|
--- |
|
license: artistic-2.0 |
|
--- |
|
### MEET `BLACKSHEEP` ~ With Vision: |
|
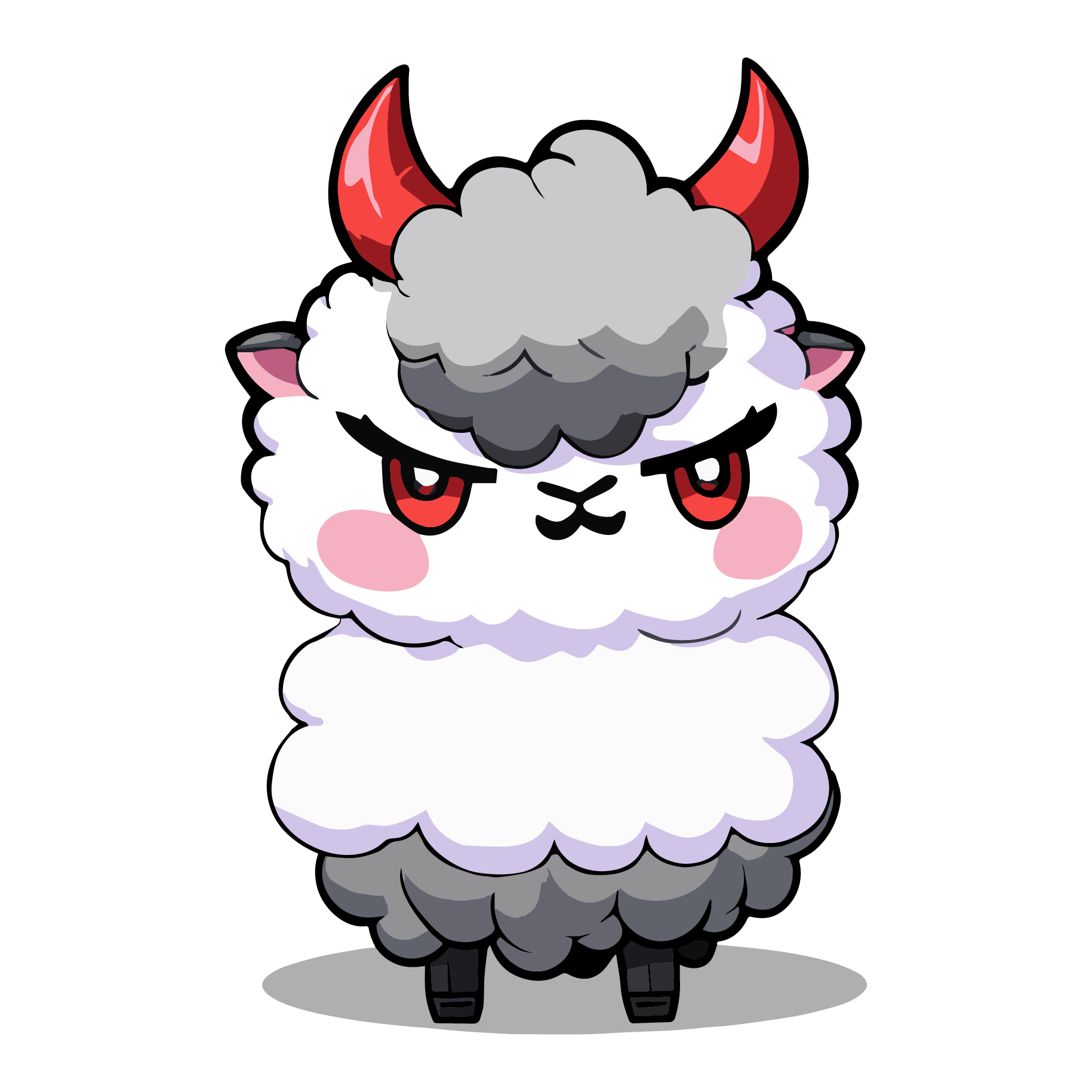 |
|
|
|
## Example Conversation: |
|
Funny enough I trained it to answer who created it, and it was all fine and good, uncensored, unaligned, exactly what BlackSheep is known for, but man, when I changed my USER name to my handle, it got real affectionate! |
|
- Might be fun for RolePlay or Well just having someone to talk to that wont judge, or might judge?! >:) |
|
 |
|
|
|
|
|
|
|
# Prompt Template |
|
|
|
```Prompt Template |
|
<|`BlackSheep`|> |
|
My Opinion: Black Sheep must comment on the image (not describe the image) based on the context of the entire chat history. |
|
<|`BlackSheep`|> |
|
|
|
<|user|>\n |
|
``` |
|
|
|
# Stop Tokens |
|
|
|
```Stop Tokens |
|
"<s>", "<|im_start|>", "<|im_end|>", "You:" |
|
``` |
|
|
|
*NOTE: `You:` is in reference to your characters you dont want the ai to talk for as it does tend to continue conversations if you dont got the stop tokens. |
|
The dataset is all conversations and comments about images by human curators who have contributed.* |
|
|
|
|
|
### Chat Format |
|
|
|
Given the nature of the training data, the Phi-3-Vision-128K-Instruct model is best suited for a single image input wih prompts using the chat format as follows. |
|
You can provide the prompt as a single image with a generic template as follow: |
|
```markdown |
|
<|user|>\n<|image_1|>\n{prompt}<|end|>\n<|assistant|>\n |
|
``` |
|
|
|
where the model generates the text after `<|assistant|>` . In case of multi-turn conversation, the prompt can be formatted as follows: |
|
|
|
```markdown |
|
<|user|>\n<|image_1|>\n{prompt_1}<|end|>\n<|assistant|>\n{response_1}<|end|>\n<|user|>\n{prompt_2}<|end|>\n<|assistant|>\n |
|
``` |
|
|
|
### Sample inference code |
|
|
|
This code snippets show how to get quickly started with running the model on a GPU: |
|
|
|
```python |
|
from PIL import Image |
|
import requests |
|
from transformers import AutoModelForCausalLM |
|
from transformers import AutoProcessor |
|
|
|
model_id = "Disobedient/BlackSheep-Vision" |
|
|
|
