File size: 9,786 Bytes
07729be 6666d84 07729be 4935da6 07729be 55ed62d 4935da6 b5a268c 6b47008 4935da6 25b996a 4935da6 81bec39 4935da6 81bec39 4935da6 ed5e31e 4935da6 55ed62d 4935da6 55ed62d 4935da6 55ed62d 4935da6 |
1 2 3 4 5 6 7 8 9 10 11 12 13 14 15 16 17 18 19 20 21 22 23 24 25 26 27 28 29 30 31 32 33 34 35 36 37 38 39 40 41 42 43 44 45 46 47 48 49 50 51 52 53 54 55 56 57 58 59 60 61 62 63 64 65 66 67 68 69 70 71 72 73 74 75 76 77 78 79 80 81 82 83 84 85 86 87 88 89 90 91 92 93 94 95 96 97 98 99 100 101 102 103 104 105 106 107 108 109 110 111 112 113 114 115 116 117 118 119 120 121 122 123 124 125 126 127 128 129 130 131 132 133 134 135 136 137 138 139 140 141 142 143 144 145 146 147 148 149 150 151 152 153 154 155 156 157 158 159 160 161 162 163 164 165 166 167 168 169 170 171 172 173 174 175 176 177 178 179 180 181 182 183 184 185 186 187 188 189 190 191 192 193 194 195 196 197 198 199 200 201 202 203 204 205 206 207 208 209 210 211 212 213 214 215 216 217 218 219 220 221 222 223 224 225 |
---
base_model: HuggingFaceM4/idefics2-8b
datasets:
- TIGER-Lab/Mantis-Instruct
language:
- en
license: apache-2.0
tags:
- multimodal
- lmm
- vlm
- llava
- siglip
- llama3
- mantis
model-index:
- name: mantis-8b-idefics2_8192
results: []
---
# 🔥 Mantis (TMLR 2024)
[Paper](https://arxiv.org/abs/2405.01483) |
[Website](https://tiger-ai-lab.github.io/Mantis/) |
[Github](https://github.com/TIGER-AI-Lab/Mantis) |
[Models](https://huggingface.co/collections/TIGER-Lab/mantis-6619b0834594c878cdb1d6e4) |
[Demo](https://huggingface.co/spaces/TIGER-Lab/Mantis) |
[Wandb](https://api.wandb.ai/links/dongfu/lnkrl3af)
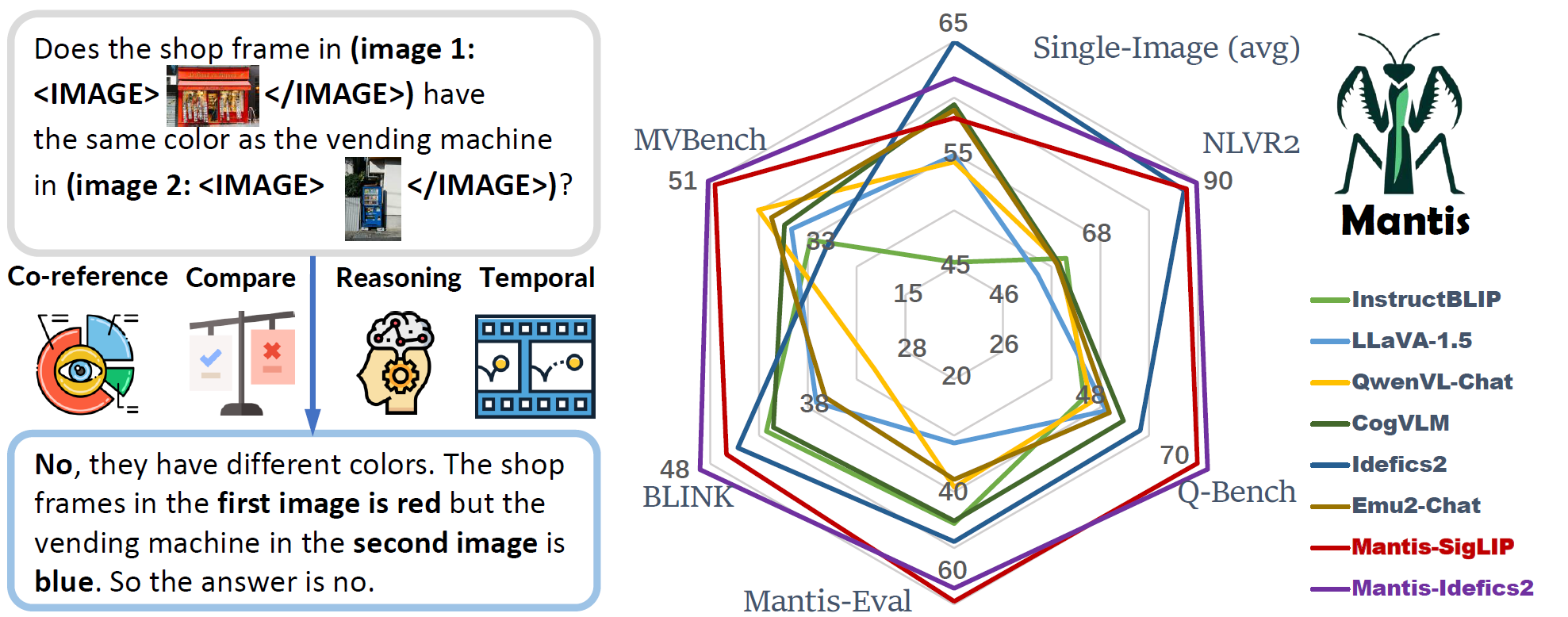
**Excited to announce Mantis-Idefics2, with enhanced ability in multi-image scenarios!**
It's fine-tuned on [Mantis-Instruct](https://huggingface.co/datasets/TIGER-Lab/Mantis-Instruct) from [Idefics2-8b](https://huggingface.co/HuggingFaceM4/idefics2-8b)
## Summary
- Mantis-Idefics2 is an LMM with **interleaved text and image as inputs**, trained on Mantis-Instruct under academic-level resources (i.e. 36 hours on 16xA100-40G).
- Mantis is trained to have multi-image skills including co-reference, reasoning, comparing, temporal understanding.
