license: apache-2.0
datasets:
- AIDC-AI/Ovis-dataset
library_name: transformers
tags:
- MLLM
pipeline_tag: image-text-to-text
language:
- en
Ovis1.6-Gemma2-27B
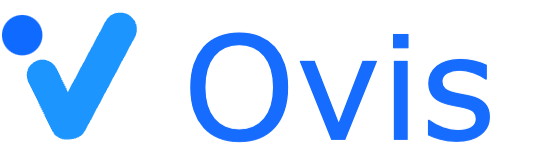
Introduction
We are pleased to announce the release of Ovis1.6-Gemma2-27B, our latest advancement in multi-modal large language models (MLLMs). Building upon the foundation of Ovis1.6, this model marks a substantial progression in multimodal AI capabilities, considerably expanding both capacity and performance while preserving the core architectural innovation of structurally aligning visual and textual embeddings.
Key Features:
Enhanced Model Performance: Ovis1.6-Gemma2-27B excels in handling complex image-text instruction tasks, demonstrating enhanced understanding and reasoning across diverse modalities.
Advanced Image Processing: Demonstrates exceptional proficiency in analyzing complex visual inputs with high accuracy and granularity.
Refined Chain-of-Thought Reasoning: Exhibits markedly improved CoT capabilities, enabling sophisticated problem-solving across visual and textual domains.
Enhanced Document Understanding: Enhances comprehension of various document types (documents, charts, tables) and improves image recognition for Chinese and English text.

Model Zoo
Succeeding Ovis1.5, Ovis1.6 further enhances high-resolution image processing, is trained on a larger, more diverse, and higher-quality dataset, and refines the training process with DPO training following instruction-tuning.
Ovis MLLMs | ViT | LLM | Model Weights | Demo |
---|---|---|---|---|
Ovis1.6-Gemma2-27B | Siglip-400M | Gemma2-27B-It | Huggingface | - |
Ovis1.6-Gemma2-9B | Siglip-400M | Gemma2-9B-It | Huggingface | Space |
Ovis1.6-Llama3.2-3B | Siglip-400M | Llama-3.2-3B-Instruct | Huggingface | Space |
Performance
With 29B parameters, Ovis1.6-Gemma2-27B achieves exceptional performance in the OpenCompass benchmark, ranking among the top-tier open-source MLLMs.

Usage
Below is a code snippet to run Ovis with multimodal inputs. For additional usage instructions, including inference wrapper and Gradio UI, please refer to Ovis GitHub.
pip install torch==2.4.0 transformers==4.46.2 numpy==1.25.0 pillow==10.3.0
import torch
from PIL import Image
from transformers import AutoModelForCausalLM
# load model
model = AutoModelForCausalLM.from_pretrained("AIDC-AI/Ovis1.6-Gemma2-27B",
torch_dtype=torch.bfloat16,
multimodal_max_length=8192,
trust_remote_code=True).cuda()
text_tokenizer = model.get_text_tokenizer()
visual_tokenizer = model.get_visual_tokenizer()
# enter image path and prompt
image_path = input("Enter image path: ")
image = Image.open(image_path)
text = input("Enter prompt: ")
query = f'<image>\n{text}'
# format conversation
prompt, input_ids, pixel_values = model.preprocess_inputs(query, [image])
attention_mask = torch.ne(input_ids, text_tokenizer.pad_token_id)
input_ids = input_ids.unsqueeze(0).to(device=model.device)
attention_mask = attention_mask.unsqueeze(0).to(device=model.device)
pixel_values = [pixel_values.to(dtype=visual_tokenizer.dtype, device=visual_tokenizer.device)]
# generate output
with torch.inference_mode():
gen_kwargs = dict(
max_new_tokens=1024,
do_sample=False,
top_p=None,
top_k=None,
temperature=None,
repetition_penalty=None,
eos_token_id=model.generation_config.eos_token_id,
pad_token_id=text_tokenizer.pad_token_id,
use_cache=True
)
output_ids = model.generate(input_ids, pixel_values=pixel_values, attention_mask=attention_mask, **gen_kwargs)[0]
output = text_tokenizer.decode(output_ids, skip_special_tokens=True)
print(f'Output:\n{output}')
FlashAttention Support
To use FlashAttention, first install flash-attn>=2.6.3
:
pip install flash-attn --no-build-isolation
Then, load model with llm_attn_implementation='flash_attention_2'
:
model = AutoModelForCausalLM.from_pretrained("AIDC-AI/Ovis1.6-Gemma2-27B",
torch_dtype=torch.bfloat16,
multimodal_max_length=8192,
llm_attn_implementation='flash_attention_2',
trust_remote_code=True).cuda()
Batch Inference
batch_inputs = [
('example_image1.jpeg', 'Describe the content of this image.'),
('example_image2.jpeg', 'What is the equation in the image?')