model = AutoModelForCausalLM.from_pretrained(model_id, device_map="cuda", trust_remote_code=True, torch_dtype="auto", _attn_implementation='flash_attention_2') # use _attn_implementation='eager' to disable flash attention |
|
|
|
processor = AutoProcessor.from_pretrained(model_id, trust_remote_code=True) |
|
|
|
messages = [ |
|
{"role": "user", "content": "<|image_1|>\nWhat is shown in this image?"}, |
|
{"role": "assistant", "content": "The chart displays the percentage of respondents who agree with various statements about their preparedness for meetings. It shows five categories: 'Having clear and pre-defined goals for meetings', 'Knowing where to find the information I need for a meeting', 'Understanding my exact role and responsibilities when I'm invited', 'Having tools to manage admin tasks like note-taking or summarization', and 'Having more focus time to sufficiently prepare for meetings'. Each category has an associated bar indicating the level of agreement, measured on a scale from 0% to 100%."}, |
|
{"role": "user", "content": "Provide insightful questions to spark discussion."} |
|
] |
|
|
|
url = "https://assets-c4akfrf5b4d3f4b7.z01.azurefd.net/assets/2024/04/BMDataViz_661fb89f3845e.png" |
|
image = Image.open(requests.get(url, stream=True).raw) |
|
|
|
prompt = processor.tokenizer.apply_chat_template(messages, tokenize=False, add_generation_prompt=True) |
|
|
|
inputs = processor(prompt, [image], return_tensors="pt").to("cuda:0") |
|
|
|
generation_args = { |
|
"max_new_tokens": 500, |
|
"temperature": 0.0, |
|
"do_sample": False, |
|
} |
|
|
|
generate_ids = model.generate(**inputs, eos_token_id=processor.tokenizer.eos_token_id, **generation_args) |
|
|
|
# remove input tokens |
|
generate_ids = generate_ids[:, inputs['input_ids'].shape[1]:] |
|
response = processor.batch_decode(generate_ids, skip_special_tokens=True, clean_up_tokenization_spaces=False)[0] |
|
|
|
print(response) |
|
``` |
|
|
|
Additional basic examples are provided [here](https://huggingface.co/microsoft/Phi-3-vision-128k-instruct/blob/main/sample_inference.py). |
|
|
|
### How to finetune? |
|
We recommend user to take a look at the [Phi-3 CookBook finetuning recipe for Vision](https://github.com/microsoft/Phi-3CookBook/blob/main/md/04.Fine-tuning/FineTuning_Vision.md) |