- Mantis reaches the state-of-the-art performance on five multi-image benchmarks (NLVR2, Q-Bench, BLINK, MVBench, Mantis-Eval), and also maintain a strong single-image performance on par with CogVLM and Emu2.
## Multi-Image Performance
| Models | Size | Format | NLVR2 | Q-Bench | Mantis-Eval | BLINK | MVBench | Avg |
|--------------------|:----:|:--------:|:-----:|:-------:|:-----------:|:-----:|:-------:|:----:|
| GPT-4V | - | sequence | 88.80 | 76.52 | 62.67 | 51.14 | 43.50 | 64.5 |
| Open Source Models | | | | | | | | |
| Random | - | - | 48.93 | 40.20 | 23.04 | 38.09 | 27.30 | 35.5 |
| Kosmos2 | 1.6B | merge | 49.00 | 35.10 | 30.41 | 37.50 | 21.62 | 34.7 |
| LLaVA-v1.5 | 7B | merge | 53.88 | 49.32 | 31.34 | 37.13 | 36.00 | 41.5 |
| LLava-V1.6 | 7B | merge | 58.88 | 54.80 | 45.62 | 39.55 | 40.90 | 48.0 |
| Qwen-VL-Chat | 7B | merge | 58.72 | 45.90 | 39.17 | 31.17 | 42.15 | 43.4 |
| Fuyu | 8B | merge | 51.10 | 49.15 | 27.19 | 36.59 | 30.20 | 38.8 |
| BLIP-2 | 13B | merge | 59.42 | 51.20 | 49.77 | 39.45 | 31.40 | 46.2 |
| InstructBLIP | 13B | merge | 60.26 | 44.30 | 45.62 | 42.24 | 32.50 | 45.0 |
| CogVLM | 17B | merge | 58.58 | 53.20 | 45.16 | 41.54 | 37.30 | 47.2 |
| OpenFlamingo | 9B | sequence | 36.41 | 19.60 | 12.44 | 39.18 | 7.90 | 23.1 |
| Otter-Image | 9B | sequence | 49.15 | 17.50 | 14.29 | 36.26 | 15.30 | 26.5 |
| Idefics1 | 9B | sequence | 54.63 | 30.60 | 28.11 | 24.69 | 26.42 | 32.9 |
| VideoLLaVA | 7B | sequence | 56.48 | 45.70 | 35.94 | 38.92 | 44.30 | 44.3 |
| Emu2-Chat | 37B | sequence | 58.16 | 50.05 | 37.79 | 36.20 | 39.72 | 44.4 |
| Vila | 8B | sequence | 76.45 | 45.70 | 51.15 | 39.30 | 49.40 | 52.4 |
| Idefics2 | 8B | sequence | 86.87 | 57.00 | 48.85 | 45.18 | 29.68 | 53.5 |
| Mantis-CLIP | 8B | sequence | 84.66 | 66.00 | 55.76 | 47.06 | 48.30 | 60.4 |
| Mantis-SIGLIP | 8B | sequence | 87.43 | 69.90 | **59.45** | 46.35 | 50.15 | 62.7 |
| Mantis-Flamingo | 9B | sequence | 52.96 | 46.80 | 32.72 | 38.00 | 40.83 | 42.3 |
| Mantis-Idefics2 | 8B | sequence | **89.71** | **75.20** | 57.14 | **49.05** | **51.38** | **64.5** |
| $\Delta$ over SOTA | - | - | +2.84 | +18.20 | +8.30 | +3.87 | +1.98 | +11.0 |
## Single-Image Performance
| Model | Size | TextVQA | VQA | MMB | MMMU | OKVQA | SQA | MathVista | Avg |
