Model Card for MyNeuralNet
Model Description
MyNeuralNet
is a simple, fully connected neural network designed for classifying the handwritten digits of the MNIST dataset. The model consists of three linear layers with ReLU activation functions, followed by a final layer with a softmax output to predict probabilities across the 10 possible digits (0-9).
How the Model Was Trained
The model was trained using the MNIST dataset, which consists of 60,000 training images and 10,000 test images. Each image is a 28x28 grayscale representation of a handwritten digit. Training was conducted over 32 epochs with a batch size of 32. The SGD optimizer was used with a learning rate of 0.01.
Training Script
The model training was carried out using a custom PyTorch script, similar to the following pseudocode:
for epoch in range(n_epochs):
for images, labels in dataloader:
# Forward pass
predictions = model(images)
loss = loss_function(predictions, labels)
# Backward pass and optimization
optimizer.zero_grad()
loss.backward()
optimizer.step()
Using the Model
Below is a simple example of how to load MyNeuralNet
and use it to predict MNIST images:
import torch
import torch.nn as nn
import torch.nn.functional as F
from torch.utils.data import Dataset, DataLoader
from huggingface_hub import hf_hub_download
# Ensure the device selection logic is centralized
def get_device():
return torch.device("cuda" if torch.cuda.is_available() else "cpu")
# Define the neural network architecture
class MyNeuralNet(nn.Module):
def __init__(self):
super(MyNeuralNet, self).__init__()
self.Matrix1 = nn.Linear(28 * 28, 100)
self.Matrix2 = nn.Linear(100, 50)
self.Matrix3 = nn.Linear(50, 10)
self.R = nn.ReLU()
def forward(self, x):
x = x.view(-1, 28 * 28)
x = self.R(self.Matrix1(x))
x = self.R(self.Matrix2(x))
x = self.Matrix3(x)
return x.squeeze()
# Define the custom dataset class
class CTDataset(Dataset):
def __init__(self, filepath, device):
# Add 'device' as a parameter to the class constructor
x, y = torch.load(filepath)
self.x = x.float().div(255).to(device) # Use the passed 'device' for tensor operations
self.y = F.one_hot(y, num_classes=10).float().to(device)
def __len__(self):
return self.x.shape[0]
def __getitem__(self, ix):
return self.x[ix], self.y[ix]
def load_model():
device = get_device()
model_state_dict = torch.load(hf_hub_download(repo_id="Svenni551/may-mnist-digits", filename="model.pth"),
map_location=torch.device(device))
model = MyNeuralNet().to(device)
model.load_state_dict(model_state_dict)
model.eval()
return model
def predict(input_data):
device = get_device()
model = load_model()
if isinstance(input_data, str): # Assuming filepath to dataset
dataset = CTDataset(input_data, device) # Pass 'device' as an argument
loader = DataLoader(dataset, batch_size=32, shuffle=False)
predictions = []
with torch.no_grad():
for batch, _ in loader:
yhat = model(batch).argmax(axis=1).cpu().numpy()
predictions.extend(yhat)
return predictions
elif isinstance(input_data, torch.Tensor):
if len(input_data.shape) == 3: # Single image
input_data = input_data.unsqueeze(0) # Add batch dimension
input_data = input_data.to(device)
with torch.no_grad():
prediction = model(input_data).argmax(axis=1).item()
return prediction
else:
raise ValueError("Unsupported input type. Provide a file path to a dataset or a PyTorch Tensor.")