]
batch_input_ids = []
batch_attention_mask = []
batch_pixel_values = []
for image_path, text in batch_inputs:
image = Image.open(image_path)
query = f'<image>\n{text}'
prompt, input_ids, pixel_values = model.preprocess_inputs(query, [image])
attention_mask = torch.ne(input_ids, text_tokenizer.pad_token_id)
input_ids = input_ids.unsqueeze(0).to(device=model.device)
attention_mask = attention_mask.unsqueeze(0).to(device=model.device)
pixel_values = [pixel_values.to(dtype=visual_tokenizer.dtype, device=visual_tokenizer.device)]
batch_input_ids.append(input_ids.squeeze())
batch_attention_mask.append(attention_mask.squeeze())
batch_pixel_values.append(pixel_values)
pad_batch_input_ids = torch.nn.utils.rnn.pad_sequence([i.flip(dims=[0]) for i in batch_input_ids],batch_first=True, padding_value=0.0).flip(dims=[1])
pad_batch_input_ids = pad_batch_input_ids[:,-model.config.multimodal_max_length:]
pad_batch_attention_mask = torch.nn.utils.rnn.pad_sequence([i.flip(dims=[0]) for i in batch_attention_mask],batch_first=True, padding_value=False).flip(dims=[1])
pad_batch_attention_mask = pad_batch_attention_mask[:,-model.config.multimodal_max_length:]
pad_batch_pixel_values = [item for sublist in batch_pixel_values for item in sublist]
# generate output
with torch.inference_mode():
gen_kwargs = dict(
max_new_tokens=1024,
do_sample=False,
top_p=None,
top_k=None,
temperature=None,
repetition_penalty=None,
eos_token_id=model.generation_config.eos_token_id,
pad_token_id=text_tokenizer.pad_token_id,
use_cache=True
)
output_ids = model.generate(pad_batch_input_ids, pixel_values=pad_batch_pixel_values, attention_mask=pad_batch_attention_mask, **gen_kwargs)
for i in range(len(batch_input_ids)):
output = text_tokenizer.decode(output_ids[i], skip_special_tokens=True)
print(f'Output_{i}:\n{output}')
Citation
If you find Ovis useful, please cite the paper
@article{lu2024ovis,
title={Ovis: Structural Embedding Alignment for Multimodal Large Language Model},
author={Shiyin Lu and Yang Li and Qing-Guo Chen and Zhao Xu and Weihua Luo and Kaifu Zhang and Han-Jia Ye},
year={2024},
journal={arXiv:2405.20797}
}
License
This project is licensed under the Apache License, Version 2.0 (SPDX-License-Identifier: Apache-2.0). Any use of the model shall be subject to Gemma's use restrictions. You must not use the model for the restricted uses set forth in the Gemma Prohibited Use Policy, which is incorporated by reference into Gemma Terms of Use, or in violation of applicable laws and regulations. To the maximum extent permitted by law, Google reserves the right to restrict (remotely or otherwise) usage of any of the Gemma Services that Google reasonably believes are in violation of Gemma Terms of Use.
Disclaimer
We used compliance-checking algorithms during the training process, to ensure the compliance of the trained model to the best of our ability. Due to the complexity of the data and the diversity of language model usage scenarios, we cannot guarantee that the model is completely free of copyright issues or improper content. If you believe anything infringes on your rights or generates improper content, please contact us, and we will promptly address the matter.