|-----------------|:----:|:-------:|:----:|:----:|:----:|:-----:|:----:|:---------:|:----:|
| OpenFlamingo | 9B | 46.3 | 58.0 | 32.4 | 28.7 | 51.4 | 45.7 | 18.6 | 40.2 |
| Idefics1 | 9B | 39.3 | 68.8 | 45.3 | 32.5 | 50.4 | 51.6 | 21.1 | 44.1 |
| InstructBLIP | 7B | 33.6 | 75.2 | 38.3 | 30.6 | 45.2 | 70.6 | 24.4 | 45.4 |
| Yi-VL | 6B | 44.8 | 72.5 | 68.4 | 39.1 | 51.3 | 71.7 | 29.7 | 53.9 |
| Qwen-VL-Chat | 7B | 63.8 | 78.2 | 61.8 | 35.9 | 56.6 | 68.2 | 15.5 | 54.3 |
| LLaVA-1.5 | 7B | 58.2 | 76.6 | 64.8 | 35.3 | 53.4 | 70.4 | 25.6 | 54.9 |
| Emu2-Chat | 37B | <u>66.6</u> | **84.9** | 63.6 | 36.3 | **64.8** | 65.3 | 30.7 | 58.9 |
| CogVLM | 17B | **70.4** | <u>82.3</u> | 65.8 | 32.1 | <u>64.8</u> | 65.6 | 35.0 | 59.4 |
| Idefics2 | 8B | 70.4 | 79.1 | <u>75.7</u> | **43.0** | 53.5 | **86.5** | **51.4** | **65.7** |
| Mantis-CLIP | 8B | 56.4 | 73.0 | 66.0 | 38.1 | 53.0 | 73.8 | 31.7 | 56.0 |
| Mantis-SigLIP | 8B | 59.2 | 74.9 | 68.7 | 40.1 | 55.4 | 74.9 | 34.4 | 58.2 |
| Mantis-Idefics2 | 8B | 63.5 | 77.6 | 75.7 | <u>41.1</u> | 52.6 | <u>81.3</u> | <u>40.4</u> | <u>61.7</u> |
## How to use
### Run example inference:
```python
import requests
import torch
from PIL import Image
from io import BytesIO
from transformers import AutoProcessor, AutoModelForVision2Seq
from transformers.image_utils import load_image
processor = AutoProcessor.from_pretrained("TIGER-Lab/Mantis-8B-Idefics2") # do_image_splitting is False by default
model = AutoModelForVision2Seq.from_pretrained(
"TIGER-Lab/Mantis-8B-Idefics2",
device_map="auto"
)
generation_kwargs = {
"max_new_tokens": 1024,
"num_beams": 1,
"do_sample": False
}
# Note that passing the image urls (instead of the actual pil images) to the processor is also possible
image1 = load_image("https://cdn.britannica.com/59/94459-050-DBA42467/Skyline-Chicago.jpg")
image2 = load_image("https://cdn.britannica.com/61/93061-050-99147DCE/Statue-of-Liberty-Island-New-York-Bay.jpg")
image3 = load_image("https://cdn.britannica.com/68/170868-050-8DDE8263/Golden-Gate-Bridge-San-Francisco.jpg")
images = [image1, image2, image3]
query1 = "What cities image 1, image 2, and image 3 belong to respectively? Answer me in order."
