File size: 5,905 Bytes
9bcd499 eec132e 9bcd499 eec132e 9bcd499 4894f0e 9bcd499 e5de89b 9bcd499 960145a 9bcd499 |
1 2 3 4 5 6 7 8 9 10 11 12 13 14 15 16 17 18 19 20 21 22 23 24 25 26 27 28 29 30 31 32 33 34 35 36 37 38 39 40 41 42 43 44 45 46 47 48 49 50 51 52 53 54 55 56 57 58 59 60 61 62 63 64 65 66 67 68 69 70 71 72 73 74 75 76 77 78 79 80 81 82 83 84 85 86 87 88 89 90 91 92 93 94 95 96 97 98 99 100 101 102 103 104 105 106 107 108 109 110 111 112 113 114 115 116 117 118 119 120 121 122 123 124 125 126 127 128 129 130 |
---
license: apache-2.0
---
# Divot: Diffusion Powers Video Tokenizer for Comprehension and Generation
[](https://arxiv.org/abs/2412.04432)
[](https://github.com/TencentARC/Divot)
>We introduce [Divot](https://arxiv.org/abs/2412.04432), a **Di**ffusion-Powered **V**ide**o** **T**okenizer, which leverages the diffusion process for self-supervised video representation learning. We posit that if a video diffusion model can effectively de-noise video clips by taking the features of a video tokenizer as the condition, then the tokenizer has successfully captured robust spatial and temporal information. Additionally, the video diffusion model inherently functions as a de-tokenizer, decoding videos from their representations.
Building upon the Divot tokenizer, we present **Divot-LLM** through video-to-text autoregression and text-to-video generation by modeling the distributions of continuous-valued Divot features with a Gaussian Mixture Model.
All models, training code and inference code are released!
## TODOs
- [x] Release the pretrained tokenizer and de-tokenizer of Divot.
- [x] Release the pretrained and instruction tuned model of Divot-LLM.
- [x] Release inference code of Divot.
- [x] Release training and inference code of Divot-LLM.
- [ ] Release training code of Divot.
- [ ] