utils.checks import check_latest_pypi_version
|
43 |
+
|
44 |
+
v_local = tuple(map(int, ultralytics.__version__.split('.')))
|
45 |
+
v_pypi = tuple(map(int, check_latest_pypi_version().split('.')))
|
46 |
+
print(f'Local version is {v_local}')
|
47 |
+
print(f'PyPI version is {v_pypi}')
|
48 |
+
d = [a - b for a, b in zip(v_local, v_pypi)] # diff
|
49 |
+
increment = (d[0] == d[1] == 0) and (0 < d[2] < 3) # only publish if patch version increments by 1 or 2
|
50 |
+
os.system(f'echo "increment={increment}" >> $GITHUB_OUTPUT')
|
51 |
+
os.system(f'echo "version={ultralytics.__version__}" >> $GITHUB_OUTPUT')
|
52 |
+
if increment:
|
53 |
+
print('Local version is higher than PyPI version. Publishing new version to PyPI ✅.')
|
54 |
+
id: check_pypi
|
55 |
+
- name: Publish to PyPI
|
56 |
+
continue-on-error: true
|
57 |
+
if: (github.event_name == 'push' || github.event.inputs.pypi == 'true') && steps.check_pypi.outputs.increment == 'True'
|
58 |
+
env:
|
59 |
+
PYPI_TOKEN: ${{ secrets.PYPI_TOKEN }}
|
60 |
+
run: |
|
61 |
+
python -m build
|
62 |
+
python -m twine upload dist/* -u __token__ -p $PYPI_TOKEN
|
63 |
+
- name: Deploy Docs
|
64 |
+
continue-on-error: true
|
65 |
+
if: (github.event_name == 'push' || github.event.inputs.docs == 'true') && github.repository == 'ultralytics/ultralytics' && github.actor == 'glenn-jocher'
|
66 |
+
env:
|
67 |
+
PERSONAL_ACCESS_TOKEN: ${{ secrets.PERSONAL_ACCESS_TOKEN }}
|
68 |
+
run: |
|
69 |
+
mkdocs build
|
70 |
+
git config --global user.name "Glenn Jocher"
|
71 |
+
git config --global user.email "glenn.jocher@ultralytics.com"
|
72 |
+
git clone https://github.com/ultralytics/docs.git docs-repo
|
73 |
+
cd docs-repo
|
74 |
+
git checkout gh-pages || git checkout -b gh-pages
|
75 |
+
rm -rf *
|
76 |
+
cp -R ../site/* .
|
77 |
+
git add .
|
78 |
+
git commit -m "Update Docs for 'ultralytics ${{ steps.check_pypi.outputs.version }}'"
|
79 |
+
git push https://${{ secrets.PERSONAL_ACCESS_TOKEN }}@github.com/ultralytics/docs.git gh-pages
|
80 |
+
- name: Extract PR Details
|
81 |
+
run: |
|
82 |
+
if [ "${{ github.event_name }}" = "pull_request" ]; then
|
83 |
+
PR_JSON=$(curl -s -H "Authorization: token ${{ secrets.GITHUB_TOKEN }}" https://api.github.com/repos/${{ github.repository }}/pulls/${{ github.event.pull_request.number }})
|
84 |
+
PR_NUMBER=${{ github.event.pull_request.number }}
|
85 |
+
PR_TITLE=$(echo $PR_JSON | jq -r '.title')
|
86 |
+
else
|
87 |
+
COMMIT_SHA=${{ github.event.after }}
|
88 |
+
PR_JSON=$(curl -s -H "Authorization: token ${{ secrets.GITHUB_TOKEN }}" "https://api.github.com/search/issues?q=repo:${{ github.repository }}+is:pr+is:merged+sha:$COMMIT_SHA")
|
89 |
+
PR_NUMBER=$(echo $PR_JSON | jq -r '.items[0].number')
|
90 |
+
PR_TITLE=$(echo $PR_JSON | jq -r '.items[0].title')
|
91 |
+
fi
|
92 |
+
echo "PR_NUMBER=$PR_NUMBER" >> $GITHUB_ENV
|
93 |
+
echo "PR_TITLE=$PR_TITLE" >> $GITHUB_ENV
|
94 |
+
- name: Notify on Slack (Success)
|
95 |
+
if: success() && github.event_name == 'push' && steps.check_pypi.outputs.increment == 'True'
|
96 |
+
uses: slackapi/slack-github-action@v1.24.0
|
97 |
+
with:
|
98 |
+
payload: |
|
99 |
+
{"text": "<!channel> GitHub Actions success for ${{ github.workflow }} ✅\n\n\n*Repository:* https://github.com/${{ github.repository }}\n*Action:* https://github.com/${{ github.repository }}/actions/runs/${{ github.run_id }}\n*Author:* ${{ github.actor }}\n*Event:* NEW 'ultralytics ${{ steps.check_pypi.outputs.version }}' pip package published 😃\n*Job Status:* ${{ job.status }}\n*Pull Request:* <https://github.com/${{ github.repository }}/pull/${{ env.PR_NUMBER }}> ${{ env.PR_TITLE }}\n"}
|
100 |
+
env:
|
101 |
+
SLACK_WEBHOOK_URL: ${{ secrets.SLACK_WEBHOOK_URL_YOLO }}
|
102 |
+
- name: Notify on Slack (Failure)
|
103 |
+
if: failure()
|
104 |
+
uses: slackapi/slack-github-action@v1.24.0
|
105 |
+
with:
|
106 |
+
payload: |
|
107 |
+
{"text": "<!channel> GitHub Actions error for ${{ github.workflow }} ❌\n\n\n*Repository:* https://github.com/${{ github.repository }}\n*Action:* https://github.com/${{ github.repository }}/actions/runs/${{ github.run_id }}\n*Author:* ${{ github.actor }}\n*Event:* ${{ github.event_name }}\n*Job Status:* ${{ job.status }}\n*Pull Request:* <https://github.com/${{ github.repository }}/pull/${{ env.PR_NUMBER }}> ${{ env.PR_TITLE }}\n"}
|
108 |
+
env:
|
109 |
+
SLACK_WEBHOOK_URL: ${{ secrets.SLACK_WEBHOOK_URL_YOLO }}
|
ultralytics/.github/workflows/stale.yml
ADDED
@@ -0,0 +1,47 @@
|
|
|
|
|
|
|
|
|
|
|
|
|
|
|
|
|
|
|
|
|
|
|
|
|
|
|
|
|
|
|
|
|
|
|
|
|
|
|
|
|
|
|
|
|
|
|
|
|
|
|
|
|
|
|
|
|
|
|
|
|
|
|
|
|
|
|
|
|
|
|
|
|
|
|
|
|
|
|
|
|
|
|
|
|
|
|
|
|
|
|
|
|
|
|
|
|
1 |
+
# Ultralytics YOLO 🚀, AGPL-3.0 license
|
2 |
+
|
3 |
+
name: Close stale issues
|
4 |
+
on:
|
5 |
+
schedule:
|
6 |
+
- cron: '0 0 * * *' # Runs at 00:00 UTC every day
|
7 |
+
|
8 |
+
jobs:
|
9 |
+
stale:
|
10 |
+
runs-on: ubuntu-latest
|
11 |
+
steps:
|
12 |
+
- uses: actions/stale@v8
|
13 |
+
with:
|
14 |
+
repo-token: ${{ secrets.GITHUB_TOKEN }}
|
15 |
+
|
16 |
+
stale-issue-message: |
|
17 |
+
👋 Hello there! We wanted to give you a friendly reminder that this issue has not had any recent activity and may be closed soon, but don't worry - you can always reopen it if needed. If you still have any questions or concerns, please feel free to let us know how we can help.
|
18 |
+
|
19 |
+
For additional resources and information, please see the links below:
|
20 |
+
|
21 |
+
- **Docs**: https://docs.ultralytics.com
|
22 |
+
- **HUB**: https://hub.ultralytics.com
|
23 |
+
- **Community**: https://community.ultralytics.com
|
24 |
+
|
25 |
+
Feel free to inform us of any other **issues** you discover or **feature requests** that come to mind in the future. Pull Requests (PRs) are also always welcomed!
|
26 |
+
|
27 |
+
Thank you for your contributions to YOLO 🚀 and Vision AI ⭐
|
28 |
+
|
29 |
+
stale-pr-message: |
|
30 |
+
👋 Hello there! We wanted to let you know that we've decided to close this pull request due to inactivity. We appreciate the effort you put into contributing to our project, but unfortunately, not all contributions are suitable or aligned with our product roadmap.
|
31 |
+
|
32 |
+
We hope you understand our decision, and please don't let it discourage you from contributing to open source projects in the future. We value all of our community members and their contributions, and we encourage you to keep exploring new projects and ways to get involved.
|
33 |
+
|
34 |
+
For additional resources and information, please see the links below:
|
35 |
+
|
36 |
+
- **Docs**: https://docs.ultralytics.com
|
37 |
+
- **HUB**: https://hub.ultralytics.com
|
38 |
+
- **Community**: https://community.ultralytics.com
|
39 |
+
|
40 |
+
Thank you for your contributions to YOLO 🚀 and Vision AI ⭐
|
41 |
+
|
42 |
+
days-before-issue-stale: 30
|
43 |
+
days-before-issue-close: 10
|
44 |
+
days-before-pr-stale: 90
|
45 |
+
days-before-pr-close: 30
|
46 |
+
exempt-issue-labels: 'documentation,tutorial,TODO'
|
47 |
+
operations-per-run: 300 # The maximum number of operations per run, used to control rate limiting.
|
ultralytics/.gitignore
ADDED
@@ -0,0 +1,165 @@
|
|
|
|
|
|
|
|
|
|
|
|
|
|
|
|
|
|
|
|
|
|
|
|
|
|
|
|
|
|
|
|
|
|
|
|
|
|
|
|
|
|
|
|
|
|
|
|
|
|
|
|
|
|
|
|
|
|
|
|
|
|
|
|
|
|
|
|
|
|
|
|
|
|
|
|
|
|
|
|
|
|
|
|
|
|
|
|
|
|
|
|
|
|
|
|
|
|
|
|
|
|
|
|
|
|
|
|
|
|
|
|
|
|
|
|
|
|
|
|
|
|
|
|
|
|
|
|
|
|
|
|
|
|
|
|
|
|
|
|
|
|
|
|
|
|
|
|
|
|
|
|
|
|
|
|
|
|
|
|
|
|
|
|
|
|
|
|
|
|
|
|
|
|
|
|
|
|
|
|
|
|
|
|
|
|
|
|
|
|
|
|
|
|
|
|
|
|
|
|
|
|
|
|
|
|
|
|
|
|
|
|
|
|
|
|
|
|
|
|
|
|
|
|
|
|
|
|
|
|
|
|
|
|
|
|
|
|
|
|
|
|
|
|
|
|
|
|
|
|
|
|
|
|
|
|
|
|
|
|
|
|
|
|
|
|
|
|
|
|
|
|
|
|
|
|
|
|
|
|
|
|
|
|
|
|
|
|
|
|
|
|
|
|
|
|
|
|
|
|
|
|
|
|
|
|
|
|
|
|
|
|
|
|
|
|
|
|
|
|
|
|
|
|
|
|
|
|
|
|
|
|
|
1 |
+
# Byte-compiled / optimized / DLL files
|
2 |
+
__pycache__/
|
3 |
+
*.py[cod]
|
4 |
+
*$py.class
|
5 |
+
|
6 |
+
# C extensions
|
7 |
+
*.so
|
8 |
+
|
9 |
+
# Distribution / packaging
|
10 |
+
.Python
|
11 |
+
build/
|
12 |
+
develop-eggs/
|
13 |
+
dist/
|
14 |
+
downloads/
|
15 |
+
eggs/
|
16 |
+
.eggs/
|
17 |
+
lib/
|
18 |
+
lib64/
|
19 |
+
parts/
|
20 |
+
sdist/
|
21 |
+
var/
|
22 |
+
wheels/
|
23 |
+
pip-wheel-metadata/
|
24 |
+
share/python-wheels/
|
25 |
+
*.egg-info/
|
26 |
+
.installed.cfg
|
27 |
+
*.egg
|
28 |
+
MANIFEST
|
29 |
+
|
30 |
+
# PyInstaller
|
31 |
+
# Usually these files are written by a python script from a template
|
32 |
+
# before PyInstaller builds the exe, so as to inject date/other infos into it.
|
33 |
+
*.manifest
|
34 |
+
*.spec
|
35 |
+
|
36 |
+
# Installer logs
|
37 |
+
pip-log.txt
|
38 |
+
pip-delete-this-directory.txt
|
39 |
+
|
40 |
+
# Unit test / coverage reports
|
41 |
+
htmlcov/
|
42 |
+
.tox/
|
43 |
+
.nox/
|
44 |
+
.coverage
|
45 |
+
.coverage.*
|
46 |
+
.cache
|
47 |
+
nosetests.xml
|
48 |
+
coverage.xml
|
49 |
+
*.cover
|
50 |
+
*.py,cover
|
51 |
+
.hypothesis/
|
52 |
+
.pytest_cache/
|
53 |
+
|
54 |
+
# Translations
|
55 |
+
*.mo
|
56 |
+
*.pot
|
57 |
+
|
58 |
+
# Django stuff:
|
59 |
+
*.log
|
60 |
+
local_settings.py
|
61 |
+
db.sqlite3
|
62 |
+
db.sqlite3-journal
|
63 |
+
|
64 |
+
# Flask stuff:
|
65 |
+
instance/
|
66 |
+
.webassets-cache
|
67 |
+
|
68 |
+
# Scrapy stuff:
|
69 |
+
.scrapy
|
70 |
+
|
71 |
+
# Sphinx documentation
|
72 |
+
docs/_build/
|
73 |
+
|
74 |
+
# PyBuilder
|
75 |
+
target/
|
76 |
+
|
77 |
+
# Jupyter Notebook
|
78 |
+
.ipynb_checkpoints
|
79 |
+
|
80 |
+
# IPython
|
81 |
+
profile_default/
|
82 |
+
ipython_config.py
|
83 |
+
|
84 |
+
# Profiling
|
85 |
+
*.pclprof
|
86 |
+
|
87 |
+
# pyenv
|
88 |
+
.python-version
|
89 |
+
|
90 |
+
# pipenv
|
91 |
+
# According to pypa/pipenv#598, it is recommended to include Pipfile.lock in version control.
|
92 |
+
# However, in case of collaboration, if having platform-specific dependencies or dependencies
|
93 |
+
# having no cross-platform support, pipenv may install dependencies that don't work, or not
|
94 |
+
# install all needed dependencies.
|
95 |
+
#Pipfile.lock
|
96 |
+
|
97 |
+
# PEP 582; used by e.g. github.com/David-OConnor/pyflow
|
98 |
+
__pypackages__/
|
99 |
+
|
100 |
+
# Celery stuff
|
101 |
+
celerybeat-schedule
|
102 |
+
celerybeat.pid
|
103 |
+
|
104 |
+
# SageMath parsed files
|
105 |
+
*.sage.py
|
106 |
+
|
107 |
+
# Environments
|
108 |
+
.env
|
109 |
+
.venv
|
110 |
+
.idea
|
111 |
+
env/
|
112 |
+
venv/
|
113 |
+
ENV/
|
114 |
+
env.bak/
|
115 |
+
venv.bak/
|
116 |
+
|
117 |
+
# Spyder project settings
|
118 |
+
.spyderproject
|
119 |
+
.spyproject
|
120 |
+
|
121 |
+
# VSCode project settings
|
122 |
+
.vscode/
|
123 |
+
|
124 |
+
# Rope project settings
|
125 |
+
.ropeproject
|
126 |
+
|
127 |
+
# mkdocs documentation
|
128 |
+
/site
|
129 |
+
mkdocs_github_authors.yaml
|
130 |
+
|
131 |
+
# mypy
|
132 |
+
.mypy_cache/
|
133 |
+
.dmypy.json
|
134 |
+
dmypy.json
|
135 |
+
|
136 |
+
# Pyre type checker
|
137 |
+
.pyre/
|
138 |
+
|
139 |
+
# datasets and projects
|
140 |
+
datasets/
|
141 |
+
runs/
|
142 |
+
wandb/
|
143 |
+
tests/
|
144 |
+
.DS_Store
|
145 |
+
|
146 |
+
# Neural Network weights -----------------------------------------------------------------------------------------------
|
147 |
+
weights/
|
148 |
+
*.weights
|
149 |
+
*.pt
|
150 |
+
*.pb
|
151 |
+
*.onnx
|
152 |
+
*.engine
|
153 |
+
*.mlmodel
|
154 |
+
*.mlpackage
|
155 |
+
*.torchscript
|
156 |
+
*.tflite
|
157 |
+
*.h5
|
158 |
+
*_saved_model/
|
159 |
+
*_web_model/
|
160 |
+
*_openvino_model/
|
161 |
+
*_paddle_model/
|
162 |
+
pnnx*
|
163 |
+
|
164 |
+
# Autogenerated files for tests
|
165 |
+
/ultralytics/assets/
|
ultralytics/.pre-commit-config.yaml
ADDED
@@ -0,0 +1,88 @@
|
|
|
|
|
|
|
|
|
|
|
|
|
|
|
|
|
|
|
|
|
|
|
|
|
|
|
|
|
|
|
|
|
|
|
|
|
|
|
|
|
|
|
|
|
|
|
|
|
|
|
|
|
|
|
|
|
|
|
|
|
|
|
|
|
|
|
|
|
|
|
|
|
|
|
|
|
|
|
|
|
|
|
|
|
|
|
|
|
|
|
|
|
|
|
|
|
|
|
|
|
|
|
|
|
|
|
|
|
|
|
|
|
|
|
|
|
|
|
|
|
|
|
|
|
|
|
|
|
|
|
|
|
|
|
|
|
|
|
|
|
|
|
|
|
|
|
|
|
|
|
|
|
|
|
|
|
|
|
|
|
|
|
|
|
|
|
|
|
|
|
|
|
|
|
|
|
|
|
1 |
+
# Ultralytics YOLO 🚀, AGPL-3.0 license
|
2 |
+
# Pre-commit hooks. For more information see https://github.com/pre-commit/pre-commit-hooks/blob/main/README.md
|
3 |
+
# Optionally remove from local hooks with 'rm .git/hooks/pre-commit'
|
4 |
+
|
5 |
+
# Define bot property if installed via https://github.com/marketplace/pre-commit-ci
|
6 |
+
ci:
|
7 |
+
autofix_prs: true
|
8 |
+
autoupdate_commit_msg: '[pre-commit.ci] pre-commit suggestions'
|
9 |
+
autoupdate_schedule: monthly
|
10 |
+
submodules: true
|
11 |
+
|
12 |
+
# Exclude directories (optional)
|
13 |
+
# exclude: 'docs/'
|
14 |
+
|
15 |
+
# Define repos to run
|
16 |
+
repos:
|
17 |
+
- repo: https://github.com/pre-commit/pre-commit-hooks
|
18 |
+
rev: v4.5.0
|
19 |
+
hooks:
|
20 |
+
- id: end-of-file-fixer
|
21 |
+
- id: trailing-whitespace
|
22 |
+
- id: check-case-conflict
|
23 |
+
# - id: check-yaml
|
24 |
+
- id: check-docstring-first
|
25 |
+
- id: double-quote-string-fixer
|
26 |
+
- id: detect-private-key
|
27 |
+
|
28 |
+
- repo: https://github.com/asottile/pyupgrade
|
29 |
+
rev: v3.15.0
|
30 |
+
hooks:
|
31 |
+
- id: pyupgrade
|
32 |
+
name: Upgrade code
|
33 |
+
|
34 |
+
- repo: https://github.com/PyCQA/isort
|
35 |
+
rev: 5.12.0
|
36 |
+
hooks:
|
37 |
+
- id: isort
|
38 |
+
name: Sort imports
|
39 |
+
|
40 |
+
- repo: https://github.com/google/yapf
|
41 |
+
rev: v0.40.2
|
42 |
+
hooks:
|
43 |
+
- id: yapf
|
44 |
+
name: YAPF formatting
|
45 |
+
|
46 |
+
- repo: https://github.com/executablebooks/mdformat
|
47 |
+
rev: 0.7.17
|
48 |
+
hooks:
|
49 |
+
- id: mdformat
|
50 |
+
name: MD formatting
|
51 |
+
additional_dependencies:
|
52 |
+
- mdformat-gfm
|
53 |
+
- mdformat-black
|
54 |
+
exclude: 'docs/.*\.md'
|
55 |
+
# exclude: "README.md|README.zh-CN.md|CONTRIBUTING.md"
|
56 |
+
|
57 |
+
- repo: https://github.com/PyCQA/flake8
|
58 |
+
rev: 6.1.0
|
59 |
+
hooks:
|
60 |
+
- id: flake8
|
61 |
+
name: PEP8
|
62 |
+
|
63 |
+
- repo: https://github.com/codespell-project/codespell
|
64 |
+
rev: v2.2.6
|
65 |
+
hooks:
|
66 |
+
- id: codespell
|
67 |
+
args:
|
68 |
+
- --ignore-words-list=crate,nd,strack,dota,ane,segway,fo,gool,winn
|
69 |
+
|
70 |
+
- repo: https://github.com/PyCQA/docformatter
|
71 |
+
rev: v1.7.5
|
72 |
+
hooks:
|
73 |
+
- id: docformatter
|
74 |
+
|
75 |
+
# - repo: https://github.com/asottile/yesqa
|
76 |
+
# rev: v1.4.0
|
77 |
+
# hooks:
|
78 |
+
# - id: yesqa
|
79 |
+
|
80 |
+
# - repo: https://github.com/asottile/dead
|
81 |
+
# rev: v1.5.0
|
82 |
+
# hooks:
|
83 |
+
# - id: dead
|
84 |
+
|
85 |
+
# - repo: https://github.com/ultralytics/pre-commit
|
86 |
+
# rev: bd60a414f80a53fb8f593d3bfed4701fc47e4b23
|
87 |
+
# hooks:
|
88 |
+
# - id: capitalize-comments
|
ultralytics/CITATION.cff
ADDED
@@ -0,0 +1,20 @@
|
|
|
|
|
|
|
|
|
|
|
|
|
|
|
|
|
|
|
|
|
|
|
|
|
|
|
|
|
|
|
|
|
|
|
|
|
|
|
|
|
|
|
1 |
+
cff-version: 1.2.0
|
2 |
+
preferred-citation:
|
3 |
+
type: software
|
4 |
+
message: If you use this software, please cite it as below.
|
5 |
+
authors:
|
6 |
+
- family-names: Jocher
|
7 |
+
given-names: Glenn
|
8 |
+
orcid: "https://orcid.org/0000-0001-5950-6979"
|
9 |
+
- family-names: Chaurasia
|
10 |
+
given-names: Ayush
|
11 |
+
orcid: "https://orcid.org/0000-0002-7603-6750"
|
12 |
+
- family-names: Qiu
|
13 |
+
given-names: Jing
|
14 |
+
orcid: "https://orcid.org/0000-0003-3783-7069"
|
15 |
+
title: "YOLO by Ultralytics"
|
16 |
+
version: 8.0.0
|
17 |
+
# doi: 10.5281/zenodo.3908559 # TODO
|
18 |
+
date-released: 2023-1-10
|
19 |
+
license: AGPL-3.0
|
20 |
+
url: "https://github.com/ultralytics/ultralytics"
|
ultralytics/CONTRIBUTING.md
ADDED
@@ -0,0 +1,115 @@
|
|
|
|
|
|
|
|
|
|
|
|
|
|
|
|
|
|
|
|
|
|
|
|
|
|
|
|
|
|
|
|
|
|
|
|
|
|
|
|
|
|
|
|
|
|
|
|
|
|
|
|
|
|
|
|
|
|
|
|
|
|
|
|
|
|
|
|
|
|
|
|
|
|
|
|
|
|
|
|
|
|
|
|
|
|
|
|
|
|
|
|
|
|
|
|
|
|
|
|
|
|
|
|
|
|
|
|
|
|
|
|
|
|
|
|
|
|
|
|
|
|
|
|
|
|
|
|
|
|
|
|
|
|
|
|
|
|
|
|
|
|
|
|
|
|
|
|
|
|
|
|
|
|
|
|
|
|
|
|
|
|
|
|
|
|
|
|
|
|
|
|
|
|
|
|
|
|
|
|
|
|
|
|
|
|
|
|
|
|
|
|
|
|
|
|
|
|
|
|
|
|
|
|
|
|
|
|
|
|
|
|
|
|
|
|
|
|
|
|
|
|
|
|
|
|
|
|
|
|
|
|
|
1 |
+
## Contributing to YOLOv8 🚀
|
2 |
+
|
3 |
+
We love your input! We want to make contributing to YOLOv8 as easy and transparent as possible, whether it's:
|
4 |
+
|
5 |
+
- Reporting a bug
|
6 |
+
- Discussing the current state of the code
|
7 |
+
- Submitting a fix
|
8 |
+
- Proposing a new feature
|
9 |
+
- Becoming a maintainer
|
10 |
+
|
11 |
+
YOLOv8 works so well due to our combined community effort, and for every small improvement you contribute you will be
|
12 |
+
helping push the frontiers of what's possible in AI 😃!
|
13 |
+
|
14 |
+
## Submitting a Pull Request (PR) 🛠️
|
15 |
+
|
16 |
+
Submitting a PR is easy! This example shows how to submit a PR for updating `requirements.txt` in 4 steps:
|
17 |
+
|
18 |
+
### 1. Select File to Update
|
19 |
+
|
20 |
+
Select `requirements.txt` to update by clicking on it in GitHub.
|
21 |
+
|
22 |
+
<p align="center"><img width="800" alt="PR_step1" src="https://user-images.githubusercontent.com/26833433/122260847-08be2600-ced4-11eb-828b-8287ace4136c.png"></p>
|
23 |
+
|
24 |
+
### 2. Click 'Edit this file'
|
25 |
+
|
26 |
+
Button is in top-right corner.
|
27 |
+
|
28 |
+
<p align="center"><img width="800" alt="PR_step2" src="https://user-images.githubusercontent.com/26833433/122260844-06f46280-ced4-11eb-9eec-b8a24be519ca.png"></p>
|
29 |
+
|
30 |
+
### 3. Make Changes
|
31 |
+
|
32 |
+
Change `matplotlib` version from `3.2.2` to `3.3`.
|
33 |
+
|
34 |
+
<p align="center"><img width="800" alt="PR_step3" src="https://user-images.githubusercontent.com/26833433/122260853-0a87e980-ced4-11eb-9fd2-3650fb6e0842.png"></p>
|
35 |
+
|
36 |
+
### 4. Preview Changes and Submit PR
|
37 |
+
|
38 |
+
Click on the **Preview changes** tab to verify your updates. At the bottom of the screen select 'Create a **new branch**
|
39 |
+
for this commit', assign your branch a descriptive name such as `fix/matplotlib_version` and click the green **Propose
|
40 |
+
changes** button. All done, your PR is now submitted to YOLOv8 for review and approval 😃!
|
41 |
+
|
42 |
+
<p align="center"><img width="800" alt="PR_step4" src="https://user-images.githubusercontent.com/26833433/122260856-0b208000-ced4-11eb-8e8e-77b6151cbcc3.png"></p>
|
43 |
+
|
44 |
+
### PR recommendations
|
45 |
+
|
46 |
+
To allow your work to be integrated as seamlessly as possible, we advise you to:
|
47 |
+
|
48 |
+
- ✅ Verify your PR is **up-to-date** with `ultralytics/ultralytics` `main` branch. If your PR is behind you can update
|
49 |
+
your code by clicking the 'Update branch' button or by running `git pull` and `git merge main` locally.
|
50 |
+
|
51 |
+
<p align="center"><img width="751" alt="PR recommendation 1" src="https://user-images.githubusercontent.com/26833433/187295893-50ed9f44-b2c9-4138-a614-de69bd1753d7.png"></p>
|
52 |
+
|
53 |
+
- ✅ Verify all YOLOv8 Continuous Integration (CI) **checks are passing**.
|
54 |
+
|
55 |
+
<p align="center"><img width="751" alt="PR recommendation 2" src="https://user-images.githubusercontent.com/26833433/187296922-545c5498-f64a-4d8c-8300-5fa764360da6.png"></p>
|
56 |
+
|
57 |
+
- ✅ Reduce changes to the absolute **minimum** required for your bug fix or feature addition. _"It is not daily increase
|
58 |
+
but daily decrease, hack away the unessential. The closer to the source, the less wastage there is."_ — Bruce Lee
|
59 |
+
|
60 |
+
### Docstrings
|
61 |
+
|
62 |
+
Not all functions or classes require docstrings but when they do, we
|
63 |
+
follow [google-style docstrings format](https://google.github.io/styleguide/pyguide.html#38-comments-and-docstrings).
|
64 |
+
Here is an example:
|
65 |
+
|
66 |
+
```python
|
67 |
+
"""
|
68 |
+
What the function does. Performs NMS on given detection predictions.
|
69 |
+
|
70 |
+
Args:
|
71 |
+
arg1: The description of the 1st argument
|
72 |
+
arg2: The description of the 2nd argument
|
73 |
+
|
74 |
+
Returns:
|
75 |
+
What the function returns. Empty if nothing is returned.
|
76 |
+
|
77 |
+
Raises:
|
78 |
+
Exception Class: When and why this exception can be raised by the function.
|
79 |
+
"""
|
80 |
+
```
|
81 |
+
|
82 |
+
## Submitting a Bug Report 🐛
|
83 |
+
|
84 |
+
If you spot a problem with YOLOv8 please submit a Bug Report!
|
85 |
+
|
86 |
+
For us to start investigating a possible problem we need to be able to reproduce it ourselves first. We've created a few
|
87 |
+
short guidelines below to help users provide what we need in order to get started.
|
88 |
+
|
89 |
+
When asking a question, people will be better able to provide help if you provide **code** that they can easily
|
90 |
+
understand and use to **reproduce** the problem. This is referred to by community members as creating
|
91 |
+
a [minimum reproducible example](https://docs.ultralytics.com/help/minimum_reproducible_example/). Your code that reproduces
|
92 |
+
the problem should be:
|
93 |
+
|
94 |
+
- ✅ **Minimal** – Use as little code as possible that still produces the same problem
|
95 |
+
- ✅ **Complete** – Provide **all** parts someone else needs to reproduce your problem in the question itself
|
96 |
+
- ✅ **Reproducible** – Test the code you're about to provide to make sure it reproduces the problem
|
97 |
+
|
98 |
+
In addition to the above requirements, for [Ultralytics](https://ultralytics.com/) to provide assistance your code
|
99 |
+
should be:
|
100 |
+
|
101 |
+
- ✅ **Current** – Verify that your code is up-to-date with current
|
102 |
+
GitHub [main](https://github.com/ultralytics/ultralytics/tree/main) branch, and if necessary `git pull` or `git clone`
|
103 |
+
a new copy to ensure your problem has not already been resolved by previous commits.
|
104 |
+
- ✅ **Unmodified** – Your problem must be reproducible without any modifications to the codebase in this
|
105 |
+
repository. [Ultralytics](https://ultralytics.com/) does not provide support for custom code ⚠️.
|
106 |
+
|
107 |
+
If you believe your problem meets all of the above criteria, please close this issue and raise a new one using the 🐛
|
108 |
+
**Bug Report** [template](https://github.com/ultralytics/ultralytics/issues/new/choose) and providing
|
109 |
+
a [minimum reproducible example](https://docs.ultralytics.com/help/minimum_reproducible_example/) to help us better
|
110 |
+
understand and diagnose your problem.
|
111 |
+
|
112 |
+
## License
|
113 |
+
|
114 |
+
By contributing, you agree that your contributions will be licensed under
|
115 |
+
the [AGPL-3.0 license](https://choosealicense.com/licenses/agpl-3.0/)
|
ultralytics/LICENSE
ADDED
@@ -0,0 +1,661 @@
|
|
|
|
|
|
|
|
|
|
|
|
|
|
|
|
|
|
|
|
|
|
|
|
|
|
|
|
|
|
|
|
|
|
|
|
|
|
|
|
|
|
|
|
|
|
|
|
|
|
|
|
|
|
|
|
|
|
|
|
|
|
|
|
|
|
|
|
|
|
|
|
|
|
|
|
|
|
|
|
|
|
|
|
|
|
|
|
|
|
|
|
|
|
|
|
|
|
|
|
|
|
|
|
|
|
|
|
|
|
|
|
|
|
|
|
|
|
|
|
|
|
|
|
|
|
|
|
|
|
|
|
|
|
|
|
|
|
|
|
|
|
|
|
|
|
|
|
|
|
|
|
|
|
|
|
|
|
|
|
|
|
|
|
|
|
|
|
|
|
|
|
|
|
|
|
|
|
|
|
|
|
|
|
|
|
|
|
|
|
|
|
|
|
|
|
|
|
|
|
|
|
|
|
|
|
|
|
|
|
|
|
|
|
|
|
|
|
|
|
|
|
|
|
|
|
|
|
|
|
|
|
|
|
|
|
|
|
|
|
|
|
|
|
|
|
|
|
|
|
|
|
|
|
|
|
|
|
|
|
|
|
|
|
|
|
|
|
|
|
|
|
|
|
|
|
|
|
|
|
|
|
|
|
|
|
|
|
|
|
|
|
|
|
|
|
|
|
|
|
|
|
|
|
|
|
|
|
|
|
|
|
|
|
|
|
|
|
|
|
|
|
|
|
|
|
|
|
|
|
|
|
|
|
|
|
|
|
|
|
|
|
|
|
|
|
|
|
|
|
|
|
|
|
|
|
|
|
|
|
|
|
|
|
|
|
|
|
|
|
|
|
|
|
|
|
|
|
|
|
|
|
|
|
|
|
|
|
|
|
|
|
|
|
|
|
|
|
|
|
|
|
|
|
|
|
|
|
|
|
|
|
|
|
|
|
|
|
|
|
|
|
|
|
|
|
|
|
|
|
|
|
|
|
|
|
|
|
|
|
|
|
|
|
|
|
|
|
|
|
|
|
|
|
|
|
|
|
|
|
|
|
|
|
|
|
|
|
|
|
|
|
|
|
|
|
|
|
|
|
|
|
|
|
|
|
|
|
|
|
|
|
|
|
|
|
|
|
|
|
|
|
|
|
|
|
|
|
|
|
|
|
|
|
|
|
|
|
|
|
|
|
|
|
|
|
|
|
|
|
|
|
|
|
|
|
|
|
|
|
|
|
|
|
|
|
|
|
|
|
|
|
|
|
|
|
|
|
|
|
|
|
|
|
|
|
|
|
|
|
|
|
|
|
|
|
|
|
|
|
|
|
|
|
|
|
|
|
|
|
|
|
|
|
|
|
|
|
|
|
|
|
|
|
|
|
|
|
|
|
|
|
|
|
|
|
|
|
|
|
|
|
|
|
|
|
|
|
|
|
|
|
|
|
|
|
|
|
|
|
|
|
|
|
|
|
|
|
|
|
|
|
|
|
|
|
|
|
|
|
|
|
|
|
|
|
|
|
|
|
|
|
|
|
|
|
|
|
|
|
|
|
|
|
|
|
|
|
|
|
|
|
|
|
|
|
|
|
|
|
|
|
|
|
|
|
|
|
|
|
|
|
|
|
|
|
|
|
|
|
|
|
|
|
|
|
|
|
|
|
|
|
|
|
|
|
|
|
|
|
|
|
|
|
|
|
|
|
|
|
|
|
|
|
|
|
|
|
|
|
|
|
|
|
|
|
|
|
|
|
|
|
|
|
|
|
|
|
|
|
|
|
|
|
|
|
|
|
|
|
|
|
|
|
|
|
|
|
|
|
|
|
|
|
|
|
|
|
|
|
|
|
|
|
|
|
|
|
|
|
|
|
|
|
|
|
|
|
|
|
|
|
|
|
|
|
|
|
|
|
|
|
|
|
|
|
|
|
|
|
|
|
|
|
|
|
|
|
|
|
|
|
|
|
|
|
|
|
|
|
|
|
|
|
|
|
|
|
|
|
|
|
|
|
|
|
|
|
|
|
|
|
|
|
|
|
|
|
|
|
|
|
|
|
|
|
|
|
|
|
|
|
|
|
|
|
|
|
|
|
|
|
|
|
|
|
|
|
|
|
|
|
|
|
|
|
|
|
|
|
|
|
|
|
|
|
|
|
|
|
|
|
|
|
|
|
|
|
|
|
|
|
|
|
|
|
|
|
|
|
|
|
|
|
|
|
|
|
|
|
|
|
|
|
|
|
|
|
|
|
|
|
|
|
|
|
|
|
|
|
|
|
|
|
|
|
|
|
|
|
|
|
|
|
|
|
|
|
|
|
|
|
|
|
|
|
|
|
|
|
|
|
|
|
|
|
|
|
|
|
|
|
|
|
|
|
|
|
|
|
|
|
|
|
|
|
|
|
|
|
|
|
|
|
|
|
|
|
|
|
|
|
|
|
|
|
|
|
|
|
|
|
|
|
|
|
|
|
|
|
|
|
|
|
|
|
|
|
|
|
|
|
|
|
|
|
|
|
|
|
|
|
|
|
|
|
|
|
|
|
|
|
|
|
|
|
|
|
|
|
|
|
|
|
|
|
|
|
|
|
|
|
|
|
|
|
|
|
|
|
|
|
|
|
|
|
|
|
|
|
|
|
|
|
|
|
|
|
|
|
|
|
|
|
|
|
|
|
|
|
|
|
|
|
|
|
|
|
|
|
|
|
|
|
|
|
|
|
|
|
|
|
|
|
|
|
|
|
|
|
|
|
|
|
|
|
|
|
|
|
|
|
|
|
|
|
|
|
|
|
|
|
|
|
|
|
|
|
|
|
|
|
|
|
|
|
|
|
|
|
|
|
|
|
|
|
|
|
|
|
|
|
|
|
|
|
|
|
|
|
|
|
|
|
|
|
|
|
|
|
|
|
|
|
|
|
|
|
|
|
|
|
|
|
|
|
|
|
|
|
|
|
|
|
|
|
|
|
|
|
|
|
|
|
|
|
|
|
|
|
|
|
|
|
|
1 |
+
GNU AFFERO GENERAL PUBLIC LICENSE
|
2 |
+
Version 3, 19 November 2007
|
3 |
+
|
4 |
+
Copyright (C) 2007 Free Software Foundation, Inc. <https://fsf.org/>
|
5 |
+
Everyone is permitted to copy and distribute verbatim copies
|
6 |
+
of this license document, but changing it is not allowed.
|
7 |
+
|
8 |
+
Preamble
|
9 |
+
|
10 |
+
The GNU Affero General Public License is a free, copyleft license for
|
11 |
+
software and other kinds of works, specifically designed to ensure
|
12 |
+
cooperation with the community in the case of network server software.
|
13 |
+
|
14 |
+
The licenses for most software and other practical works are designed
|
15 |
+
to take away your freedom to share and change the works. By contrast,
|
16 |
+
our General Public Licenses are intended to guarantee your freedom to
|
17 |
+
share and change all versions of a program--to make sure it remains free
|
18 |
+
software for all its users.
|
19 |
+
|
20 |
+
When we speak of free software, we are referring to freedom, not
|
21 |
+
price. Our General Public Licenses are designed to make sure that you
|
22 |
+
have the freedom to distribute copies of free software (and charge for
|
23 |
+
them if you wish), that you receive source code or can get it if you
|
24 |
+
want it, that you can change the software or use pieces of it in new
|
25 |
+
free programs, and that you know you can do these things.
|
26 |
+
|
27 |
+
Developers that use our General Public Licenses protect your rights
|
28 |
+
with two steps: (1) assert copyright on the software, and (2) offer
|
29 |
+
you this License which gives you legal permission to copy, distribute
|
30 |
+
and/or modify the software.
|
31 |
+
|
32 |
+
A secondary benefit of defending all users' freedom is that
|
33 |
+
improvements made in alternate versions of the program, if they
|
34 |
+
receive widespread use, become available for other developers to
|
35 |
+
incorporate. Many developers of free software are heartened and
|
36 |
+
encouraged by the resulting cooperation. However, in the case of
|
37 |
+
software used on network servers, this result may fail to come about.
|
38 |
+
The GNU General Public License permits making a modified version and
|
39 |
+
letting the public access it on a server without ever releasing its
|
40 |
+
source code to the public.
|
41 |
+
|
42 |
+
The GNU Affero General Public License is designed specifically to
|
43 |
+
ensure that, in such cases, the modified source code becomes available
|
44 |
+
to the community. It requires the operator of a network server to
|
45 |
+
provide the source code of the modified version running there to the
|
46 |
+
users of that server. Therefore, public use of a modified version, on
|
47 |
+
a publicly accessible server, gives the public access to the source
|
48 |
+
code of the modified version.
|
49 |
+
|
50 |
+
An older license, called the Affero General Public License and
|
51 |
+
published by Affero, was designed to accomplish similar goals. This is
|
52 |
+
a different license, not a version of the Affero GPL, but Affero has
|
53 |
+
released a new version of the Affero GPL which permits relicensing under
|
54 |
+
this license.
|
55 |
+
|
56 |
+
The precise terms and conditions for copying, distribution and
|
57 |
+
modification follow.
|
58 |
+
|
59 |
+
TERMS AND CONDITIONS
|
60 |
+
|
61 |
+
0. Definitions.
|
62 |
+
|
63 |
+
"This License" refers to version 3 of the GNU Affero General Public License.
|
64 |
+
|
65 |
+
"Copyright" also means copyright-like laws that apply to other kinds of
|
66 |
+
works, such as semiconductor masks.
|
67 |
+
|
68 |
+
"The Program" refers to any copyrightable work licensed under this
|
69 |
+
License. Each licensee is addressed as "you". "Licensees" and
|
70 |
+
"recipients" may be individuals or organizations.
|
71 |
+
|
72 |
+
To "modify" a work means to copy from or adapt all or part of the work
|
73 |
+
in a fashion requiring copyright permission, other than the making of an
|
74 |
+
exact copy. The resulting work is called a "modified version" of the
|
75 |
+
earlier work or a work "based on" the earlier work.
|
76 |
+
|
77 |
+
A "covered work" means either the unmodified Program or a work based
|
78 |
+
on the Program.
|
79 |
+
|
80 |
+
To "propagate" a work means to do anything with it that, without
|
81 |
+
permission, would make you directly or secondarily liable for
|
82 |
+
infringement under applicable copyright law, except executing it on a
|
83 |
+
computer or modifying a private copy. Propagation includes copying,
|
84 |
+
distribution (with or without modification), making available to the
|
85 |
+
public, and in some countries other activities as well.
|
86 |
+
|
87 |
+
To "convey" a work means any kind of propagation that enables other
|
88 |
+
parties to make or receive copies. Mere interaction with a user through
|
89 |
+
a computer network, with no transfer of a copy, is not conveying.
|
90 |
+
|
91 |
+
An interactive user interface displays "Appropriate Legal Notices"
|
92 |
+
to the extent that it includes a convenient and prominently visible
|
93 |
+
feature that (1) displays an appropriate copyright notice, and (2)
|
94 |
+
tells the user that there is no warranty for the work (except to the
|
95 |
+
extent that warranties are provided), that licensees may convey the
|
96 |
+
work under this License, and how to view a copy of this License. If
|
97 |
+
the interface presents a list of user commands or options, such as a
|
98 |
+
menu, a prominent item in the list meets this criterion.
|
99 |
+
|
100 |
+
1. Source Code.
|
101 |
+
|
102 |
+
The "source code" for a work means the preferred form of the work
|
103 |
+
for making modifications to it. "Object code" means any non-source
|
104 |
+
form of a work.
|
105 |
+
|
106 |
+
A "Standard Interface" means an interface that either is an official
|
107 |
+
standard defined by a recognized standards body, or, in the case of
|
108 |
+
interfaces specified for a particular programming language, one that
|
109 |
+
is widely used among developers working in that language.
|
110 |
+
|
111 |
+
The "System Libraries" of an executable work include anything, other
|
112 |
+
than the work as a whole, that (a) is included in the normal form of
|
113 |
+
packaging a Major Component, but which is not part of that Major
|
114 |
+
Component, and (b) serves only to enable use of the work with that
|
115 |
+
Major Component, or to implement a Standard Interface for which an
|
116 |
+
implementation is available to the public in source code form. A
|
117 |
+
"Major Component", in this context, means a major essential component
|
118 |
+
(kernel, window system, and so on) of the specific operating system
|
119 |
+
(if any) on which the executable work runs, or a compiler used to
|
120 |
+
produce the work, or an object code interpreter used to run it.
|
121 |
+
|
122 |
+
The "Corresponding Source" for a work in object code form means all
|
123 |
+
the source code needed to generate, install, and (for an executable
|
124 |
+
work) run the object code and to modify the work, including scripts to
|
125 |
+
control those activities. However, it does not include the work's
|
126 |
+
System Libraries, or general-purpose tools or generally available free
|
127 |
+
programs which are used unmodified in performing those activities but
|
128 |
+
which are not part of the work. For example, Corresponding Source
|
129 |
+
includes interface definition files associated with source files for
|
130 |
+
the work, and the source code for shared libraries and dynamically
|
131 |
+
linked subprograms that the work is specifically designed to require,
|
132 |
+
such as by intimate data communication or control flow between those
|
133 |
+
subprograms and other parts of the work.
|
134 |
+
|
135 |
+
The Corresponding Source need not include anything that users
|
136 |
+
can regenerate automatically from other parts of the Corresponding
|
137 |
+
Source.
|
138 |
+
|
139 |
+
The Corresponding Source for a work in source code form is that
|
140 |
+
same work.
|
141 |
+
|
142 |
+
2. Basic Permissions.
|
143 |
+
|
144 |
+
All rights granted under this License are granted for the term of
|
145 |
+
copyright on the Program, and are irrevocable provided the stated
|
146 |
+
conditions are met. This License explicitly affirms your unlimited
|
147 |
+
permission to run the unmodified Program. The output from running a
|
148 |
+
covered work is covered by this License only if the output, given its
|
149 |
+
content, constitutes a covered work. This License acknowledges your
|
150 |
+
rights of fair use or other equivalent, as provided by copyright law.
|
151 |
+
|
152 |
+
You may make, run and propagate covered works that you do not
|
153 |
+
convey, without conditions so long as your license otherwise remains
|
154 |
+
in force. You may convey covered works to others for the sole purpose
|
155 |
+
of having them make modifications exclusively for you, or provide you
|
156 |
+
with facilities for running those works, provided that you comply with
|
157 |
+
the terms of this License in conveying all material for which you do
|
158 |
+
not control copyright. Those thus making or running the covered works
|
159 |
+
for you must do so exclusively on your behalf, under your direction
|
160 |
+
and control, on terms that prohibit them from making any copies of
|
161 |
+
your copyrighted material outside their relationship with you.
|
162 |
+
|
163 |
+
Conveying under any other circumstances is permitted solely under
|
164 |
+
the conditions stated below. Sublicensing is not allowed; section 10
|
165 |
+
makes it unnecessary.
|
166 |
+
|
167 |
+
3. Protecting Users' Legal Rights From Anti-Circumvention Law.
|
168 |
+
|
169 |
+
No covered work shall be deemed part of an effective technological
|
170 |
+
measure under any applicable law fulfilling obligations under article
|
171 |
+
11 of the WIPO copyright treaty adopted on 20 December 1996, or
|
172 |
+
similar laws prohibiting or restricting circumvention of such
|
173 |
+
measures.
|
174 |
+
|
175 |
+
When you convey a covered work, you waive any legal power to forbid
|
176 |
+
circumvention of technological measures to the extent such circumvention
|
177 |
+
is effected by exercising rights under this License with respect to
|
178 |
+
the covered work, and you disclaim any intention to limit operation or
|
179 |
+
modification of the work as a means of enforcing, against the work's
|
180 |
+
users, your or third parties' legal rights to forbid circumvention of
|
181 |
+
technological measures.
|
182 |
+
|
183 |
+
4. Conveying Verbatim Copies.
|
184 |
+
|
185 |
+
You may convey verbatim copies of the Program's source code as you
|
186 |
+
receive it, in any medium, provided that you conspicuously and
|
187 |
+
appropriately publish on each copy an appropriate copyright notice;
|
188 |
+
keep intact all notices stating that this License and any
|
189 |
+
non-permissive terms added in accord with section 7 apply to the code;
|
190 |
+
keep intact all notices of the absence of any warranty; and give all
|
191 |
+
recipients a copy of this License along with the Program.
|
192 |
+
|
193 |
+
You may charge any price or no price for each copy that you convey,
|
194 |
+
and you may offer support or warranty protection for a fee.
|
195 |
+
|
196 |
+
5. Conveying Modified Source Versions.
|
197 |
+
|
198 |
+
You may convey a work based on the Program, or the modifications to
|
199 |
+
produce it from the Program, in the form of source code under the
|
200 |
+
terms of section 4, provided that you also meet all of these conditions:
|
201 |
+
|
202 |
+
a) The work must carry prominent notices stating that you modified
|
203 |
+
it, and giving a relevant date.
|
204 |
+
|
205 |
+
b) The work must carry prominent notices stating that it is
|
206 |
+
released under this License and any conditions added under section
|
207 |
+
7. This requirement modifies the requirement in section 4 to
|
208 |
+
"keep intact all notices".
|
209 |
+
|
210 |
+
c) You must license the entire work, as a whole, under this
|
211 |
+
License to anyone who comes into possession of a copy. This
|
212 |
+
License will therefore apply, along with any applicable section 7
|
213 |
+
additional terms, to the whole of the work, and all its parts,
|
214 |
+
regardless of how they are packaged. This License gives no
|
215 |
+
permission to license the work in any other way, but it does not
|
216 |
+
invalidate such permission if you have separately received it.
|
217 |
+
|
218 |
+
d) If the work has interactive user interfaces, each must display
|
219 |
+
Appropriate Legal Notices; however, if the Program has interactive
|
220 |
+
interfaces that do not display Appropriate Legal Notices, your
|
221 |
+
work need not make them do so.
|
222 |
+
|
223 |
+
A compilation of a covered work with other separate and independent
|
224 |
+
works, which are not by their nature extensions of the covered work,
|
225 |
+
and which are not combined with it such as to form a larger program,
|
226 |
+
in or on a volume of a storage or distribution medium, is called an
|
227 |
+
"aggregate" if the compilation and its resulting copyright are not
|
228 |
+
used to limit the access or legal rights of the compilation's users
|
229 |
+
beyond what the individual works permit. Inclusion of a covered work
|
230 |
+
in an aggregate does not cause this License to apply to the other
|
231 |
+
parts of the aggregate.
|
232 |
+
|
233 |
+
6. Conveying Non-Source Forms.
|
234 |
+
|
235 |
+
You may convey a covered work in object code form under the terms
|
236 |
+
of sections 4 and 5, provided that you also convey the
|
237 |
+
machine-readable Corresponding Source under the terms of this License,
|
238 |
+
in one of these ways:
|
239 |
+
|
240 |
+
a) Convey the object code in, or embodied in, a physical product
|
241 |
+
(including a physical distribution medium), accompanied by the
|
242 |
+
Corresponding Source fixed on a durable physical medium
|
243 |
+
customarily used for software interchange.
|
244 |
+
|
245 |
+
b) Convey the object code in, or embodied in, a physical product
|
246 |
+
(including a physical distribution medium), accompanied by a
|
247 |
+
written offer, valid for at least three years and valid for as
|
248 |
+
long as you offer spare parts or customer support for that product
|
249 |
+
model, to give anyone who possesses the object code either (1) a
|
250 |
+
copy of the Corresponding Source for all the software in the
|
251 |
+
product that is covered by this License, on a durable physical
|
252 |
+
medium customarily used for software interchange, for a price no
|
253 |
+
more than your reasonable cost of physically performing this
|
254 |
+
conveying of source, or (2) access to copy the
|
255 |
+
Corresponding Source from a network server at no charge.
|
256 |
+
|
257 |
+
c) Convey individual copies of the object code with a copy of the
|
258 |
+
written offer to provide the Corresponding Source. This
|
259 |
+
alternative is allowed only occasionally and noncommercially, and
|
260 |
+
only if you received the object code with such an offer, in accord
|
261 |
+
with subsection 6b.
|
262 |
+
|
263 |
+
d) Convey the object code by offering access from a designated
|
264 |
+
place (gratis or for a charge), and offer equivalent access to the
|
265 |
+
Corresponding Source in the same way through the same place at no
|
266 |
+
further charge. You need not require recipients to copy the
|
267 |
+
Corresponding Source along with the object code. If the place to
|
268 |
+
copy the object code is a network server, the Corresponding Source
|
269 |
+
may be on a different server (operated by you or a third party)
|
270 |
+
that supports equivalent copying facilities, provided you maintain
|
271 |
+
clear directions next to the object code saying where to find the
|
272 |
+
Corresponding Source. Regardless of what server hosts the
|
273 |
+
Corresponding Source, you remain obligated to ensure that it is
|
274 |
+
available for as long as needed to satisfy these requirements.
|
275 |
+
|
276 |
+
e) Convey the object code using peer-to-peer transmission, provided
|
277 |
+
you inform other peers where the object code and Corresponding
|
278 |
+
Source of the work are being offered to the general public at no
|
279 |
+
charge under subsection 6d.
|
280 |
+
|
281 |
+
A separable portion of the object code, whose source code is excluded
|
282 |
+
from the Corresponding Source as a System Library, need not be
|
283 |
+
included in conveying the object code work.
|
284 |
+
|
285 |
+
A "User Product" is either (1) a "consumer product", which means any
|
286 |
+
tangible personal property which is normally used for personal, family,
|
287 |
+
or household purposes, or (2) anything designed or sold for incorporation
|
288 |
+
into a dwelling. In determining whether a product is a consumer product,
|
289 |
+
doubtful cases shall be resolved in favor of coverage. For a particular
|
290 |
+
product received by a particular user, "normally used" refers to a
|
291 |
+
typical or common use of that class of product, regardless of the status
|
292 |
+
of the particular user or of the way in which the particular user
|
293 |
+
actually uses, or expects or is expected to use, the product. A product
|
294 |
+
is a consumer product regardless of whether the product has substantial
|
295 |
+
commercial, industrial or non-consumer uses, unless such uses represent
|
296 |
+
the only significant mode of use of the product.
|
297 |
+
|
298 |
+
"Installation Information" for a User Product means any methods,
|
299 |
+
procedures, authorization keys, or other information required to install
|
300 |
+
and execute modified versions of a covered work in that User Product from
|
301 |
+
a modified version of its Corresponding Source. The information must
|
302 |
+
suffice to ensure that the continued functioning of the modified object
|
303 |
+
code is in no case prevented or interfered with solely because
|
304 |
+
modification has been made.
|
305 |
+
|
306 |
+
If you convey an object code work under this section in, or with, or
|
307 |
+
specifically for use in, a User Product, and the conveying occurs as
|
308 |
+
part of a transaction in which the right of possession and use of the
|
309 |
+
User Product is transferred to the recipient in perpetuity or for a
|
310 |
+
fixed term (regardless of how the transaction is characterized), the
|
311 |
+
Corresponding Source conveyed under this section must be accompanied
|
312 |
+
by the Installation Information. But this requirement does not apply
|
313 |
+
if neither you nor any third party retains the ability to install
|
314 |
+
modified object code on the User Product (for example, the work has
|
315 |
+
been installed in ROM).
|
316 |
+
|
317 |
+
The requirement to provide Installation Information does not include a
|
318 |
+
requirement to continue to provide support service, warranty, or updates
|
319 |
+
for a work that has been modified or installed by the recipient, or for
|
320 |
+
the User Product in which it has been modified or installed. Access to a
|
321 |
+
network may be denied when the modification itself materially and
|
322 |
+
adversely affects the operation of the network or violates the rules and
|
323 |
+
protocols for communication across the network.
|
324 |
+
|
325 |
+
Corresponding Source conveyed, and Installation Information provided,
|
326 |
+
in accord with this section must be in a format that is publicly
|
327 |
+
documented (and with an implementation available to the public in
|
328 |
+
source code form), and must require no special password or key for
|
329 |
+
unpacking, reading or copying.
|
330 |
+
|
331 |
+
7. Additional Terms.
|
332 |
+
|
333 |
+
"Additional permissions" are terms that supplement the terms of this
|
334 |
+
License by making exceptions from one or more of its conditions.
|
335 |
+
Additional permissions that are applicable to the entire Program shall
|
336 |
+
be treated as though they were included in this License, to the extent
|
337 |
+
that they are valid under applicable law. If additional permissions
|
338 |
+
apply only to part of the Program, that part may be used separately
|
339 |
+
under those permissions, but the entire Program remains governed by
|
340 |
+
this License without regard to the additional permissions.
|
341 |
+
|
342 |
+
When you convey a copy of a covered work, you may at your option
|
343 |
+
remove any additional permissions from that copy, or from any part of
|
344 |
+
it. (Additional permissions may be written to require their own
|
345 |
+
removal in certain cases when you modify the work.) You may place
|
346 |
+
additional permissions on material, added by you to a covered work,
|
347 |
+
for which you have or can give appropriate copyright permission.
|
348 |
+
|
349 |
+
Notwithstanding any other provision of this License, for material you
|
350 |
+
add to a covered work, you may (if authorized by the copyright holders of
|
351 |
+
that material) supplement the terms of this License with terms:
|
352 |
+
|
353 |
+
a) Disclaiming warranty or limiting liability differently from the
|
354 |
+
terms of sections 15 and 16 of this License; or
|
355 |
+
|
356 |
+
b) Requiring preservation of specified reasonable legal notices or
|
357 |
+
author attributions in that material or in the Appropriate Legal
|
358 |
+
Notices displayed by works containing it; or
|
359 |
+
|
360 |
+
c) Prohibiting misrepresentation of the origin of that material, or
|
361 |
+
requiring that modified versions of such material be marked in
|
362 |
+
reasonable ways as different from the original version; or
|
363 |
+
|
364 |
+
d) Limiting the use for publicity purposes of names of licensors or
|
365 |
+
authors of the material; or
|
366 |
+
|
367 |
+
e) Declining to grant rights under trademark law for use of some
|
368 |
+
trade names, trademarks, or service marks; or
|
369 |
+
|
370 |
+
f) Requiring indemnification of licensors and authors of that
|
371 |
+
material by anyone who conveys the material (or modified versions of
|
372 |
+
it) with contractual assumptions of liability to the recipient, for
|
373 |
+
any liability that these contractual assumptions directly impose on
|
374 |
+
those licensors and authors.
|
375 |
+
|
376 |
+
All other non-permissive additional terms are considered "further
|
377 |
+
restrictions" within the meaning of section 10. If the Program as you
|
378 |
+
received it, or any part of it, contains a notice stating that it is
|
379 |
+
governed by this License along with a term that is a further
|
380 |
+
restriction, you may remove that term. If a license document contains
|
381 |
+
a further restriction but permits relicensing or conveying under this
|
382 |
+
License, you may add to a covered work material governed by the terms
|
383 |
+
of that license document, provided that the further restriction does
|
384 |
+
not survive such relicensing or conveying.
|
385 |
+
|
386 |
+
If you add terms to a covered work in accord with this section, you
|
387 |
+
must place, in the relevant source files, a statement of the
|
388 |
+
additional terms that apply to those files, or a notice indicating
|
389 |
+
where to find the applicable terms.
|
390 |
+
|
391 |
+
Additional terms, permissive or non-permissive, may be stated in the
|
392 |
+
form of a separately written license, or stated as exceptions;
|
393 |
+
the above requirements apply either way.
|
394 |
+
|
395 |
+
8. Termination.
|
396 |
+
|
397 |
+
You may not propagate or modify a covered work except as expressly
|
398 |
+
provided under this License. Any attempt otherwise to propagate or
|
399 |
+
modify it is void, and will automatically terminate your rights under
|
400 |
+
this License (including any patent licenses granted under the third
|
401 |
+
paragraph of section 11).
|
402 |
+
|
403 |
+
However, if you cease all violation of this License, then your
|
404 |
+
license from a particular copyright holder is reinstated (a)
|
405 |
+
provisionally, unless and until the copyright holder explicitly and
|
406 |
+
finally terminates your license, and (b) permanently, if the copyright
|
407 |
+
holder fails to notify you of the violation by some reasonable means
|
408 |
+
prior to 60 days after the cessation.
|
409 |
+
|
410 |
+
Moreover, your license from a particular copyright holder is
|
411 |
+
reinstated permanently if the copyright holder notifies you of the
|
412 |
+
violation by some reasonable means, this is the first time you have
|
413 |
+
received notice of violation of this License (for any work) from that
|
414 |
+
copyright holder, and you cure the violation prior to 30 days after
|
415 |
+
your receipt of the notice.
|
416 |
+
|
417 |
+
Termination of your rights under this section does not terminate the
|
418 |
+
licenses of parties who have received copies or rights from you under
|
419 |
+
this License. If your rights have been terminated and not permanently
|
420 |
+
reinstated, you do not qualify to receive new licenses for the same
|
421 |
+
material under section 10.
|
422 |
+
|
423 |
+
9. Acceptance Not Required for Having Copies.
|
424 |
+
|
425 |
+
You are not required to accept this License in order to receive or
|
426 |
+
run a copy of the Program. Ancillary propagation of a covered work
|
427 |
+
occurring solely as a consequence of using peer-to-peer transmission
|
428 |
+
to receive a copy likewise does not require acceptance. However,
|
429 |
+
nothing other than this License grants you permission to propagate or
|
430 |
+
modify any covered work. These actions infringe copyright if you do
|
431 |
+
not accept this License. Therefore, by modifying or propagating a
|
432 |
+
covered work, you indicate your acceptance of this License to do so.
|
433 |
+
|
434 |
+
10. Automatic Licensing of Downstream Recipients.
|
435 |
+
|
436 |
+
Each time you convey a covered work, the recipient automatically
|
437 |
+
receives a license from the original licensors, to run, modify and
|
438 |
+
propagate that work, subject to this License. You are not responsible
|
439 |
+
for enforcing compliance by third parties with this License.
|
440 |
+
|
441 |
+
An "entity transaction" is a transaction transferring control of an
|
442 |
+
organization, or substantially all assets of one, or subdividing an
|
443 |
+
organization, or merging organizations. If propagation of a covered
|
444 |
+
work results from an entity transaction, each party to that
|
445 |
+
transaction who receives a copy of the work also receives whatever
|
446 |
+
licenses to the work the party's predecessor in interest had or could
|
447 |
+
give under the previous paragraph, plus a right to possession of the
|
448 |
+
Corresponding Source of the work from the predecessor in interest, if
|
449 |
+
the predecessor has it or can get it with reasonable efforts.
|
450 |
+
|
451 |
+
You may not impose any further restrictions on the exercise of the
|
452 |
+
rights granted or affirmed under this License. For example, you may
|
453 |
+
not impose a license fee, royalty, or other charge for exercise of
|
454 |
+
rights granted under this License, and you may not initiate litigation
|
455 |
+
(including a cross-claim or counterclaim in a lawsuit) alleging that
|
456 |
+
any patent claim is infringed by making, using, selling, offering for
|
457 |
+
sale, or importing the Program or any portion of it.
|
458 |
+
|
459 |
+
11. Patents.
|
460 |
+
|
461 |
+
A "contributor" is a copyright holder who authorizes use under this
|
462 |
+
License of the Program or a work on which the Program is based. The
|
463 |
+
work thus licensed is called the contributor's "contributor version".
|
464 |
+
|
465 |
+
A contributor's "essential patent claims" are all patent claims
|
466 |
+
owned or controlled by the contributor, whether already acquired or
|
467 |
+
hereafter acquired, that would be infringed by some manner, permitted
|
468 |
+
by this License, of making, using, or selling its contributor version,
|
469 |
+
but do not include claims that would be infringed only as a
|
470 |
+
consequence of further modification of the contributor version. For
|
471 |
+
purposes of this definition, "control" includes the right to grant
|
472 |
+
patent sublicenses in a manner consistent with the requirements of
|
473 |
+
this License.
|
474 |
+
|
475 |
+
Each contributor grants you a non-exclusive, worldwide, royalty-free
|
476 |
+
patent license under the contributor's essential patent claims, to
|
477 |
+
make, use, sell, offer for sale, import and otherwise run, modify and
|
478 |
+
propagate the contents of its contributor version.
|
479 |
+
|
480 |
+
In the following three paragraphs, a "patent license" is any express
|
481 |
+
agreement or commitment, however denominated, not to enforce a patent
|
482 |
+
(such as an express permission to practice a patent or covenant not to
|
483 |
+
sue for patent infringement). To "grant" such a patent license to a
|
484 |
+
party means to make such an agreement or commitment not to enforce a
|
485 |
+
patent against the party.
|
486 |
+
|
487 |
+
If you convey a covered work, knowingly relying on a patent license,
|
488 |
+
and the Corresponding Source of the work is not available for anyone
|
489 |
+
to copy, free of charge and under the terms of this License, through a
|
490 |
+
publicly available network server or other readily accessible means,
|
491 |
+
then you must either (1) cause the Corresponding Source to be so
|
492 |
+
available, or (2) arrange to deprive yourself of the benefit of the
|
493 |
+
patent license for this particular work, or (3) arrange, in a manner
|
494 |
+
consistent with the requirements of this License, to extend the patent
|
495 |
+
license to downstream recipients. "Knowingly relying" means you have
|
496 |
+
actual knowledge that, but for the patent license, your conveying the
|
497 |
+
covered work in a country, or your recipient's use of the covered work
|
498 |
+
in a country, would infringe one or more identifiable patents in that
|
499 |
+
country that you have reason to believe are valid.
|
500 |
+
|
501 |
+
If, pursuant to or in connection with a single transaction or
|
502 |
+
arrangement, you convey, or propagate by procuring conveyance of, a
|
503 |
+
covered work, and grant a patent license to some of the parties
|
504 |
+
receiving the covered work authorizing them to use, propagate, modify
|
505 |
+
or convey a specific copy of the covered work, then the patent license
|
506 |
+
you grant is automatically extended to all recipients of the covered
|
507 |
+
work and works based on it.
|
508 |
+
|
509 |
+
A patent license is "discriminatory" if it does not include within
|
510 |
+
the scope of its coverage, prohibits the exercise of, or is
|
511 |
+
conditioned on the non-exercise of one or more of the rights that are
|
512 |
+
specifically granted under this License. You may not convey a covered
|
513 |
+
work if you are a party to an arrangement with a third party that is
|
514 |
+
in the business of distributing software, under which you make payment
|
515 |
+
to the third party based on the extent of your activity of conveying
|
516 |
+
the work, and under which the third party grants, to any of the
|
517 |
+
parties who would receive the covered work from you, a discriminatory
|
518 |
+
patent license (a) in connection with copies of the covered work
|
519 |
+
conveyed by you (or copies made from those copies), or (b) primarily
|
520 |
+
for and in connection with specific products or compilations that
|
521 |
+
contain the covered work, unless you entered into that arrangement,
|
522 |
+
or that patent license was granted, prior to 28 March 2007.
|
523 |
+
|
524 |
+
Nothing in this License shall be construed as excluding or limiting
|
525 |
+
any implied license or other defenses to infringement that may
|
526 |
+
otherwise be available to you under applicable patent law.
|
527 |
+
|
528 |
+
12. No Surrender of Others' Freedom.
|
529 |
+
|
530 |
+
If conditions are imposed on you (whether by court order, agreement or
|
531 |
+
otherwise) that contradict the conditions of this License, they do not
|
532 |
+
excuse you from the conditions of this License. If you cannot convey a
|
533 |
+
covered work so as to satisfy simultaneously your obligations under this
|
534 |
+
License and any other pertinent obligations, then as a consequence you may
|
535 |
+
not convey it at all. For example, if you agree to terms that obligate you
|
536 |
+
to collect a royalty for further conveying from those to whom you convey
|
537 |
+
the Program, the only way you could satisfy both those terms and this
|
538 |
+
License would be to refrain entirely from conveying the Program.
|
539 |
+
|
540 |
+
13. Remote Network Interaction; Use with the GNU General Public License.
|
541 |
+
|
542 |
+
Notwithstanding any other provision of this License, if you modify the
|
543 |
+
Program, your modified version must prominently offer all users
|
544 |
+
interacting with it remotely through a computer network (if your version
|
545 |
+
supports such interaction) an opportunity to receive the Corresponding
|
546 |
+
Source of your version by providing access to the Corresponding Source
|
547 |
+
from a network server at no charge, through some standard or customary
|
548 |
+
means of facilitating copying of software. This Corresponding Source
|
549 |
+
shall include the Corresponding Source for any work covered by version 3
|
550 |
+
of the GNU General Public License that is incorporated pursuant to the
|
551 |
+
following paragraph.
|
552 |
+
|
553 |
+
Notwithstanding any other provision of this License, you have
|
554 |
+
permission to link or combine any covered work with a work licensed
|
555 |
+
under version 3 of the GNU General Public License into a single
|
556 |
+
combined work, and to convey the resulting work. The terms of this
|
557 |
+
License will continue to apply to the part which is the covered work,
|
558 |
+
but the work with which it is combined will remain governed by version
|
559 |
+
3 of the GNU General Public License.
|
560 |
+
|
561 |
+
14. Revised Versions of this License.
|
562 |
+
|
563 |
+
The Free Software Foundation may publish revised and/or new versions of
|
564 |
+
the GNU Affero General Public License from time to time. Such new versions
|
565 |
+
will be similar in spirit to the present version, but may differ in detail to
|
566 |
+
address new problems or concerns.
|
567 |
+
|
568 |
+
Each version is given a distinguishing version number. If the
|
569 |
+
Program specifies that a certain numbered version of the GNU Affero General
|
570 |
+
Public License "or any later version" applies to it, you have the
|
571 |
+
option of following the terms and conditions either of that numbered
|
572 |
+
version or of any later version published by the Free Software
|
573 |
+
Foundation. If the Program does not specify a version number of the
|
574 |
+
GNU Affero General Public License, you may choose any version ever published
|
575 |
+
by the Free Software Foundation.
|
576 |
+
|
577 |
+
If the Program specifies that a proxy can decide which future
|
578 |
+
versions of the GNU Affero General Public License can be used, that proxy's
|
579 |
+
public statement of acceptance of a version permanently authorizes you
|
580 |
+
to choose that version for the Program.
|
581 |
+
|
582 |
+
Later license versions may give you additional or different
|
583 |
+
permissions. However, no additional obligations are imposed on any
|
584 |
+
author or copyright holder as a result of your choosing to follow a
|
585 |
+
later version.
|
586 |
+
|
587 |
+
15. Disclaimer of Warranty.
|
588 |
+
|
589 |
+
THERE IS NO WARRANTY FOR THE PROGRAM, TO THE EXTENT PERMITTED BY
|
590 |
+
APPLICABLE LAW. EXCEPT WHEN OTHERWISE STATED IN WRITING THE COPYRIGHT
|
591 |
+
HOLDERS AND/OR OTHER PARTIES PROVIDE THE PROGRAM "AS IS" WITHOUT WARRANTY
|
592 |
+
OF ANY KIND, EITHER EXPRESSED OR IMPLIED, INCLUDING, BUT NOT LIMITED TO,
|
593 |
+
THE IMPLIED WARRANTIES OF MERCHANTABILITY AND FITNESS FOR A PARTICULAR
|
594 |
+
PURPOSE. THE ENTIRE RISK AS TO THE QUALITY AND PERFORMANCE OF THE PROGRAM
|
595 |
+
IS WITH YOU. SHOULD THE PROGRAM PROVE DEFECTIVE, YOU ASSUME THE COST OF
|
596 |
+
ALL NECESSARY SERVICING, REPAIR OR CORRECTION.
|
597 |
+
|
598 |
+
16. Limitation of Liability.
|
599 |
+
|
600 |
+
IN NO EVENT UNLESS REQUIRED BY APPLICABLE LAW OR AGREED TO IN WRITING
|
601 |
+
WILL ANY COPYRIGHT HOLDER, OR ANY OTHER PARTY WHO MODIFIES AND/OR CONVEYS
|
602 |
+
THE PROGRAM AS PERMITTED ABOVE, BE LIABLE TO YOU FOR DAMAGES, INCLUDING ANY
|
603 |
+
GENERAL, SPECIAL, INCIDENTAL OR CONSEQUENTIAL DAMAGES ARISING OUT OF THE
|
604 |
+
USE OR INABILITY TO USE THE PROGRAM (INCLUDING BUT NOT LIMITED TO LOSS OF
|
605 |
+
DATA OR DATA BEING RENDERED INACCURATE OR LOSSES SUSTAINED BY YOU OR THIRD
|
606 |
+
PARTIES OR A FAILURE OF THE PROGRAM TO OPERATE WITH ANY OTHER PROGRAMS),
|
607 |
+
EVEN IF SUCH HOLDER OR OTHER PARTY HAS BEEN ADVISED OF THE POSSIBILITY OF
|
608 |
+
SUCH DAMAGES.
|
609 |
+
|
610 |
+
17. Interpretation of Sections 15 and 16.
|
611 |
+
|
612 |
+
If the disclaimer of warranty and limitation of liability provided
|
613 |
+
above cannot be given local legal effect according to their terms,
|
614 |
+
reviewing courts shall apply local law that most closely approximates
|
615 |
+
an absolute waiver of all civil liability in connection with the
|
616 |
+
Program, unless a warranty or assumption of liability accompanies a
|
617 |
+
copy of the Program in return for a fee.
|
618 |
+
|
619 |
+
END OF TERMS AND CONDITIONS
|
620 |
+
|
621 |
+
How to Apply These Terms to Your New Programs
|
622 |
+
|
623 |
+
If you develop a new program, and you want it to be of the greatest
|
624 |
+
possible use to the public, the best way to achieve this is to make it
|
625 |
+
free software which everyone can redistribute and change under these terms.
|
626 |
+
|
627 |
+
To do so, attach the following notices to the program. It is safest
|
628 |
+
to attach them to the start of each source file to most effectively
|
629 |
+
state the exclusion of warranty; and each file should have at least
|
630 |
+
the "copyright" line and a pointer to where the full notice is found.
|
631 |
+
|
632 |
+
<one line to give the program's name and a brief idea of what it does.>
|
633 |
+
Copyright (C) <year> <name of author>
|
634 |
+
|
635 |
+
This program is free software: you can redistribute it and/or modify
|
636 |
+
it under the terms of the GNU Affero General Public License as published by
|
637 |
+
the Free Software Foundation, either version 3 of the License, or
|
638 |
+
(at your option) any later version.
|
639 |
+
|
640 |
+
This program is distributed in the hope that it will be useful,
|
641 |
+
but WITHOUT ANY WARRANTY; without even the implied warranty of
|
642 |
+
MERCHANTABILITY or FITNESS FOR A PARTICULAR PURPOSE. See the
|
643 |
+
GNU Affero General Public License for more details.
|
644 |
+
|
645 |
+
You should have received a copy of the GNU Affero General Public License
|
646 |
+
along with this program. If not, see <https://www.gnu.org/licenses/>.
|
647 |
+
|
648 |
+
Also add information on how to contact you by electronic and paper mail.
|
649 |
+
|
650 |
+
If your software can interact with users remotely through a computer
|
651 |
+
network, you should also make sure that it provides a way for users to
|
652 |
+
get its source. For example, if your program is a web application, its
|
653 |
+
interface could display a "Source" link that leads users to an archive
|
654 |
+
of the code. There are many ways you could offer source, and different
|
655 |
+
solutions will be better for different programs; see section 13 for the
|
656 |
+
specific requirements.
|
657 |
+
|
658 |
+
You should also get your employer (if you work as a programmer) or school,
|
659 |
+
if any, to sign a "copyright disclaimer" for the program, if necessary.
|
660 |
+
For more information on this, and how to apply and follow the GNU AGPL, see
|
661 |
+
<https://www.gnu.org/licenses/>.
|
ultralytics/MANIFEST.in
ADDED
@@ -0,0 +1,8 @@
|
|
|
|
|
|
|
|
|
|
|
|
|
|
|
|
|
|
|
1 |
+
include *.md
|
2 |
+
include requirements.txt
|
3 |
+
include LICENSE
|
4 |
+
include setup.py
|
5 |
+
include ultralytics/assets/bus.jpg
|
6 |
+
include ultralytics/assets/zidane.jpg
|
7 |
+
include tests/*.py
|
8 |
+
recursive-include ultralytics *.yaml
|
ultralytics/README.md
ADDED
@@ -0,0 +1,276 @@
|
|
|
|
|
|
|
|
|
|
|
|
|
|
|
|
|
|
|
|
|
|
|
|
|
|
|
|
|
|
|
|
|
|
|
|
|
|
|
|
|
|
|
|
|
|
|
|
|
|
|
|
|
|
|
|
|
|
|
|
|
|
|
|
|
|
|
|
|
|
|
|
|
|
|
|
|
|
|
|
|
|
|
|
|
|
|
|
|
|
|
|
|
|
|
|
|
|
|
|
|
|
|
|
|
|
|
|
|
|
|
|
|
|
|
|
|
|
|
|
|
|
|
|
|
|
|
|
|
|
|
|
|
|
|
|
|
|
|
|
|
|
|
|
|
|
|
|
|
|
|
|
|
|
|
|
|
|
|
|
|
|
|
|
|
|
|
|
|
|
|
|
|
|
|
|
|
|
|
|
|
|
|
|
|
|
|
|
|
|
|
|
|
|
|
|
|
|
|
|
|
|
|
|
|
|
|
|
|
|
|
|
|
|
|
|
|
|
|
|
|
|
|
|
|
|
|
|
|
|
|
|
|
|
|
|
|
|
|
|
|
|
|
|
|
|
|
|
|
|
|
|
|
|
|
|
|
|
|
|
|
|
|
|
|
|
|
|
|
|
|
|
|
|
|
|
|
|
|
|
|
|
|
|
|
|
|
|
|
|
|
|
|
|
|
|
|
|
|
|
|
|
|
|
|
|
|
|
|
|
|
|
|
|
|
|
|
|
|
|
|
|
|
|
|
|
|
|
|
|
|
|
|
|
|
|
|
|
|
|
|
|
|
|
|
|
|
|
|
|
|
|
|
|
|
|
|
|
|
|
|
|
|
|
|
|
|
|
|
|
|
|
|
|
|
|
|
|
|
|
|
|
|
|
|
|
|
|
|
|
|
|
|
|
|
|
|
|
|
|
|
|
|
|
|
|
|
|
|
|
|
|
|
|
|
|
|
|
|
|
|
|
|
|
|
|
|
|
|
|
|
|
|
|
|
|
|
|
|
|
|
|
|
|
|
|
|
|
|
|
|
|
|
|
|
|
|
|
|
|
|
|
|
|
|
|
|
|
|
|
|
|
|
|
|
|
|
|
|
|
|
|
|
|
|
|
|
|
|
|
|
|
|
|
|
|
|
|
|
|
|
|
|
|
|
|
|
|
|
|
|
|
|
|
|
|
|
|
|
|
|
|
|
|
|
|
|
|
|
|
|
|
|
|
|
|
|
|
|
|
|
|
|
|
|
|
|
|
|
|
|
|
|
|
|
1 |
+
<div align="center">
|
2 |
+
<p>
|
3 |
+
<a href="https://yolovision.ultralytics.com/" target="_blank">
|
4 |
+
<img width="100%" src="https://raw.githubusercontent.com/ultralytics/assets/main/im/banner-yolo-vision-2023.png"></a>
|
5 |
+
</p>
|
6 |
+
|
7 |
+
[English](README.md) | [简体中文](README.zh-CN.md)
|
8 |
+
<br>
|
9 |
+
|
10 |
+
<div>
|
11 |
+
<a href="https://github.com/ultralytics/ultralytics/actions/workflows/ci.yaml"><img src="https://github.com/ultralytics/ultralytics/actions/workflows/ci.yaml/badge.svg" alt="Ultralytics CI"></a>
|
12 |
+
<a href="https://codecov.io/github/ultralytics/ultralytics"><img src="https://codecov.io/github/ultralytics/ultralytics/branch/main/graph/badge.svg?token=HHW7IIVFVY" alt="Ultralytics Code Coverage"></a>
|
13 |
+
<a href="https://zenodo.org/badge/latestdoi/264818686"><img src="https://zenodo.org/badge/264818686.svg" alt="YOLOv8 Citation"></a>
|
14 |
+
<a href="https://hub.docker.com/r/ultralytics/ultralytics"><img src="https://img.shields.io/docker/pulls/ultralytics/ultralytics?logo=docker" alt="Docker Pulls"></a>
|
15 |
+
<br>
|
16 |
+
<a href="https://console.paperspace.com/github/ultralytics/ultralytics"><img src="https://assets.paperspace.io/img/gradient-badge.svg" alt="Run on Gradient"/></a>
|
17 |
+
<a href="https://colab.research.google.com/github/ultralytics/ultralytics/blob/main/examples/tutorial.ipynb"><img src="https://colab.research.google.com/assets/colab-badge.svg" alt="Open In Colab"></a>
|
18 |
+
<a href="https://www.kaggle.com/ultralytics/yolov8"><img src="https://kaggle.com/static/images/open-in-kaggle.svg" alt="Open In Kaggle"></a>
|
19 |
+
</div>
|
20 |
+
<br>
|
21 |
+
|
22 |
+
[Ultralytics](https://ultralytics.com) [YOLOv8](https://github.com/ultralytics/ultralytics) is a cutting-edge, state-of-the-art (SOTA) model that builds upon the success of previous YOLO versions and introduces new features and improvements to further boost performance and flexibility. YOLOv8 is designed to be fast, accurate, and easy to use, making it an excellent choice for a wide range of object detection and tracking, instance segmentation, image classification and pose estimation tasks.
|
23 |
+
|
24 |
+
We hope that the resources here will help you get the most out of YOLOv8. Please browse the YOLOv8 <a href="https://docs.ultralytics.com/">Docs</a> for details, raise an issue on <a href="https://github.com/ultralytics/ultralytics/issues/new/choose">GitHub</a> for support, and join our <a href="https://ultralytics.com/discord">Discord</a> community for questions and discussions!
|
25 |
+
|
26 |
+
To request an Enterprise License please complete the form at [Ultralytics Licensing](https://ultralytics.com/license).
|
27 |
+
|
28 |
+
<img width="100%" src="https://raw.githubusercontent.com/ultralytics/assets/main/yolov8/yolo-comparison-plots.png"></a>
|
29 |
+
|
30 |
+
<div align="center">
|
31 |
+
<a href="https://github.com/ultralytics"><img src="https://github.com/ultralytics/assets/raw/main/social/logo-social-github.png" width="2%" alt="Ultralytics GitHub"></a>
|
32 |
+
<img src="https://github.com/ultralytics/assets/raw/main/social/logo-transparent.png" width="2%">
|
33 |
+
<a href="https://www.linkedin.com/company/ultralytics/"><img src="https://github.com/ultralytics/assets/raw/main/social/logo-social-linkedin.png" width="2%" alt="Ultralytics LinkedIn"></a>
|
34 |
+
<img src="https://github.com/ultralytics/assets/raw/main/social/logo-transparent.png" width="2%">
|
35 |
+
<a href="https://twitter.com/ultralytics"><img src="https://github.com/ultralytics/assets/raw/main/social/logo-social-twitter.png" width="2%" alt="Ultralytics Twitter"></a>
|
36 |
+
<img src="https://github.com/ultralytics/assets/raw/main/social/logo-transparent.png" width="2%">
|
37 |
+
<a href="https://youtube.com/ultralytics"><img src="https://github.com/ultralytics/assets/raw/main/social/logo-social-youtube.png" width="2%" alt="Ultralytics YouTube"></a>
|
38 |
+
<img src="https://github.com/ultralytics/assets/raw/main/social/logo-transparent.png" width="2%">
|
39 |
+
<a href="https://www.tiktok.com/@ultralytics"><img src="https://github.com/ultralytics/assets/raw/main/social/logo-social-tiktok.png" width="2%" alt="Ultralytics TikTok"></a>
|
40 |
+
<img src="https://github.com/ultralytics/assets/raw/main/social/logo-transparent.png" width="2%">
|
41 |
+
<a href="https://www.instagram.com/ultralytics/"><img src="https://github.com/ultralytics/assets/raw/main/social/logo-social-instagram.png" width="2%" alt="Ultralytics Instagram"></a>
|
42 |
+
<img src="https://github.com/ultralytics/assets/raw/main/social/logo-transparent.png" width="2%">
|
43 |
+
<a href="https://ultralytics.com/discord"><img src="https://github.com/ultralytics/assets/raw/main/social/logo-social-discord.png" width="2%" alt="Ultralytics Discord"></a>
|
44 |
+
</div>
|
45 |
+
</div>
|
46 |
+
|
47 |
+
## <div align="center">Documentation</div>
|
48 |
+
|
49 |
+
See below for a quickstart installation and usage example, and see the [YOLOv8 Docs](https://docs.ultralytics.com) for full documentation on training, validation, prediction and deployment.
|
50 |
+
|
51 |
+
<details open>
|
52 |
+
<summary>Install</summary>
|
53 |
+
|
54 |
+
Pip install the ultralytics package including all [requirements](https://github.com/ultralytics/ultralytics/blob/main/requirements.txt) in a [**Python>=3.8**](https://www.python.org/) environment with [**PyTorch>=1.8**](https://pytorch.org/get-started/locally/).
|
55 |
+
|
56 |
+
[](https://badge.fury.io/py/ultralytics) [](https://pepy.tech/project/ultralytics)
|
57 |
+
|
58 |
+
```bash
|
59 |
+
pip install ultralytics
|
60 |
+
```
|
61 |
+
|
62 |
+
For alternative installation methods including [Conda](https://anaconda.org/conda-forge/ultralytics), [Docker](https://hub.docker.com/r/ultralytics/ultralytics), and Git, please refer to the [Quickstart Guide](https://docs.ultralytics.com/quickstart).
|
63 |
+
|
64 |
+
</details>
|
65 |
+
|
66 |
+
<details open>
|
67 |
+
<summary>Usage</summary>
|
68 |
+
|
69 |
+
#### CLI
|
70 |
+
|
71 |
+
YOLOv8 may be used directly in the Command Line Interface (CLI) with a `yolo` command:
|
72 |
+
|
73 |
+
```bash
|
74 |
+
yolo predict model=yolov8n.pt source='https://ultralytics.com/images/bus.jpg'
|
75 |
+
```
|
76 |
+
|
77 |
+
`yolo` can be used for a variety of tasks and modes and accepts additional arguments, i.e. `imgsz=640`. See the YOLOv8 [CLI Docs](https://docs.ultralytics.com/usage/cli) for examples.
|
78 |
+
|
79 |
+
#### Python
|
80 |
+
|
81 |
+
YOLOv8 may also be used directly in a Python environment, and accepts the same [arguments](https://docs.ultralytics.com/usage/cfg/) as in the CLI example above:
|
82 |
+
|
83 |
+
```python
|
84 |
+
from ultralytics import YOLO
|
85 |
+
|
86 |
+
# Load a model
|
87 |
+
model = YOLO("yolov8n.yaml") # build a new model from scratch
|
88 |
+
model = YOLO("yolov8n.pt") # load a pretrained model (recommended for training)
|
89 |
+
|
90 |
+
# Use the model
|
91 |
+
model.train(data="coco128.yaml", epochs=3) # train the model
|
92 |
+
metrics = model.val() # evaluate model performance on the validation set
|
93 |
+
results = model("https://ultralytics.com/images/bus.jpg") # predict on an image
|
94 |
+
path = model.export(format="onnx") # export the model to ONNX format
|
95 |
+
```
|
96 |
+
|
97 |
+
[Models](https://github.com/ultralytics/ultralytics/tree/main/ultralytics/cfg/models) download automatically from the latest Ultralytics [release](https://github.com/ultralytics/assets/releases). See YOLOv8 [Python Docs](https://docs.ultralytics.com/usage/python) for more examples.
|
98 |
+
|
99 |
+
</details>
|
100 |
+
|
101 |
+
## <div align="center">Models</div>
|
102 |
+
|
103 |
+
YOLOv8 [Detect](https://docs.ultralytics.com/tasks/detect), [Segment](https://docs.ultralytics.com/tasks/segment) and [Pose](https://docs.ultralytics.com/tasks/pose) models pretrained on the [COCO](https://docs.ultralytics.com/datasets/detect/coco) dataset are available here, as well as YOLOv8 [Classify](https://docs.ultralytics.com/tasks/classify) models pretrained on the [ImageNet](https://docs.ultralytics.com/datasets/classify/imagenet) dataset. [Track](https://docs.ultralytics.com/modes/track) mode is available for all Detect, Segment and Pose models.
|
104 |
+
|
105 |
+
<img width="1024" src="https://raw.githubusercontent.com/ultralytics/assets/main/im/banner-tasks.png" alt="Ultralytics YOLO supported tasks">
|
106 |
+
|
107 |
+
All [Models](https://github.com/ultralytics/ultralytics/tree/main/ultralytics/cfg/models) download automatically from the latest Ultralytics [release](https://github.com/ultralytics/assets/releases) on first use.
|
108 |
+
|
109 |
+
<details open><summary>Detection (COCO)</summary>
|
110 |
+
|
111 |
+
See [Detection Docs](https://docs.ultralytics.com/tasks/detect/) for usage examples with these models trained on [COCO](https://docs.ultralytics.com/datasets/detect/coco/), which include 80 pre-trained classes.
|
112 |
+
|
113 |
+
| Model | size<br><sup>(pixels) | mAP<sup>val<br>50-95 | Speed<br><sup>CPU ONNX<br>(ms) | Speed<br><sup>A100 TensorRT<br>(ms) | params<br><sup>(M) | FLOPs<br><sup>(B) |
|
114 |
+
| ------------------------------------------------------------------------------------ | --------------------- | -------------------- | ------------------------------ | ----------------------------------- | ------------------ | ----------------- |
|
115 |
+
| [YOLOv8n](https://github.com/ultralytics/assets/releases/download/v0.0.0/yolov8n.pt) | 640 | 37.3 | 80.4 | 0.99 | 3.2 | 8.7 |
|
116 |
+
| [YOLOv8s](https://github.com/ultralytics/assets/releases/download/v0.0.0/yolov8s.pt) | 640 | 44.9 | 128.4 | 1.20 | 11.2 | 28.6 |
|
117 |
+
| [YOLOv8m](https://github.com/ultralytics/assets/releases/download/v0.0.0/yolov8m.pt) | 640 | 50.2 | 234.7 | 1.83 | 25.9 | 78.9 |
|
118 |
+
| [YOLOv8l](https://github.com/ultralytics/assets/releases/download/v0.0.0/yolov8l.pt) | 640 | 52.9 | 375.2 | 2.39 | 43.7 | 165.2 |
|
119 |
+
| [YOLOv8x](https://github.com/ultralytics/assets/releases/download/v0.0.0/yolov8x.pt) | 640 | 53.9 | 479.1 | 3.53 | 68.2 | 257.8 |
|
120 |
+
|
121 |
+
- **mAP<sup>val</sup>** values are for single-model single-scale on [COCO val2017](http://cocodataset.org) dataset.
|
122 |
+
<br>Reproduce by `yolo val detect data=coco.yaml device=0`
|
123 |
+
- **Speed** averaged over COCO val images using an [Amazon EC2 P4d](https://aws.amazon.com/ec2/instance-types/p4/) instance.
|
124 |
+
<br>Reproduce by `yolo val detect data=coco.yaml batch=1 device=0|cpu`
|
125 |
+
|
126 |
+
</details>
|
127 |
+
|
128 |
+
<details><summary>Detection (Open Image V7)</summary>
|
129 |
+
|
130 |
+
See [Detection Docs](https://docs.ultralytics.com/tasks/detect/) for usage examples with these models trained on [Open Image V7](https://docs.ultralytics.com/datasets/detect/open-images-v7/), which include 600 pre-trained classes.
|
131 |
+
|
132 |
+
| Model | size<br><sup>(pixels) | mAP<sup>val<br>50-95 | Speed<br><sup>CPU ONNX<br>(ms) | Speed<br><sup>A100 TensorRT<br>(ms) | params<br><sup>(M) | FLOPs<br><sup>(B) |
|
133 |
+
| ----------------------------------------------------------------------------------------- | --------------------- | -------------------- | ------------------------------ | ----------------------------------- | ------------------ | ----------------- |
|
134 |
+
| [YOLOv8n](https://github.com/ultralytics/assets/releases/download/v0.0.0/yolov8n-oiv7.pt) | 640 | 18.4 | 142.4 | 1.21 | 3.5 | 10.5 |
|
135 |
+
| [YOLOv8s](https://github.com/ultralytics/assets/releases/download/v0.0.0/yolov8s-oiv7.pt) | 640 | 27.7 | 183.1 | 1.40 | 11.4 | 29.7 |
|
136 |
+
| [YOLOv8m](https://github.com/ultralytics/assets/releases/download/v0.0.0/yolov8m-oiv7.pt) | 640 | 33.6 | 408.5 | 2.26 | 26.2 | 80.6 |
|
137 |
+
| [YOLOv8l](https://github.com/ultralytics/assets/releases/download/v0.0.0/yolov8l-oiv7.pt) | 640 | 34.9 | 596.9 | 2.43 | 44.1 | 167.4 |
|
138 |
+
| [YOLOv8x](https://github.com/ultralytics/assets/releases/download/v0.0.0/yolov8x-oiv7.pt) | 640 | 36.3 | 860.6 | 3.56 | 68.7 | 260.6 |
|
139 |
+
|
140 |
+
- **mAP<sup>val</sup>** values are for single-model single-scale on [Open Image V7](https://docs.ultralytics.com/datasets/detect/open-images-v7/) dataset.
|
141 |
+
<br>Reproduce by `yolo val detect data=open-images-v7.yaml device=0`
|
142 |
+
- **Speed** averaged over COCO val images using an [Amazon EC2 P4d](https://aws.amazon.com/ec2/instance-types/p4/) instance.
|
143 |
+
<br>Reproduce by `yolo val detect data=open-images-v7.yaml batch=1 device=0|cpu`
|
144 |
+
|
145 |
+
</details>
|
146 |
+
|
147 |
+
<details><summary>Segmentation (COCO)</summary>
|
148 |
+
|
149 |
+
See [Segmentation Docs](https://docs.ultralytics.com/tasks/segment/) for usage examples with these models trained on [COCO-Seg](https://docs.ultralytics.com/datasets/segment/coco/), which include 80 pre-trained classes.
|
150 |
+
|
151 |
+
| Model | size<br><sup>(pixels) | mAP<sup>box<br>50-95 | mAP<sup>mask<br>50-95 | Speed<br><sup>CPU ONNX<br>(ms) | Speed<br><sup>A100 TensorRT<br>(ms) | params<br><sup>(M) | FLOPs<br><sup>(B) |
|
152 |
+
| -------------------------------------------------------------------------------------------- | --------------------- | -------------------- | --------------------- | ------------------------------ | ----------------------------------- | ------------------ | ----------------- |
|
153 |
+
| [YOLOv8n-seg](https://github.com/ultralytics/assets/releases/download/v0.0.0/yolov8n-seg.pt) | 640 | 36.7 | 30.5 | 96.1 | 1.21 | 3.4 | 12.6 |
|
154 |
+
| [YOLOv8s-seg](https://github.com/ultralytics/assets/releases/download/v0.0.0/yolov8s-seg.pt) | 640 | 44.6 | 36.8 | 155.7 | 1.47 | 11.8 | 42.6 |
|
155 |
+
| [YOLOv8m-seg](https://github.com/ultralytics/assets/releases/download/v0.0.0/yolov8m-seg.pt) | 640 | 49.9 | 40.8 | 317.0 | 2.18 | 27.3 | 110.2 |
|
156 |
+
| [YOLOv8l-seg](https://github.com/ultralytics/assets/releases/download/v0.0.0/yolov8l-seg.pt) | 640 | 52.3 | 42.6 | 572.4 | 2.79 | 46.0 | 220.5 |
|
157 |
+
| [YOLOv8x-seg](https://github.com/ultralytics/assets/releases/download/v0.0.0/yolov8x-seg.pt) | 640 | 53.4 | 43.4 | 712.1 | 4.02 | 71.8 | 344.1 |
|
158 |
+
|
159 |
+
- **mAP<sup>val</sup>** values are for single-model single-scale on [COCO val2017](http://cocodataset.org) dataset.
|
160 |
+
<br>Reproduce by `yolo val segment data=coco-seg.yaml device=0`
|
161 |
+
- **Speed** averaged over COCO val images using an [Amazon EC2 P4d](https://aws.amazon.com/ec2/instance-types/p4/) instance.
|
162 |
+
<br>Reproduce by `yolo val segment data=coco-seg.yaml batch=1 device=0|cpu`
|
163 |
+
|
164 |
+
</details>
|
165 |
+
|
166 |
+
<details><summary>Pose (COCO)</summary>
|
167 |
+
|
168 |
+
See [Pose Docs](https://docs.ultralytics.com/tasks/pose/) for usage examples with these models trained on [COCO-Pose](https://docs.ultralytics.com/datasets/pose/coco/), which include 1 pre-trained class, person.
|
169 |
+
|
170 |
+
| Model | size<br><sup>(pixels) | mAP<sup>pose<br>50-95 | mAP<sup>pose<br>50 | Speed<br><sup>CPU ONNX<br>(ms) | Speed<br><sup>A100 TensorRT<br>(ms) | params<br><sup>(M) | FLOPs<br><sup>(B) |
|
171 |
+
| ---------------------------------------------------------------------------------------------------- | --------------------- | --------------------- | ------------------ | ------------------------------ | ----------------------------------- | ------------------ | ----------------- |
|
172 |
+
| [YOLOv8n-pose](https://github.com/ultralytics/assets/releases/download/v0.0.0/yolov8n-pose.pt) | 640 | 50.4 | 80.1 | 131.8 | 1.18 | 3.3 | 9.2 |
|
173 |
+
| [YOLOv8s-pose](https://github.com/ultralytics/assets/releases/download/v0.0.0/yolov8s-pose.pt) | 640 | 60.0 | 86.2 | 233.2 | 1.42 | 11.6 | 30.2 |
|
174 |
+
| [YOLOv8m-pose](https://github.com/ultralytics/assets/releases/download/v0.0.0/yolov8m-pose.pt) | 640 | 65.0 | 88.8 | 456.3 | 2.00 | 26.4 | 81.0 |
|
175 |
+
| [YOLOv8l-pose](https://github.com/ultralytics/assets/releases/download/v0.0.0/yolov8l-pose.pt) | 640 | 67.6 | 90.0 | 784.5 | 2.59 | 44.4 | 168.6 |
|
176 |
+
| [YOLOv8x-pose](https://github.com/ultralytics/assets/releases/download/v0.0.0/yolov8x-pose.pt) | 640 | 69.2 | 90.2 | 1607.1 | 3.73 | 69.4 | 263.2 |
|
177 |
+
| [YOLOv8x-pose-p6](https://github.com/ultralytics/assets/releases/download/v0.0.0/yolov8x-pose-p6.pt) | 1280 | 71.6 | 91.2 | 4088.7 | 10.04 | 99.1 | 1066.4 |
|
178 |
+
|
179 |
+
- **mAP<sup>val</sup>** values are for single-model single-scale on [COCO Keypoints val2017](http://cocodataset.org)
|
180 |
+
dataset.
|
181 |
+
<br>Reproduce by `yolo val pose data=coco-pose.yaml device=0`
|
182 |
+
- **Speed** averaged over COCO val images using an [Amazon EC2 P4d](https://aws.amazon.com/ec2/instance-types/p4/) instance.
|
183 |
+
<br>Reproduce by `yolo val pose data=coco-pose.yaml batch=1 device=0|cpu`
|
184 |
+
|
185 |
+
</details>
|
186 |
+
|
187 |
+
<details><summary>Classification (ImageNet)</summary>
|
188 |
+
|
189 |
+
See [Classification Docs](https://docs.ultralytics.com/tasks/classify/) for usage examples with these models trained on [ImageNet](https://docs.ultralytics.com/datasets/classify/imagenet/), which include 1000 pretrained classes.
|
190 |
+
|
191 |
+
| Model | size<br><sup>(pixels) | acc<br><sup>top1 | acc<br><sup>top5 | Speed<br><sup>CPU ONNX<br>(ms) | Speed<br><sup>A100 TensorRT<br>(ms) | params<br><sup>(M) | FLOPs<br><sup>(B) at 640 |
|
192 |
+
| -------------------------------------------------------------------------------------------- | --------------------- | ---------------- | ---------------- | ------------------------------ | ----------------------------------- | ------------------ | ------------------------ |
|
193 |
+
| [YOLOv8n-cls](https://github.com/ultralytics/assets/releases/download/v0.0.0/yolov8n-cls.pt) | 224 | 66.6 | 87.0 | 12.9 | 0.31 | 2.7 | 4.3 |
|
194 |
+
| [YOLOv8s-cls](https://github.com/ultralytics/assets/releases/download/v0.0.0/yolov8s-cls.pt) | 224 | 72.3 | 91.1 | 23.4 | 0.35 | 6.4 | 13.5 |
|
195 |
+
| [YOLOv8m-cls](https://github.com/ultralytics/assets/releases/download/v0.0.0/yolov8m-cls.pt) | 224 | 76.4 | 93.2 | 85.4 | 0.62 | 17.0 | 42.7 |
|
196 |
+
| [YOLOv8l-cls](https://github.com/ultralytics/assets/releases/download/v0.0.0/yolov8l-cls.pt) | 224 | 78.0 | 94.1 | 163.0 | 0.87 | 37.5 | 99.7 |
|
197 |
+
| [YOLOv8x-cls](https://github.com/ultralytics/assets/releases/download/v0.0.0/yolov8x-cls.pt) | 224 | 78.4 | 94.3 | 232.0 | 1.01 | 57.4 | 154.8 |
|
198 |
+
|
199 |
+
- **acc** values are model accuracies on the [ImageNet](https://www.image-net.org/) dataset validation set.
|
200 |
+
<br>Reproduce by `yolo val classify data=path/to/ImageNet device=0`
|
201 |
+
- **Speed** averaged over ImageNet val images using an [Amazon EC2 P4d](https://aws.amazon.com/ec2/instance-types/p4/) instance.
|
202 |
+
<br>Reproduce by `yolo val classify data=path/to/ImageNet batch=1 device=0|cpu`
|
203 |
+
|
204 |
+
</details>
|
205 |
+
|
206 |
+
## <div align="center">Integrations</div>
|
207 |
+
|
208 |
+
Our key integrations with leading AI platforms extend the functionality of Ultralytics' offerings, enhancing tasks like dataset labeling, training, visualization, and model management. Discover how Ultralytics, in collaboration with [Roboflow](https://roboflow.com/?ref=ultralytics), ClearML, [Comet](https://bit.ly/yolov8-readme-comet), Neural Magic and [OpenVINO](https://docs.ultralytics.com/integrations/openvino), can optimize your AI workflow.
|
209 |
+
|
210 |
+
<br>
|
211 |
+
<a href="https://bit.ly/ultralytics_hub" target="_blank">
|
212 |
+
<img width="100%" src="https://github.com/ultralytics/assets/raw/main/yolov8/banner-integrations.png"></a>
|
213 |
+
<br>
|
214 |
+
<br>
|
215 |
+
|
216 |
+
<div align="center">
|
217 |
+
<a href="https://roboflow.com/?ref=ultralytics">
|
218 |
+
<img src="https://github.com/ultralytics/assets/raw/main/partners/logo-roboflow.png" width="10%"></a>
|
219 |
+
<img src="https://github.com/ultralytics/assets/raw/main/social/logo-transparent.png" width="15%" height="0" alt="">
|
220 |
+
<a href="https://cutt.ly/yolov5-readme-clearml">
|
221 |
+
<img src="https://github.com/ultralytics/assets/raw/main/partners/logo-clearml.png" width="10%"></a>
|
222 |
+
<img src="https://github.com/ultralytics/assets/raw/main/social/logo-transparent.png" width="15%" height="0" alt="">
|
223 |
+
<a href="https://bit.ly/yolov8-readme-comet">
|
224 |
+
<img src="https://github.com/ultralytics/assets/raw/main/partners/logo-comet.png" width="10%"></a>
|
225 |
+
<img src="https://github.com/ultralytics/assets/raw/main/social/logo-transparent.png" width="15%" height="0" alt="">
|
226 |
+
<a href="https://bit.ly/yolov5-neuralmagic">
|
227 |
+
<img src="https://github.com/ultralytics/assets/raw/main/partners/logo-neuralmagic.png" width="10%"></a>
|
228 |
+
</div>
|
229 |
+
|
230 |
+
| Roboflow | ClearML ⭐ NEW | Comet ⭐ NEW | Neural Magic ⭐ NEW |
|
231 |
+
| :--------------------------------------------------------------------------------------------------------------------------: | :---------------------------------------------------------------------------------------------------------------------------------: | :-------------------------------------------------------------------------------------------------------------------------------------------------------: | :----------------------------------------------------------------------------------------------------: |
|
232 |
+
| Label and export your custom datasets directly to YOLOv8 for training with [Roboflow](https://roboflow.com/?ref=ultralytics) | Automatically track, visualize and even remotely train YOLOv8 using [ClearML](https://cutt.ly/yolov5-readme-clearml) (open-source!) | Free forever, [Comet](https://bit.ly/yolov8-readme-comet) lets you save YOLOv8 models, resume training, and interactively visualize and debug predictions | Run YOLOv8 inference up to 6x faster with [Neural Magic DeepSparse](https://bit.ly/yolov5-neuralmagic) |
|
233 |
+
|
234 |
+
## <div align="center">Ultralytics HUB</div>
|
235 |
+
|
236 |
+
Experience seamless AI with [Ultralytics HUB](https://bit.ly/ultralytics_hub) ⭐, the all-in-one solution for data visualization, YOLOv5 and YOLOv8 🚀 model training and deployment, without any coding. Transform images into actionable insights and bring your AI visions to life with ease using our cutting-edge platform and user-friendly [Ultralytics App](https://ultralytics.com/app_install). Start your journey for **Free** now!
|
237 |
+
|
238 |
+
<a href="https://bit.ly/ultralytics_hub" target="_blank">
|
239 |
+
<img width="100%" src="https://github.com/ultralytics/assets/raw/main/im/ultralytics-hub.png" alt="Ultralytics HUB preview image"></a>
|
240 |
+
|
241 |
+
## <div align="center">Contribute</div>
|
242 |
+
|
243 |
+
We love your input! YOLOv5 and YOLOv8 would not be possible without help from our community. Please see our [Contributing Guide](https://docs.ultralytics.com/help/contributing) to get started, and fill out our [Survey](https://ultralytics.com/survey?utm_source=github&utm_medium=social&utm_campaign=Survey) to send us feedback on your experience. Thank you 🙏 to all our contributors!
|
244 |
+
|
245 |
+
<!-- SVG image from https://opencollective.com/ultralytics/contributors.svg?width=990 -->
|
246 |
+
|
247 |
+
<a href="https://github.com/ultralytics/yolov5/graphs/contributors">
|
248 |
+
<img width="100%" src="https://github.com/ultralytics/assets/raw/main/im/image-contributors.png"></a>
|
249 |
+
|
250 |
+
## <div align="center">License</div>
|
251 |
+
|
252 |
+
Ultralytics offers two licensing options to accommodate diverse use cases:
|
253 |
+
|
254 |
+
- **AGPL-3.0 License**: This [OSI-approved](https://opensource.org/licenses/) open-source license is ideal for students and enthusiasts, promoting open collaboration and knowledge sharing. See the [LICENSE](https://github.com/ultralytics/ultralytics/blob/main/LICENSE) file for more details.
|
255 |
+
- **Enterprise License**: Designed for commercial use, this license permits seamless integration of Ultralytics software and AI models into commercial goods and services, bypassing the open-source requirements of AGPL-3.0. If your scenario involves embedding our solutions into a commercial offering, reach out through [Ultralytics Licensing](https://ultralytics.com/license).
|
256 |
+
|
257 |
+
## <div align="center">Contact</div>
|
258 |
+
|
259 |
+
For Ultralytics bug reports and feature requests please visit [GitHub Issues](https://github.com/ultralytics/ultralytics/issues), and join our [Discord](https://ultralytics.com/discord) community for questions and discussions!
|
260 |
+
|
261 |
+
<br>
|
262 |
+
<div align="center">
|
263 |
+
<a href="https://github.com/ultralytics"><img src="https://github.com/ultralytics/assets/raw/main/social/logo-social-github.png" width="3%" alt="Ultralytics GitHub"></a>
|
264 |
+
<img src="https://github.com/ultralytics/assets/raw/main/social/logo-transparent.png" width="3%">
|
265 |
+
<a href="https://www.linkedin.com/company/ultralytics/"><img src="https://github.com/ultralytics/assets/raw/main/social/logo-social-linkedin.png" width="3%" alt="Ultralytics LinkedIn"></a>
|
266 |
+
<img src="https://github.com/ultralytics/assets/raw/main/social/logo-transparent.png" width="3%">
|
267 |
+
<a href="https://twitter.com/ultralytics"><img src="https://github.com/ultralytics/assets/raw/main/social/logo-social-twitter.png" width="3%" alt="Ultralytics Twitter"></a>
|
268 |
+
<img src="https://github.com/ultralytics/assets/raw/main/social/logo-transparent.png" width="3%">
|
269 |
+
<a href="https://youtube.com/ultralytics"><img src="https://github.com/ultralytics/assets/raw/main/social/logo-social-youtube.png" width="3%" alt="Ultralytics YouTube"></a>
|
270 |
+
<img src="https://github.com/ultralytics/assets/raw/main/social/logo-transparent.png" width="3%">
|
271 |
+
<a href="https://www.tiktok.com/@ultralytics"><img src="https://github.com/ultralytics/assets/raw/main/social/logo-social-tiktok.png" width="3%" alt="Ultralytics TikTok"></a>
|
272 |
+
<img src="https://github.com/ultralytics/assets/raw/main/social/logo-transparent.png" width="3%">
|
273 |
+
<a href="https://www.instagram.com/ultralytics/"><img src="https://github.com/ultralytics/assets/raw/main/social/logo-social-instagram.png" width="3%" alt="Ultralytics Instagram"></a>
|
274 |
+
<img src="https://github.com/ultralytics/assets/raw/main/social/logo-transparent.png" width="3%">
|
275 |
+
<a href="https://ultralytics.com/discord"><img src="https://github.com/ultralytics/assets/raw/main/social/logo-social-discord.png" width="3%" alt="Ultralytics Discord"></a>
|
276 |
+
</div>
|
ultralytics/README.zh-CN.md
ADDED
@@ -0,0 +1,275 @@
|
|
|
|
|
|
|
|
|
|
|
|
|
|
|
|
|
|
|
|
|
|
|
|
|
|
|
|
|
|
|
|
|
|
|
|
|
|
|
|
|
|
|
|
|
|
|
|
|
|
|
|
|
|
|
|
|
|
|
|
|
|
|
|
|
|
|
|
|
|
|
|
|
|
|
|
|
|
|
|
|
|
|
|
|
|
|
|
|
|
|
|
|
|
|
|
|
|
|
|
|
|
|
|
|
|
|
|
|
|
|
|
|
|
|
|
|
|
|
|
|
|
|
|
|
|
|
|
|
|
|
|
|
|
|
|
|
|
|
|
|
|
|
|
|
|
|
|
|
|
|
|
|
|
|
|
|
|
|
|
|
|
|
|
|
|
|
|
|
|
|
|
|
|
|
|
|
|
|
|
|
|
|
|
|
|
|
|
|
|
|
|
|
|
|
|
|
|
|
|
|
|
|
|
|
|
|
|
|
|
|
|
|
|
|
|
|
|
|
|
|
|
|
|
|
|
|
|
|
|
|
|
|
|
|
|
|
|
|
|
|
|
|
|
|
|
|
|
|
|
|
|
|
|
|
|
|
|
|
|
|
|
|
|
|
|
|
|
|
|
|
|
|
|
|
|
|
|
|
|
|
|
|
|
|
|
|
|
|
|
|
|
|
|
|
|
|
|
|
|
|
|
|
|
|
|
|
|
|
|
|
|
|
|
|
|
|
|
|
|
|
|
|
|
|
|
|
|
|
|
|
|
|
|
|
|
|
|
|
|
|
|
|
|
|
|
|
|
|
|
|
|
|
|
|
|
|
|
|
|
|
|
|
|
|
|
|
|
|
|
|
|
|
|
|
|
|
|
|
|
|
|
|
|
|
|
|
|
|
|
|
|
|
|
|
|
|
|
|
|
|
|
|
|
|
|
|
|
|
|
|
|
|
|
|
|
|
|
|
|
|
|
|
|
|
|
|
|
|
|
|
|
|
|
|
|
|
|
|
|
|
|
|
|
|
|
|
|
|
|
|
|
|
|
|
|
|
|
|
|
|
|
|
|
|
|
|
|
|
|
|
|
|
|
|
|
|
|
|
|
|
|
|
|
|
|
|
|
|
|
|
|
|
|
|
|
|
|
|
|
|
|
|
|
|
|
|
|
|
|
|
|
|
|
|
|
|
|
|
|
|
|
|
|
|
|
|
|
|
|
|
|
|
|
|
|
|
|
|
|
|
|
|
|
|
|
|
|
|
|
|
|
|
1 |
+
<div align="center">
|
2 |
+
<p>
|
3 |
+
<a href="https://yolovision.ultralytics.com/" target="_blank">
|
4 |
+
<img width="100%" src="https://raw.githubusercontent.com/ultralytics/assets/main/im/banner-yolo-vision-2023.png"></a>
|
5 |
+
</p>
|
6 |
+
|
7 |
+
[English](README.md) | [简体中文](README.zh-CN.md)
|
8 |
+
<br>
|
9 |
+
|
10 |
+
<div>
|
11 |
+
<a href="https://github.com/ultralytics/ultralytics/actions/workflows/ci.yaml"><img src="https://github.com/ultralytics/ultralytics/actions/workflows/ci.yaml/badge.svg" alt="Ultralytics CI"></a>
|
12 |
+
<a href="https://codecov.io/github/ultralytics/ultralytics"><img src="https://codecov.io/github/ultralytics/ultralytics/branch/main/graph/badge.svg?token=HHW7IIVFVY" alt="Ultralytics Code Coverage"></a>
|
13 |
+
<a href="https://zenodo.org/badge/latestdoi/264818686"><img src="https://zenodo.org/badge/264818686.svg" alt="YOLOv8 Citation"></a>
|
14 |
+
<a href="https://hub.docker.com/r/ultralytics/ultralytics"><img src="https://img.shields.io/docker/pulls/ultralytics/ultralytics?logo=docker" alt="Docker Pulls"></a>
|
15 |
+
<br>
|
16 |
+
<a href="https://console.paperspace.com/github/ultralytics/ultralytics"><img src="https://assets.paperspace.io/img/gradient-badge.svg" alt="Run on Gradient"/></a>
|
17 |
+
<a href="https://colab.research.google.com/github/ultralytics/ultralytics/blob/main/examples/tutorial.ipynb"><img src="https://colab.research.google.com/assets/colab-badge.svg" alt="Open In Colab"></a>
|
18 |
+
<a href="https://www.kaggle.com/ultralytics/yolov8"><img src="https://kaggle.com/static/images/open-in-kaggle.svg" alt="Open In Kaggle"></a>
|
19 |
+
</div>
|
20 |
+
<br>
|
21 |
+
|
22 |
+
[Ultralytics](https://ultralytics.com) [YOLOv8](https://github.com/ultralytics/ultralytics) 是一款前沿、最先进(SOTA)的模型,基于先前 YOLO 版本的成功,引入了新功能和改进,进一步提升性能和灵活性。YOLOv8 设计快速、准确且易于使用,使其成为各种物体检测与跟踪、实例分割、图像分类和姿态估计任务的绝佳选择。
|
23 |
+
|
24 |
+
我们希望这里的资源能帮助您充分利用 YOLOv8。请浏览 YOLOv8 <a href="https://docs.ultralytics.com/">文档</a> 了解详细信息,在 <a href="https://github.com/ultralytics/ultralytics/issues/new/choose">GitHub</a> 上提交问题以获得支持,并加入我们的 <a href="https://ultralytics.com/discord">Discord</a> 社区进行问题和讨论!
|
25 |
+
|
26 |
+
如需申请企业许可,请在 [Ultralytics Licensing](https://ultralytics.com/license) 处填写表格
|
27 |
+
|
28 |
+
<img width="100%" src="https://raw.githubusercontent.com/ultralytics/assets/main/yolov8/yolo-comparison-plots.png"></a>
|
29 |
+
|
30 |
+
<div align="center">
|
31 |
+
<a href="https://github.com/ultralytics"><img src="https://github.com/ultralytics/assets/raw/main/social/logo-social-github.png" width="2%" alt="Ultralytics GitHub"></a>
|
32 |
+
<img src="https://github.com/ultralytics/assets/raw/main/social/logo-transparent.png" width="2%">
|
33 |
+
<a href="https://www.linkedin.com/company/ultralytics/"><img src="https://github.com/ultralytics/assets/raw/main/social/logo-social-linkedin.png" width="2%" alt="Ultralytics LinkedIn"></a>
|
34 |
+
<img src="https://github.com/ultralytics/assets/raw/main/social/logo-transparent.png" width="2%">
|
35 |
+
<a href="https://twitter.com/ultralytics"><img src="https://github.com/ultralytics/assets/raw/main/social/logo-social-twitter.png" width="2%" alt="Ultralytics Twitter"></a>
|
36 |
+
<img src="https://github.com/ultralytics/assets/raw/main/social/logo-transparent.png" width="2%">
|
37 |
+
<a href="https://youtube.com/ultralytics"><img src="https://github.com/ultralytics/assets/raw/main/social/logo-social-youtube.png" width="2%" alt="Ultralytics YouTube"></a>
|
38 |
+
<img src="https://github.com/ultralytics/assets/raw/main/social/logo-transparent.png" width="2%">
|
39 |
+
<a href="https://www.tiktok.com/@ultralytics"><img src="https://github.com/ultralytics/assets/raw/main/social/logo-social-tiktok.png" width="2%" alt="Ultralytics TikTok"></a>
|
40 |
+
<img src="https://github.com/ultralytics/assets/raw/main/social/logo-transparent.png" width="2%">
|
41 |
+
<a href="https://www.instagram.com/ultralytics/"><img src="https://github.com/ultralytics/assets/raw/main/social/logo-social-instagram.png" width="2%" alt="Ultralytics Instagram"></a>
|
42 |
+
<img src="https://github.com/ultralytics/assets/raw/main/social/logo-transparent.png" width="2%">
|
43 |
+
<a href="https://ultralytics.com/discord"><img src="https://github.com/ultralytics/assets/raw/main/social/logo-social-discord.png" width="2%" alt="Ultralytics Discord"></a>
|
44 |
+
</div>
|
45 |
+
</div>
|
46 |
+
|
47 |
+
## <div align="center">文档</div>
|
48 |
+
|
49 |
+
请参阅下面的快速安装和使用示例,以及 [YOLOv8 文档](https://docs.ultralytics.com) 上有关培训、验证、预测和部署的完整文档。
|
50 |
+
|
51 |
+
<details open>
|
52 |
+
<summary>安装</summary>
|
53 |
+
|
54 |
+
使用Pip在一个[**Python>=3.8**](https://www.python.org/)环境中安装`ultralytics`包,此环境还需包含[**PyTorch>=1.8**](https://pytorch.org/get-started/locally/)。这也会安装所有必要的[依赖项](https://github.com/ultralytics/ultralytics/blob/main/requirements.txt)。
|
55 |
+
|
56 |
+
[](https://badge.fury.io/py/ultralytics) [](https://pepy.tech/project/ultralytics)
|
57 |
+
|
58 |
+
```bash
|
59 |
+
pip install ultralytics
|
60 |
+
```
|
61 |
+
|
62 |
+
如需使用包括[Conda](https://anaconda.org/conda-forge/ultralytics)、[Docker](https://hub.docker.com/r/ultralytics/ultralytics)和Git在内的其他安装方法,请参考[快速入门指南](https://docs.ultralytics.com/quickstart)。
|
63 |
+
|
64 |
+
</details>
|
65 |
+
|
66 |
+
<details open>
|
67 |
+
<summary>Usage</summary>
|
68 |
+
|
69 |
+
#### CLI
|
70 |
+
|
71 |
+
YOLOv8 可以在命令行界面(CLI)中直接使用,只需输入 `yolo` 命令:
|
72 |
+
|
73 |
+
```bash
|
74 |
+
yolo predict model=yolov8n.pt source='https://ultralytics.com/images/bus.jpg'
|
75 |
+
```
|
76 |
+
|
77 |
+
`yolo` 可用于各种任务和模式,并接受其他参数,例如 `imgsz=640`。查看 YOLOv8 [CLI 文档](https://docs.ultralytics.com/usage/cli)以获取示例。
|
78 |
+
|
79 |
+
#### Python
|
80 |
+
|
81 |
+
YOLOv8 也可以在 Python 环境中直接使用,并接受与上述 CLI 示例中相同的[参数](https://docs.ultralytics.com/usage/cfg/):
|
82 |
+
|
83 |
+
```python
|
84 |
+
from ultralytics import YOLO
|
85 |
+
|
86 |
+
# 加载模型
|
87 |
+
model = YOLO("yolov8n.yaml") # 从头开始构建新模型
|
88 |
+
model = YOLO("yolov8n.pt") # 加载预训练模型(建议用于训练)
|
89 |
+
|
90 |
+
# 使用模型
|
91 |
+
model.train(data="coco128.yaml", epochs=3) # 训练模型
|
92 |
+
metrics = model.val() # 在验证集上评估模型性能
|
93 |
+
results = model("https://ultralytics.com/images/bus.jpg") # 对图像进行预测
|
94 |
+
success = model.export(format="onnx") # 将模型导出为 ONNX 格式
|
95 |
+
```
|
96 |
+
|
97 |
+
[模型](https://github.com/ultralytics/ultralytics/tree/main/ultralytics/cfg/models) 会自动从最新的 Ultralytics [发布版本](https://github.com/ultralytics/assets/releases)中下载。查看 YOLOv8 [Python 文档](https://docs.ultralytics.com/usage/python)以获取更多示例。
|
98 |
+
|
99 |
+
</details>
|
100 |
+
|
101 |
+
## <div align="center">模型</div>
|
102 |
+
|
103 |
+
在[COCO](https://docs.ultralytics.com/datasets/detect/coco)数据集上预训练的YOLOv8 [检测](https://docs.ultralytics.com/tasks/detect),[分割](https://docs.ultralytics.com/tasks/segment)和[姿态](https://docs.ultralytics.com/tasks/pose)模型可以在这里找到,以及在[ImageNet](https://docs.ultralytics.com/datasets/classify/imagenet)数据集上预训练的YOLOv8 [分类](https://docs.ultralytics.com/tasks/classify)模型。所有的检测,分割和姿态模型都支持[追踪](https://docs.ultralytics.com/modes/track)模式。
|
104 |
+
|
105 |
+
<img width="1024" src="https://raw.githubusercontent.com/ultralytics/assets/main/im/banner-tasks.png" alt="Ultralytics YOLO supported tasks">
|
106 |
+
|
107 |
+
所有[模型](https://github.com/ultralytics/ultralytics/tree/main/ultralytics/cfg/models)在首次使用时会自动从最新的Ultralytics [发布版本](https://github.com/ultralytics/assets/releases)下载。
|
108 |
+
|
109 |
+
<details open><summary>检测 (COCO)</summary>
|
110 |
+
|
111 |
+
查看[检测文档](https://docs.ultralytics.com/tasks/detect/)以获取这些在[COCO](https://docs.ultralytics.com/datasets/detect/coco/)上训练的模型的使用示例,其中包括80个预训练类别。
|
112 |
+
|
113 |
+
| 模型 | 尺寸<br><sup>(像素) | mAP<sup>val<br>50-95 | 速度<br><sup>CPU ONNX<br>(ms) | 速度<br><sup>A100 TensorRT<br>(ms) | 参数<br><sup>(M) | FLOPs<br><sup>(B) |
|
114 |
+
| ------------------------------------------------------------------------------------ | --------------- | -------------------- | --------------------------- | -------------------------------- | -------------- | ----------------- |
|
115 |
+
| [YOLOv8n](https://github.com/ultralytics/assets/releases/download/v0.0.0/yolov8n.pt) | 640 | 37.3 | 80.4 | 0.99 | 3.2 | 8.7 |
|
116 |
+
| [YOLOv8s](https://github.com/ultralytics/assets/releases/download/v0.0.0/yolov8s.pt) | 640 | 44.9 | 128.4 | 1.20 | 11.2 | 28.6 |
|
117 |
+
| [YOLOv8m](https://github.com/ultralytics/assets/releases/download/v0.0.0/yolov8m.pt) | 640 | 50.2 | 234.7 | 1.83 | 25.9 | 78.9 |
|
118 |
+
| [YOLOv8l](https://github.com/ultralytics/assets/releases/download/v0.0.0/yolov8l.pt) | 640 | 52.9 | 375.2 | 2.39 | 43.7 | 165.2 |
|
119 |
+
| [YOLOv8x](https://github.com/ultralytics/assets/releases/download/v0.0.0/yolov8x.pt) | 640 | 53.9 | 479.1 | 3.53 | 68.2 | 257.8 |
|
120 |
+
|
121 |
+
- **mAP<sup>val</sup>** 值是基于单模型单尺度在 [COCO val2017](http://cocodataset.org) 数据集上的结果。
|
122 |
+
<br>通过 `yolo val detect data=coco.yaml device=0` 复现
|
123 |
+
- **速度** 是使用 [Amazon EC2 P4d](https://aws.amazon.com/ec2/instance-types/p4/) 实例对 COCO val 图像进行平均计算的。
|
124 |
+
<br>通过 `yolo val detect data=coco.yaml batch=1 device=0|cpu` 复现
|
125 |
+
|
126 |
+
</details>
|
127 |
+
|
128 |
+
<details><summary>检测(Open Image V7)</summary>
|
129 |
+
|
130 |
+
查看[检测文档](https://docs.ultralytics.com/tasks/detect/)以获取这些在[Open Image V7](https://docs.ultralytics.com/datasets/detect/open-images-v7/)上训练的模型的使用示例,其中包括600个预训练类别。
|
131 |
+
|
132 |
+
| 模型 | 尺寸<br><sup>(像素) | mAP<sup>验证<br>50-95 | 速度<br><sup>CPU ONNX<br>(毫秒) | 速度<br><sup>A100 TensorRT<br>(毫秒) | 参数<br><sup>(M) | 浮点运算<br><sup>(B) |
|
133 |
+
| ----------------------------------------------------------------------------------------- | --------------- | ------------------- | --------------------------- | -------------------------------- | -------------- | ---------------- |
|
134 |
+
| [YOLOv8n](https://github.com/ultralytics/assets/releases/download/v0.0.0/yolov8n-oiv7.pt) | 640 | 18.4 | 142.4 | 1.21 | 3.5 | 10.5 |
|
135 |
+
| [YOLOv8s](https://github.com/ultralytics/assets/releases/download/v0.0.0/yolov8s-oiv7.pt) | 640 | 27.7 | 183.1 | 1.40 | 11.4 | 29.7 |
|
136 |
+
| [YOLOv8m](https://github.com/ultralytics/assets/releases/download/v0.0.0/yolov8m-oiv7.pt) | 640 | 33.6 | 408.5 | 2.26 | 26.2 | 80.6 |
|
137 |
+
| [YOLOv8l](https://github.com/ultralytics/assets/releases/download/v0.0.0/yolov8l-oiv7.pt) | 640 | 34.9 | 596.9 | 2.43 | 44.1 | 167.4 |
|
138 |
+
| [YOLOv8x](https://github.com/ultralytics/assets/releases/download/v0.0.0/yolov8x-oiv7.pt) | 640 | 36.3 | 860.6 | 3.56 | 68.7 | 260.6 |
|
139 |
+
|
140 |
+
- **mAP<sup>验证</sup>** 值适用于在[Open Image V7](https://docs.ultralytics.com/datasets/detect/open-images-v7/)数据集上的单模型单尺度。
|
141 |
+
<br>通过 `yolo val detect data=open-images-v7.yaml device=0` 以复现。
|
142 |
+
- **速度** 在使用[Amazon EC2 P4d](https://aws.amazon.com/ec2/instance-types/p4/)实例对COCO验证图像进行平均测算。
|
143 |
+
<br>通过 `yolo val detect data=open-images-v7.yaml batch=1 device=0|cpu` 以复现。
|
144 |
+
|
145 |
+
</details>
|
146 |
+
|
147 |
+
<details><summary>分割 (COCO)</summary>
|
148 |
+
|
149 |
+
查看[分割文档](https://docs.ultralytics.com/tasks/segment/)以获取这些在[COCO-Seg](https://docs.ultralytics.com/datasets/segment/coco/)上训练的模型的使用示例,其中包括80个预训练类别。
|
150 |
+
|
151 |
+
| 模型 | 尺寸<br><sup>(像素) | mAP<sup>box<br>50-95 | mAP<sup>mask<br>50-95 | 速度<br><sup>CPU ONNX<br>(ms) | 速度<br><sup>A100 TensorRT<br>(ms) | 参数<br><sup>(M) | FLOPs<br><sup>(B) |
|
152 |
+
| -------------------------------------------------------------------------------------------- | --------------- | -------------------- | --------------------- | --------------------------- | -------------------------------- | -------------- | ----------------- |
|
153 |
+
| [YOLOv8n-seg](https://github.com/ultralytics/assets/releases/download/v0.0.0/yolov8n-seg.pt) | 640 | 36.7 | 30.5 | 96.1 | 1.21 | 3.4 | 12.6 |
|
154 |
+
| [YOLOv8s-seg](https://github.com/ultralytics/assets/releases/download/v0.0.0/yolov8s-seg.pt) | 640 | 44.6 | 36.8 | 155.7 | 1.47 | 11.8 | 42.6 |
|
155 |
+
| [YOLOv8m-seg](https://github.com/ultralytics/assets/releases/download/v0.0.0/yolov8m-seg.pt) | 640 | 49.9 | 40.8 | 317.0 | 2.18 | 27.3 | 110.2 |
|
156 |
+
| [YOLOv8l-seg](https://github.com/ultralytics/assets/releases/download/v0.0.0/yolov8l-seg.pt) | 640 | 52.3 | 42.6 | 572.4 | 2.79 | 46.0 | 220.5 |
|
157 |
+
| [YOLOv8x-seg](https://github.com/ultralytics/assets/releases/download/v0.0.0/yolov8x-seg.pt) | 640 | 53.4 | 43.4 | 712.1 | 4.02 | 71.8 | 344.1 |
|
158 |
+
|
159 |
+
- **mAP<sup>val</sup>** 值是基于单模型单尺度在 [COCO val2017](http://cocodataset.org) 数据集上的结果。
|
160 |
+
<br>通过 `yolo val segment data=coco-seg.yaml device=0` 复现
|
161 |
+
- **速度** 是使用 [Amazon EC2 P4d](https://aws.amazon.com/ec2/instance-types/p4/) 实例对 COCO val 图像进行平均计算的。
|
162 |
+
<br>通过 `yolo val segment data=coco-seg.yaml batch=1 device=0|cpu` 复现
|
163 |
+
|
164 |
+
</details>
|
165 |
+
|
166 |
+
<details><summary>姿态 (COCO)</summary>
|
167 |
+
|
168 |
+
查看[姿态文档](https://docs.ultralytics.com/tasks/pose/)以获取这些在[COCO-Pose](https://docs.ultralytics.com/datasets/pose/coco/)上训练的模型的使用示例,其中包括1个预训练类别,即人。
|
169 |
+
|
170 |
+
| 模型 | 尺寸<br><sup>(像素) | mAP<sup>pose<br>50-95 | mAP<sup>pose<br>50 | 速度<br><sup>CPU ONNX<br>(ms) | 速度<br><sup>A100 TensorRT<br>(ms) | 参数<br><sup>(M) | FLOPs<br><sup>(B) |
|
171 |
+
| ---------------------------------------------------------------------------------------------------- | --------------- | --------------------- | ------------------ | --------------------------- | -------------------------------- | -------------- | ----------------- |
|
172 |
+
| [YOLOv8n-pose](https://github.com/ultralytics/assets/releases/download/v0.0.0/yolov8n-pose.pt) | 640 | 50.4 | 80.1 | 131.8 | 1.18 | 3.3 | 9.2 |
|
173 |
+
| [YOLOv8s-pose](https://github.com/ultralytics/assets/releases/download/v0.0.0/yolov8s-pose.pt) | 640 | 60.0 | 86.2 | 233.2 | 1.42 | 11.6 | 30.2 |
|
174 |
+
| [YOLOv8m-pose](https://github.com/ultralytics/assets/releases/download/v0.0.0/yolov8m-pose.pt) | 640 | 65.0 | 88.8 | 456.3 | 2.00 | 26.4 | 81.0 |
|
175 |
+
| [YOLOv8l-pose](https://github.com/ultralytics/assets/releases/download/v0.0.0/yolov8l-pose.pt) | 640 | 67.6 | 90.0 | 784.5 | 2.59 | 44.4 | 168.6 |
|
176 |
+
| [YOLOv8x-pose](https://github.com/ultralytics/assets/releases/download/v0.0.0/yolov8x-pose.pt) | 640 | 69.2 | 90.2 | 1607.1 | 3.73 | 69.4 | 263.2 |
|
177 |
+
| [YOLOv8x-pose-p6](https://github.com/ultralytics/assets/releases/download/v0.0.0/yolov8x-pose-p6.pt) | 1280 | 71.6 | 91.2 | 4088.7 | 10.04 | 99.1 | 1066.4 |
|
178 |
+
|
179 |
+
- **mAP<sup>val</sup>** 值是基于单模型单尺度在 [COCO Keypoints val2017](http://cocodataset.org) 数据集上的结果。
|
180 |
+
<br>通过 `yolo val pose data=coco-pose.yaml device=0` 复现
|
181 |
+
- **速度** 是使用 [Amazon EC2 P4d](https://aws.amazon.com/ec2/instance-types/p4/) 实例对 COCO val 图像进行平均计算的。
|
182 |
+
<br>通过 `yolo val pose data=coco-pose.yaml batch=1 device=0|cpu` 复现
|
183 |
+
|
184 |
+
</details>
|
185 |
+
|
186 |
+
<details><summary>分类 (ImageNet)</summary>
|
187 |
+
|
188 |
+
查看[分类文档](https://docs.ultralytics.com/tasks/classify/)以获取这些在[ImageNet](https://docs.ultralytics.com/datasets/classify/imagenet/)上训练的模型的使用示例,其中包括1000个预训练类别。
|
189 |
+
|
190 |
+
| 模型 | 尺寸<br><sup>(像素) | acc<br><sup>top1 | acc<br><sup>top5 | 速度<br><sup>CPU ONNX<br>(ms) | 速度<br><sup>A100 TensorRT<br>(ms) | 参数<br><sup>(M) | FLOPs<br><sup>(B) at 640 |
|
191 |
+
| -------------------------------------------------------------------------------------------- | --------------- | ---------------- | ---------------- | --------------------------- | -------------------------------- | -------------- | ------------------------ |
|
192 |
+
| [YOLOv8n-cls](https://github.com/ultralytics/assets/releases/download/v0.0.0/yolov8n-cls.pt) | 224 | 66.6 | 87.0 | 12.9 | 0.31 | 2.7 | 4.3 |
|
193 |
+
| [YOLOv8s-cls](https://github.com/ultralytics/assets/releases/download/v0.0.0/yolov8s-cls.pt) | 224 | 72.3 | 91.1 | 23.4 | 0.35 | 6.4 | 13.5 |
|
194 |
+
| [YOLOv8m-cls](https://github.com/ultralytics/assets/releases/download/v0.0.0/yolov8m-cls.pt) | 224 | 76.4 | 93.2 | 85.4 | 0.62 | 17.0 | 42.7 |
|
195 |
+
| [YOLOv8l-cls](https://github.com/ultralytics/assets/releases/download/v0.0.0/yolov8l-cls.pt) | 224 | 78.0 | 94.1 | 163.0 | 0.87 | 37.5 | 99.7 |
|
196 |
+
| [YOLOv8x-cls](https://github.com/ultralytics/assets/releases/download/v0.0.0/yolov8x-cls.pt) | 224 | 78.4 | 94.3 | 232.0 | 1.01 | 57.4 | 154.8 |
|
197 |
+
|
198 |
+
- **acc** 值是模型在 [ImageNet](https://www.image-net.org/) 数据集验证集上的准确率。
|
199 |
+
<br>通过 `yolo val classify data=path/to/ImageNet device=0` 复现
|
200 |
+
- **速度** 是使用 [Amazon EC2 P4d](https://aws.amazon.com/ec2/instance-types/p4/) 实例对 ImageNet val 图像进行平均计算的。
|
201 |
+
<br>通过 `yolo val classify data=path/to/ImageNet batch=1 device=0|cpu` 复现
|
202 |
+
|
203 |
+
</details>
|
204 |
+
|
205 |
+
## <div align="center">集成</div>
|
206 |
+
|
207 |
+
我们与领先的AI平台的关键整合扩展了Ultralytics产品的功能,增强了数据集标签化、训练、可视化和模型管理等任务。探索Ultralytics如何与[Roboflow](https://roboflow.com/?ref=ultralytics)、ClearML、[Comet](https://bit.ly/yolov8-readme-comet)、Neural Magic以及[OpenVINO](https://docs.ultralytics.com/integrations/openvino)合作,优化您的AI工作流程。
|
208 |
+
|
209 |
+
<br>
|
210 |
+
<a href="https://bit.ly/ultralytics_hub" target="_blank">
|
211 |
+
<img width="100%" src="https://github.com/ultralytics/assets/raw/main/yolov8/banner-integrations.png"></a>
|
212 |
+
<br>
|
213 |
+
<br>
|
214 |
+
|
215 |
+
<div align="center">
|
216 |
+
<a href="https://roboflow.com/?ref=ultralytics">
|
217 |
+
<img src="https://github.com/ultralytics/assets/raw/main/partners/logo-roboflow.png" width="10%" /></a>
|
218 |
+
<img src="https://github.com/ultralytics/assets/raw/main/social/logo-transparent.png" width="15%" height="0" alt="" />
|
219 |
+
<a href="https://cutt.ly/yolov5-readme-clearml">
|
220 |
+
<img src="https://github.com/ultralytics/assets/raw/main/partners/logo-clearml.png" width="10%" /></a>
|
221 |
+
<img src="https://github.com/ultralytics/assets/raw/main/social/logo-transparent.png" width="15%" height="0" alt="" />
|
222 |
+
<a href="https://bit.ly/yolov8-readme-comet">
|
223 |
+
<img src="https://github.com/ultralytics/assets/raw/main/partners/logo-comet.png" width="10%" /></a>
|
224 |
+
<img src="https://github.com/ultralytics/assets/raw/main/social/logo-transparent.png" width="15%" height="0" alt="" />
|
225 |
+
<a href="https://bit.ly/yolov5-neuralmagic">
|
226 |
+
<img src="https://github.com/ultralytics/assets/raw/main/partners/logo-neuralmagic.png" width="10%" /></a>
|
227 |
+
</div>
|
228 |
+
|
229 |
+
| Roboflow | ClearML ⭐ NEW | Comet ⭐ NEW | Neural Magic ⭐ NEW |
|
230 |
+
| :--------------------------------------------------------------------------------: | :----------------------------------------------------------------------------: | :----------------------------------------------------------------------------------: | :-----------------------------------------------------------------------------------: |
|
231 |
+
| 使用 [Roboflow](https://roboflow.com/?ref=ultralytics) 将您的自定义数据集直接标记并导出至 YOLOv8 进行训练 | 使用 [ClearML](https://cutt.ly/yolov5-readme-clearml)(开源!)自动跟踪、可视化,甚至远程训练 YOLOv8 | 免费且永久,[Comet](https://bit.ly/yolov8-readme-comet) 让您保存 YOLOv8 模型、恢复训练,并以交互式方式查看和调试预测 | 使用 [Neural Magic DeepSparse](https://bit.ly/yolov5-neuralmagic) 使 YOLOv8 推理速度提高多达 6 倍 |
|
232 |
+
|
233 |
+
## <div align="center">Ultralytics HUB</div>
|
234 |
+
|
235 |
+
体验 [Ultralytics HUB](https://bit.ly/ultralytics_hub) ⭐ 带来的无缝 AI,这是一个一体化解决方案,用于数据可视化、YOLOv5 和即将推出的 YOLOv8 🚀 模型训练和部署,无需任何编码。通过我们先进的平台和用户友好的 [Ultralytics 应用程序](https://ultralytics.com/app_install),轻松将图像转化为可操作的见解,并实现您的 AI 愿景。现在就开始您的**免费**之旅!
|
236 |
+
|
237 |
+
<a href="https://bit.ly/ultralytics_hub" target="_blank">
|
238 |
+
<img width="100%" src="https://github.com/ultralytics/assets/raw/main/im/ultralytics-hub.png" alt="Ultralytics HUB preview image"></a>
|
239 |
+
|
240 |
+
## <div align="center">贡献</div>
|
241 |
+
|
242 |
+
我们喜欢您的参与!没有社区的帮助,YOLOv5 和 YOLOv8 将无法实现。请参阅我们的[贡献指南](https://docs.ultralytics.com/help/contributing)以开始使用,并填写我们的[调查问卷](https://ultralytics.com/survey?utm_source=github&utm_medium=social&utm_campaign=Survey)向我们提供您的使用体验反馈。感谢所有贡献者的支持!🙏
|
243 |
+
|
244 |
+
<!-- SVG image from https://opencollective.com/ultralytics/contributors.svg?width=990 -->
|
245 |
+
|
246 |
+
<a href="https://github.com/ultralytics/yolov5/graphs/contributors">
|
247 |
+
<img width="100%" src="https://github.com/ultralytics/assets/raw/main/im/image-contributors.png"></a>
|
248 |
+
|
249 |
+
## <div align="center">许可证</div>
|
250 |
+
|
251 |
+
Ultralytics 提供两种许可证选项以适应各种使用场景:
|
252 |
+
|
253 |
+
- **AGPL-3.0 许可证**:这个[OSI 批准](https://opensource.org/licenses/)的开源许可证非常适合学生和爱好者,可以推动开放的协作和知识分享。请查看[LICENSE](https://github.com/ultralytics/ultralytics/blob/main/LICENSE) 文件以了解更多细节。
|
254 |
+
- **企业许可证**:专为商业用途设计,该许可证允许将 Ultralytics 的软件和 AI 模型无缝集成到商业产品和服务中,从而绕过 AGPL-3.0 的开源要求。��果您的场景涉及将我们的解决方案嵌入到商业产品中,请通过 [Ultralytics Licensing](https://ultralytics.com/license)与我们联系。
|
255 |
+
|
256 |
+
## <div align="center">联系方式</div>
|
257 |
+
|
258 |
+
对于 Ultralytics 的错误报告和功能请求,请访问 [GitHub Issues](https://github.com/ultralytics/ultralytics/issues),并加入我们的 [Discord](https://ultralytics.com/discord) 社区进行问题和讨论!
|
259 |
+
|
260 |
+
<br>
|
261 |
+
<div align="center">
|
262 |
+
<a href="https://github.com/ultralytics"><img src="https://github.com/ultralytics/assets/raw/main/social/logo-social-github.png" width="3%" alt="Ultralytics GitHub"></a>
|
263 |
+
<img src="https://github.com/ultralytics/assets/raw/main/social/logo-transparent.png" width="3%">
|
264 |
+
<a href="https://www.linkedin.com/company/ultralytics/"><img src="https://github.com/ultralytics/assets/raw/main/social/logo-social-linkedin.png" width="3%" alt="Ultralytics LinkedIn"></a>
|
265 |
+
<img src="https://github.com/ultralytics/assets/raw/main/social/logo-transparent.png" width="3%">
|
266 |
+
<a href="https://twitter.com/ultralytics"><img src="https://github.com/ultralytics/assets/raw/main/social/logo-social-twitter.png" width="3%" alt="Ultralytics Twitter"></a>
|
267 |
+
<img src="https://github.com/ultralytics/assets/raw/main/social/logo-transparent.png" width="3%">
|
268 |
+
<a href="https://youtube.com/ultralytics"><img src="https://github.com/ultralytics/assets/raw/main/social/logo-social-youtube.png" width="3%" alt="Ultralytics YouTube"></a>
|
269 |
+
<img src="https://github.com/ultralytics/assets/raw/main/social/logo-transparent.png" width="3%">
|
270 |
+
<a href="https://www.tiktok.com/@ultralytics"><img src="https://github.com/ultralytics/assets/raw/main/social/logo-social-tiktok.png" width="3%" alt="Ultralytics TikTok"></a>
|
271 |
+
<img src="https://github.com/ultralytics/assets/raw/main/social/logo-transparent.png" width="3%">
|
272 |
+
<a href="https://www.instagram.com/ultralytics/"><img src="https://github.com/ultralytics/assets/raw/main/social/logo-social-instagram.png" width="3%" alt="Ultralytics Instagram"></a>
|
273 |
+
<img src="https://github.com/ultralytics/assets/raw/main/social/logo-transparent.png" width="3%">
|
274 |
+
<a href="https://ultralytics.com/discord"><img src="https://github.com/ultralytics/assets/raw/main/social/logo-social-discord.png" width="3%" alt="Ultralytics Discord"></a>
|
275 |
+
</div>
|
ultralytics/docker/Dockerfile
ADDED
@@ -0,0 +1,82 @@
|
|
|
|
|
|
|
|
|
|
|
|
|
|
|
|
|
|
|
|
|
|
|
|
|
|
|
|
|
|
|
|
|
|
|
|
|
|
|
|
|
|
|
|
|
|
|
|
|
|
|
|
|
|
|
|
|
|
|
|
|
|
|
|
|
|
|
|
|
|
|
|
|
|
|
|
|
|
|
|
|
|
|
|
|
|
|
|
|
|
|
|
|
|
|
|
|
|
|
|
|
|
|
|
|
|
|
|
|
|
|
|
|
|
|
|
|
|
|
|
|
|
|
|
|
|
|
|
|
|
|
|
|
|
|
|
|
|
|
|
|
|
|
|
|
|
|
|
|
|
|
|
|
|
|
|
|
|
|
|
|
|
|
|
|
|
|
1 |
+
# Ultralytics YOLO 🚀, AGPL-3.0 license
|
2 |
+
# Builds ultralytics/ultralytics:latest image on DockerHub https://hub.docker.com/r/ultralytics/ultralytics
|
3 |
+
# Image is CUDA-optimized for YOLOv8 single/multi-GPU training and inference
|
4 |
+
|
5 |
+
# Start FROM PyTorch image https://hub.docker.com/r/pytorch/pytorch or nvcr.io/nvidia/pytorch:23.03-py3
|
6 |
+
FROM pytorch/pytorch:2.1.0-cuda12.1-cudnn8-runtime
|
7 |
+
RUN pip install --no-cache nvidia-tensorrt --index-url https://pypi.ngc.nvidia.com
|
8 |
+
|
9 |
+
# Downloads to user config dir
|
10 |
+
ADD https://ultralytics.com/assets/Arial.ttf https://ultralytics.com/assets/Arial.Unicode.ttf /root/.config/Ultralytics/
|
11 |
+
|
12 |
+
# Install linux packages
|
13 |
+
# g++ required to build 'tflite_support' and 'lap' packages, libusb-1.0-0 required for 'tflite_support' package
|
14 |
+
RUN apt update \
|
15 |
+
&& apt install --no-install-recommends -y gcc git zip curl htop libgl1 libglib2.0-0 libpython3-dev gnupg g++ libusb-1.0-0
|
16 |
+
|
17 |
+
# Security updates
|
18 |
+
# https://security.snyk.io/vuln/SNYK-UBUNTU1804-OPENSSL-3314796
|
19 |
+
RUN apt upgrade --no-install-recommends -y openssl tar
|
20 |
+
|
21 |
+
# Create working directory
|
22 |
+
WORKDIR /usr/src/ultralytics
|
23 |
+
|
24 |
+
# Copy contents
|
25 |
+
# COPY . /usr/src/ultralytics # git permission issues inside container
|
26 |
+
RUN git clone https://github.com/ultralytics/ultralytics -b main /usr/src/ultralytics
|
27 |
+
ADD https://github.com/ultralytics/assets/releases/download/v0.0.0/yolov8n.pt /usr/src/ultralytics/
|
28 |
+
|
29 |
+
# Install pip packages
|
30 |
+
RUN python3 -m pip install --upgrade pip wheel
|
31 |
+
RUN pip install --no-cache -e ".[export]" albumentations comet pycocotools pytest-cov
|
32 |
+
|
33 |
+
# Run exports to AutoInstall packages
|
34 |
+
RUN yolo export model=tmp/yolov8n.pt format=edgetpu imgsz=32
|
35 |
+
RUN yolo export model=tmp/yolov8n.pt format=ncnn imgsz=32
|
36 |
+
# Requires <= Python 3.10, bug with paddlepaddle==2.5.0 https://github.com/PaddlePaddle/X2Paddle/issues/991
|
37 |
+
RUN pip install --no-cache paddlepaddle==2.4.2 x2paddle
|
38 |
+
# Fix error: `np.bool` was a deprecated alias for the builtin `bool` segmentation error in Tests
|
39 |
+
RUN pip install --no-cache numpy==1.23.5
|
40 |
+
# Remove exported models
|
41 |
+
RUN rm -rf tmp
|
42 |
+
|
43 |
+
# Set environment variables
|
44 |
+
ENV OMP_NUM_THREADS=1
|
45 |
+
# Avoid DDP error "MKL_THREADING_LAYER=INTEL is incompatible with libgomp.so.1 library" https://github.com/pytorch/pytorch/issues/37377
|
46 |
+
ENV MKL_THREADING_LAYER=GNU
|
47 |
+
|
48 |
+
|
49 |
+
# Usage Examples -------------------------------------------------------------------------------------------------------
|
50 |
+
|
51 |
+
# Build and Push
|
52 |
+
# t=ultralytics/ultralytics:latest && sudo docker build -f docker/Dockerfile -t $t . && sudo docker push $t
|
53 |
+
|
54 |
+
# Pull and Run with access to all GPUs
|
55 |
+
# t=ultralytics/ultralytics:latest && sudo docker pull $t && sudo docker run -it --ipc=host --gpus all $t
|
56 |
+
|
57 |
+
# Pull and Run with access to GPUs 2 and 3 (inside container CUDA devices will appear as 0 and 1)
|
58 |
+
# t=ultralytics/ultralytics:latest && sudo docker pull $t && sudo docker run -it --ipc=host --gpus '"device=2,3"' $t
|
59 |
+
|
60 |
+
# Pull and Run with local directory access
|
61 |
+
# t=ultralytics/ultralytics:latest && sudo docker pull $t && sudo docker run -it --ipc=host --gpus all -v "$(pwd)"/datasets:/usr/src/datasets $t
|
62 |
+
|
63 |
+
# Kill all
|
64 |
+
# sudo docker kill $(sudo docker ps -q)
|
65 |
+
|
66 |
+
# Kill all image-based
|
67 |
+
# sudo docker kill $(sudo docker ps -qa --filter ancestor=ultralytics/ultralytics:latest)
|
68 |
+
|
69 |
+
# DockerHub tag update
|
70 |
+
# t=ultralytics/ultralytics:latest tnew=ultralytics/ultralytics:v6.2 && sudo docker pull $t && sudo docker tag $t $tnew && sudo docker push $tnew
|
71 |
+
|
72 |
+
# Clean up
|
73 |
+
# sudo docker system prune -a --volumes
|
74 |
+
|
75 |
+
# Update Ubuntu drivers
|
76 |
+
# https://www.maketecheasier.com/install-nvidia-drivers-ubuntu/
|
77 |
+
|
78 |
+
# DDP test
|
79 |
+
# python -m torch.distributed.run --nproc_per_node 2 --master_port 1 train.py --epochs 3
|
80 |
+
|
81 |
+
# GCP VM from Image
|
82 |
+
# docker.io/ultralytics/ultralytics:latest
|
ultralytics/docker/Dockerfile-arm64
ADDED
@@ -0,0 +1,44 @@
|
|
|
|
|
|
|
|
|
|
|
|
|
|
|
|
|
|
|
|
|
|
|
|
|
|
|
|
|
|
|
|
|
|
|
|
|
|
|
|
|
|
|
|
|
|
|
|
|
|
|
|
|
|
|
|
|
|
|
|
|
|
|
|
|
|
|
|
|
|
|
|
|
|
|
|
|
|
|
|
|
|
|
|
|
|
|
|
|
|
|
1 |
+
# Ultralytics YOLO 🚀, AGPL-3.0 license
|
2 |
+
# Builds ultralytics/ultralytics:latest-arm64 image on DockerHub https://hub.docker.com/r/ultralytics/ultralytics
|
3 |
+
# Image is aarch64-compatible for Apple M1 and other ARM architectures i.e. Jetson Nano and Raspberry Pi
|
4 |
+
|
5 |
+
# Start FROM Ubuntu image https://hub.docker.com/_/ubuntu
|
6 |
+
FROM arm64v8/ubuntu:22.04
|
7 |
+
|
8 |
+
# Downloads to user config dir
|
9 |
+
ADD https://ultralytics.com/assets/Arial.ttf https://ultralytics.com/assets/Arial.Unicode.ttf /root/.config/Ultralytics/
|
10 |
+
|
11 |
+
# Install linux packages
|
12 |
+
# g++ required to build 'tflite_support' and 'lap' packages, libusb-1.0-0 required for 'tflite_support' package
|
13 |
+
RUN apt update \
|
14 |
+
&& apt install --no-install-recommends -y python3-pip git zip curl htop gcc libgl1 libglib2.0-0 libpython3-dev gnupg g++ libusb-1.0-0
|
15 |
+
|
16 |
+
# Create working directory
|
17 |
+
WORKDIR /usr/src/ultralytics
|
18 |
+
|
19 |
+
# Copy contents
|
20 |
+
# COPY . /usr/src/ultralytics # git permission issues inside container
|
21 |
+
RUN git clone https://github.com/ultralytics/ultralytics -b main /usr/src/ultralytics
|
22 |
+
ADD https://github.com/ultralytics/assets/releases/download/v0.0.0/yolov8n.pt /usr/src/ultralytics/
|
23 |
+
|
24 |
+
# Install pip packages
|
25 |
+
RUN python3 -m pip install --upgrade pip wheel
|
26 |
+
RUN pip install --no-cache -e .
|
27 |
+
|
28 |
+
# Creates a symbolic link to make 'python' point to 'python3'
|
29 |
+
RUN ln -sf /usr/bin/python3 /usr/bin/python
|
30 |
+
|
31 |
+
|
32 |
+
# Usage Examples -------------------------------------------------------------------------------------------------------
|
33 |
+
|
34 |
+
# Build and Push
|
35 |
+
# t=ultralytics/ultralytics:latest-arm64 && sudo docker build --platform linux/arm64 -f docker/Dockerfile-arm64 -t $t . && sudo docker push $t
|
36 |
+
|
37 |
+
# Run
|
38 |
+
# t=ultralytics/ultralytics:latest-arm64 && sudo docker run -it --ipc=host $t
|
39 |
+
|
40 |
+
# Pull and Run
|
41 |
+
# t=ultralytics/ultralytics:latest-arm64 && sudo docker pull $t && sudo docker run -it --ipc=host $t
|
42 |
+
|
43 |
+
# Pull and Run with local volume mounted
|
44 |
+
# t=ultralytics/ultralytics:latest-arm64 && sudo docker pull $t && sudo docker run -it --ipc=host -v "$(pwd)"/datasets:/usr/src/datasets $t
|
ultralytics/docker/Dockerfile-conda
ADDED
@@ -0,0 +1,38 @@
|
|
|
|
|
|
|
|
|
|
|
|
|
|
|
|
|
|
|
|
|
|
|
|
|
|
|
|
|
|
|
|
|
|
|
|
|
|
|
|
|
|
|
|
|
|
|
|
|
|
|
|
|
|
|
|
|
|
|
|
|
|
|
|
|
|
|
|
|
|
|
|
|
|
|
|
|
|
|
1 |
+
# Ultralytics YOLO 🚀, AGPL-3.0 license
|
2 |
+
# Builds ultralytics/ultralytics:latest-conda image on DockerHub https://hub.docker.com/r/ultralytics/ultralytics
|
3 |
+
# Image is optimized for Ultralytics Anaconda (https://anaconda.org/conda-forge/ultralytics) installation and usage
|
4 |
+
|
5 |
+
# Start FROM miniconda3 image https://hub.docker.com/r/continuumio/miniconda3
|
6 |
+
FROM continuumio/miniconda3:latest
|
7 |
+
|
8 |
+
# Downloads to user config dir
|
9 |
+
ADD https://ultralytics.com/assets/Arial.ttf https://ultralytics.com/assets/Arial.Unicode.ttf /root/.config/Ultralytics/
|
10 |
+
|
11 |
+
# Install linux packages
|
12 |
+
RUN apt update \
|
13 |
+
&& apt install --no-install-recommends -y libgl1
|
14 |
+
|
15 |
+
# Copy contents
|
16 |
+
ADD https://github.com/ultralytics/assets/releases/download/v0.0.0/yolov8n.pt .
|
17 |
+
|
18 |
+
# Install conda packages
|
19 |
+
# mkl required to fix 'OSError: libmkl_intel_lp64.so.2: cannot open shared object file: No such file or directory'
|
20 |
+
RUN conda config --set solver libmamba && \
|
21 |
+
conda install pytorch torchvision pytorch-cuda=11.8 -c pytorch -c nvidia && \
|
22 |
+
conda install -c conda-forge ultralytics mkl
|
23 |
+
# conda install -c pytorch -c nvidia -c conda-forge pytorch torchvision pytorch-cuda=11.8 ultralytics mkl
|
24 |
+
|
25 |
+
|
26 |
+
# Usage Examples -------------------------------------------------------------------------------------------------------
|
27 |
+
|
28 |
+
# Build and Push
|
29 |
+
# t=ultralytics/ultralytics:latest-conda && sudo docker build -f docker/Dockerfile-cpu -t $t . && sudo docker push $t
|
30 |
+
|
31 |
+
# Run
|
32 |
+
# t=ultralytics/ultralytics:latest-conda && sudo docker run -it --ipc=host $t
|
33 |
+
|
34 |
+
# Pull and Run
|
35 |
+
# t=ultralytics/ultralytics:latest-conda && sudo docker pull $t && sudo docker run -it --ipc=host $t
|
36 |
+
|
37 |
+
# Pull and Run with local volume mounted
|
38 |
+
# t=ultralytics/ultralytics:latest-conda && sudo docker pull $t && sudo docker run -it --ipc=host -v "$(pwd)"/datasets:/usr/src/datasets $t
|
ultralytics/docker/Dockerfile-cpu
ADDED
@@ -0,0 +1,55 @@
|
|
|
|
|
|
|
|
|
|
|
|
|
|
|
|
|
|
|
|
|
|
|
|
|
|
|
|
|
|
|
|
|
|
|
|
|
|
|
|
|
|
|
|
|
|
|
|
|
|
|
|
|
|
|
|
|
|
|
|
|
|
|
|
|
|
|
|
|
|
|
|
|
|
|
|
|
|
|
|
|
|
|
|
|
|
|
|
|
|
|
|
|
|
|
|
|
|
|
|
|
|
|
|
|
|
|
|
|
|
|
|
|
1 |
+
# Ultralytics YOLO 🚀, AGPL-3.0 license
|
2 |
+
# Builds ultralytics/ultralytics:latest-cpu image on DockerHub https://hub.docker.com/r/ultralytics/ultralytics
|
3 |
+
# Image is CPU-optimized for ONNX, OpenVINO and PyTorch YOLOv8 deployments
|
4 |
+
|
5 |
+
# Start FROM Ubuntu image https://hub.docker.com/_/ubuntu
|
6 |
+
FROM ubuntu:mantic-20231011
|
7 |
+
|
8 |
+
# Downloads to user config dir
|
9 |
+
ADD https://ultralytics.com/assets/Arial.ttf https://ultralytics.com/assets/Arial.Unicode.ttf /root/.config/Ultralytics/
|
10 |
+
|
11 |
+
# Install linux packages
|
12 |
+
# g++ required to build 'tflite_support' and 'lap' packages, libusb-1.0-0 required for 'tflite_support' package
|
13 |
+
RUN apt update \
|
14 |
+
&& apt install --no-install-recommends -y python3-pip git zip curl htop libgl1 libglib2.0-0 libpython3-dev gnupg g++ libusb-1.0-0
|
15 |
+
|
16 |
+
# Create working directory
|
17 |
+
WORKDIR /usr/src/ultralytics
|
18 |
+
|
19 |
+
# Copy contents
|
20 |
+
# COPY . /usr/src/ultralytics # git permission issues inside container
|
21 |
+
RUN git clone https://github.com/ultralytics/ultralytics -b main /usr/src/ultralytics
|
22 |
+
ADD https://github.com/ultralytics/assets/releases/download/v0.0.0/yolov8n.pt /usr/src/ultralytics/
|
23 |
+
|
24 |
+
# Remove python3.11/EXTERNALLY-MANAGED or use 'pip install --break-system-packages' avoid 'externally-managed-environment' Ubuntu nightly error
|
25 |
+
RUN rm -rf /usr/lib/python3.11/EXTERNALLY-MANAGED
|
26 |
+
|
27 |
+
# Install pip packages
|
28 |
+
RUN python3 -m pip install --upgrade pip wheel
|
29 |
+
RUN pip install --no-cache -e ".[export]" --extra-index-url https://download.pytorch.org/whl/cpu
|
30 |
+
|
31 |
+
# Run exports to AutoInstall packages
|
32 |
+
RUN yolo export model=tmp/yolov8n.pt format=edgetpu imgsz=32
|
33 |
+
RUN yolo export model=tmp/yolov8n.pt format=ncnn imgsz=32
|
34 |
+
# Requires <= Python 3.10, bug with paddlepaddle==2.5.0 https://github.com/PaddlePaddle/X2Paddle/issues/991
|
35 |
+
# RUN pip install --no-cache paddlepaddle==2.4.2 x2paddle
|
36 |
+
# Remove exported models
|
37 |
+
RUN rm -rf tmp
|
38 |
+
|
39 |
+
# Creates a symbolic link to make 'python' point to 'python3'
|
40 |
+
RUN ln -sf /usr/bin/python3 /usr/bin/python
|
41 |
+
|
42 |
+
|
43 |
+
# Usage Examples -------------------------------------------------------------------------------------------------------
|
44 |
+
|
45 |
+
# Build and Push
|
46 |
+
# t=ultralytics/ultralytics:latest-cpu && sudo docker build -f docker/Dockerfile-cpu -t $t . && sudo docker push $t
|
47 |
+
|
48 |
+
# Run
|
49 |
+
# t=ultralytics/ultralytics:latest-cpu && sudo docker run -it --ipc=host $t
|
50 |
+
|
51 |
+
# Pull and Run
|
52 |
+
# t=ultralytics/ultralytics:latest-cpu && sudo docker pull $t && sudo docker run -it --ipc=host $t
|
53 |
+
|
54 |
+
# Pull and Run with local volume mounted
|
55 |
+
# t=ultralytics/ultralytics:latest-cpu && sudo docker pull $t && sudo docker run -it --ipc=host -v "$(pwd)"/datasets:/usr/src/datasets $t
|
ultralytics/docker/Dockerfile-jetson
ADDED
@@ -0,0 +1,48 @@
|
|
|
|
|
|
|
|
|
|
|
|
|
|
|
|
|
|
|
|
|
|
|
|
|
|
|
|
|
|
|
|
|
|
|
|
|
|
|
|
|
|
|
|
|
|
|
|
|
|
|
|
|
|
|
|
|
|
|
|
|
|
|
|
|
|
|
|
|
|
|
|
|
|
|
|
|
|
|
|
|
|
|
|
|
|
|
|
|
|
|
|
|
|
|
|
|
|
|
1 |
+
# Ultralytics YOLO 🚀, AGPL-3.0 license
|
2 |
+
# Builds ultralytics/ultralytics:jetson image on DockerHub https://hub.docker.com/r/ultralytics/ultralytics
|
3 |
+
# Supports JetPack for YOLOv8 on Jetson Nano, TX1/TX2, Xavier NX, AGX Xavier, AGX Orin, and Orin NX
|
4 |
+
|
5 |
+
# Start FROM https://catalog.ngc.nvidia.com/orgs/nvidia/containers/l4t-pytorch
|
6 |
+
FROM nvcr.io/nvidia/l4t-pytorch:r35.2.1-pth2.0-py3
|
7 |
+
|
8 |
+
# Downloads to user config dir
|
9 |
+
ADD https://ultralytics.com/assets/Arial.ttf https://ultralytics.com/assets/Arial.Unicode.ttf /root/.config/Ultralytics/
|
10 |
+
|
11 |
+
# Install linux packages
|
12 |
+
# g++ required to build 'tflite_support' and 'lap' packages, libusb-1.0-0 required for 'tflite_support' package
|
13 |
+
RUN apt update \
|
14 |
+
&& apt install --no-install-recommends -y gcc git zip curl htop libgl1 libglib2.0-0 libpython3-dev gnupg g++ libusb-1.0-0
|
15 |
+
|
16 |
+
# Create working directory
|
17 |
+
WORKDIR /usr/src/ultralytics
|
18 |
+
|
19 |
+
# Copy contents
|
20 |
+
# COPY . /usr/src/ultralytics # git permission issues inside container
|
21 |
+
RUN git clone https://github.com/ultralytics/ultralytics -b main /usr/src/ultralytics
|
22 |
+
ADD https://github.com/ultralytics/assets/releases/download/v0.0.0/yolov8n.pt /usr/src/ultralytics/
|
23 |
+
|
24 |
+
# Remove opencv-python from requirements.txt as it conflicts with opencv-python installed in base image
|
25 |
+
RUN grep -v '^opencv-python' requirements.txt > tmp.txt && mv tmp.txt requirements.txt
|
26 |
+
|
27 |
+
# Install pip packages manually for TensorRT compatibility https://github.com/NVIDIA/TensorRT/issues/2567
|
28 |
+
RUN python3 -m pip install --upgrade pip wheel
|
29 |
+
RUN pip install --no-cache tqdm matplotlib pyyaml psutil pandas onnx "numpy==1.23"
|
30 |
+
RUN pip install --no-cache -e .
|
31 |
+
|
32 |
+
# Set environment variables
|
33 |
+
ENV OMP_NUM_THREADS=1
|
34 |
+
|
35 |
+
|
36 |
+
# Usage Examples -------------------------------------------------------------------------------------------------------
|
37 |
+
|
38 |
+
# Build and Push
|
39 |
+
# t=ultralytics/ultralytics:latest-jetson && sudo docker build --platform linux/arm64 -f docker/Dockerfile-jetson -t $t . && sudo docker push $t
|
40 |
+
|
41 |
+
# Run
|
42 |
+
# t=ultralytics/ultralytics:latest-jetson && sudo docker run -it --ipc=host $t
|
43 |
+
|
44 |
+
# Pull and Run
|
45 |
+
# t=ultralytics/ultralytics:latest-jetson && sudo docker pull $t && sudo docker run -it --ipc=host $t
|
46 |
+
|
47 |
+
# Pull and Run with NVIDIA runtime
|
48 |
+
# t=ultralytics/ultralytics:latest-jetson && sudo docker pull $t && sudo docker run -it --ipc=host --runtime=nvidia $t
|
ultralytics/docker/Dockerfile-python
ADDED
@@ -0,0 +1,52 @@
|
|
|
|
|
|
|
|
|
|
|
|
|
|
|
|
|
|
|
|
|
|
|
|
|
|
|
|
|
|
|
|
|
|
|
|
|
|
|
|
|
|
|
|
|
|
|
|
|
|
|
|
|
|
|
|
|
|
|
|
|
|
|
|
|
|
|
|
|
|
|
|
|
|
|
|
|
|
|
|
|
|
|
|
|
|
|
|
|
|
|
|
|
|
|
|
|
|
|
|
|
|
|
|
|
|
|
1 |
+
# Ultralytics YOLO 🚀, AGPL-3.0 license
|
2 |
+
# Builds ultralytics/ultralytics:latest-cpu image on DockerHub https://hub.docker.com/r/ultralytics/ultralytics
|
3 |
+
# Image is CPU-optimized for ONNX, OpenVINO and PyTorch YOLOv8 deployments
|
4 |
+
|
5 |
+
# Use the official Python 3.10 slim-bookworm as base image
|
6 |
+
FROM python:3.10-slim-bookworm
|
7 |
+
|
8 |
+
# Downloads to user config dir
|
9 |
+
ADD https://ultralytics.com/assets/Arial.ttf https://ultralytics.com/assets/Arial.Unicode.ttf /root/.config/Ultralytics/
|
10 |
+
|
11 |
+
# Install linux packages
|
12 |
+
# g++ required to build 'tflite_support' and 'lap' packages, libusb-1.0-0 required for 'tflite_support' package
|
13 |
+
RUN apt update \
|
14 |
+
&& apt install --no-install-recommends -y python3-pip git zip curl htop libgl1 libglib2.0-0 libpython3-dev gnupg g++ libusb-1.0-0
|
15 |
+
|
16 |
+
# Create working directory
|
17 |
+
WORKDIR /usr/src/ultralytics
|
18 |
+
|
19 |
+
# Copy contents
|
20 |
+
# COPY . /usr/src/ultralytics # git permission issues inside container
|
21 |
+
RUN git clone https://github.com/ultralytics/ultralytics -b main /usr/src/ultralytics
|
22 |
+
ADD https://github.com/ultralytics/assets/releases/download/v0.0.0/yolov8n.pt /usr/src/ultralytics/
|
23 |
+
|
24 |
+
# Remove python3.11/EXTERNALLY-MANAGED or use 'pip install --break-system-packages' avoid 'externally-managed-environment' Ubuntu nightly error
|
25 |
+
# RUN rm -rf /usr/lib/python3.11/EXTERNALLY-MANAGED
|
26 |
+
|
27 |
+
# Install pip packages
|
28 |
+
RUN python3 -m pip install --upgrade pip wheel
|
29 |
+
RUN pip install --no-cache -e ".[export]" --extra-index-url https://download.pytorch.org/whl/cpu
|
30 |
+
|
31 |
+
# Run exports to AutoInstall packages
|
32 |
+
RUN yolo export model=tmp/yolov8n.pt format=edgetpu imgsz=32
|
33 |
+
RUN yolo export model=tmp/yolov8n.pt format=ncnn imgsz=32
|
34 |
+
# Requires <= Python 3.10, bug with paddlepaddle==2.5.0 https://github.com/PaddlePaddle/X2Paddle/issues/991
|
35 |
+
RUN pip install --no-cache paddlepaddle==2.4.2 x2paddle
|
36 |
+
# Remove exported models
|
37 |
+
RUN rm -rf tmp
|
38 |
+
|
39 |
+
|
40 |
+
# Usage Examples -------------------------------------------------------------------------------------------------------
|
41 |
+
|
42 |
+
# Build and Push
|
43 |
+
# t=ultralytics/ultralytics:latest-python && sudo docker build -f docker/Dockerfile-python -t $t . && sudo docker push $t
|
44 |
+
|
45 |
+
# Run
|
46 |
+
# t=ultralytics/ultralytics:latest-python && sudo docker run -it --ipc=host $t
|
47 |
+
|
48 |
+
# Pull and Run
|
49 |
+
# t=ultralytics/ultralytics:latest-python && sudo docker pull $t && sudo docker run -it --ipc=host $t
|
50 |
+
|
51 |
+
# Pull and Run with local volume mounted
|
52 |
+
# t=ultralytics/ultralytics:latest-python && sudo docker pull $t && sudo docker run -it --ipc=host -v "$(pwd)"/datasets:/usr/src/datasets $t
|
ultralytics/docker/Dockerfile-runner
ADDED
@@ -0,0 +1,38 @@
|
|
|
|
|
|
|
|
|
|
|
|
|
|
|
|
|
|
|
|
|
|
|
|
|
|
|
|
|
|
|
|
|
|
|
|
|
|
|
|
|
|
|
|
|
|
|
|
|
|
|
|
|
|
|
|
|
|
|
|
|
|
|
|
|
|
|
|
|
|
|
|
|
|
|
|
|
|
|
1 |
+
# Ultralytics YOLO 🚀, AGPL-3.0 license
|
2 |
+
# Builds GitHub actions CI runner image for deployment to DockerHub https://hub.docker.com/r/ultralytics/ultralytics
|
3 |
+
# Image is CUDA-optimized for YOLOv8 single/multi-GPU training and inference tests
|
4 |
+
|
5 |
+
# Start FROM Ultralytics GPU image
|
6 |
+
FROM ultralytics/ultralytics:latest
|
7 |
+
|
8 |
+
# Set the working directory
|
9 |
+
WORKDIR /actions-runner
|
10 |
+
|
11 |
+
# Download and unpack the latest runner from https://github.com/actions/runner
|
12 |
+
RUN FILENAME=actions-runner-linux-x64-2.309.0.tar.gz && \
|
13 |
+
curl -o $FILENAME -L https://github.com/actions/runner/releases/download/v2.309.0/$FILENAME && \
|
14 |
+
tar xzf $FILENAME && \
|
15 |
+
rm $FILENAME
|
16 |
+
|
17 |
+
# Install runner dependencies
|
18 |
+
ENV RUNNER_ALLOW_RUNASROOT=1
|
19 |
+
ENV DEBIAN_FRONTEND=noninteractive
|
20 |
+
RUN ./bin/installdependencies.sh && \
|
21 |
+
apt-get -y install libicu-dev
|
22 |
+
|
23 |
+
# Inline ENTRYPOINT command to configure and start runner with default TOKEN and NAME
|
24 |
+
ENTRYPOINT sh -c './config.sh --url https://github.com/ultralytics/ultralytics \
|
25 |
+
--token ${GITHUB_RUNNER_TOKEN:-TOKEN} \
|
26 |
+
--name ${GITHUB_RUNNER_NAME:-NAME} \
|
27 |
+
--labels gpu-latest \
|
28 |
+
--replace && \
|
29 |
+
./run.sh'
|
30 |
+
|
31 |
+
|
32 |
+
# Usage Examples -------------------------------------------------------------------------------------------------------
|
33 |
+
|
34 |
+
# Build and Push
|
35 |
+
# t=ultralytics/ultralytics:latest-runner && sudo docker build -f docker/Dockerfile-runner -t $t . && sudo docker push $t
|
36 |
+
|
37 |
+
# Pull and Run in detached mode with access to GPUs 0 and 1
|
38 |
+
# t=ultralytics/ultralytics:latest-runner && sudo docker run -d -e GITHUB_RUNNER_TOKEN=TOKEN -e GITHUB_RUNNER_NAME=NAME --ipc=host --gpus '"device=0,1"' $t
|
ultralytics/docs/CNAME
ADDED
@@ -0,0 +1 @@
|
|
|
|
|
1 |
+
docs.ultralytics.com
|
ultralytics/docs/README.md
ADDED
@@ -0,0 +1,73 @@
|
|
|
|
|
|
|
|
|
|
|
|
|
|
|
|
|
|
|
|
|
|
|
|
|
|
|
|
|
|
|
|
|
|
|
|
|
|
|
|
|
|
|
|
|
|
|
|
|
|
|
|
|
|
|
|
|
|
|
|
|
|
|
|
|
|
|
|
|
|
|
|
|
|
|
|
|
|
|
|
|
|
|
|
|
|
|
|
|
|
|
|
|
|
|
|
|
|
|
|
|
|
|
|
|
|
|
|
|
|
|
|
|
|
|
|
|
|
|
|
|
|
|
|
|
|
|
|
|
|
|
|
|
|
|
|
|
|
|
|
|
|
|
|
|
|
|
|
|
1 |
+
# Ultralytics Docs
|
2 |
+
|
3 |
+
Ultralytics Docs are deployed to [https://docs.ultralytics.com](https://docs.ultralytics.com).
|
4 |
+
|
5 |
+
[](https://github.com/ultralytics/docs/actions/workflows/pages/pages-build-deployment) [](https://github.com/ultralytics/docs/actions/workflows/links.yml)
|
6 |
+
|
7 |
+
### Install Ultralytics package
|
8 |
+
|
9 |
+
[](https://badge.fury.io/py/ultralytics) [](https://pepy.tech/project/ultralytics)
|
10 |
+
|
11 |
+
To install the ultralytics package in developer mode, you will need to have Git and Python 3 installed on your system. Then, follow these steps:
|
12 |
+
|
13 |
+
1. Clone the ultralytics repository to your local machine using Git:
|
14 |
+
|
15 |
+
```bash
|
16 |
+
git clone https://github.com/ultralytics/ultralytics.git
|
17 |
+
```
|
18 |
+
|
19 |
+
2. Navigate to the root directory of the repository:
|
20 |
+
|
21 |
+
```bash
|
22 |
+
cd ultralytics
|
23 |
+
```
|
24 |
+
|
25 |
+
3. Install the package in developer mode using pip:
|
26 |
+
|
27 |
+
```bash
|
28 |
+
pip install -e '.[dev]'
|
29 |
+
```
|
30 |
+
|
31 |
+
This will install the ultralytics package and its dependencies in developer mode, allowing you to make changes to the package code and have them reflected immediately in your Python environment.
|
32 |
+
|
33 |
+
Note that you may need to use the pip3 command instead of pip if you have multiple versions of Python installed on your system.
|
34 |
+
|
35 |
+
### Building and Serving Locally
|
36 |
+
|
37 |
+
The `mkdocs serve` command is used to build and serve a local version of the MkDocs documentation site. It is typically used during the development and testing phase of a documentation project.
|
38 |
+
|
39 |
+
```bash
|
40 |
+
mkdocs serve
|
41 |
+
```
|
42 |
+
|
43 |
+
Here is a breakdown of what this command does:
|
44 |
+
|
45 |
+
- `mkdocs`: This is the command-line interface (CLI) for the MkDocs static site generator. It is used to build and serve MkDocs sites.
|
46 |
+
- `serve`: This is a subcommand of the `mkdocs` CLI that tells it to build and serve the documentation site locally.
|
47 |
+
- `-a`: This flag specifies the hostname and port number to bind the server to. The default value is `localhost:8000`.
|
48 |
+
- `-t`: This flag specifies the theme to use for the documentation site. The default value is `mkdocs`.
|
49 |
+
- `-s`: This flag tells the `serve` command to serve the site in silent mode, which means it will not display any log messages or progress updates. When you run the `mkdocs serve` command, it will build the documentation site using the files in the `docs/` directory and serve it at the specified hostname and port number. You can then view the site by going to the URL in your web browser.
|
50 |
+
|
51 |
+
While the site is being served, you can make changes to the documentation files and see them reflected in the live site immediately. This is useful for testing and debugging your documentation before deploying it to a live server.
|
52 |
+
|
53 |
+
To stop the serve command and terminate the local server, you can use the `CTRL+C` keyboard shortcut.
|
54 |
+
|
55 |
+
### Deploying Your Documentation Site
|
56 |
+
|
57 |
+
To deploy your MkDocs documentation site, you will need to choose a hosting provider and a deployment method. Some popular options include GitHub Pages, GitLab Pages, and Amazon S3.
|
58 |
+
|
59 |
+
Before you can deploy your site, you will need to configure your `mkdocs.yml` file to specify the remote host and any other necessary deployment settings.
|
60 |
+
|
61 |
+
Once you have configured your `mkdocs.yml` file, you can use the `mkdocs deploy` command to build and deploy your site. This command will build the documentation site using the files in the `docs/` directory and the specified configuration file and theme, and then deploy the site to the specified remote host.
|
62 |
+
|
63 |
+
For example, to deploy your site to GitHub Pages using the gh-deploy plugin, you can use the following command:
|
64 |
+
|
65 |
+
```bash
|
66 |
+
mkdocs gh-deploy
|
67 |
+
```
|
68 |
+
|
69 |
+
If you are using GitHub Pages, you can set a custom domain for your documentation site by going to the "Settings" page for your repository and updating the "Custom domain" field in the "GitHub Pages" section.
|
70 |
+
|
71 |
+
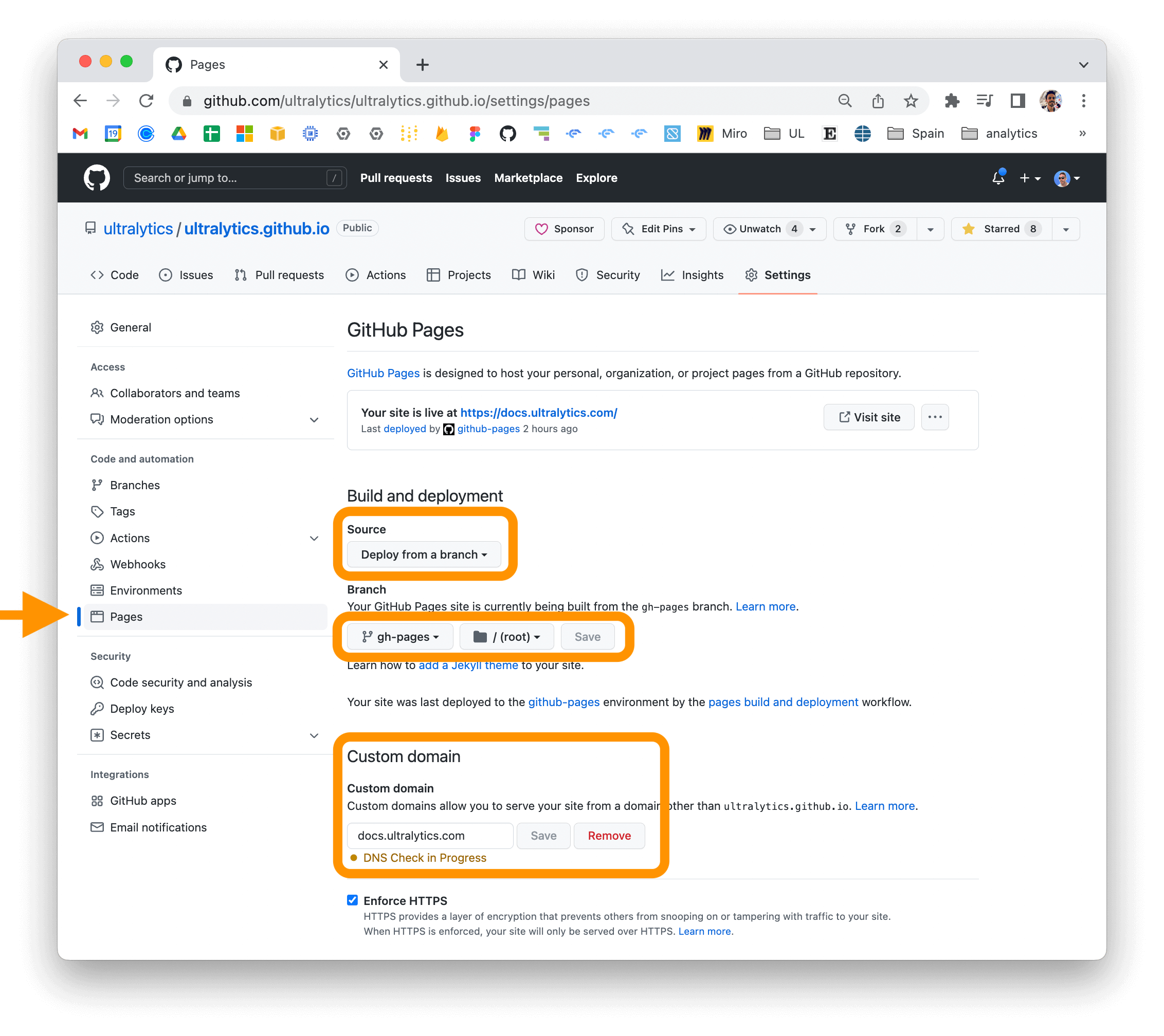
|
72 |
+
|
73 |
+
For more information on deploying your MkDocs documentation site, see the [MkDocs documentation](https://www.mkdocs.org/user-guide/deploying-your-docs/).
|
ultralytics/docs/SECURITY.md
ADDED
@@ -0,0 +1,26 @@
|
|
|
|
|
|
|
|
|
|
|
|
|
|
|
|
|
|
|
|
|
|
|
|
|
|
|
|
|
|
|
|
|
|
|
|
|
|
|
|
|
|
|
|
|
|
|
|
|
|
|
|
|
|
|
1 |
+
---
|
2 |
+
description: Discover how Ultralytics ensures the safety of user data and systems. Check out the measures we have implemented, including Snyk and GitHub CodeQL Scanning.
|
3 |
+
keywords: Ultralytics, Security Policy, data security, open-source projects, Snyk scanning, CodeQL scanning, vulnerability detection, threat prevention
|
4 |
+
---
|
5 |
+
|
6 |
+
# Security Policy
|
7 |
+
|
8 |
+
At [Ultralytics](https://ultralytics.com), the security of our users' data and systems is of utmost importance. To ensure the safety and security of our [open-source projects](https://github.com/ultralytics), we have implemented several measures to detect and prevent security vulnerabilities.
|
9 |
+
|
10 |
+
## Snyk Scanning
|
11 |
+
|
12 |
+
We use [Snyk](https://snyk.io/advisor/python/ultralytics) to regularly scan all Ultralytics repositories for vulnerabilities and security issues. Our goal is to identify and remediate any potential threats as soon as possible, to minimize any risks to our users.
|
13 |
+
|
14 |
+
[](https://snyk.io/advisor/python/ultralytics)
|
15 |
+
|
16 |
+
## GitHub CodeQL Scanning
|
17 |
+
|
18 |
+
In addition to our Snyk scans, we also use GitHub's [CodeQL](https://docs.github.com/en/code-security/code-scanning/automatically-scanning-your-code-for-vulnerabilities-and-errors/about-code-scanning-with-codeql) scans to proactively identify and address security vulnerabilities across all Ultralytics repositories.
|
19 |
+
|
20 |
+
[](https://github.com/ultralytics/ultralytics/actions/workflows/codeql.yaml)
|
21 |
+
|
22 |
+
## Reporting Security Issues
|
23 |
+
|
24 |
+
If you suspect or discover a security vulnerability in any of our repositories, please let us know immediately. You can reach out to us directly via our [contact form](https://ultralytics.com/contact) or via [security@ultralytics.com](mailto:security@ultralytics.com). Our security team will investigate and respond as soon as possible.
|
25 |
+
|
26 |
+
We appreciate your help in keeping all Ultralytics open-source projects secure and safe for everyone.
|
ultralytics/docs/assets/favicon.ico
ADDED
|
ultralytics/docs/build_reference.py
ADDED
@@ -0,0 +1,127 @@
|
|
|
|
|
|
|
|
|
|
|
|
|
|
|
|
|
|
|
|
|
|
|
|
|
|
|
|
|
|
|
|
|
|
|
|
|
|
|
|
|
|
|
|
|
|
|
|
|
|
|
|
|
|
|
|
|
|
|
|
|
|
|
|
|
|
|
|
|
|
|
|
|
|
|
|
|
|
|
|
|
|
|
|
|
|
|
|
|
|
|
|
|
|
|
|
|
|
|
|
|
|
|
|
|
|
|
|
|
|
|
|
|
|
|
|
|
|
|
|
|
|
|
|
|
|
|
|
|
|
|
|
|
|
|
|
|
|
|
|
|
|
|
|
|
|
|
|
|
|
|
|
|
|
|
|
|
|
|
|
|
|
|
|
|
|
|
|
|
|
|
|
|
|
|
|
|
|
|
|
|
|
|
|
|
|
|
|
|
|
|
|
|
|
|
|
|
|
|
|
|
|
|
|
|
|
|
|
|
|
|
|
|
|
|
|
|
|
|
|
|
|
|
|
|
|
|
|
|
|
|
|
|
|
|
|
|
|
|
|
|
|
|
|
|
|
|
|
|
|
|
|
|
|
|
|
|
1 |
+
# Ultralytics YOLO 🚀, AGPL-3.0 license
|
2 |
+
"""
|
3 |
+
Helper file to build Ultralytics Docs reference section. Recursively walks through ultralytics dir and builds an MkDocs
|
4 |
+
reference section of *.md files composed of classes and functions, and also creates a nav menu for use in mkdocs.yaml.
|
5 |
+
|
6 |
+
Note: Must be run from repository root directory. Do not run from docs directory.
|
7 |
+
"""
|
8 |
+
|
9 |
+
import re
|
10 |
+
from collections import defaultdict
|
11 |
+
from pathlib import Path
|
12 |
+
|
13 |
+
from ultralytics.utils import ROOT
|
14 |
+
|
15 |
+
NEW_YAML_DIR = ROOT.parent
|
16 |
+
CODE_DIR = ROOT
|
17 |
+
REFERENCE_DIR = ROOT.parent / 'docs/reference'
|
18 |
+
|
19 |
+
|
20 |
+
def extract_classes_and_functions(filepath: Path) -> tuple:
|
21 |
+
"""Extracts class and function names from a given Python file."""
|
22 |
+
content = filepath.read_text()
|
23 |
+
class_pattern = r'(?:^|\n)class\s(\w+)(?:\(|:)'
|
24 |
+
func_pattern = r'(?:^|\n)def\s(\w+)\('
|
25 |
+
|
26 |
+
classes = re.findall(class_pattern, content)
|
27 |
+
functions = re.findall(func_pattern, content)
|
28 |
+
|
29 |
+
return classes, functions
|
30 |
+
|
31 |
+
|
32 |
+
def create_markdown(py_filepath: Path, module_path: str, classes: list, functions: list):
|
33 |
+
"""Creates a Markdown file containing the API reference for the given Python module."""
|
34 |
+
md_filepath = py_filepath.with_suffix('.md')
|
35 |
+
|
36 |
+
# Read existing content and keep header content between first two ---
|
37 |
+
header_content = ''
|
38 |
+
if md_filepath.exists():
|
39 |
+
existing_content = md_filepath.read_text()
|
40 |
+
header_parts = existing_content.split('---')
|
41 |
+
for part in header_parts:
|
42 |
+
if 'description:' in part or 'comments:' in part:
|
43 |
+
header_content += f'---{part}---\n\n'
|
44 |
+
|
45 |
+
module_name = module_path.replace('.__init__', '')
|
46 |
+
module_path = module_path.replace('.', '/')
|
47 |
+
url = f'https://github.com/ultralytics/ultralytics/blob/main/{module_path}.py'
|
48 |
+
title_content = (
|
49 |
+
f'# Reference for `{module_path}.py`\n\n'
|
50 |
+
f'!!! note\n\n'
|
51 |
+
f' Full source code for this file is available at [{url}]({url}). Help us fix any issues you see by submitting a [Pull Request](https://docs.ultralytics.com/help/contributing/) 🛠️. Thank you 🙏!\n\n'
|
52 |
+
)
|
53 |
+
md_content = [f'---\n## ::: {module_name}.{class_name}\n<br><br>\n' for class_name in classes]
|
54 |
+
md_content.extend(f'---\n## ::: {module_name}.{func_name}\n<br><br>\n' for func_name in functions)
|
55 |
+
md_content = header_content + title_content + '\n'.join(md_content)
|
56 |
+
if not md_content.endswith('\n'):
|
57 |
+
md_content += '\n'
|
58 |
+
|
59 |
+
md_filepath.parent.mkdir(parents=True, exist_ok=True)
|
60 |
+
md_filepath.write_text(md_content)
|
61 |
+
|
62 |
+
return md_filepath.relative_to(NEW_YAML_DIR)
|
63 |
+
|
64 |
+
|
65 |
+
def nested_dict() -> defaultdict:
|
66 |
+
"""Creates and returns a nested defaultdict."""
|
67 |
+
return defaultdict(nested_dict)
|
68 |
+
|
69 |
+
|
70 |
+
def sort_nested_dict(d: dict) -> dict:
|
71 |
+
"""Sorts a nested dictionary recursively."""
|
72 |
+
return {key: sort_nested_dict(value) if isinstance(value, dict) else value for key, value in sorted(d.items())}
|
73 |
+
|
74 |
+
|
75 |
+
def create_nav_menu_yaml(nav_items: list):
|
76 |
+
"""Creates a YAML file for the navigation menu based on the provided list of items."""
|
77 |
+
nav_tree = nested_dict()
|
78 |
+
|
79 |
+
for item_str in nav_items:
|
80 |
+
item = Path(item_str)
|
81 |
+
parts = item.parts
|
82 |
+
current_level = nav_tree['reference']
|
83 |
+
for part in parts[2:-1]: # skip the first two parts (docs and reference) and the last part (filename)
|
84 |
+
current_level = current_level[part]
|
85 |
+
|
86 |
+
md_file_name = parts[-1].replace('.md', '')
|
87 |
+
current_level[md_file_name] = item
|
88 |
+
|
89 |
+
nav_tree_sorted = sort_nested_dict(nav_tree)
|
90 |
+
|
91 |
+
def _dict_to_yaml(d, level=0):
|
92 |
+
"""Converts a nested dictionary to a YAML-formatted string with indentation."""
|
93 |
+
yaml_str = ''
|
94 |
+
indent = ' ' * level
|
95 |
+
for k, v in d.items():
|
96 |
+
if isinstance(v, dict):
|
97 |
+
yaml_str += f'{indent}- {k}:\n{_dict_to_yaml(v, level + 1)}'
|
98 |
+
else:
|
99 |
+
yaml_str += f"{indent}- {k}: {str(v).replace('docs/', '')}\n"
|
100 |
+
return yaml_str
|
101 |
+
|
102 |
+
# Print updated YAML reference section
|
103 |
+
print('Scan complete, new mkdocs.yaml reference section is:\n\n', _dict_to_yaml(nav_tree_sorted))
|
104 |
+
|
105 |
+
# Save new YAML reference section
|
106 |
+
# (NEW_YAML_DIR / 'nav_menu_updated.yml').write_text(_dict_to_yaml(nav_tree_sorted))
|
107 |
+
|
108 |
+
|
109 |
+
def main():
|
110 |
+
"""Main function to extract class and function names, create Markdown files, and generate a YAML navigation menu."""
|
111 |
+
nav_items = []
|
112 |
+
|
113 |
+
for py_filepath in CODE_DIR.rglob('*.py'):
|
114 |
+
classes, functions = extract_classes_and_functions(py_filepath)
|
115 |
+
|
116 |
+
if classes or functions:
|
117 |
+
py_filepath_rel = py_filepath.relative_to(CODE_DIR)
|
118 |
+
md_filepath = REFERENCE_DIR / py_filepath_rel
|
119 |
+
module_path = f"ultralytics.{py_filepath_rel.with_suffix('').as_posix().replace('/', '.')}"
|
120 |
+
md_rel_filepath = create_markdown(md_filepath, module_path, classes, functions)
|
121 |
+
nav_items.append(str(md_rel_filepath))
|
122 |
+
|
123 |
+
create_nav_menu_yaml(nav_items)
|
124 |
+
|
125 |
+
|
126 |
+
if __name__ == '__main__':
|
127 |
+
main()
|
ultralytics/docs/datasets/classify/caltech101.md
ADDED
@@ -0,0 +1,81 @@
|
|
|
|
|
|
|
|
|
|
|
|
|
|
|
|
|
|
|
|
|
|
|
|
|
|
|
|
|
|
|
|
|
|
|
|
|
|
|
|
|
|
|
|
|
|
|
|
|
|
|
|
|
|
|
|
|
|
|
|
|
|
|
|
|
|
|
|
|
|
|
|
|
|
|
|
|
|
|
|
|
|
|
|
|
|
|
|
|
|
|
|
|
|
|
|
|
|
|
|
|
|
|
|
|
|
|
|
|
|
|
|
|
|
|
|
|
|
|
|
|
|
|
|
|
|
|
|
|
|
|
|
|
|
|
|
|
|
|
|
|
|
|
|
|
|
|
|
|
|
|
|
|
|
|
|
|
|
|
|
|
|
|
|
|
1 |
+
---
|
2 |
+
comments: true
|
3 |
+
description: Learn about the Caltech-101 dataset, its structure and uses in machine learning. Includes instructions to train a YOLO model using this dataset.
|
4 |
+
keywords: Caltech-101, dataset, YOLO training, machine learning, object recognition, ultralytics
|
5 |
+
---
|
6 |
+
|
7 |
+
# Caltech-101 Dataset
|
8 |
+
|
9 |
+
The [Caltech-101](https://data.caltech.edu/records/mzrjq-6wc02) dataset is a widely used dataset for object recognition tasks, containing around 9,000 images from 101 object categories. The categories were chosen to reflect a variety of real-world objects, and the images themselves were carefully selected and annotated to provide a challenging benchmark for object recognition algorithms.
|
10 |
+
|
11 |
+
## Key Features
|
12 |
+
|
13 |
+
- The Caltech-101 dataset comprises around 9,000 color images divided into 101 categories.
|
14 |
+
- The categories encompass a wide variety of objects, including animals, vehicles, household items, and people.
|
15 |
+
- The number of images per category varies, with about 40 to 800 images in each category.
|
16 |
+
- Images are of variable sizes, with most images being medium resolution.
|
17 |
+
- Caltech-101 is widely used for training and testing in the field of machine learning, particularly for object recognition tasks.
|
18 |
+
|
19 |
+
## Dataset Structure
|
20 |
+
|
21 |
+
Unlike many other datasets, the Caltech-101 dataset is not formally split into training and testing sets. Users typically create their own splits based on their specific needs. However, a common practice is to use a random subset of images for training (e.g., 30 images per category) and the remaining images for testing.
|
22 |
+
|
23 |
+
## Applications
|
24 |
+
|
25 |
+
The Caltech-101 dataset is extensively used for training and evaluating deep learning models in object recognition tasks, such as Convolutional Neural Networks (CNNs), Support Vector Machines (SVMs), and various other machine learning algorithms. Its wide variety of categories and high-quality images make it an excellent dataset for research and development in the field of machine learning and computer vision.
|
26 |
+
|
27 |
+
## Usage
|
28 |
+
|
29 |
+
To train a YOLO model on the Caltech-101 dataset for 100 epochs, you can use the following code snippets. For a comprehensive list of available arguments, refer to the model [Training](../../modes/train.md) page.
|
30 |
+
|
31 |
+
!!! example "Train Example"
|
32 |
+
|
33 |
+
=== "Python"
|
34 |
+
|
35 |
+
```python
|
36 |
+
from ultralytics import YOLO
|
37 |
+
|
38 |
+
# Load a model
|
39 |
+
model = YOLO('yolov8n-cls.pt') # load a pretrained model (recommended for training)
|
40 |
+
|
41 |
+
# Train the model
|
42 |
+
results = model.train(data='caltech101', epochs=100, imgsz=416)
|
43 |
+
```
|
44 |
+
|
45 |
+
=== "CLI"
|
46 |
+
|
47 |
+
```bash
|
48 |
+
# Start training from a pretrained *.pt model
|
49 |
+
yolo detect train data=caltech101 model=yolov8n-cls.pt epochs=100 imgsz=416
|
50 |
+
```
|
51 |
+
|
52 |
+
## Sample Images and Annotations
|
53 |
+
|
54 |
+
The Caltech-101 dataset contains high-quality color images of various objects, providing a well-structured dataset for object recognition tasks. Here are some examples of images from the dataset:
|
55 |
+
|
56 |
+
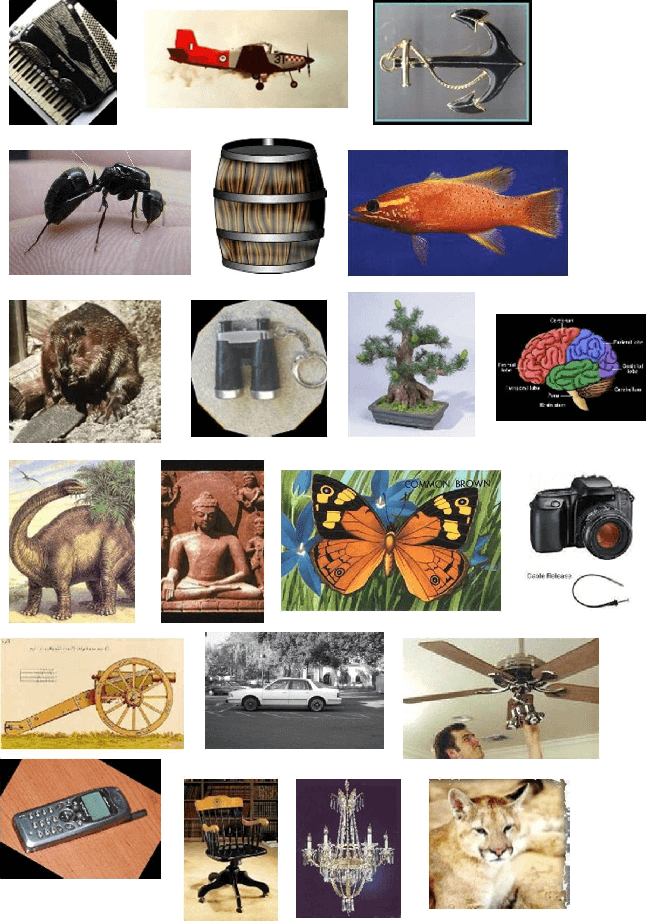
|
57 |
+
|
58 |
+
The example showcases the variety and complexity of the objects in the Caltech-101 dataset, emphasizing the significance of a diverse dataset for training robust object recognition models.
|
59 |
+
|
60 |
+
## Citations and Acknowledgments
|
61 |
+
|
62 |
+
If you use the Caltech-101 dataset in your research or development work, please cite the following paper:
|
63 |
+
|
64 |
+
!!! note ""
|
65 |
+
|
66 |
+
=== "BibTeX"
|
67 |
+
|
68 |
+
```bibtex
|
69 |
+
@article{fei2007learning,
|
70 |
+
title={Learning generative visual models from few training examples: An incremental Bayesian approach tested on 101 object categories},
|
71 |
+
author={Fei-Fei, Li and Fergus, Rob and Perona, Pietro},
|
72 |
+
journal={Computer vision and Image understanding},
|
73 |
+
volume={106},
|
74 |
+
number={1},
|
75 |
+
pages={59--70},
|
76 |
+
year={2007},
|
77 |
+
publisher={Elsevier}
|
78 |
+
}
|
79 |
+
```
|
80 |
+
|
81 |
+
We would like to acknowledge Li Fei-Fei, Rob Fergus, and Pietro Perona for creating and maintaining the Caltech-101 dataset as a valuable resource for the machine learning and computer vision research community. For more information about the Caltech-101 dataset and its creators, visit the [Caltech-101 dataset website](https://data.caltech.edu/records/mzrjq-6wc02).
|
ultralytics/docs/datasets/classify/caltech256.md
ADDED
@@ -0,0 +1,78 @@
|
|
|
|
|
|
|
|
|
|
|
|
|
|
|
|
|
|
|
|
|
|
|
|
|
|
|
|
|
|
|
|
|
|
|
|
|
|
|
|
|
|
|
|
|
|
|
|
|
|
|
|
|
|
|
|
|
|
|
|
|
|
|
|
|
|
|
|
|
|
|
|
|
|
|
|
|
|
|
|
|
|
|
|
|
|
|
|
|
|
|
|
|
|
|
|
|
|
|
|
|
|
|
|
|
|
|
|
|
|
|
|
|
|
|
|
|
|
|
|
|
|
|
|
|
|
|
|
|
|
|
|
|
|
|
|
|
|
|
|
|
|
|
|
|
|
|
|
|
|
|
|
|
|
|
|
|
|
|
1 |
+
---
|
2 |
+
comments: true
|
3 |
+
description: Explore the Caltech-256 dataset, a diverse collection of images used for object recognition tasks in machine learning. Learn to train a YOLO model on the dataset.
|
4 |
+
keywords: Ultralytics, YOLO, Caltech-256, dataset, object recognition, machine learning, computer vision, deep learning
|
5 |
+
---
|
6 |
+
|
7 |
+
# Caltech-256 Dataset
|
8 |
+
|
9 |
+
The [Caltech-256](https://data.caltech.edu/records/nyy15-4j048) dataset is an extensive collection of images used for object classification tasks. It contains around 30,000 images divided into 257 categories (256 object categories and 1 background category). The images are carefully curated and annotated to provide a challenging and diverse benchmark for object recognition algorithms.
|
10 |
+
|
11 |
+
## Key Features
|
12 |
+
|
13 |
+
- The Caltech-256 dataset comprises around 30,000 color images divided into 257 categories.
|
14 |
+
- Each category contains a minimum of 80 images.
|
15 |
+
- The categories encompass a wide variety of real-world objects, including animals, vehicles, household items, and people.
|
16 |
+
- Images are of variable sizes and resolutions.
|
17 |
+
- Caltech-256 is widely used for training and testing in the field of machine learning, particularly for object recognition tasks.
|
18 |
+
|
19 |
+
## Dataset Structure
|
20 |
+
|
21 |
+
Like Caltech-101, the Caltech-256 dataset does not have a formal split between training and testing sets. Users typically create their own splits according to their specific needs. A common practice is to use a random subset of images for training and the remaining images for testing.
|
22 |
+
|
23 |
+
## Applications
|
24 |
+
|
25 |
+
The Caltech-256 dataset is extensively used for training and evaluating deep learning models in object recognition tasks, such as Convolutional Neural Networks (CNNs), Support Vector Machines (SVMs), and various other machine learning algorithms. Its diverse set of categories and high-quality images make it an invaluable dataset for research and development in the field of machine learning and computer vision.
|
26 |
+
|
27 |
+
## Usage
|
28 |
+
|
29 |
+
To train a YOLO model on the Caltech-256 dataset for 100 epochs, you can use the following code snippets. For a comprehensive list of available arguments, refer to the model [Training](../../modes/train.md) page.
|
30 |
+
|
31 |
+
!!! example "Train Example"
|
32 |
+
|
33 |
+
=== "Python"
|
34 |
+
|
35 |
+
```python
|
36 |
+
from ultralytics import YOLO
|
37 |
+
|
38 |
+
# Load a model
|
39 |
+
model = YOLO('yolov8n-cls.pt') # load a pretrained model (recommended for training)
|
40 |
+
|
41 |
+
# Train the model
|
42 |
+
results = model.train(data='caltech256', epochs=100, imgsz=416)
|
43 |
+
```
|
44 |
+
|
45 |
+
=== "CLI"
|
46 |
+
|
47 |
+
```bash
|
48 |
+
# Start training from a pretrained *.pt model
|
49 |
+
yolo detect train data=caltech256 model=yolov8n-cls.pt epochs=100 imgsz=416
|
50 |
+
```
|
51 |
+
|
52 |
+
## Sample Images and Annotations
|
53 |
+
|
54 |
+
The Caltech-256 dataset contains high-quality color images of various objects, providing a comprehensive dataset for object recognition tasks. Here are some examples of images from the dataset ([credit](https://ml4a.github.io/demos/tsne_viewer.html)):
|
55 |
+
|
56 |
+
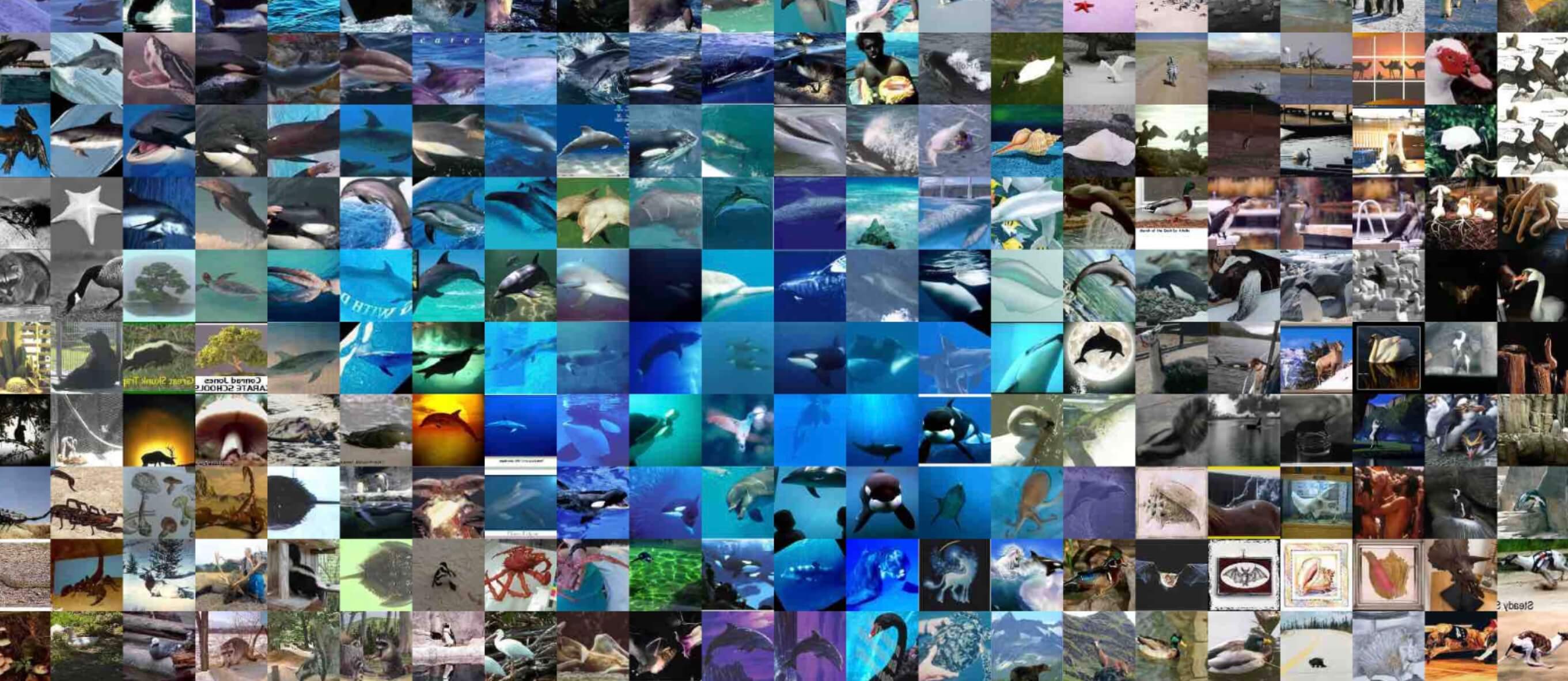
|
57 |
+
|
58 |
+
The example showcases the diversity and complexity of the objects in the Caltech-256 dataset, emphasizing the importance of a varied dataset for training robust object recognition models.
|
59 |
+
|
60 |
+
## Citations and Acknowledgments
|
61 |
+
|
62 |
+
If you use the Caltech-256 dataset in your research or development work, please cite the following paper:
|
63 |
+
|
64 |
+
!!! note ""
|
65 |
+
|
66 |
+
=== "BibTeX"
|
67 |
+
|
68 |
+
```bibtex
|
69 |
+
@article{griffin2007caltech,
|
70 |
+
title={Caltech-256 object category dataset},
|
71 |
+
author={Griffin, Gregory and Holub, Alex and Perona, Pietro},
|
72 |
+
year={2007}
|
73 |
+
}
|
74 |
+
```
|
75 |
+
|
76 |
+
We would like to acknowledge Gregory Griffin, Alex Holub, and Pietro Perona for creating and maintaining the Caltech-256 dataset as a valuable resource for the machine learning and computer vision research community. For more information about the
|
77 |
+
|
78 |
+
Caltech-256 dataset and its creators, visit the [Caltech-256 dataset website](https://data.caltech.edu/records/nyy15-4j048).
|
ultralytics/docs/datasets/classify/cifar10.md
ADDED
@@ -0,0 +1,80 @@
|
|
|
|
|
|
|
|
|
|
|
|
|
|
|
|
|
|
|
|
|
|
|
|
|
|
|
|
|
|
|
|
|
|
|
|
|
|
|
|
|
|
|
|
|
|
|
|
|
|
|
|
|
|
|
|
|
|
|
|
|
|
|
|
|
|
|
|
|
|
|
|
|
|
|
|
|
|
|
|
|
|
|
|
|
|
|
|
|
|
|
|
|
|
|
|
|
|
|
|
|
|
|
|
|
|
|
|
|
|
|
|
|
|
|
|
|
|
|
|
|
|
|
|
|
|
|
|
|
|
|
|
|
|
|
|
|
|
|
|
|
|
|
|
|
|
|
|
|
|
|
|
|
|
|
|
|
|
|
|
|
|
|
1 |
+
---
|
2 |
+
comments: true
|
3 |
+
description: Explore the CIFAR-10 dataset, widely used for training in machine learning and computer vision, and learn how to use it with Ultralytics YOLO.
|
4 |
+
keywords: CIFAR-10, dataset, machine learning, image classification, computer vision, YOLO, Ultralytics, training, testing, deep learning, Convolutional Neural Networks, Support Vector Machines
|
5 |
+
---
|
6 |
+
|
7 |
+
# CIFAR-10 Dataset
|
8 |
+
|
9 |
+
The [CIFAR-10](https://www.cs.toronto.edu/~kriz/cifar.html) (Canadian Institute For Advanced Research) dataset is a collection of images used widely for machine learning and computer vision algorithms. It was developed by researchers at the CIFAR institute and consists of 60,000 32x32 color images in 10 different classes.
|
10 |
+
|
11 |
+
## Key Features
|
12 |
+
|
13 |
+
- The CIFAR-10 dataset consists of 60,000 images, divided into 10 classes.
|
14 |
+
- Each class contains 6,000 images, split into 5,000 for training and 1,000 for testing.
|
15 |
+
- The images are colored and of size 32x32 pixels.
|
16 |
+
- The 10 different classes represent airplanes, cars, birds, cats, deer, dogs, frogs, horses, ships, and trucks.
|
17 |
+
- CIFAR-10 is commonly used for training and testing in the field of machine learning and computer vision.
|
18 |
+
|
19 |
+
## Dataset Structure
|
20 |
+
|
21 |
+
The CIFAR-10 dataset is split into two subsets:
|
22 |
+
|
23 |
+
1. **Training Set**: This subset contains 50,000 images used for training machine learning models.
|
24 |
+
2. **Testing Set**: This subset consists of 10,000 images used for testing and benchmarking the trained models.
|
25 |
+
|
26 |
+
## Applications
|
27 |
+
|
28 |
+
The CIFAR-10 dataset is widely used for training and evaluating deep learning models in image classification tasks, such as Convolutional Neural Networks (CNNs), Support Vector Machines (SVMs), and various other machine learning algorithms. The diversity of the dataset in terms of classes and the presence of color images make it a well-rounded dataset for research and development in the field of machine learning and computer vision.
|
29 |
+
|
30 |
+
## Usage
|
31 |
+
|
32 |
+
To train a YOLO model on the CIFAR-10 dataset for 100 epochs with an image size of 32x32, you can use the following code snippets. For a comprehensive list of available arguments, refer to the model [Training](../../modes/train.md) page.
|
33 |
+
|
34 |
+
!!! example "Train Example"
|
35 |
+
|
36 |
+
=== "Python"
|
37 |
+
|
38 |
+
```python
|
39 |
+
from ultralytics import YOLO
|
40 |
+
|
41 |
+
# Load a model
|
42 |
+
model = YOLO('yolov8n-cls.pt') # load a pretrained model (recommended for training)
|
43 |
+
|
44 |
+
# Train the model
|
45 |
+
results = model.train(data='cifar10', epochs=100, imgsz=32)
|
46 |
+
```
|
47 |
+
|
48 |
+
=== "CLI"
|
49 |
+
|
50 |
+
```bash
|
51 |
+
# Start training from a pretrained *.pt model
|
52 |
+
yolo detect train data=cifar10 model=yolov8n-cls.pt epochs=100 imgsz=32
|
53 |
+
```
|
54 |
+
|
55 |
+
## Sample Images and Annotations
|
56 |
+
|
57 |
+
The CIFAR-10 dataset contains color images of various objects, providing a well-structured dataset for image classification tasks. Here are some examples of images from the dataset:
|
58 |
+
|
59 |
+
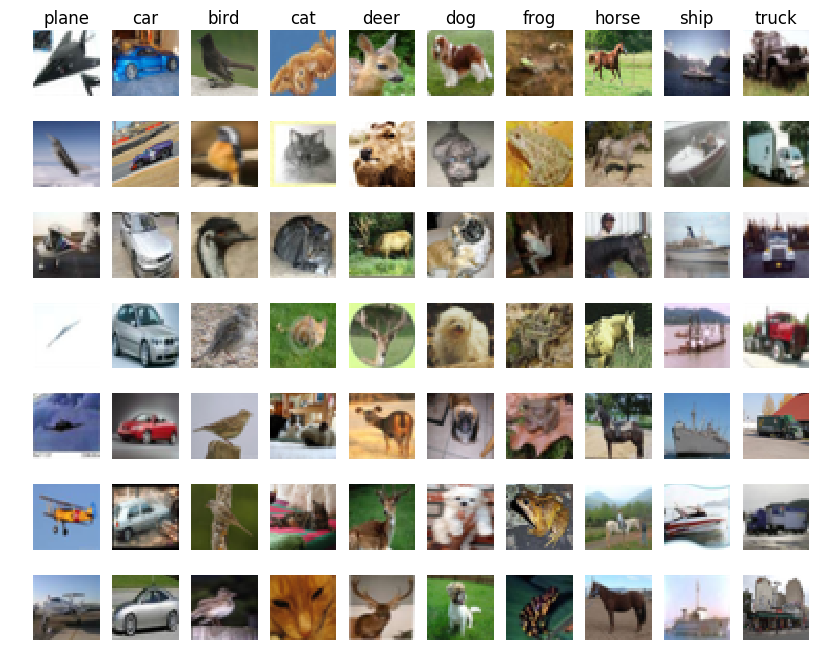
|
60 |
+
|
61 |
+
The example showcases the variety and complexity of the objects in the CIFAR-10 dataset, highlighting the importance of a diverse dataset for training robust image classification models.
|
62 |
+
|
63 |
+
## Citations and Acknowledgments
|
64 |
+
|
65 |
+
If you use the CIFAR-10 dataset in your research or development work, please cite the following paper:
|
66 |
+
|
67 |
+
!!! note ""
|
68 |
+
|
69 |
+
=== "BibTeX"
|
70 |
+
|
71 |
+
```bibtex
|
72 |
+
@TECHREPORT{Krizhevsky09learningmultiple,
|
73 |
+
author={Alex Krizhevsky},
|
74 |
+
title={Learning multiple layers of features from tiny images},
|
75 |
+
institution={},
|
76 |
+
year={2009}
|
77 |
+
}
|
78 |
+
```
|
79 |
+
|
80 |
+
We would like to acknowledge Alex Krizhevsky for creating and maintaining the CIFAR-10 dataset as a valuable resource for the machine learning and computer vision research community. For more information about the CIFAR-10 dataset and its creator, visit the [CIFAR-10 dataset website](https://www.cs.toronto.edu/~kriz/cifar.html).
|
ultralytics/docs/datasets/classify/cifar100.md
ADDED
@@ -0,0 +1,80 @@
|
|
|
|
|
|
|
|
|
|
|
|
|
|
|
|
|
|
|
|
|
|
|
|
|
|
|
|
|
|
|
|
|
|
|
|
|
|
|
|
|
|
|
|
|
|
|
|
|
|
|
|
|
|
|
|
|
|
|
|
|
|
|
|
|
|
|
|
|
|
|
|
|
|
|
|
|
|
|
|
|
|
|
|
|
|
|
|
|
|
|
|
|
|
|
|
|
|
|
|
|
|
|
|
|
|
|
|
|
|
|
|
|
|
|
|
|
|
|
|
|
|
|
|
|
|
|
|
|
|
|
|
|
|
|
|
|
|
|
|
|
|
|
|
|
|
|
|
|
|
|
|
|
|
|
|
|
|
|
|
|
|
|
1 |
+
---
|
2 |
+
comments: true
|
3 |
+
description: Discover how to leverage the CIFAR-100 dataset for machine learning and computer vision tasks with YOLO. Gain insights on its structure, use, and utilization for model training.
|
4 |
+
keywords: Ultralytics, YOLO, CIFAR-100 dataset, image classification, machine learning, computer vision, YOLO model training
|
5 |
+
---
|
6 |
+
|
7 |
+
# CIFAR-100 Dataset
|
8 |
+
|
9 |
+
The [CIFAR-100](https://www.cs.toronto.edu/~kriz/cifar.html) (Canadian Institute For Advanced Research) dataset is a significant extension of the CIFAR-10 dataset, composed of 60,000 32x32 color images in 100 different classes. It was developed by researchers at the CIFAR institute, offering a more challenging dataset for more complex machine learning and computer vision tasks.
|
10 |
+
|
11 |
+
## Key Features
|
12 |
+
|
13 |
+
- The CIFAR-100 dataset consists of 60,000 images, divided into 100 classes.
|
14 |
+
- Each class contains 600 images, split into 500 for training and 100 for testing.
|
15 |
+
- The images are colored and of size 32x32 pixels.
|
16 |
+
- The 100 different classes are grouped into 20 coarse categories for higher level classification.
|
17 |
+
- CIFAR-100 is commonly used for training and testing in the field of machine learning and computer vision.
|
18 |
+
|
19 |
+
## Dataset Structure
|
20 |
+
|
21 |
+
The CIFAR-100 dataset is split into two subsets:
|
22 |
+
|
23 |
+
1. **Training Set**: This subset contains 50,000 images used for training machine learning models.
|
24 |
+
2. **Testing Set**: This subset consists of 10,000 images used for testing and benchmarking the trained models.
|
25 |
+
|
26 |
+
## Applications
|
27 |
+
|
28 |
+
The CIFAR-100 dataset is extensively used for training and evaluating deep learning models in image classification tasks, such as Convolutional Neural Networks (CNNs), Support Vector Machines (SVMs), and various other machine learning algorithms. The diversity of the dataset in terms of classes and the presence of color images make it a more challenging and comprehensive dataset for research and development in the field of machine learning and computer vision.
|
29 |
+
|
30 |
+
## Usage
|
31 |
+
|
32 |
+
To train a YOLO model on the CIFAR-100 dataset for 100 epochs with an image size of 32x32, you can use the following code snippets. For a comprehensive list of available arguments, refer to the model [Training](../../modes/train.md) page.
|
33 |
+
|
34 |
+
!!! example "Train Example"
|
35 |
+
|
36 |
+
=== "Python"
|
37 |
+
|
38 |
+
```python
|
39 |
+
from ultralytics import YOLO
|
40 |
+
|
41 |
+
# Load a model
|
42 |
+
model = YOLO('yolov8n-cls.pt') # load a pretrained model (recommended for training)
|
43 |
+
|
44 |
+
# Train the model
|
45 |
+
results = model.train(data='cifar100', epochs=100, imgsz=32)
|
46 |
+
```
|
47 |
+
|
48 |
+
=== "CLI"
|
49 |
+
|
50 |
+
```bash
|
51 |
+
# Start training from a pretrained *.pt model
|
52 |
+
yolo detect train data=cifar100 model=yolov8n-cls.pt epochs=100 imgsz=32
|
53 |
+
```
|
54 |
+
|
55 |
+
## Sample Images and Annotations
|
56 |
+
|
57 |
+
The CIFAR-100 dataset contains color images of various objects, providing a well-structured dataset for image classification tasks. Here are some examples of images from the dataset:
|
58 |
+
|
59 |
+
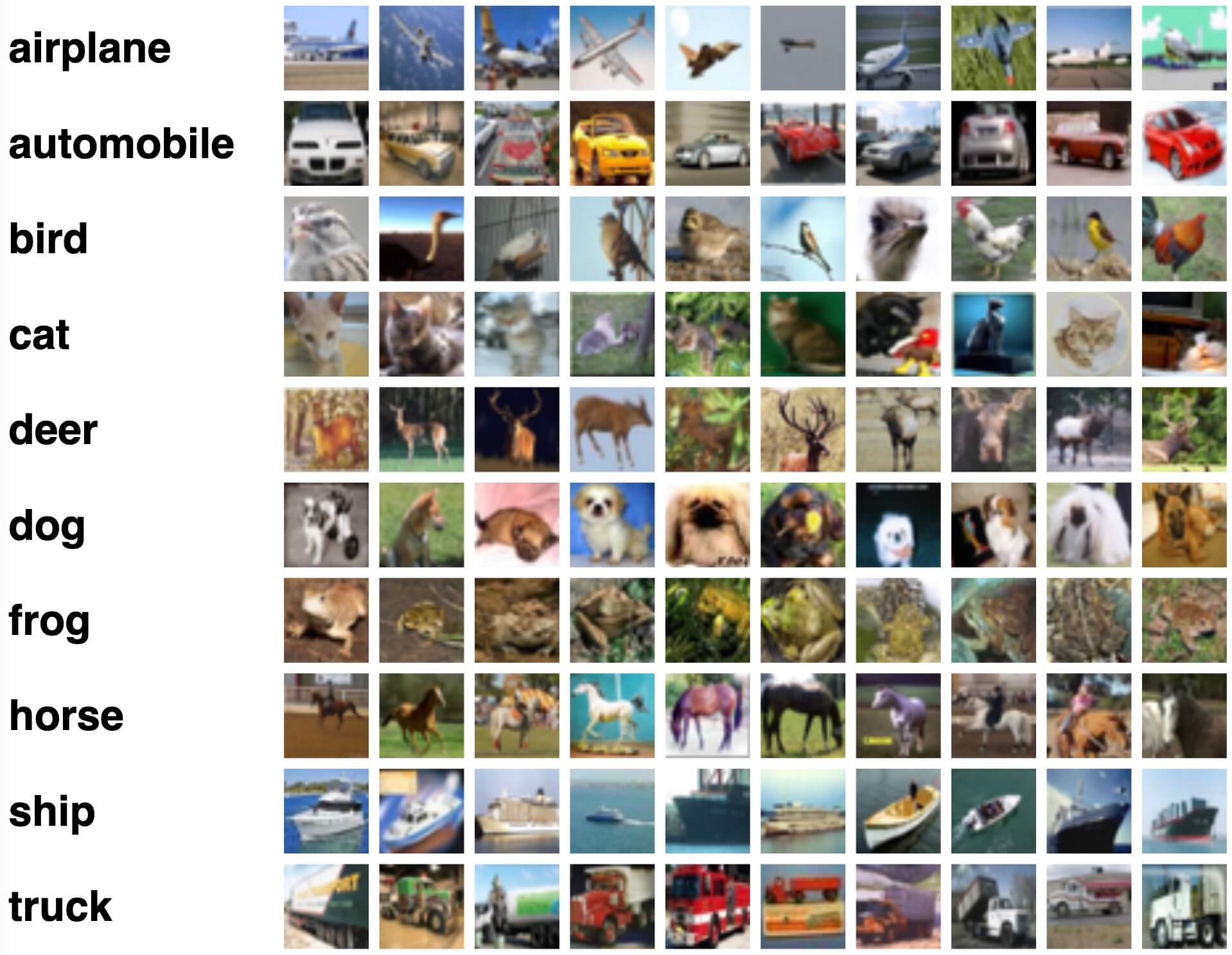
|
60 |
+
|
61 |
+
The example showcases the variety and complexity of the objects in the CIFAR-100 dataset, highlighting the importance of a diverse dataset for training robust image classification models.
|
62 |
+
|
63 |
+
## Citations and Acknowledgments
|
64 |
+
|
65 |
+
If you use the CIFAR-100 dataset in your research or development work, please cite the following paper:
|
66 |
+
|
67 |
+
!!! note ""
|
68 |
+
|
69 |
+
=== "BibTeX"
|
70 |
+
|
71 |
+
```bibtex
|
72 |
+
@TECHREPORT{Krizhevsky09learningmultiple,
|
73 |
+
author={Alex Krizhevsky},
|
74 |
+
title={Learning multiple layers of features from tiny images},
|
75 |
+
institution={},
|
76 |
+
year={2009}
|
77 |
+
}
|
78 |
+
```
|
79 |
+
|
80 |
+
We would like to acknowledge Alex Krizhevsky for creating and maintaining the CIFAR-100 dataset as a valuable resource for the machine learning and computer vision research community. For more information about the CIFAR-100 dataset and its creator, visit the [CIFAR-100 dataset website](https://www.cs.toronto.edu/~kriz/cifar.html).
|
ultralytics/docs/datasets/classify/fashion-mnist.md
ADDED
@@ -0,0 +1,79 @@
|
|
|
|
|
|
|
|
|
|
|
|
|
|
|
|
|
|
|
|
|
|
|
|
|
|
|
|
|
|
|
|
|
|
|
|
|
|
|
|
|
|
|
|
|
|
|
|
|
|
|
|
|
|
|
|
|
|
|
|
|
|
|
|
|
|
|
|
|
|
|
|
|
|
|
|
|
|
|
|
|
|
|
|
|
|
|
|
|
|
|
|
|
|
|
|
|
|
|
|
|
|
|
|
|
|
|
|
|
|
|
|
|
|
|
|
|
|
|
|
|
|
|
|
|
|
|
|
|
|
|
|
|
|
|
|
|
|
|
|
|
|
|
|
|
|
|
|
|
|
|
|
|
|
|
|
|
|
|
|
|
1 |
+
---
|
2 |
+
comments: true
|
3 |
+
description: Learn how to use the Fashion-MNIST dataset for image classification with the Ultralytics YOLO model. Covers dataset structure, labels, applications, and usage.
|
4 |
+
keywords: Ultralytics, YOLO, Fashion-MNIST, dataset, image classification, machine learning, deep learning, neural networks, training, testing
|
5 |
+
---
|
6 |
+
|
7 |
+
# Fashion-MNIST Dataset
|
8 |
+
|
9 |
+
The [Fashion-MNIST](https://github.com/zalandoresearch/fashion-mnist) dataset is a database of Zalando's article images—consisting of a training set of 60,000 examples and a test set of 10,000 examples. Each example is a 28x28 grayscale image, associated with a label from 10 classes. Fashion-MNIST is intended to serve as a direct drop-in replacement for the original MNIST dataset for benchmarking machine learning algorithms.
|
10 |
+
|
11 |
+
## Key Features
|
12 |
+
|
13 |
+
- Fashion-MNIST contains 60,000 training images and 10,000 testing images of Zalando's article images.
|
14 |
+
- The dataset comprises grayscale images of size 28x28 pixels.
|
15 |
+
- Each pixel has a single pixel-value associated with it, indicating the lightness or darkness of that pixel, with higher numbers meaning darker. This pixel-value is an integer between 0 and 255.
|
16 |
+
- Fashion-MNIST is widely used for training and testing in the field of machine learning, especially for image classification tasks.
|
17 |
+
|
18 |
+
## Dataset Structure
|
19 |
+
|
20 |
+
The Fashion-MNIST dataset is split into two subsets:
|
21 |
+
|
22 |
+
1. **Training Set**: This subset contains 60,000 images used for training machine learning models.
|
23 |
+
2. **Testing Set**: This subset consists of 10,000 images used for testing and benchmarking the trained models.
|
24 |
+
|
25 |
+
## Labels
|
26 |
+
|
27 |
+
Each training and test example is assigned to one of the following labels:
|
28 |
+
|
29 |
+
0. T-shirt/top
|
30 |
+
1. Trouser
|
31 |
+
2. Pullover
|
32 |
+
3. Dress
|
33 |
+
4. Coat
|
34 |
+
5. Sandal
|
35 |
+
6. Shirt
|
36 |
+
7. Sneaker
|
37 |
+
8. Bag
|
38 |
+
9. Ankle boot
|
39 |
+
|
40 |
+
## Applications
|
41 |
+
|
42 |
+
The Fashion-MNIST dataset is widely used for training and evaluating deep learning models in image classification tasks, such as Convolutional Neural Networks (CNNs), Support Vector Machines (SVMs), and various other machine learning algorithms. The dataset's simple and well-structured format makes it an essential resource for researchers and practitioners in the field of machine learning and computer vision.
|
43 |
+
|
44 |
+
## Usage
|
45 |
+
|
46 |
+
To train a CNN model on the Fashion-MNIST dataset for 100 epochs with an image size of 28x28, you can use the following code snippets. For a comprehensive list of available arguments, refer to the model [Training](../../modes/train.md) page.
|
47 |
+
|
48 |
+
!!! example "Train Example"
|
49 |
+
|
50 |
+
=== "Python"
|
51 |
+
|
52 |
+
```python
|
53 |
+
from ultralytics import YOLO
|
54 |
+
|
55 |
+
# Load a model
|
56 |
+
model = YOLO('yolov8n-cls.pt') # load a pretrained model (recommended for training)
|
57 |
+
|
58 |
+
# Train the model
|
59 |
+
results = model.train(data='fashion-mnist', epochs=100, imgsz=28)
|
60 |
+
```
|
61 |
+
|
62 |
+
=== "CLI"
|
63 |
+
|
64 |
+
```bash
|
65 |
+
# Start training from a pretrained *.pt model
|
66 |
+
yolo detect train data=fashion-mnist model=yolov8n-cls.pt epochs=100 imgsz=28
|
67 |
+
```
|
68 |
+
|
69 |
+
## Sample Images and Annotations
|
70 |
+
|
71 |
+
The Fashion-MNIST dataset contains grayscale images of Zalando's article images, providing a well-structured dataset for image classification tasks. Here are some examples of images from the dataset:
|
72 |
+
|
73 |
+
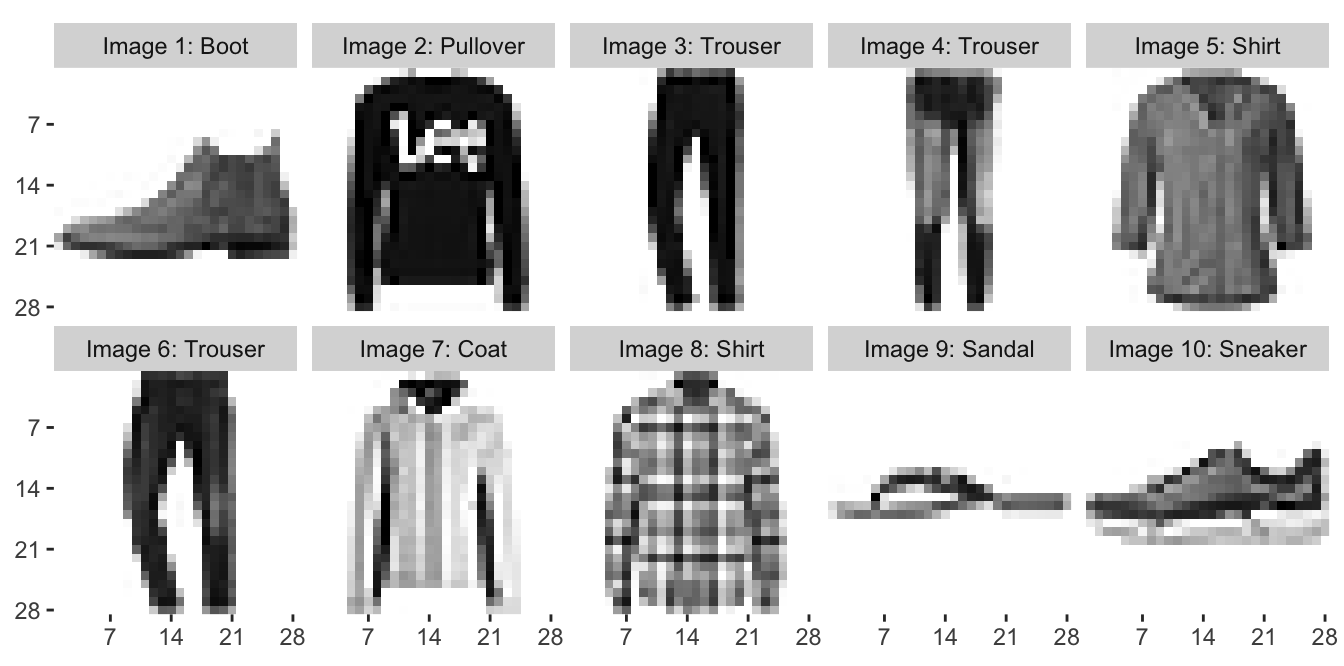
|
74 |
+
|
75 |
+
The example showcases the variety and complexity of the images in the Fashion-MNIST dataset, highlighting the importance of a diverse dataset for training robust image classification models.
|
76 |
+
|
77 |
+
## Acknowledgments
|
78 |
+
|
79 |
+
If you use the Fashion-MNIST dataset in your research or development work, please acknowledge the dataset by linking to the [GitHub repository](https://github.com/zalandoresearch/fashion-mnist). This dataset was made available by Zalando Research.
|
ultralytics/docs/datasets/classify/imagenet.md
ADDED
@@ -0,0 +1,83 @@
|
|
|
|
|
|
|
|
|
|
|
|
|
|
|
|
|
|
|
|
|
|
|
|
|
|
|
|
|
|
|
|
|
|
|
|
|
|
|
|
|
|
|
|
|
|
|
|
|
|
|
|
|
|
|
|
|
|
|
|
|
|
|
|
|
|
|
|
|
|
|
|
|
|
|
|
|
|
|
|
|
|
|
|
|
|
|
|
|
|
|
|
|
|
|
|
|
|
|
|
|
|
|
|
|
|
|
|
|
|
|
|
|
|
|
|
|
|
|
|
|
|
|
|
|
|
|
|
|
|
|
|
|
|
|
|
|
|
|
|
|
|
|
|
|
|
|
|
|
|
|
|
|
|
|
|
|
|
|
|
|
|
|
|
|
|
|
|
|
1 |
+
---
|
2 |
+
comments: true
|
3 |
+
description: Understand how to use ImageNet, an extensive annotated image dataset for object recognition research, with Ultralytics YOLO models. Learn about its structure, usage, and significance in computer vision.
|
4 |
+
keywords: Ultralytics, YOLO, ImageNet, dataset, object recognition, deep learning, computer vision, machine learning, dataset training, model training, image classification, object detection
|
5 |
+
---
|
6 |
+
|
7 |
+
# ImageNet Dataset
|
8 |
+
|
9 |
+
[ImageNet](https://www.image-net.org/) is a large-scale database of annotated images designed for use in visual object recognition research. It contains over 14 million images, with each image annotated using WordNet synsets, making it one of the most extensive resources available for training deep learning models in computer vision tasks.
|
10 |
+
|
11 |
+
## Key Features
|
12 |
+
|
13 |
+
- ImageNet contains over 14 million high-resolution images spanning thousands of object categories.
|
14 |
+
- The dataset is organized according to the WordNet hierarchy, with each synset representing a category.
|
15 |
+
- ImageNet is widely used for training and benchmarking in the field of computer vision, particularly for image classification and object detection tasks.
|
16 |
+
- The annual ImageNet Large Scale Visual Recognition Challenge (ILSVRC) has been instrumental in advancing computer vision research.
|
17 |
+
|
18 |
+
## Dataset Structure
|
19 |
+
|
20 |
+
The ImageNet dataset is organized using the WordNet hierarchy. Each node in the hierarchy represents a category, and each category is described by a synset (a collection of synonymous terms). The images in ImageNet are annotated with one or more synsets, providing a rich resource for training models to recognize various objects and their relationships.
|
21 |
+
|
22 |
+
## ImageNet Large Scale Visual Recognition Challenge (ILSVRC)
|
23 |
+
|
24 |
+
The annual [ImageNet Large Scale Visual Recognition Challenge (ILSVRC)](http://image-net.org/challenges/LSVRC/) has been an important event in the field of computer vision. It has provided a platform for researchers and developers to evaluate their algorithms and models on a large-scale dataset with standardized evaluation metrics. The ILSVRC has led to significant advancements in the development of deep learning models for image classification, object detection, and other computer vision tasks.
|
25 |
+
|
26 |
+
## Applications
|
27 |
+
|
28 |
+
The ImageNet dataset is widely used for training and evaluating deep learning models in various computer vision tasks, such as image classification, object detection, and object localization. Some popular deep learning architectures, such as AlexNet, VGG, and ResNet, were developed and benchmarked using the ImageNet dataset.
|
29 |
+
|
30 |
+
## Usage
|
31 |
+
|
32 |
+
To train a deep learning model on the ImageNet dataset for 100 epochs with an image size of 224x224, you can use the following code snippets. For a comprehensive list of available arguments, refer to the model [Training](../../modes/train.md) page.
|
33 |
+
|
34 |
+
!!! example "Train Example"
|
35 |
+
|
36 |
+
=== "Python"
|
37 |
+
|
38 |
+
```python
|
39 |
+
from ultralytics import YOLO
|
40 |
+
|
41 |
+
# Load a model
|
42 |
+
model = YOLO('yolov8n-cls.pt') # load a pretrained model (recommended for training)
|
43 |
+
|
44 |
+
# Train the model
|
45 |
+
results = model.train(data='imagenet', epochs=100, imgsz=224)
|
46 |
+
```
|
47 |
+
|
48 |
+
=== "CLI"
|
49 |
+
|
50 |
+
```bash
|
51 |
+
# Start training from a pretrained *.pt model
|
52 |
+
yolo train data=imagenet model=yolov8n-cls.pt epochs=100 imgsz=224
|
53 |
+
```
|
54 |
+
|
55 |
+
## Sample Images and Annotations
|
56 |
+
|
57 |
+
The ImageNet dataset contains high-resolution images spanning thousands of object categories, providing a diverse and extensive dataset for training and evaluating computer vision models. Here are some examples of images from the dataset:
|
58 |
+
|
59 |
+
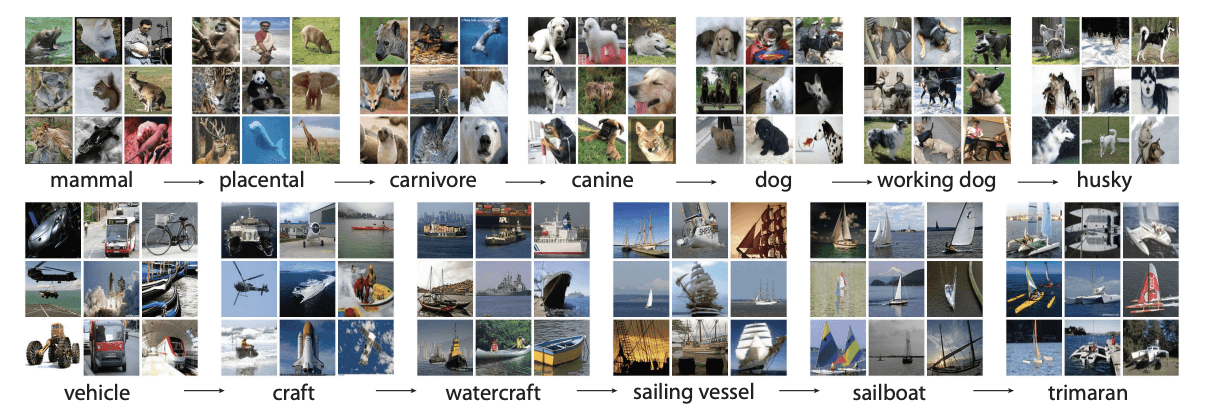
|
60 |
+
|
61 |
+
The example showcases the variety and complexity of the images in the ImageNet dataset, highlighting the importance of a diverse dataset for training robust computer vision models.
|
62 |
+
|
63 |
+
## Citations and Acknowledgments
|
64 |
+
|
65 |
+
If you use the ImageNet dataset in your research or development work, please cite the following paper:
|
66 |
+
|
67 |
+
!!! note ""
|
68 |
+
|
69 |
+
=== "BibTeX"
|
70 |
+
|
71 |
+
```bibtex
|
72 |
+
@article{ILSVRC15,
|
73 |
+
author = {Olga Russakovsky and Jia Deng and Hao Su and Jonathan Krause and Sanjeev Satheesh and Sean Ma and Zhiheng Huang and Andrej Karpathy and Aditya Khosla and Michael Bernstein and Alexander C. Berg and Li Fei-Fei},
|
74 |
+
title={ImageNet Large Scale Visual Recognition Challenge},
|
75 |
+
year={2015},
|
76 |
+
journal={International Journal of Computer Vision (IJCV)},
|
77 |
+
volume={115},
|
78 |
+
number={3},
|
79 |
+
pages={211-252}
|
80 |
+
}
|
81 |
+
```
|
82 |
+
|
83 |
+
We would like to acknowledge the ImageNet team, led by Olga Russakovsky, Jia Deng, and Li Fei-Fei, for creating and maintaining the ImageNet dataset as a valuable resource for the machine learning and computer vision research community. For more information about the ImageNet dataset and its creators, visit the [ImageNet website](https://www.image-net.org/).
|
ultralytics/docs/datasets/classify/imagenet10.md
ADDED
@@ -0,0 +1,78 @@
|
|
|
|
|
|
|
|
|
|
|
|
|
|
|
|
|
|
|
|
|
|
|
|
|
|
|
|
|
|
|
|
|
|
|
|
|
|
|
|
|
|
|
|
|
|
|
|
|
|
|
|
|
|
|
|
|
|
|
|
|
|
|
|
|
|
|
|
|
|
|
|
|
|
|
|
|
|
|
|
|
|
|
|
|
|
|
|
|
|
|
|
|
|
|
|
|
|
|
|
|
|
|
|
|
|
|
|
|
|
|
|
|
|
|
|
|
|
|
|
|
|
|
|
|
|
|
|
|
|
|
|
|
|
|
|
|
|
|
|
|
|
|
|
|
|
|
|
|
|
|
|
|
|
|
|
|
|
|
1 |
+
---
|
2 |
+
comments: true
|
3 |
+
description: Explore the compact ImageNet10 Dataset developed by Ultralytics. Ideal for fast testing of computer vision training pipelines and CV model sanity checks.
|
4 |
+
keywords: Ultralytics, YOLO, ImageNet10 Dataset, Image detection, Deep Learning, ImageNet, AI model testing, Computer vision, Machine learning
|
5 |
+
---
|
6 |
+
|
7 |
+
# ImageNet10 Dataset
|
8 |
+
|
9 |
+
The [ImageNet10](https://github.com/ultralytics/yolov5/releases/download/v1.0/imagenet10.zip) dataset is a small-scale subset of the [ImageNet](https://www.image-net.org/) database, developed by [Ultralytics](https://ultralytics.com) and designed for CI tests, sanity checks, and fast testing of training pipelines. This dataset is composed of the first image in the training set and the first image from the validation set of the first 10 classes in ImageNet. Although significantly smaller, it retains the structure and diversity of the original ImageNet dataset.
|
10 |
+
|
11 |
+
## Key Features
|
12 |
+
|
13 |
+
- ImageNet10 is a compact version of ImageNet, with 20 images representing the first 10 classes of the original dataset.
|
14 |
+
- The dataset is organized according to the WordNet hierarchy, mirroring the structure of the full ImageNet dataset.
|
15 |
+
- It is ideally suited for CI tests, sanity checks, and rapid testing of training pipelines in computer vision tasks.
|
16 |
+
- Although not designed for model benchmarking, it can provide a quick indication of a model's basic functionality and correctness.
|
17 |
+
|
18 |
+
## Dataset Structure
|
19 |
+
|
20 |
+
The ImageNet10 dataset, like the original ImageNet, is organized using the WordNet hierarchy. Each of the 10 classes in ImageNet10 is described by a synset (a collection of synonymous terms). The images in ImageNet10 are annotated with one or more synsets, providing a compact resource for testing models to recognize various objects and their relationships.
|
21 |
+
|
22 |
+
## Applications
|
23 |
+
|
24 |
+
The ImageNet10 dataset is useful for quickly testing and debugging computer vision models and pipelines. Its small size allows for rapid iteration, making it ideal for continuous integration tests and sanity checks. It can also be used for fast preliminary testing of new models or changes to existing models before moving on to full-scale testing with the complete ImageNet dataset.
|
25 |
+
|
26 |
+
## Usage
|
27 |
+
|
28 |
+
To test a deep learning model on the ImageNet10 dataset with an image size of 224x224, you can use the following code snippets. For a comprehensive list of available arguments, refer to the model [Training](../../modes/train.md) page.
|
29 |
+
|
30 |
+
!!! example "Test Example"
|
31 |
+
|
32 |
+
=== "Python"
|
33 |
+
|
34 |
+
```python
|
35 |
+
from ultralytics import YOLO
|
36 |
+
|
37 |
+
# Load a model
|
38 |
+
model = YOLO('yolov8n-cls.pt') # load a pretrained model (recommended for training)
|
39 |
+
|
40 |
+
# Train the model
|
41 |
+
results = model.train(data='imagenet10', epochs=5, imgsz=224)
|
42 |
+
```
|
43 |
+
|
44 |
+
=== "CLI"
|
45 |
+
|
46 |
+
```bash
|
47 |
+
# Start training from a pretrained *.pt model
|
48 |
+
yolo train data=imagenet10 model=yolov8n-cls.pt epochs=5 imgsz=224
|
49 |
+
```
|
50 |
+
|
51 |
+
## Sample Images and Annotations
|
52 |
+
|
53 |
+
The ImageNet10 dataset contains a subset of images from the original ImageNet dataset. These images are chosen to represent the first 10 classes in the dataset, providing a diverse yet compact dataset for quick testing and evaluation.