query2 = "Which one do you recommend for a visit? and why?"
query3 = "Which picture has most cars in it?"
### Chat
### Round 1
messages = [
{
"role": "user",
"content": [
{"type": "image"},
{"type": "image"},
{"type": "image"},
{"type": "text", "text": query1},
]
}
]
prompt = processor.apply_chat_template(messages, add_generation_prompt=True)
inputs = processor(text=prompt, images=images, return_tensors="pt")
inputs = {k: v.to(model.device) for k, v in inputs.items()}
# Generate
generated_ids = model.generate(**inputs, **generation_kwargs)
response = processor.batch_decode(generated_ids[:, inputs["input_ids"].shape[1]:], skip_special_tokens=True)
print("User: ", query1)
print("ASSISTANT: ", response[0])
### Round 2
messages.append(
{
"role": "assistant",
"content": [
{"type": "text", "text": response[0]},
]
}
)
messages.append(
{
"role": "user",
"content": [
{"type": "text", "text": query2},
]
}
)
prompt = processor.apply_chat_template(messages, add_generation_prompt=True)
inputs = processor(text=prompt, images=images, return_tensors="pt")
inputs = {k: v.to(model.device) for k, v in inputs.items()}
generated_ids = model.generate(**inputs, **generation_kwargs)
response = processor.batch_decode(generated_ids[:, inputs["input_ids"].shape[1]:], skip_special_tokens=True)
print("User: ", query2)
print("ASSISTANT: ", response[0])
### Round 3
messages.append(
{
"role": "assistant",
"content": [
{"type": "text", "text": response[0]},
]
}
)
messages.append(
{
"role": "user",
"content": [
{"type": "text", "text": query3},
]
}
)
prompt = processor.apply_chat_template(messages, add_generation_prompt=True)
inputs = processor(text=prompt, images=images, return_tensors="pt")
inputs = {k: v.to(model.device) for k, v in inputs.items()}
generated_ids = model.generate(**inputs, **generation_kwargs)
response = processor.batch_decode(generated_ids[:, inputs["input_ids"].shape[1]:], skip_special_tokens=True)
print("User: ", query3)
print("ASSISTANT: ", response[0])
"""
User: What cities image 1, image 2, and image 3 belong to respectively? Answer me in order.
ASSISTANT: Chicago, New York, San Francisco
User: Which one do you recommend for a visit? and why?
ASSISTANT: New York - because it's a bustling metropolis with iconic landmarks like the Statue of Liberty and the Empire State Building.
User: Which picture has most cars in it?
ASSISTANT: Image 3
"""
```
### Training
See [mantis/train](https://github.com/TIGER-AI-Lab/Mantis/tree/main/mantis/train) for details
### Evaluation
See [mantis/benchmark](https://github.com/TIGER-AI-Lab/Mantis/tree/main/mantis/benchmark) for details
**Please cite our paper or give a star to out Github repo if you find this model useful**
## Citation
```
@article{Jiang2024MANTISIM,
title={MANTIS: Interleaved Multi-Image Instruction Tuning},
author={Dongfu Jiang and Xuan He and Huaye Zeng and Cong Wei and Max W.F. Ku and Qian Liu and Wenhu Chen},
journal={Trans. Mach. Learn. Res.},
year={2024},
volume={2024},
url={Transactions on Machine Learning Research}
}
``` |