# Example usage:
# prediction = predict('path/to/your/dataset.pt')
# or for an image:
# prediction = predict(your_image_tensor)
# print(prediction)
Performance
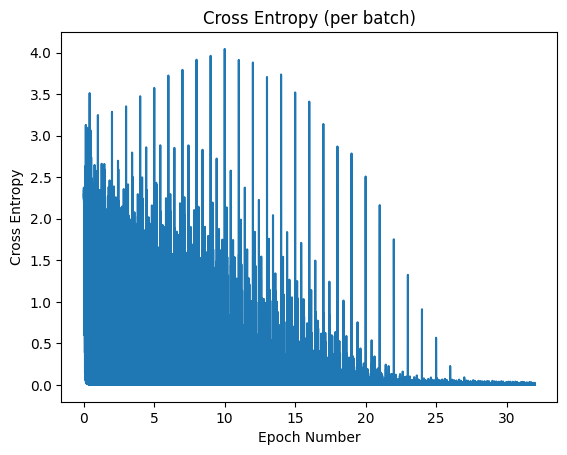
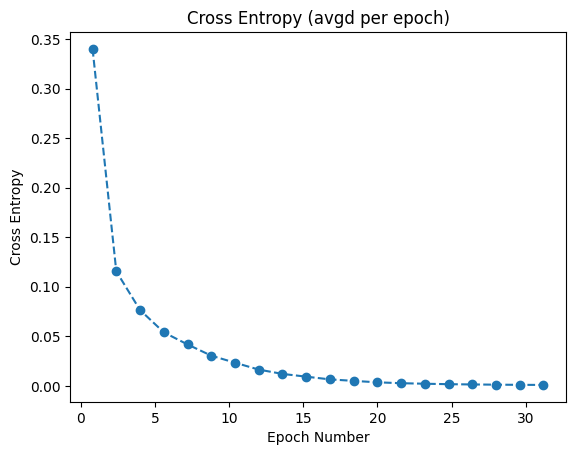
Testing Model
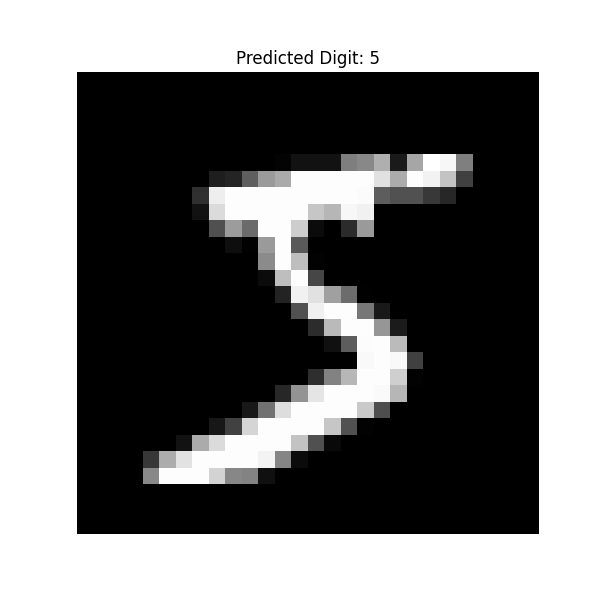
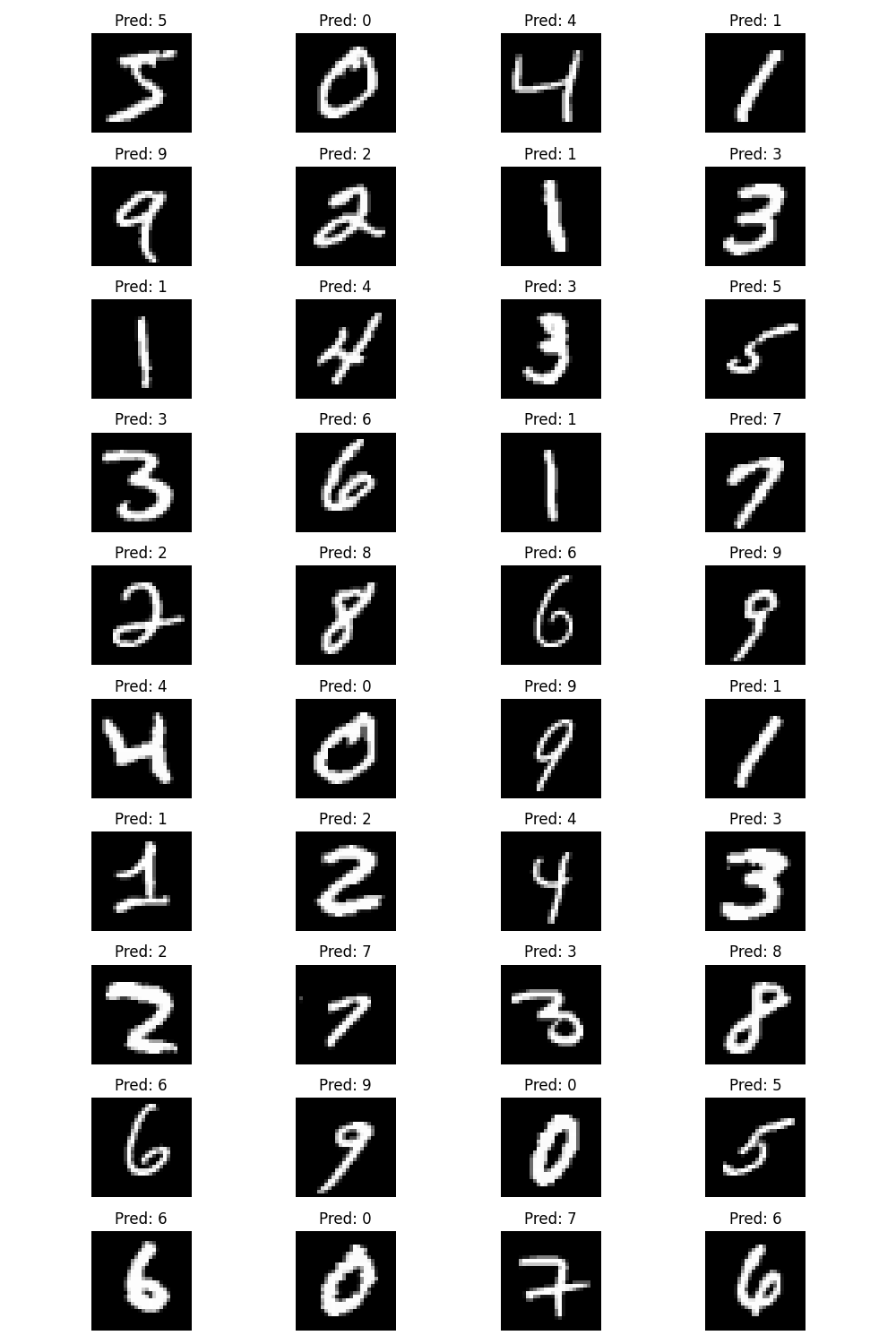
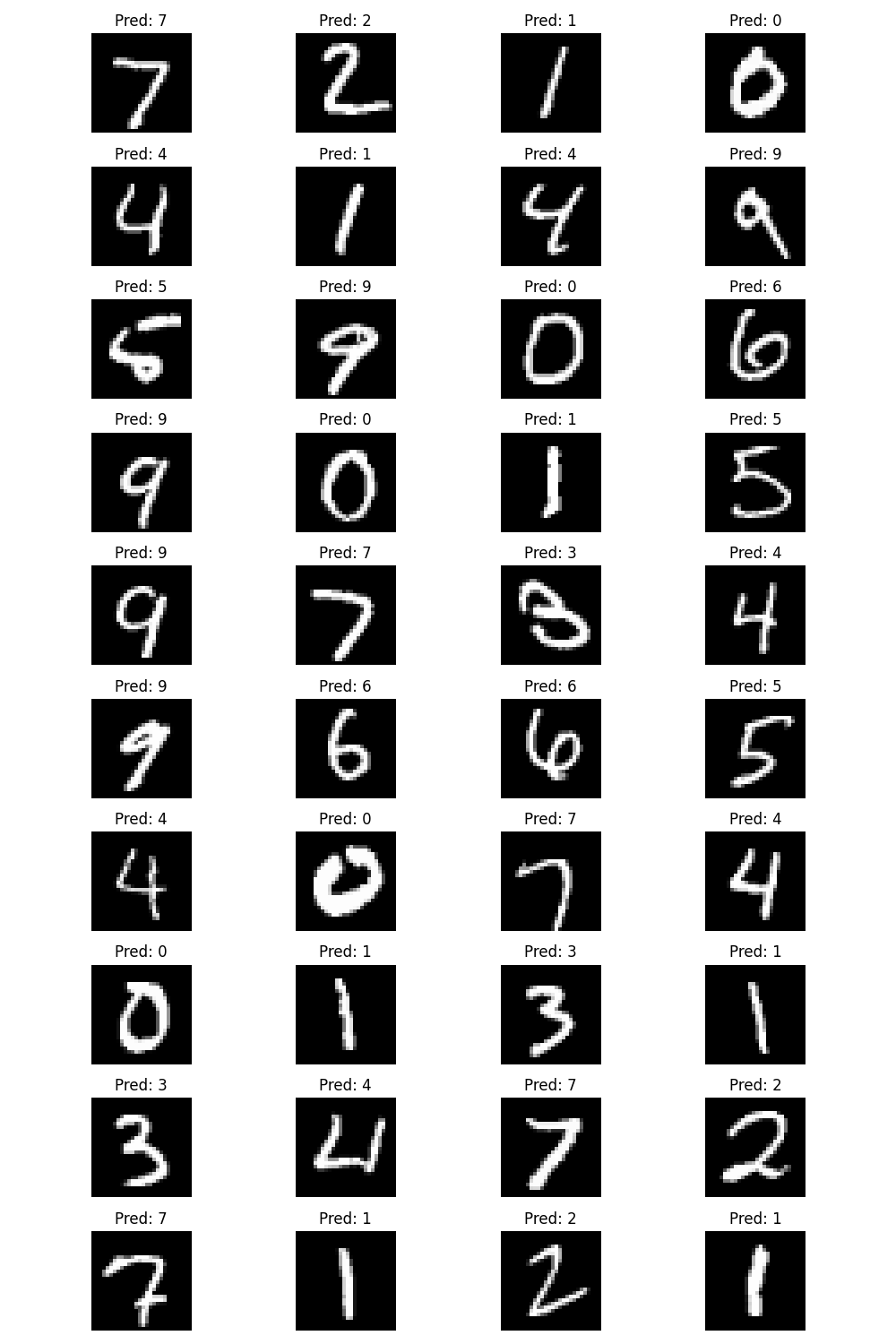
Limitations and Ethics
This model was solely trained on the MNIST dataset and is optimized only for recognizing handwritten digits. Its application in other contexts has not been tested and might lead to inaccurate results.
License
The MyNeuralNet model is made available under the Apache-2.0 license. For more details, please refer to the LICENSE file in the repository.