|
54 |
+
|
55 |
+
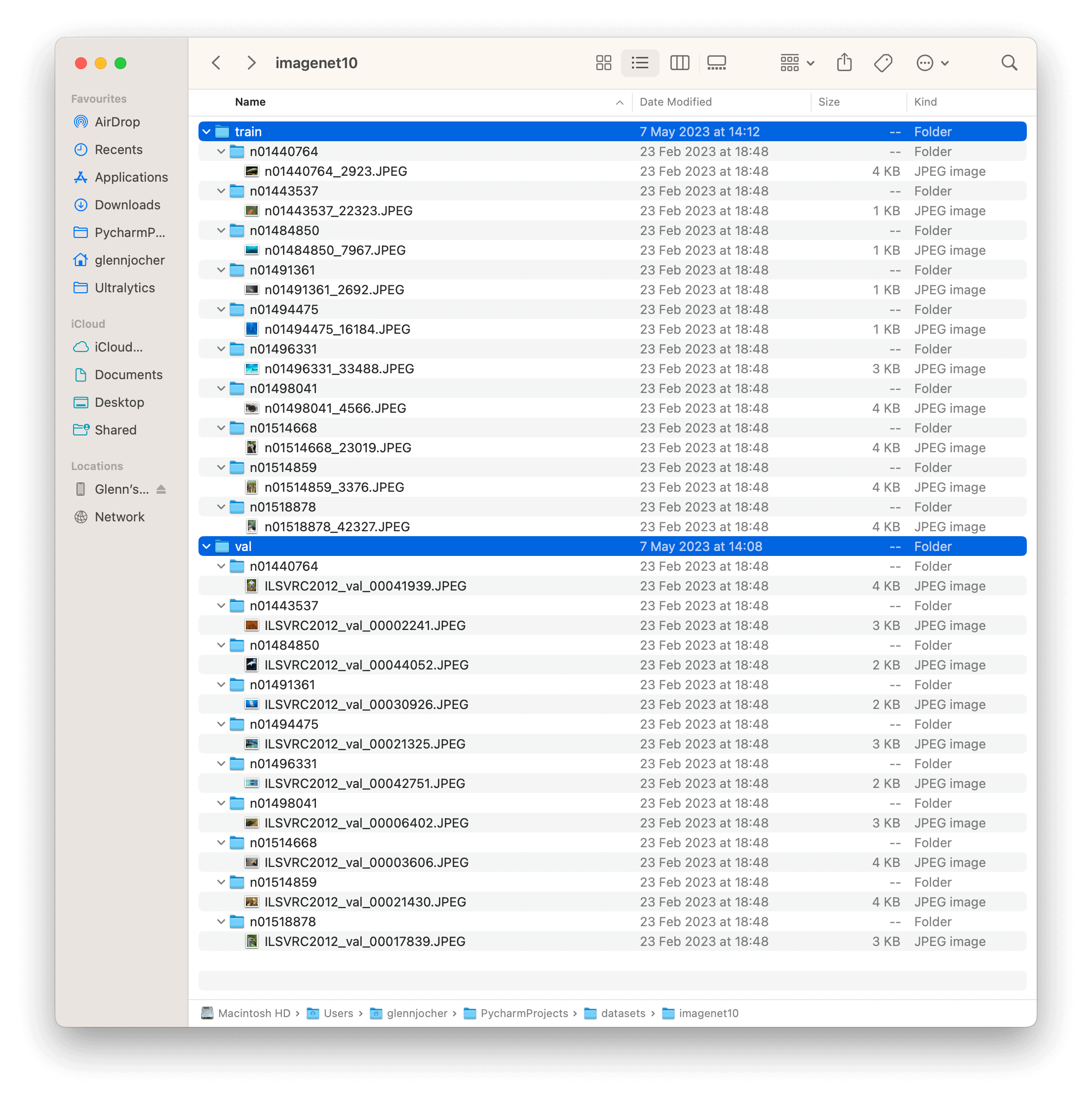
|
56 |
+
The example showcases the variety and complexity of the images in the ImageNet10 dataset, highlighting its usefulness for sanity checks and quick testing of computer vision models.
|
57 |
+
|
58 |
+
## Citations and Acknowledgments
|
59 |
+
|
60 |
+
If you use the ImageNet10 dataset in your research or development work, please cite the original ImageNet paper:
|
61 |
+
|
62 |
+
!!! note ""
|
63 |
+
|
64 |
+
=== "BibTeX"
|
65 |
+
|
66 |
+
```bibtex
|
67 |
+
@article{ILSVRC15,
|
68 |
+
author = {Olga Russakovsky and Jia Deng and Hao Su and Jonathan Krause and Sanjeev Satheesh and Sean Ma and Zhiheng Huang and Andrej Karpathy and Aditya Khosla and Michael Bernstein and Alexander C. Berg and Li Fei-Fei},
|
69 |
+
title={ImageNet Large Scale Visual Recognition Challenge},
|
70 |
+
year={2015},
|
71 |
+
journal={International Journal of Computer Vision (IJCV)},
|
72 |
+
volume={115},
|
73 |
+
number={3},
|
74 |
+
pages={211-252}
|
75 |
+
}
|
76 |
+
```
|
77 |
+
|
78 |
+
We would like to acknowledge the ImageNet team, led by Olga Russakovsky, Jia Deng, and Li Fei-Fei, for creating and maintaining the ImageNet dataset. The ImageNet10 dataset, while a compact subset, is a valuable resource for quick testing and debugging in the machine learning and computer vision research community. For more information about the ImageNet dataset and its creators, visit the [ImageNet website](https://www.image-net.org/).
|
ultralytics/docs/datasets/classify/imagenette.md
ADDED
@@ -0,0 +1,113 @@
|
|
|
|
|
|
|
|
|
|
|
|
|
|
|
|
|
|
|
|
|
|
|
|
|
|
|
|
|
|
|
|
|
|
|
|
|
|
|
|
|
|
|
|
|
|
|
|
|
|
|
|
|
|
|
|
|
|
|
|
|
|
|
|
|
|
|
|
|
|
|
|
|
|
|
|
|
|
|
|
|
|
|
|
|
|
|
|
|
|
|
|
|
|
|
|
|
|
|
|
|
|
|
|
|
|
|
|
|
|
|
|
|
|
|
|
|
|
|
|
|
|
|
|
|
|
|
|
|
|
|
|
|
|
|
|
|
|
|
|
|
|
|
|
|
|
|
|
|
|
|
|
|
|
|
|
|
|
|
|
|
|
|
|
|
|
|
|
|
|
|
|
|
|
|
|
|
|
|
|
|
|
|
|
|
|
|
|
|
|
|
|
|
|
|
|
|
|
|
|
|
|
|
|
|
|
|
|
|
|
|
|
|
|
|
|
|
|
|
|
|
|
|
|
|
|
|
|
|
1 |
+
---
|
2 |
+
comments: true
|
3 |
+
description: Learn about the ImageNette dataset and its usage in deep learning model training. Find code snippets for model training and explore ImageNette datatypes.
|
4 |
+
keywords: ImageNette dataset, Ultralytics, YOLO, Image classification, Machine Learning, Deep learning, Training code snippets, CNN, ImageNette160, ImageNette320
|
5 |
+
---
|
6 |
+
|
7 |
+
# ImageNette Dataset
|
8 |
+
|
9 |
+
The [ImageNette](https://github.com/fastai/imagenette) dataset is a subset of the larger [Imagenet](http://www.image-net.org/) dataset, but it only includes 10 easily distinguishable classes. It was created to provide a quicker, easier-to-use version of Imagenet for software development and education.
|
10 |
+
|
11 |
+
## Key Features
|
12 |
+
|
13 |
+
- ImageNette contains images from 10 different classes such as tench, English springer, cassette player, chain saw, church, French horn, garbage truck, gas pump, golf ball, parachute.
|
14 |
+
- The dataset comprises colored images of varying dimensions.
|
15 |
+
- ImageNette is widely used for training and testing in the field of machine learning, especially for image classification tasks.
|
16 |
+
|
17 |
+
## Dataset Structure
|
18 |
+
|
19 |
+
The ImageNette dataset is split into two subsets:
|
20 |
+
|
21 |
+
1. **Training Set**: This subset contains several thousands of images used for training machine learning models. The exact number varies per class.
|
22 |
+
2. **Validation Set**: This subset consists of several hundreds of images used for validating and benchmarking the trained models. Again, the exact number varies per class.
|
23 |
+
|
24 |
+
## Applications
|
25 |
+
|
26 |
+
The ImageNette dataset is widely used for training and evaluating deep learning models in image classification tasks, such as Convolutional Neural Networks (CNNs), and various other machine learning algorithms. The dataset's straightforward format and well-chosen classes make it a handy resource for both beginner and experienced practitioners in the field of machine learning and computer vision.
|
27 |
+
|
28 |
+
## Usage
|
29 |
+
|
30 |
+
To train a model on the ImageNette dataset for 100 epochs with a standard image size of 224x224, you can use the following code snippets. For a comprehensive list of available arguments, refer to the model [Training](../../modes/train.md) page.
|
31 |
+
|
32 |
+
!!! example "Train Example"
|
33 |
+
|
34 |
+
=== "Python"
|
35 |
+
|
36 |
+
```python
|
37 |
+
from ultralytics import YOLO
|
38 |
+
|
39 |
+
# Load a model
|
40 |
+
model = YOLO('yolov8n-cls.pt') # load a pretrained model (recommended for training)
|
41 |
+
|
42 |
+
# Train the model
|
43 |
+
results = model.train(data='imagenette', epochs=100, imgsz=224)
|
44 |
+
```
|
45 |
+
|
46 |
+
=== "CLI"
|
47 |
+
|
48 |
+
```bash
|
49 |
+
# Start training from a pretrained *.pt model
|
50 |
+
yolo detect train data=imagenette model=yolov8n-cls.pt epochs=100 imgsz=224
|
51 |
+
```
|
52 |
+
|
53 |
+
## Sample Images and Annotations
|
54 |
+
|
55 |
+
The ImageNette dataset contains colored images of various objects and scenes, providing a diverse dataset for image classification tasks. Here are some examples of images from the dataset:
|
56 |
+
|
57 |
+
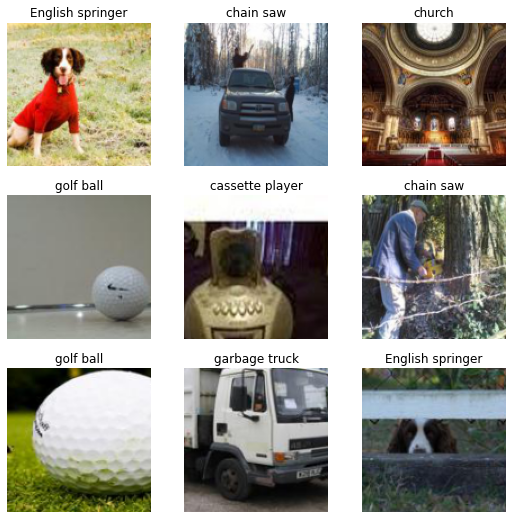
|
58 |
+
|
59 |
+
The example showcases the variety and complexity of the images in the ImageNette dataset, highlighting the importance of a diverse dataset for training robust image classification models.
|
60 |
+
|
61 |
+
## ImageNette160 and ImageNette320
|
62 |
+
|
63 |
+
For faster prototyping and training, the ImageNette dataset is also available in two reduced sizes: ImageNette160 and ImageNette320. These datasets maintain the same classes and structure as the full ImageNette dataset, but the images are resized to a smaller dimension. As such, these versions of the dataset are particularly useful for preliminary model testing, or when computational resources are limited.
|
64 |
+
|
65 |
+
To use these datasets, simply replace 'imagenette' with 'imagenette160' or 'imagenette320' in the training command. The following code snippets illustrate this:
|
66 |
+
|
67 |
+
!!! example "Train Example with ImageNette160"
|
68 |
+
|
69 |
+
=== "Python"
|
70 |
+
|
71 |
+
```python
|
72 |
+
from ultralytics import YOLO
|
73 |
+
|
74 |
+
# Load a model
|
75 |
+
model = YOLO('yolov8n-cls.pt') # load a pretrained model (recommended for training)
|
76 |
+
|
77 |
+
# Train the model with ImageNette160
|
78 |
+
results = model.train(data='imagenette160', epochs=100, imgsz=160)
|
79 |
+
```
|
80 |
+
|
81 |
+
=== "CLI"
|
82 |
+
|
83 |
+
```bash
|
84 |
+
# Start training from a pretrained *.pt model with ImageNette160
|
85 |
+
yolo detect train data=imagenette160 model=yolov8n-cls.pt epochs=100 imgsz=160
|
86 |
+
```
|
87 |
+
|
88 |
+
!!! example "Train Example with ImageNette320"
|
89 |
+
|
90 |
+
=== "Python"
|
91 |
+
|
92 |
+
```python
|
93 |
+
from ultralytics import YOLO
|
94 |
+
|
95 |
+
# Load a model
|
96 |
+
model = YOLO('yolov8n-cls.pt') # load a pretrained model (recommended for training)
|
97 |
+
|
98 |
+
# Train the model with ImageNette320
|
99 |
+
results = model.train(data='imagenette320', epochs=100, imgsz=320)
|
100 |
+
```
|
101 |
+
|
102 |
+
=== "CLI"
|
103 |
+
|
104 |
+
```bash
|
105 |
+
# Start training from a pretrained *.pt model with ImageNette320
|
106 |
+
yolo detect train data=imagenette320 model=yolov8n-cls.pt epochs=100 imgsz=320
|
107 |
+
```
|
108 |
+
|
109 |
+
These smaller versions of the dataset allow for rapid iterations during the development process while still providing valuable and realistic image classification tasks.
|
110 |
+
|
111 |
+
## Citations and Acknowledgments
|
112 |
+
|
113 |
+
If you use the ImageNette dataset in your research or development work, please acknowledge it appropriately. For more information about the ImageNette dataset, visit the [ImageNette dataset GitHub page](https://github.com/fastai/imagenette).
|
ultralytics/docs/datasets/classify/imagewoof.md
ADDED
@@ -0,0 +1,84 @@
|
|
|
|
|
|
|
|
|
|
|
|
|
|
|
|
|
|
|
|
|
|
|
|
|
|
|
|
|
|
|
|
|
|
|
|
|
|
|
|
|
|
|
|
|
|
|
|
|
|
|
|
|
|
|
|
|
|
|
|
|
|
|
|
|
|
|
|
|
|
|
|
|
|
|
|
|
|
|
|
|
|
|
|
|
|
|
|
|
|
|
|
|
|
|
|
|
|
|
|
|
|
|
|
|
|
|
|
|
|
|
|
|
|
|
|
|
|
|
|
|
|
|
|
|
|
|
|
|
|
|
|
|
|
|
|
|
|
|
|
|
|
|
|
|
|
|
|
|
|
|
|
|
|
|
|
|
|
|
|
|
|
|
|
|
|
|
|
|
|
|
1 |
+
---
|
2 |
+
comments: true
|
3 |
+
description: Explore the ImageWoof dataset, designed for challenging dog breed classification. Train AI models with Ultralytics YOLO using this dataset.
|
4 |
+
keywords: ImageWoof, image classification, dog breeds, machine learning, deep learning, Ultralytics, YOLO, dataset
|
5 |
+
---
|
6 |
+
|
7 |
+
# ImageWoof Dataset
|
8 |
+
|
9 |
+
The [ImageWoof](https://github.com/fastai/imagenette) dataset is a subset of the ImageNet consisting of 10 classes that are challenging to classify, since they're all dog breeds. It was created as a more difficult task for image classification algorithms to solve, aiming at encouraging development of more advanced models.
|
10 |
+
|
11 |
+
## Key Features
|
12 |
+
|
13 |
+
- ImageWoof contains images of 10 different dog breeds: Australian terrier, Border terrier, Samoyed, Beagle, Shih-Tzu, English foxhound, Rhodesian ridgeback, Dingo, Golden retriever, and Old English sheepdog.
|
14 |
+
- The dataset provides images at various resolutions (full size, 320px, 160px), accommodating for different computational capabilities and research needs.
|
15 |
+
- It also includes a version with noisy labels, providing a more realistic scenario where labels might not always be reliable.
|
16 |
+
|
17 |
+
## Dataset Structure
|
18 |
+
|
19 |
+
The ImageWoof dataset structure is based on the dog breed classes, with each breed having its own directory of images.
|
20 |
+
|
21 |
+
## Applications
|
22 |
+
|
23 |
+
The ImageWoof dataset is widely used for training and evaluating deep learning models in image classification tasks, especially when it comes to more complex and similar classes. The dataset's challenge lies in the subtle differences between the dog breeds, pushing the limits of model's performance and generalization.
|
24 |
+
|
25 |
+
## Usage
|
26 |
+
|
27 |
+
To train a CNN model on the ImageWoof dataset for 100 epochs with an image size of 224x224, you can use the following code snippets. For a comprehensive list of available arguments, refer to the model [Training](../../modes/train.md) page.
|
28 |
+
|
29 |
+
!!! example "Train Example"
|
30 |
+
|
31 |
+
=== "Python"
|
32 |
+
|
33 |
+
```python
|
34 |
+
from ultralytics import YOLO
|
35 |
+
|
36 |
+
# Load a model
|
37 |
+
model = YOLO('yolov8n-cls.pt') # load a pretrained model (recommended for training)
|
38 |
+
|
39 |
+
# Train the model
|
40 |
+
results = model.train(data='imagewoof', epochs=100, imgsz=224)
|
41 |
+
```
|
42 |
+
|
43 |
+
=== "CLI"
|
44 |
+
|
45 |
+
```bash
|
46 |
+
# Start training from a pretrained *.pt model
|
47 |
+
yolo detect train data=imagewoof model=yolov8n-cls.pt epochs=100 imgsz=224
|
48 |
+
```
|
49 |
+
|
50 |
+
## Dataset Variants
|
51 |
+
|
52 |
+
ImageWoof dataset comes in three different sizes to accommodate various research needs and computational capabilities:
|
53 |
+
|
54 |
+
1. **Full Size (imagewoof)**: This is the original version of the ImageWoof dataset. It contains full-sized images and is ideal for final training and performance benchmarking.
|
55 |
+
|
56 |
+
2. **Medium Size (imagewoof320)**: This version contains images resized to have a maximum edge length of 320 pixels. It's suitable for faster training without significantly sacrificing model performance.
|
57 |
+
|
58 |
+
3. **Small Size (imagewoof160)**: This version contains images resized to have a maximum edge length of 160 pixels. It's designed for rapid prototyping and experimentation where training speed is a priority.
|
59 |
+
|
60 |
+
To use these variants in your training, simply replace 'imagewoof' in the dataset argument with 'imagewoof320' or 'imagewoof160'. For example:
|
61 |
+
|
62 |
+
```python
|
63 |
+
# For medium-sized dataset
|
64 |
+
model.train(data='imagewoof320', epochs=100, imgsz=224)
|
65 |
+
|
66 |
+
# For small-sized dataset
|
67 |
+
model.train(data='imagewoof160', epochs=100, imgsz=224)
|
68 |
+
```
|
69 |
+
|
70 |
+
It's important to note that using smaller images will likely yield lower performance in terms of classification accuracy. However, it's an excellent way to iterate quickly in the early stages of model development and prototyping.
|
71 |
+
|
72 |
+
## Sample Images and Annotations
|
73 |
+
|
74 |
+
The ImageWoof dataset contains colorful images of various dog breeds, providing a challenging dataset for image classification tasks. Here are some examples of images from the dataset:
|
75 |
+
|
76 |
+
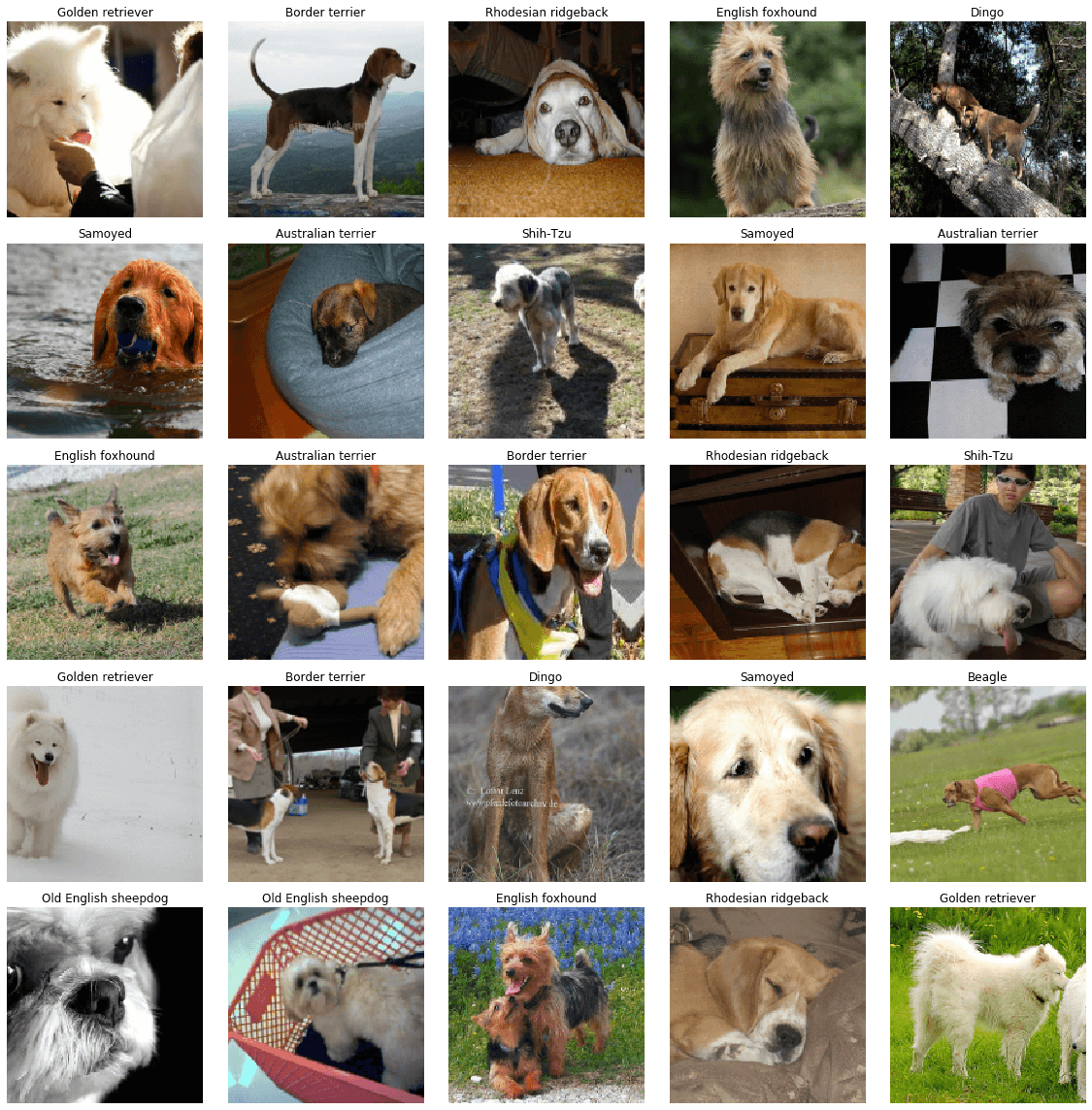
|
77 |
+
|
78 |
+
The example showcases the subtle differences and similarities among the different dog breeds in the ImageWoof dataset, highlighting the complexity and difficulty of the classification task.
|
79 |
+
|
80 |
+
## Citations and Acknowledgments
|
81 |
+
|
82 |
+
If you use the ImageWoof dataset in your research or development work, please make sure to acknowledge the creators of the dataset by linking to the [official dataset repository](https://github.com/fastai/imagenette).
|
83 |
+
|
84 |
+
We would like to acknowledge the FastAI team for creating and maintaining the ImageWoof dataset as a valuable resource for the machine learning and computer vision research community. For more information about the ImageWoof dataset, visit the [ImageWoof dataset repository](https://github.com/fastai/imagenette).
|
ultralytics/docs/datasets/classify/index.md
ADDED
@@ -0,0 +1,120 @@
|
|
|
|
|
|
|
|
|
|
|
|
|
|
|
|
|
|
|
|
|
|
|
|
|
|
|
|
|
|
|
|
|
|
|
|
|
|
|
|
|
|
|
|
|
|
|
|
|
|
|
|
|
|
|
|
|
|
|
|
|
|
|
|
|
|
|
|
|
|
|
|
|
|
|
|
|
|
|
|
|
|
|
|
|
|
|
|
|
|
|
|
|
|
|
|
|
|
|
|
|
|
|
|
|
|
|
|
|
|
|
|
|
|
|
|
|
|
|
|
|
|
|
|
|
|
|
|
|
|
|
|
|
|
|
|
|
|
|
|
|
|
|
|
|
|
|
|
|
|
|
|
|
|
|
|
|
|
|
|
|
|
|
|
|
|
|
|
|
|
|
|
|
|
|
|
|
|
|
|
|
|
|
|
|
|
|
|
|
|
|
|
|
|
|
|
|
|
|
|
|
|
|
|
|
|
|
|
|
|
|
|
|
|
|
|
|
|
|
|
|
|
|
|
|
|
|
|
|
|
|
|
|
|
|
|
|
|
|
|
|
|
|
1 |
+
---
|
2 |
+
comments: true
|
3 |
+
description: Explore image classification datasets supported by Ultralytics, learn the standard dataset format, and set up your own dataset for training models.
|
4 |
+
keywords: Ultralytics, image classification, dataset, machine learning, CIFAR-10, ImageNet, MNIST, torchvision
|
5 |
+
---
|
6 |
+
|
7 |
+
# Image Classification Datasets Overview
|
8 |
+
|
9 |
+
## Dataset format
|
10 |
+
|
11 |
+
The folder structure for classification datasets in torchvision typically follows a standard format:
|
12 |
+
|
13 |
+
```
|
14 |
+
root/
|
15 |
+
|-- class1/
|
16 |
+
| |-- img1.jpg
|
17 |
+
| |-- img2.jpg
|
18 |
+
| |-- ...
|
19 |
+
|
|
20 |
+
|-- class2/
|
21 |
+
| |-- img1.jpg
|
22 |
+
| |-- img2.jpg
|
23 |
+
| |-- ...
|
24 |
+
|
|
25 |
+
|-- class3/
|
26 |
+
| |-- img1.jpg
|
27 |
+
| |-- img2.jpg
|
28 |
+
| |-- ...
|
29 |
+
|
|
30 |
+
|-- ...
|
31 |
+
```
|
32 |
+
|
33 |
+
In this folder structure, the `root` directory contains one subdirectory for each class in the dataset. Each subdirectory is named after the corresponding class and contains all the images for that class. Each image file is named uniquely and is typically in a common image file format such as JPEG or PNG.
|
34 |
+
|
35 |
+
** Example **
|
36 |
+
|
37 |
+
For example, in the CIFAR10 dataset, the folder structure would look like this:
|
38 |
+
|
39 |
+
```
|
40 |
+
cifar-10-/
|
41 |
+
|
|
42 |
+
|-- train/
|
43 |
+
| |-- airplane/
|
44 |
+
| | |-- 10008_airplane.png
|
45 |
+
| | |-- 10009_airplane.png
|
46 |
+
| | |-- ...
|
47 |
+
| |
|
48 |
+
| |-- automobile/
|
49 |
+
| | |-- 1000_automobile.png
|
50 |
+
| | |-- 1001_automobile.png
|
51 |
+
| | |-- ...
|
52 |
+
| |
|
53 |
+
| |-- bird/
|
54 |
+
| | |-- 10014_bird.png
|
55 |
+
| | |-- 10015_bird.png
|
56 |
+
| | |-- ...
|
57 |
+
| |
|
58 |
+
| |-- ...
|
59 |
+
|
|
60 |
+
|-- test/
|
61 |
+
| |-- airplane/
|
62 |
+
| | |-- 10_airplane.png
|
63 |
+
| | |-- 11_airplane.png
|
64 |
+
| | |-- ...
|
65 |
+
| |
|
66 |
+
| |-- automobile/
|
67 |
+
| | |-- 100_automobile.png
|
68 |
+
| | |-- 101_automobile.png
|
69 |
+
| | |-- ...
|
70 |
+
| |
|
71 |
+
| |-- bird/
|
72 |
+
| | |-- 1000_bird.png
|
73 |
+
| | |-- 1001_bird.png
|
74 |
+
| | |-- ...
|
75 |
+
| |
|
76 |
+
| |-- ...
|
77 |
+
```
|
78 |
+
|
79 |
+
In this example, the `train` directory contains subdirectories for each class in the dataset, and each class subdirectory contains all the images for that class. The `test` directory has a similar structure. The `root` directory also contains other files that are part of the CIFAR10 dataset.
|
80 |
+
|
81 |
+
## Usage
|
82 |
+
|
83 |
+
!!! example ""
|
84 |
+
|
85 |
+
=== "Python"
|
86 |
+
|
87 |
+
```python
|
88 |
+
from ultralytics import YOLO
|
89 |
+
|
90 |
+
# Load a model
|
91 |
+
model = YOLO('yolov8n-cls.pt') # load a pretrained model (recommended for training)
|
92 |
+
|
93 |
+
# Train the model
|
94 |
+
results = model.train(data='path/to/dataset', epochs=100, imgsz=640)
|
95 |
+
```
|
96 |
+
=== "CLI"
|
97 |
+
|
98 |
+
```bash
|
99 |
+
# Start training from a pretrained *.pt model
|
100 |
+
yolo detect train data=path/to/data model=yolov8n-cls.pt epochs=100 imgsz=640
|
101 |
+
```
|
102 |
+
|
103 |
+
## Supported Datasets
|
104 |
+
|
105 |
+
Ultralytics supports the following datasets with automatic download:
|
106 |
+
|
107 |
+
* [Caltech 101](caltech101.md): A dataset containing images of 101 object categories for image classification tasks.
|
108 |
+
* [Caltech 256](caltech256.md): An extended version of Caltech 101 with 256 object categories and more challenging images.
|
109 |
+
* [CIFAR-10](cifar10.md): A dataset of 60K 32x32 color images in 10 classes, with 6K images per class.
|
110 |
+
* [CIFAR-100](cifar100.md): An extended version of CIFAR-10 with 100 object categories and 600 images per class.
|
111 |
+
* [Fashion-MNIST](fashion-mnist.md): A dataset consisting of 70,000 grayscale images of 10 fashion categories for image classification tasks.
|
112 |
+
* [ImageNet](imagenet.md): A large-scale dataset for object detection and image classification with over 14 million images and 20,000 categories.
|
113 |
+
* [ImageNet-10](imagenet10.md): A smaller subset of ImageNet with 10 categories for faster experimentation and testing.
|
114 |
+
* [Imagenette](imagenette.md): A smaller subset of ImageNet that contains 10 easily distinguishable classes for quicker training and testing.
|
115 |
+
* [Imagewoof](imagewoof.md): A more challenging subset of ImageNet containing 10 dog breed categories for image classification tasks.
|
116 |
+
* [MNIST](mnist.md): A dataset of 70,000 grayscale images of handwritten digits for image classification tasks.
|
117 |
+
|
118 |
+
### Adding your own dataset
|
119 |
+
|
120 |
+
If you have your own dataset and would like to use it for training classification models with Ultralytics, ensure that it follows the format specified above under "Dataset format" and then point your `data` argument to the dataset directory.
|
ultralytics/docs/datasets/classify/mnist.md
ADDED
@@ -0,0 +1,86 @@
|
|
|
|
|
|
|
|
|
|
|
|
|
|
|
|
|
|
|
|
|
|
|
|
|
|
|
|
|
|
|
|
|
|
|
|
|
|
|
|
|
|
|
|
|
|
|
|
|
|
|
|
|
|
|
|
|
|
|
|
|
|
|
|
|
|
|
|
|
|
|
|
|
|
|
|
|
|
|
|
|
|
|
|
|
|
|
|
|
|
|
|
|
|
|
|
|
|
|
|
|
|
|
|
|
|
|
|
|
|
|
|
|
|
|
|
|
|
|
|
|
|
|
|
|
|
|
|
|
|
|
|
|
|
|
|
|
|
|
|
|
|
|
|
|
|
|
|
|
|
|
|
|
|
|
|
|
|
|
|
|
|
|
|
|
|
|
|
|
|
|
|
|
|
|
1 |
+
---
|
2 |
+
comments: true
|
3 |
+
description: Detailed guide on the MNIST Dataset, a benchmark in the machine learning community for image classification tasks. Learn about its structure, usage and application.
|
4 |
+
keywords: MNIST dataset, Ultralytics, image classification, machine learning, computer vision, deep learning, AI, dataset guide
|
5 |
+
---
|
6 |
+
|
7 |
+
# MNIST Dataset
|
8 |
+
|
9 |
+
The [MNIST](http://yann.lecun.com/exdb/mnist/) (Modified National Institute of Standards and Technology) dataset is a large database of handwritten digits that is commonly used for training various image processing systems and machine learning models. It was created by "re-mixing" the samples from NIST's original datasets and has become a benchmark for evaluating the performance of image classification algorithms.
|
10 |
+
|
11 |
+
## Key Features
|
12 |
+
|
13 |
+
- MNIST contains 60,000 training images and 10,000 testing images of handwritten digits.
|
14 |
+
- The dataset comprises grayscale images of size 28x28 pixels.
|
15 |
+
- The images are normalized to fit into a 28x28 pixel bounding box and anti-aliased, introducing grayscale levels.
|
16 |
+
- MNIST is widely used for training and testing in the field of machine learning, especially for image classification tasks.
|
17 |
+
|
18 |
+
## Dataset Structure
|
19 |
+
|
20 |
+
The MNIST dataset is split into two subsets:
|
21 |
+
|
22 |
+
1. **Training Set**: This subset contains 60,000 images of handwritten digits used for training machine learning models.
|
23 |
+
2. **Testing Set**: This subset consists of 10,000 images used for testing and benchmarking the trained models.
|
24 |
+
|
25 |
+
## Extended MNIST (EMNIST)
|
26 |
+
|
27 |
+
Extended MNIST (EMNIST) is a newer dataset developed and released by NIST to be the successor to MNIST. While MNIST included images only of handwritten digits, EMNIST includes all the images from NIST Special Database 19, which is a large database of handwritten uppercase and lowercase letters as well as digits. The images in EMNIST were converted into the same 28x28 pixel format, by the same process, as were the MNIST images. Accordingly, tools that work with the older, smaller MNIST dataset will likely work unmodified with EMNIST.
|
28 |
+
|
29 |
+
## Applications
|
30 |
+
|
31 |
+
The MNIST dataset is widely used for training and evaluating deep learning models in image classification tasks, such as Convolutional Neural Networks (CNNs), Support Vector Machines (SVMs), and various other machine learning algorithms. The dataset's simple and well-structured format makes it an essential resource for researchers and practitioners in the field of machine learning and computer vision.
|
32 |
+
|
33 |
+
## Usage
|
34 |
+
|
35 |
+
To train a CNN model on the MNIST dataset for 100 epochs with an image size of 32x32, you can use the following code snippets. For a comprehensive list of available arguments, refer to the model [Training](../../modes/train.md) page.
|
36 |
+
|
37 |
+
!!! example "Train Example"
|
38 |
+
|
39 |
+
=== "Python"
|
40 |
+
|
41 |
+
```python
|
42 |
+
from ultralytics import YOLO
|
43 |
+
|
44 |
+
# Load a model
|
45 |
+
model = YOLO('yolov8n-cls.pt') # load a pretrained model (recommended for training)
|
46 |
+
|
47 |
+
# Train the model
|
48 |
+
results = model.train(data='mnist', epochs=100, imgsz=32)
|
49 |
+
```
|
50 |
+
|
51 |
+
=== "CLI"
|
52 |
+
|
53 |
+
```bash
|
54 |
+
# Start training from a pretrained *.pt model
|
55 |
+
cnn detect train data=mnist model=yolov8n-cls.pt epochs=100 imgsz=28
|
56 |
+
```
|
57 |
+
|
58 |
+
## Sample Images and Annotations
|
59 |
+
|
60 |
+
The MNIST dataset contains grayscale images of handwritten digits, providing a well-structured dataset for image classification tasks. Here are some examples of images from the dataset:
|
61 |
+
|
62 |
+
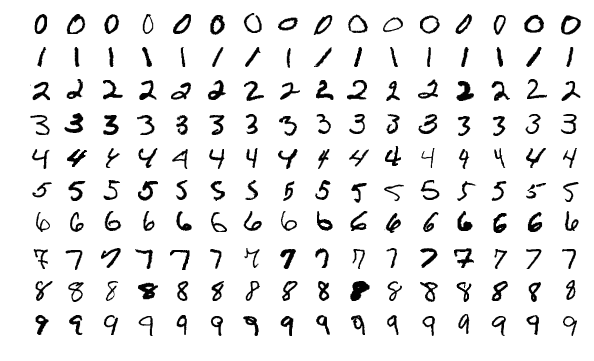
|
63 |
+
|
64 |
+
The example showcases the variety and complexity of the handwritten digits in the MNIST dataset, highlighting the importance of a diverse dataset for training robust image classification models.
|
65 |
+
|
66 |
+
## Citations and Acknowledgments
|
67 |
+
|
68 |
+
If you use the MNIST dataset in your
|
69 |
+
|
70 |
+
research or development work, please cite the following paper:
|
71 |
+
|
72 |
+
!!! note ""
|
73 |
+
|
74 |
+
=== "BibTeX"
|
75 |
+
|
76 |
+
```bibtex
|
77 |
+
@article{lecun2010mnist,
|
78 |
+
title={MNIST handwritten digit database},
|
79 |
+
author={LeCun, Yann and Cortes, Corinna and Burges, CJ},
|
80 |
+
journal={ATT Labs [Online]. Available: http://yann.lecun.com/exdb/mnist},
|
81 |
+
volume={2},
|
82 |
+
year={2010}
|
83 |
+
}
|
84 |
+
```
|
85 |
+
|
86 |
+
We would like to acknowledge Yann LeCun, Corinna Cortes, and Christopher J.C. Burges for creating and maintaining the MNIST dataset as a valuable resource for the machine learning and computer vision research community. For more information about the MNIST dataset and its creators, visit the [MNIST dataset website](http://yann.lecun.com/exdb/mnist/).
|
ultralytics/docs/datasets/detect/argoverse.md
ADDED
@@ -0,0 +1,97 @@
|
|
|
|
|
|
|
|
|
|
|
|
|
|
|
|
|
|
|
|
|
|
|
|
|
|
|
|
|
|
|
|
|
|
|
|
|
|
|
|
|
|
|
|
|
|
|
|
|
|
|
|
|
|
|
|
|
|
|
|
|
|
|
|
|
|
|
|
|
|
|
|
|
|
|
|
|
|
|
|
|
|
|
|
|
|
|
|
|
|
|
|
|
|
|
|
|
|
|
|
|
|
|
|
|
|
|
|
|
|
|
|
|
|
|
|
|
|
|
|
|
|
|
|
|
|
|
|
|
|
|
|
|
|
|
|
|
|
|
|
|
|
|
|
|
|
|
|
|
|
|
|
|
|
|
|
|
|
|
|
|
|
|
|
|
|
|
|
|
|
|
|
|
|
|
|
|
|
|
|
|
|
|
|
|
|
|
|
|
|
|
|
|
|
|
|
|
1 |
+
---
|
2 |
+
comments: true
|
3 |
+
description: Explore Argoverse, a comprehensive dataset for autonomous driving tasks including 3D tracking, motion forecasting and depth estimation used in YOLO.
|
4 |
+
keywords: Argoverse dataset, autonomous driving, YOLO, 3D tracking, motion forecasting, LiDAR data, HD maps, ultralytics documentation
|
5 |
+
---
|
6 |
+
|
7 |
+
# Argoverse Dataset
|
8 |
+
|
9 |
+
The [Argoverse](https://www.argoverse.org/) dataset is a collection of data designed to support research in autonomous driving tasks, such as 3D tracking, motion forecasting, and stereo depth estimation. Developed by Argo AI, the dataset provides a wide range of high-quality sensor data, including high-resolution images, LiDAR point clouds, and map data.
|
10 |
+
|
11 |
+
!!! note
|
12 |
+
|
13 |
+
The Argoverse dataset *.zip file required for training was removed from Amazon S3 after the shutdown of Argo AI by Ford, but we have made it available for manual download on [Google Drive](https://drive.google.com/file/d/1st9qW3BeIwQsnR0t8mRpvbsSWIo16ACi/view?usp=drive_link).
|
14 |
+
|
15 |
+
## Key Features
|
16 |
+
|
17 |
+
- Argoverse contains over 290K labeled 3D object tracks and 5 million object instances across 1,263 distinct scenes.
|
18 |
+
- The dataset includes high-resolution camera images, LiDAR point clouds, and richly annotated HD maps.
|
19 |
+
- Annotations include 3D bounding boxes for objects, object tracks, and trajectory information.
|
20 |
+
- Argoverse provides multiple subsets for different tasks, such as 3D tracking, motion forecasting, and stereo depth estimation.
|
21 |
+
|
22 |
+
## Dataset Structure
|
23 |
+
|
24 |
+
The Argoverse dataset is organized into three main subsets:
|
25 |
+
|
26 |
+
1. **Argoverse 3D Tracking**: This subset contains 113 scenes with over 290K labeled 3D object tracks, focusing on 3D object tracking tasks. It includes LiDAR point clouds, camera images, and sensor calibration information.
|
27 |
+
2. **Argoverse Motion Forecasting**: This subset consists of 324K vehicle trajectories collected from 60 hours of driving data, suitable for motion forecasting tasks.
|
28 |
+
3. **Argoverse Stereo Depth Estimation**: This subset is designed for stereo depth estimation tasks and includes over 10K stereo image pairs with corresponding LiDAR point clouds for ground truth depth estimation.
|
29 |
+
|
30 |
+
## Applications
|
31 |
+
|
32 |
+
The Argoverse dataset is widely used for training and evaluating deep learning models in autonomous driving tasks such as 3D object tracking, motion forecasting, and stereo depth estimation. The dataset's diverse set of sensor data, object annotations, and map information make it a valuable resource for researchers and practitioners in the field of autonomous driving.
|
33 |
+
|
34 |
+
## Dataset YAML
|
35 |
+
|
36 |
+
A YAML (Yet Another Markup Language) file is used to define the dataset configuration. It contains information about the dataset's paths, classes, and other relevant information. For the case of the Argoverse dataset, the `Argoverse.yaml` file is maintained at [https://github.com/ultralytics/ultralytics/blob/main/ultralytics/cfg/datasets/Argoverse.yaml](https://github.com/ultralytics/ultralytics/blob/main/ultralytics/cfg/datasets/Argoverse.yaml).
|
37 |
+
|
38 |
+
!!! example "ultralytics/cfg/datasets/Argoverse.yaml"
|
39 |
+
|
40 |
+
```yaml
|
41 |
+
--8<-- "ultralytics/cfg/datasets/Argoverse.yaml"
|
42 |
+
```
|
43 |
+
|
44 |
+
## Usage
|
45 |
+
|
46 |
+
To train a YOLOv8n model on the Argoverse dataset for 100 epochs with an image size of 640, you can use the following code snippets. For a comprehensive list of available arguments, refer to the model [Training](../../modes/train.md) page.
|
47 |
+
|
48 |
+
!!! example "Train Example"
|
49 |
+
|
50 |
+
=== "Python"
|
51 |
+
|
52 |
+
```python
|
53 |
+
from ultralytics import YOLO
|
54 |
+
|
55 |
+
# Load a model
|
56 |
+
model = YOLO('yolov8n.pt') # load a pretrained model (recommended for training)
|
57 |
+
|
58 |
+
# Train the model
|
59 |
+
results = model.train(data='Argoverse.yaml', epochs=100, imgsz=640)
|
60 |
+
```
|
61 |
+
|
62 |
+
=== "CLI"
|
63 |
+
|
64 |
+
```bash
|
65 |
+
# Start training from a pretrained *.pt model
|
66 |
+
yolo detect train data=Argoverse.yaml model=yolov8n.pt epochs=100 imgsz=640
|
67 |
+
```
|
68 |
+
|
69 |
+
## Sample Data and Annotations
|
70 |
+
|
71 |
+
The Argoverse dataset contains a diverse set of sensor data, including camera images, LiDAR point clouds, and HD map information, providing rich context for autonomous driving tasks. Here are some examples of data from the dataset, along with their corresponding annotations:
|
72 |
+
|
73 |
+
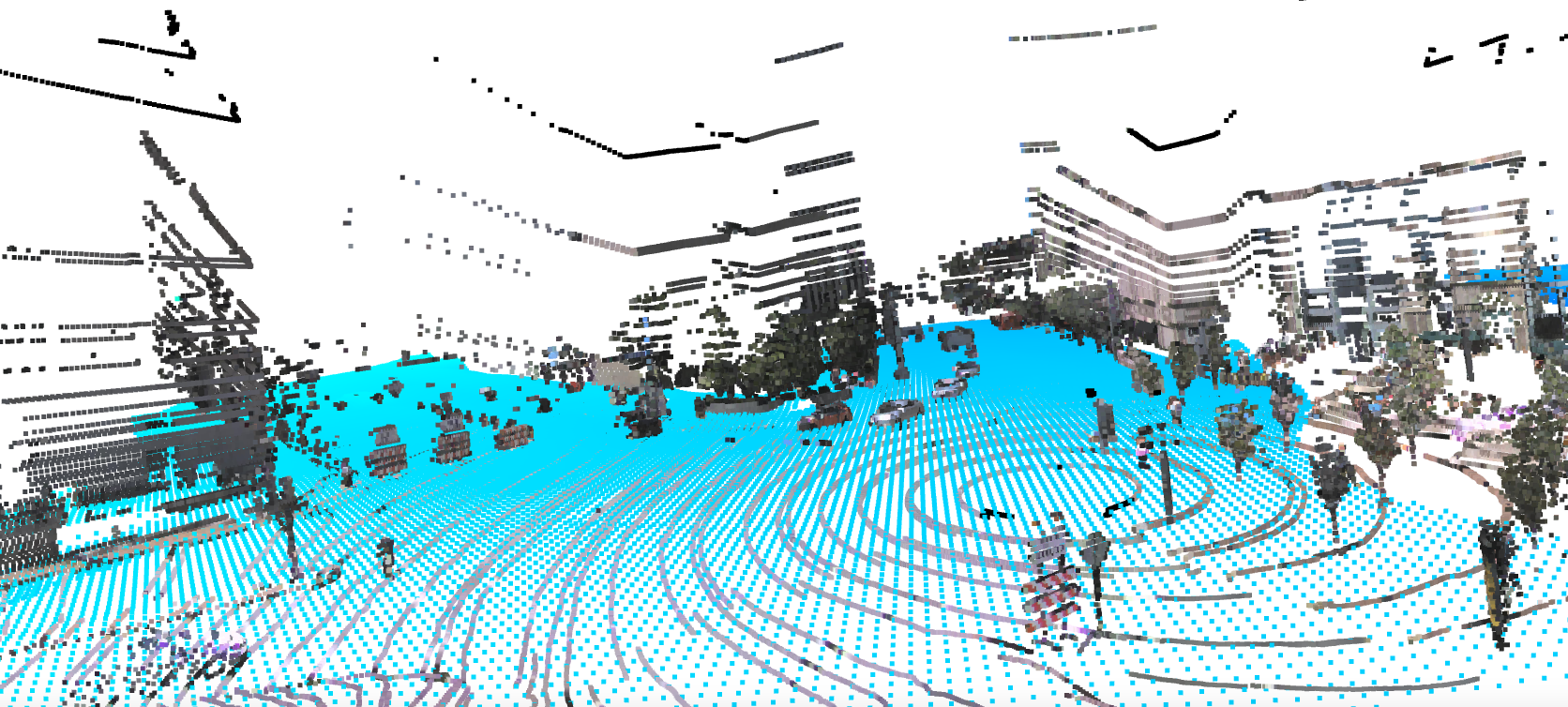
|
74 |
+
|
75 |
+
- **Argoverse 3D Tracking**: This image demonstrates an example of 3D object tracking, where objects are annotated with 3D bounding boxes. The dataset provides LiDAR point clouds and camera images to facilitate the development of models for this task.
|
76 |
+
|
77 |
+
The example showcases the variety and complexity of the data in the Argoverse dataset and highlights the importance of high-quality sensor data for autonomous driving tasks.
|
78 |
+
|
79 |
+
## Citations and Acknowledgments
|
80 |
+
|
81 |
+
If you use the Argoverse dataset in your research or development work, please cite the following paper:
|
82 |
+
|
83 |
+
!!! note ""
|
84 |
+
|
85 |
+
=== "BibTeX"
|
86 |
+
|
87 |
+
```bibtex
|
88 |
+
@inproceedings{chang2019argoverse,
|
89 |
+
title={Argoverse: 3D Tracking and Forecasting with Rich Maps},
|
90 |
+
author={Chang, Ming-Fang and Lambert, John and Sangkloy, Patsorn and Singh, Jagjeet and Bak, Slawomir and Hartnett, Andrew and Wang, Dequan and Carr, Peter and Lucey, Simon and Ramanan, Deva and others},
|
91 |
+
booktitle={Proceedings of the IEEE/CVF Conference on Computer Vision and Pattern Recognition},
|
92 |
+
pages={8748--8757},
|
93 |
+
year={2019}
|
94 |
+
}
|
95 |
+
```
|
96 |
+
|
97 |
+
We would like to acknowledge Argo AI for creating and maintaining the Argoverse dataset as a valuable resource for the autonomous driving research community. For more information about the Argoverse dataset and its creators, visit the [Argoverse dataset website](https://www.argoverse.org/).
|
ultralytics/docs/datasets/detect/coco.md
ADDED
@@ -0,0 +1,94 @@
|
|
|
|
|
|
|
|
|
|
|
|
|
|
|
|
|
|
|
|
|
|
|
|
|
|
|
|
|
|
|
|
|
|
|
|
|
|
|
|
|
|
|
|
|
|
|
|
|
|
|
|
|
|
|
|
|
|
|
|
|
|
|
|
|
|
|
|
|
|
|
|
|
|
|
|
|
|
|
|
|
|
|
|
|
|
|
|
|
|
|
|
|
|
|
|
|
|
|
|
|
|
|
|
|
|
|
|
|
|
|
|
|
|
|
|
|
|
|
|
|
|
|
|
|
|
|
|
|
|
|
|
|
|
|
|
|
|
|
|
|
|
|
|
|
|
|
|
|
|
|
|
|
|
|
|
|
|
|
|
|
|
|
|
|
|
|
|
|
|
|
|
|
|
|
|
|
|
|
|
|
|
|
|
|
|
|
|
|
|
|
1 |
+
---
|
2 |
+
comments: true
|
3 |
+
description: Learn how COCO, a leading dataset for object detection and segmentation, integrates with Ultralytics. Discover ways to use it for training YOLO models.
|
4 |
+
keywords: Ultralytics, COCO dataset, object detection, YOLO, YOLO model training, image segmentation, computer vision, deep learning models
|
5 |
+
---
|
6 |
+
|
7 |
+
# COCO Dataset
|
8 |
+
|
9 |
+
The [COCO](https://cocodataset.org/#home) (Common Objects in Context) dataset is a large-scale object detection, segmentation, and captioning dataset. It is designed to encourage research on a wide variety of object categories and is commonly used for benchmarking computer vision models. It is an essential dataset for researchers and developers working on object detection, segmentation, and pose estimation tasks.
|
10 |
+
|
11 |
+
## Key Features
|
12 |
+
|
13 |
+
- COCO contains 330K images, with 200K images having annotations for object detection, segmentation, and captioning tasks.
|
14 |
+
- The dataset comprises 80 object categories, including common objects like cars, bicycles, and animals, as well as more specific categories such as umbrellas, handbags, and sports equipment.
|
15 |
+
- Annotations include object bounding boxes, segmentation masks, and captions for each image.
|
16 |
+
- COCO provides standardized evaluation metrics like mean Average Precision (mAP) for object detection, and mean Average Recall (mAR) for segmentation tasks, making it suitable for comparing model performance.
|
17 |
+
|
18 |
+
## Dataset Structure
|
19 |
+
|
20 |
+
The COCO dataset is split into three subsets:
|
21 |
+
|
22 |
+
1. **Train2017**: This subset contains 118K images for training object detection, segmentation, and captioning models.
|
23 |
+
2. **Val2017**: This subset has 5K images used for validation purposes during model training.
|
24 |
+
3. **Test2017**: This subset consists of 20K images used for testing and benchmarking the trained models. Ground truth annotations for this subset are not publicly available, and the results are submitted to the [COCO evaluation server](https://codalab.lisn.upsaclay.fr/competitions/7384) for performance evaluation.
|
25 |
+
|
26 |
+
## Applications
|
27 |
+
|
28 |
+
The COCO dataset is widely used for training and evaluating deep learning models in object detection (such as YOLO, Faster R-CNN, and SSD), instance segmentation (such as Mask R-CNN), and keypoint detection (such as OpenPose). The dataset's diverse set of object categories, large number of annotated images, and standardized evaluation metrics make it an essential resource for computer vision researchers and practitioners.
|
29 |
+
|
30 |
+
## Dataset YAML
|
31 |
+
|
32 |
+
A YAML (Yet Another Markup Language) file is used to define the dataset configuration. It contains information about the dataset's paths, classes, and other relevant information. In the case of the COCO dataset, the `coco.yaml` file is maintained at [https://github.com/ultralytics/ultralytics/blob/main/ultralytics/cfg/datasets/coco.yaml](https://github.com/ultralytics/ultralytics/blob/main/ultralytics/cfg/datasets/coco.yaml).
|
33 |
+
|
34 |
+
!!! example "ultralytics/cfg/datasets/coco.yaml"
|
35 |
+
|
36 |
+
```yaml
|
37 |
+
--8<-- "ultralytics/cfg/datasets/coco.yaml"
|
38 |
+
```
|
39 |
+
|
40 |
+
## Usage
|
41 |
+
|
42 |
+
To train a YOLOv8n model on the COCO dataset for 100 epochs with an image size of 640, you can use the following code snippets. For a comprehensive list of available arguments, refer to the model [Training](../../modes/train.md) page.
|
43 |
+
|
44 |
+
!!! example "Train Example"
|
45 |
+
|
46 |
+
=== "Python"
|
47 |
+
|
48 |
+
```python
|
49 |
+
from ultralytics import YOLO
|
50 |
+
|
51 |
+
# Load a model
|
52 |
+
model = YOLO('yolov8n.pt') # load a pretrained model (recommended for training)
|
53 |
+
|
54 |
+
# Train the model
|
55 |
+
results = model.train(data='coco.yaml', epochs=100, imgsz=640)
|
56 |
+
```
|
57 |
+
|
58 |
+
=== "CLI"
|
59 |
+
|
60 |
+
```bash
|
61 |
+
# Start training from a pretrained *.pt model
|
62 |
+
yolo detect train data=coco.yaml model=yolov8n.pt epochs=100 imgsz=640
|
63 |
+
```
|
64 |
+
|
65 |
+
## Sample Images and Annotations
|
66 |
+
|
67 |
+
The COCO dataset contains a diverse set of images with various object categories and complex scenes. Here are some examples of images from the dataset, along with their corresponding annotations:
|
68 |
+
|
69 |
+
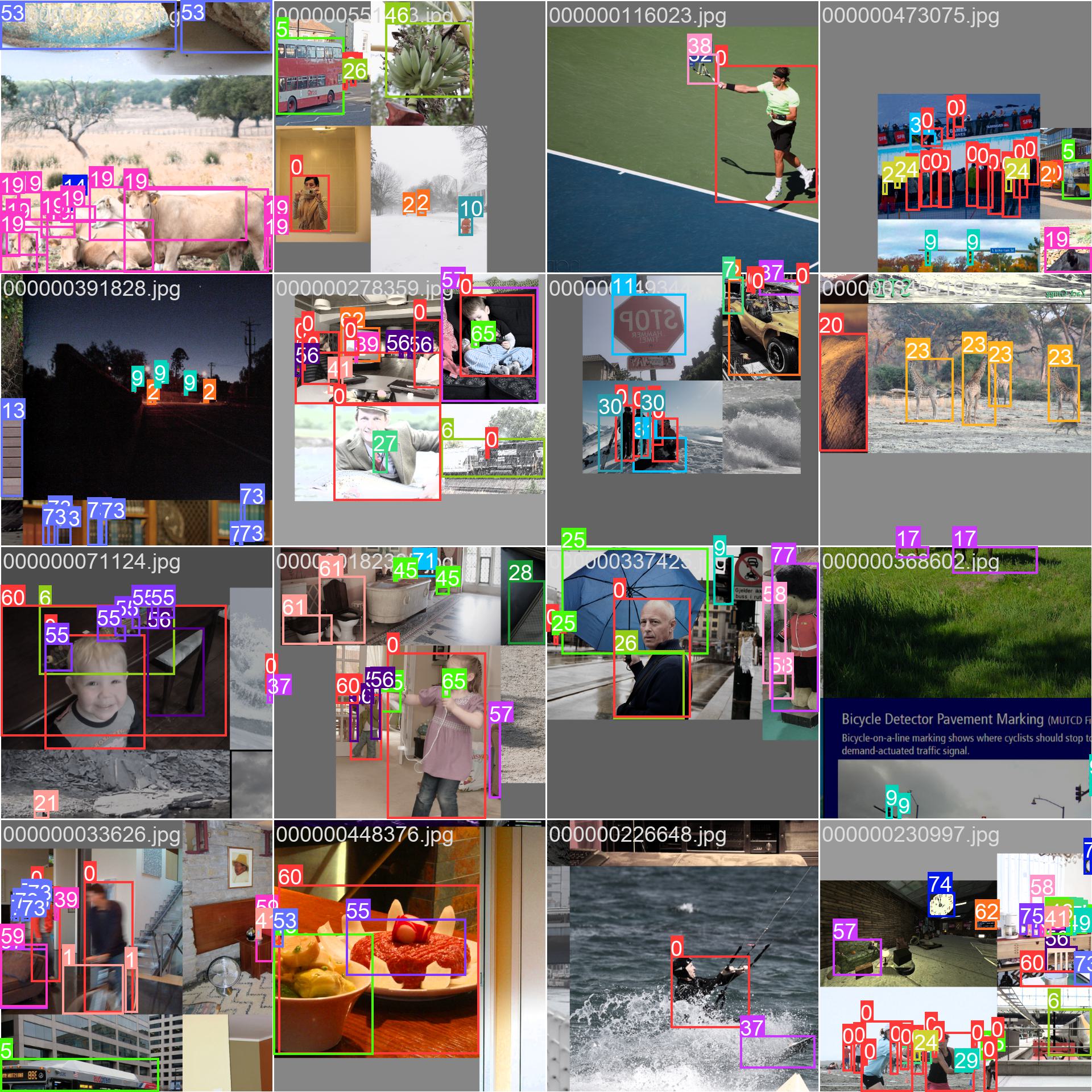
|
70 |
+
|
71 |
+
- **Mosaiced Image**: This image demonstrates a training batch composed of mosaiced dataset images. Mosaicing is a technique used during training that combines multiple images into a single image to increase the variety of objects and scenes within each training batch. This helps improve the model's ability to generalize to different object sizes, aspect ratios, and contexts.
|
72 |
+
|
73 |
+
The example showcases the variety and complexity of the images in the COCO dataset and the benefits of using mosaicing during the training process.
|
74 |
+
|
75 |
+
## Citations and Acknowledgments
|
76 |
+
|
77 |
+
If you use the COCO dataset in your research or development work, please cite the following paper:
|
78 |
+
|
79 |
+
!!! note ""
|
80 |
+
|
81 |
+
=== "BibTeX"
|
82 |
+
|
83 |
+
```bibtex
|
84 |
+
@misc{lin2015microsoft,
|
85 |
+
title={Microsoft COCO: Common Objects in Context},
|
86 |
+
author={Tsung-Yi Lin and Michael Maire and Serge Belongie and Lubomir Bourdev and Ross Girshick and James Hays and Pietro Perona and Deva Ramanan and C. Lawrence Zitnick and Piotr Dollár},
|
87 |
+
year={2015},
|
88 |
+
eprint={1405.0312},
|
89 |
+
archivePrefix={arXiv},
|
90 |
+
primaryClass={cs.CV}
|
91 |
+
}
|
92 |
+
```
|
93 |
+
|
94 |
+
We would like to acknowledge the COCO Consortium for creating and maintaining this valuable resource for the computer vision community. For more information about the COCO dataset and its creators, visit the [COCO dataset website](https://cocodataset.org/#home).
|
ultralytics/docs/datasets/detect/coco8.md
ADDED
@@ -0,0 +1,80 @@
|
|
|
|
|
|
|
|
|
|
|
|
|
|
|
|
|
|
|
|
|
|
|
|
|
|
|
|
|
|
|
|
|
|
|
|
|
|
|
|
|
|
|
|
|
|
|
|
|
|
|
|
|
|
|
|
|
|
|
|
|
|
|
|
|
|
|
|
|
|
|
|
|
|
|
|
|
|
|
|
|
|
|
|
|
|
|
|
|
|
|
|
|
|
|
|
|
|
|
|
|
|
|
|
|
|
|
|
|
|
|
|
|
|
|
|
|
|
|
|
|
|
|
|
|
|
|
|
|
|
|
|
|
|
|
|
|
|
|
|
|
|
|
|
|
|
|
|
|
|
|
|
|
|
|
|
|
|
|
|
|
|
|
1 |
+
---
|
2 |
+
comments: true
|
3 |
+
description: Discover the benefits of using the practical and diverse COCO8 dataset for object detection model testing. Learn to configure and use it via Ultralytics HUB and YOLOv8.
|
4 |
+
keywords: Ultralytics, COCO8 dataset, object detection, model testing, dataset configuration, detection approaches, sanity check, training pipelines, YOLOv8
|
5 |
+
---
|
6 |
+
|
7 |
+
# COCO8 Dataset
|
8 |
+
|
9 |
+
## Introduction
|
10 |
+
|
11 |
+
[Ultralytics](https://ultralytics.com) COCO8 is a small, but versatile object detection dataset composed of the first 8 images of the COCO train 2017 set, 4 for training and 4 for validation. This dataset is ideal for testing and debugging object detection models, or for experimenting with new detection approaches. With 8 images, it is small enough to be easily manageable, yet diverse enough to test training pipelines for errors and act as a sanity check before training larger datasets.
|
12 |
+
|
13 |
+
This dataset is intended for use with Ultralytics [HUB](https://hub.ultralytics.com)
|
14 |
+
and [YOLOv8](https://github.com/ultralytics/ultralytics).
|
15 |
+
|
16 |
+
## Dataset YAML
|
17 |
+
|
18 |
+
A YAML (Yet Another Markup Language) file is used to define the dataset configuration. It contains information about the dataset's paths, classes, and other relevant information. In the case of the COCO8 dataset, the `coco8.yaml` file is maintained at [https://github.com/ultralytics/ultralytics/blob/main/ultralytics/cfg/datasets/coco8.yaml](https://github.com/ultralytics/ultralytics/blob/main/ultralytics/cfg/datasets/coco8.yaml).
|
19 |
+
|
20 |
+
!!! example "ultralytics/cfg/datasets/coco8.yaml"
|
21 |
+
|
22 |
+
```yaml
|
23 |
+
--8<-- "ultralytics/cfg/datasets/coco8.yaml"
|
24 |
+
```
|
25 |
+
|
26 |
+
## Usage
|
27 |
+
|
28 |
+
To train a YOLOv8n model on the COCO8 dataset for 100 epochs with an image size of 640, you can use the following code snippets. For a comprehensive list of available arguments, refer to the model [Training](../../modes/train.md) page.
|
29 |
+
|
30 |
+
!!! example "Train Example"
|
31 |
+
|
32 |
+
=== "Python"
|
33 |
+
|
34 |
+
```python
|
35 |
+
from ultralytics import YOLO
|
36 |
+
|
37 |
+
# Load a model
|
38 |
+
model = YOLO('yolov8n.pt') # load a pretrained model (recommended for training)
|
39 |
+
|
40 |
+
# Train the model
|
41 |
+
results = model.train(data='coco8.yaml', epochs=100, imgsz=640)
|
42 |
+
```
|
43 |
+
|
44 |
+
=== "CLI"
|
45 |
+
|
46 |
+
```bash
|
47 |
+
# Start training from a pretrained *.pt model
|
48 |
+
yolo detect train data=coco8.yaml model=yolov8n.pt epochs=100 imgsz=640
|
49 |
+
```
|
50 |
+
|
51 |
+
## Sample Images and Annotations
|
52 |
+
|
53 |
+
Here are some examples of images from the COCO8 dataset, along with their corresponding annotations:
|
54 |
+
|
55 |
+
<img src="https://user-images.githubusercontent.com/26833433/236818348-e6260a3d-0454-436b-83a9-de366ba07235.jpg" alt="Dataset sample image" width="800">
|
56 |
+
|
57 |
+
- **Mosaiced Image**: This image demonstrates a training batch composed of mosaiced dataset images. Mosaicing is a technique used during training that combines multiple images into a single image to increase the variety of objects and scenes within each training batch. This helps improve the model's ability to generalize to different object sizes, aspect ratios, and contexts.
|
58 |
+
|
59 |
+
The example showcases the variety and complexity of the images in the COCO8 dataset and the benefits of using mosaicing during the training process.
|
60 |
+
|
61 |
+
## Citations and Acknowledgments
|
62 |
+
|
63 |
+
If you use the COCO dataset in your research or development work, please cite the following paper:
|
64 |
+
|
65 |
+
!!! note ""
|
66 |
+
|
67 |
+
=== "BibTeX"
|
68 |
+
|
69 |
+
```bibtex
|
70 |
+
@misc{lin2015microsoft,
|
71 |
+
title={Microsoft COCO: Common Objects in Context},
|
72 |
+
author={Tsung-Yi Lin and Michael Maire and Serge Belongie and Lubomir Bourdev and Ross Girshick and James Hays and Pietro Perona and Deva Ramanan and C. Lawrence Zitnick and Piotr Dollár},
|
73 |
+
year={2015},
|
74 |
+
eprint={1405.0312},
|
75 |
+
archivePrefix={arXiv},
|
76 |
+
primaryClass={cs.CV}
|
77 |
+
}
|
78 |
+
```
|
79 |
+
|
80 |
+
We would like to acknowledge the COCO Consortium for creating and maintaining this valuable resource for the computer vision community. For more information about the COCO dataset and its creators, visit the [COCO dataset website](https://cocodataset.org/#home).
|
ultralytics/docs/datasets/detect/globalwheat2020.md
ADDED
@@ -0,0 +1,91 @@
|
|
|
|
|
|
|
|
|
|
|
|
|
|
|
|
|
|
|
|
|
|
|
|
|
|
|
|
|
|
|
|
|
|
|
|
|
|
|
|
|
|
|
|
|
|
|
|
|
|
|
|
|
|
|
|
|
|
|
|
|
|
|
|
|
|
|
|
|
|
|
|
|
|
|
|
|
|
|
|
|
|
|
|
|
|
|
|
|
|
|
|
|
|
|
|
|
|
|
|
|
|
|
|
|
|
|
|
|
|
|
|
|
|
|
|
|
|
|
|
|
|
|
|
|
|
|
|
|
|
|
|
|
|
|
|
|
|
|
|
|
|
|
|
|
|
|
|
|
|
|
|
|
|
|
|
|
|
|
|
|
|
|
|
|
|
|
|
|
|
|
|
|
|
|
|
|
|
|
|
|
|
|
|
|
1 |
+
---
|
2 |
+
comments: true
|
3 |
+
description: Understand how to utilize the vast Global Wheat Head Dataset for building wheat head detection models. Features, structure, applications, usage, sample data, and citation.
|
4 |
+
keywords: Ultralytics, YOLO, Global Wheat Head Dataset, wheat head detection, plant phenotyping, crop management, deep learning, outdoor images, annotations, YAML configuration
|
5 |
+
---
|
6 |
+
|
7 |
+
# Global Wheat Head Dataset
|
8 |
+
|
9 |
+
The [Global Wheat Head Dataset](http://www.global-wheat.com/) is a collection of images designed to support the development of accurate wheat head detection models for applications in wheat phenotyping and crop management. Wheat heads, also known as spikes, are the grain-bearing parts of the wheat plant. Accurate estimation of wheat head density and size is essential for assessing crop health, maturity, and yield potential. The dataset, created by a collaboration of nine research institutes from seven countries, covers multiple growing regions to ensure models generalize well across different environments.
|
10 |
+
|
11 |
+
## Key Features
|
12 |
+
|
13 |
+
- The dataset contains over 3,000 training images from Europe (France, UK, Switzerland) and North America (Canada).
|
14 |
+
- It includes approximately 1,000 test images from Australia, Japan, and China.
|
15 |
+
- Images are outdoor field images, capturing the natural variability in wheat head appearances.
|
16 |
+
- Annotations include wheat head bounding boxes to support object detection tasks.
|
17 |
+
|
18 |
+
## Dataset Structure
|
19 |
+
|
20 |
+
The Global Wheat Head Dataset is organized into two main subsets:
|
21 |
+
|
22 |
+
1. **Training Set**: This subset contains over 3,000 images from Europe and North America. The images are labeled with wheat head bounding boxes, providing ground truth for training object detection models.
|
23 |
+
2. **Test Set**: This subset consists of approximately 1,000 images from Australia, Japan, and China. These images are used for evaluating the performance of trained models on unseen genotypes, environments, and observational conditions.
|
24 |
+
|
25 |
+
## Applications
|
26 |
+
|
27 |
+
The Global Wheat Head Dataset is widely used for training and evaluating deep learning models in wheat head detection tasks. The dataset's diverse set of images, capturing a wide range of appearances, environments, and conditions, make it a valuable resource for researchers and practitioners in the field of plant phenotyping and crop management.
|
28 |
+
|
29 |
+
## Dataset YAML
|
30 |
+
|
31 |
+
A YAML (Yet Another Markup Language) file is used to define the dataset configuration. It contains information about the dataset's paths, classes, and other relevant information. For the case of the Global Wheat Head Dataset, the `GlobalWheat2020.yaml` file is maintained at [https://github.com/ultralytics/ultralytics/blob/main/ultralytics/cfg/datasets/GlobalWheat2020.yaml](https://github.com/ultralytics/ultralytics/blob/main/ultralytics/cfg/datasets/GlobalWheat2020.yaml).
|
32 |
+
|
33 |
+
!!! example "ultralytics/cfg/datasets/GlobalWheat2020.yaml"
|
34 |
+
|
35 |
+
```yaml
|
36 |
+
--8<-- "ultralytics/cfg/datasets/GlobalWheat2020.yaml"
|
37 |
+
```
|
38 |
+
|
39 |
+
## Usage
|
40 |
+
|
41 |
+
To train a YOLOv8n model on the Global Wheat Head Dataset for 100 epochs with an image size of 640, you can use the following code snippets. For a comprehensive list of available arguments, refer to the model [Training](../../modes/train.md) page.
|
42 |
+
|
43 |
+
!!! example "Train Example"
|
44 |
+
|
45 |
+
=== "Python"
|
46 |
+
|
47 |
+
```python
|
48 |
+
from ultralytics import YOLO
|
49 |
+
|
50 |
+
# Load a model
|
51 |
+
model = YOLO('yolov8n.pt') # load a pretrained model (recommended for training)
|
52 |
+
|
53 |
+
# Train the model
|
54 |
+
results = model.train(data='GlobalWheat2020.yaml', epochs=100, imgsz=640)
|
55 |
+
```
|
56 |
+
|
57 |
+
=== "CLI"
|
58 |
+
|
59 |
+
```bash
|
60 |
+
# Start training from a pretrained *.pt model
|
61 |
+
yolo detect train data=GlobalWheat2020.yaml model=yolov8n.pt epochs=100 imgsz=640
|
62 |
+
```
|
63 |
+
|
64 |
+
## Sample Data and Annotations
|
65 |
+
|
66 |
+
The Global Wheat Head Dataset contains a diverse set of outdoor field images, capturing the natural variability in wheat head appearances, environments, and conditions. Here are some examples of data from the dataset, along with their corresponding annotations:
|
67 |
+
|
68 |
+
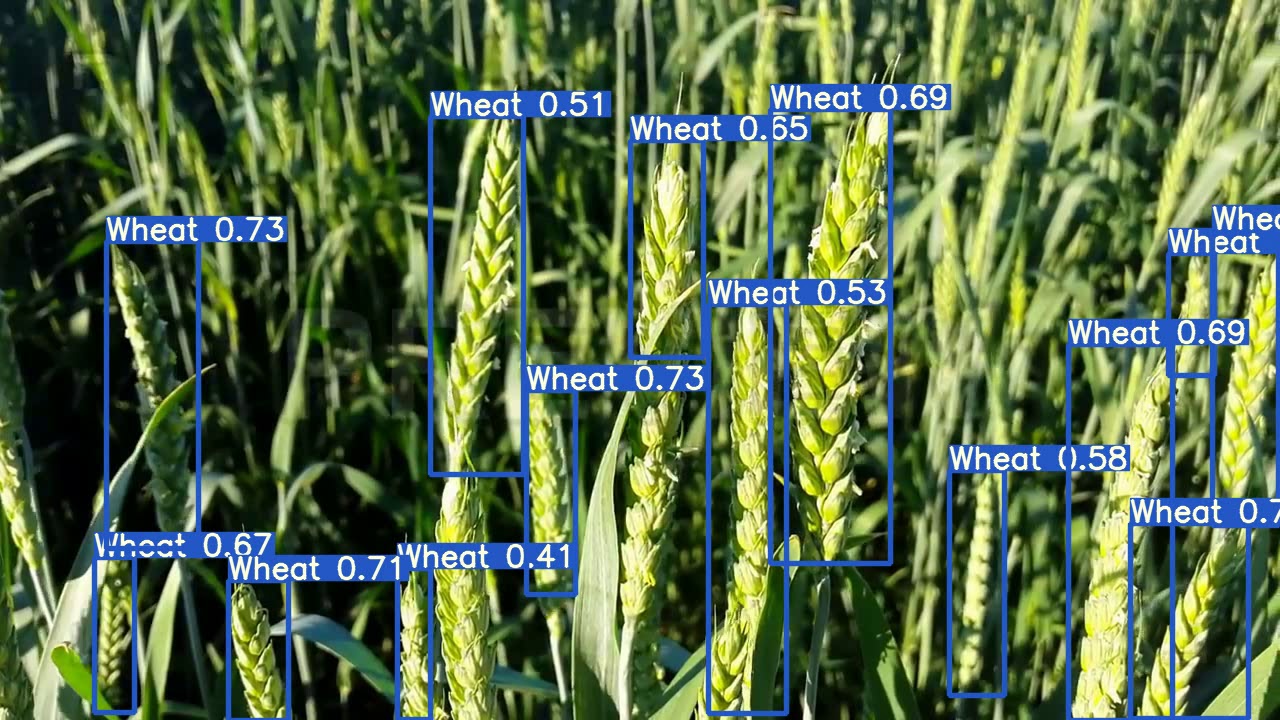
|
69 |
+
|
70 |
+
- **Wheat Head Detection**: This image demonstrates an example of wheat head detection, where wheat heads are annotated with bounding boxes. The dataset provides a variety of images to facilitate the development of models for this task.
|
71 |
+
|
72 |
+
The example showcases the variety and complexity of the data in the Global Wheat Head Dataset and highlights the importance of accurate wheat head detection for applications in wheat phenotyping and crop management.
|
73 |
+
|
74 |
+
## Citations and Acknowledgments
|
75 |
+
|
76 |
+
If you use the Global Wheat Head Dataset in your research or development work, please cite the following paper:
|
77 |
+
|
78 |
+
!!! note ""
|
79 |
+
|
80 |
+
=== "BibTeX"
|
81 |
+
|
82 |
+
```bibtex
|
83 |
+
@article{david2020global,
|
84 |
+
title={Global Wheat Head Detection (GWHD) Dataset: A Large and Diverse Dataset of High-Resolution RGB-Labelled Images to Develop and Benchmark Wheat Head Detection Methods},
|
85 |
+
author={David, Etienne and Madec, Simon and Sadeghi-Tehran, Pouria and Aasen, Helge and Zheng, Bangyou and Liu, Shouyang and Kirchgessner, Norbert and Ishikawa, Goro and Nagasawa, Koichi and Badhon, Minhajul and others},
|
86 |
+
journal={arXiv preprint arXiv:2005.02162},
|
87 |
+
year={2020}
|
88 |
+
}
|
89 |
+
```
|
90 |
+
|
91 |
+
We would like to acknowledge the researchers and institutions that contributed to the creation and maintenance of the Global Wheat Head Dataset as a valuable resource for the plant phenotyping and crop management research community. For more information about the dataset and its creators, visit the [Global Wheat Head Dataset website](http://www.global-wheat.com/).
|
ultralytics/docs/datasets/detect/index.md
ADDED
@@ -0,0 +1,108 @@
|
|
|
|
|
|
|
|
|
|
|
|
|
|
|
|
|
|
|
|
|
|
|
|
|
|
|
|
|
|
|
|
|
|
|
|
|
|
|
|
|
|
|
|
|
|
|
|
|
|
|
|
|
|
|
|
|
|
|
|
|
|
|
|
|
|
|
|
|
|
|
|
|
|
|
|
|
|
|
|
|
|
|
|
|
|
|
|
|
|
|
|
|
|
|
|
|
|
|
|
|
|
|
|
|
|
|
|
|
|
|
|
|
|
|
|
|
|
|
|
|
|
|
|
|
|
|
|
|
|
|
|
|
|
|
|
|
|
|
|
|
|
|
|
|
|
|
|
|
|
|
|
|
|
|
|
|
|
|
|
|
|
|
|
|
|
|
|
|
|
|
|
|
|
|
|
|
|
|
|
|
|
|
|
|
|
|
|
|
|
|
|
|
|
|
|
|
|
|
|
|
|
|
|
|
|
|
|
|
|
|
|
|
|
|
|
|
|
|
1 |
+
---
|
2 |
+
comments: true
|
3 |
+
description: Navigate through supported dataset formats, methods to utilize them and how to add your own datasets. Get insights on porting or converting label formats.
|
4 |
+
keywords: Ultralytics, YOLO, datasets, object detection, dataset formats, label formats, data conversion
|
5 |
+
---
|
6 |
+
|
7 |
+
# Object Detection Datasets Overview
|
8 |
+
|
9 |
+
Training a robust and accurate object detection model requires a comprehensive dataset. This guide introduces various formats of datasets that are compatible with the Ultralytics YOLO model and provides insights into their structure, usage, and how to convert between different formats.
|
10 |
+
|
11 |
+
## Supported Dataset Formats
|
12 |
+
|
13 |
+
### Ultralytics YOLO format
|
14 |
+
|
15 |
+
The Ultralytics YOLO format is a dataset configuration format that allows you to define the dataset root directory, the relative paths to training/validation/testing image directories or *.txt files containing image paths, and a dictionary of class names. Here is an example:
|
16 |
+
|
17 |
+
```yaml
|
18 |
+
# Train/val/test sets as 1) dir: path/to/imgs, 2) file: path/to/imgs.txt, or 3) list: [path/to/imgs1, path/to/imgs2, ..]
|
19 |
+
path: ../datasets/coco8 # dataset root dir
|
20 |
+
train: images/train # train images (relative to 'path') 4 images
|
21 |
+
val: images/val # val images (relative to 'path') 4 images
|
22 |
+
test: # test images (optional)
|
23 |
+
|
24 |
+
# Classes (80 COCO classes)
|
25 |
+
names:
|
26 |
+
0: person
|
27 |
+
1: bicycle
|
28 |
+
2: car
|
29 |
+
...
|
30 |
+
77: teddy bear
|
31 |
+
78: hair drier
|
32 |
+
79: toothbrush
|
33 |
+
```
|
34 |
+
|
35 |
+
Labels for this format should be exported to YOLO format with one `*.txt` file per image. If there are no objects in an image, no `*.txt` file is required. The `*.txt` file should be formatted with one row per object in `class x_center y_center width height` format. Box coordinates must be in **normalized xywh** format (from 0 to 1). If your boxes are in pixels, you should divide `x_center` and `width` by image width, and `y_center` and `height` by image height. Class numbers should be zero-indexed (start with 0).
|
36 |
+
|
37 |
+
<p align="center"><img width="750" src="https://user-images.githubusercontent.com/26833433/91506361-c7965000-e886-11ea-8291-c72b98c25eec.jpg" alt="Example labelled image"></p>
|
38 |
+
|
39 |
+
The label file corresponding to the above image contains 2 persons (class `0`) and a tie (class `27`):
|
40 |
+
|
41 |
+
<p align="center"><img width="428" src="https://user-images.githubusercontent.com/26833433/112467037-d2568c00-8d66-11eb-8796-55402ac0d62f.png" alt="Example label file"></p>
|
42 |
+
|
43 |
+
When using the Ultralytics YOLO format, organize your training and validation images and labels as shown in the example below.
|
44 |
+
|
45 |
+
<p align="center"><img width="700" src="https://user-images.githubusercontent.com/26833433/134436012-65111ad1-9541-4853-81a6-f19a3468b75f.png" alt="Example dataset directory structure"></p>
|
46 |
+
|
47 |
+
## Usage
|
48 |
+
|
49 |
+
Here's how you can use these formats to train your model:
|
50 |
+
|
51 |
+
!!! example ""
|
52 |
+
|
53 |
+
=== "Python"
|
54 |
+
|
55 |
+
```python
|
56 |
+
from ultralytics import YOLO
|
57 |
+
|
58 |
+
# Load a model
|
59 |
+
model = YOLO('yolov8n.pt') # load a pretrained model (recommended for training)
|
60 |
+
|
61 |
+
# Train the model
|
62 |
+
results = model.train(data='coco8.yaml', epochs=100, imgsz=640)
|
63 |
+
```
|
64 |
+
=== "CLI"
|
65 |
+
|
66 |
+
```bash
|
67 |
+
# Start training from a pretrained *.pt model
|
68 |
+
yolo detect train data=coco8.yaml model=yolov8n.pt epochs=100 imgsz=640
|
69 |
+
```
|
70 |
+
|
71 |
+
## Supported Datasets
|
72 |
+
|
73 |
+
Here is a list of the supported datasets and a brief description for each:
|
74 |
+
|
75 |
+
- [**Argoverse**](./argoverse.md): A collection of sensor data collected from autonomous vehicles. It contains 3D tracking annotations for car objects.
|
76 |
+
- [**COCO**](./coco.md): Common Objects in Context (COCO) is a large-scale object detection, segmentation, and captioning dataset with 80 object categories.
|
77 |
+
- [**COCO8**](./coco8.md): A smaller subset of the COCO dataset, COCO8 is more lightweight and faster to train.
|
78 |
+
- [**GlobalWheat2020**](./globalwheat2020.md): A dataset containing images of wheat heads for the Global Wheat Challenge 2020.
|
79 |
+
- [**Objects365**](./objects365.md): A large-scale object detection dataset with 365 object categories and 600k images, aimed at advancing object detection research.
|
80 |
+
- [**OpenImagesV7**](./open-images-v7.md): A comprehensive dataset by Google with 1.7M train images and 42k validation images.
|
81 |
+
- [**SKU-110K**](./sku-110k.md): A dataset containing images of densely packed retail products, intended for retail environment object detection.
|
82 |
+
- [**VisDrone**](./visdrone.md): A dataset focusing on drone-based images, containing various object categories like cars, pedestrians, and cyclists.
|
83 |
+
- [**VOC**](./voc.md): PASCAL VOC is a popular object detection dataset with 20 object categories including vehicles, animals, and furniture.
|
84 |
+
- [**xView**](./xview.md): A dataset containing high-resolution satellite imagery, designed for the detection of various object classes in overhead views.
|
85 |
+
|
86 |
+
### Adding your own dataset
|
87 |
+
|
88 |
+
If you have your own dataset and would like to use it for training detection models with Ultralytics YOLO format, ensure that it follows the format specified above under "Ultralytics YOLO format". Convert your annotations to the required format and specify the paths, number of classes, and class names in the YAML configuration file.
|
89 |
+
|
90 |
+
## Port or Convert Label Formats
|
91 |
+
|
92 |
+
### COCO Dataset Format to YOLO Format
|
93 |
+
|
94 |
+
You can easily convert labels from the popular COCO dataset format to the YOLO format using the following code snippet:
|
95 |
+
|
96 |
+
!!! example ""
|
97 |
+
|
98 |
+
=== "Python"
|
99 |
+
|
100 |
+
```python
|
101 |
+
from ultralytics.data.converter import convert_coco
|
102 |
+
|
103 |
+
convert_coco(labels_dir='path/to/coco/annotations/')
|
104 |
+
```
|
105 |
+
|
106 |
+
This conversion tool can be used to convert the COCO dataset or any dataset in the COCO format to the Ultralytics YOLO format.
|
107 |
+
|
108 |
+
Remember to double-check if the dataset you want to use is compatible with your model and follows the necessary format conventions. Properly formatted datasets are crucial for training successful object detection models.
|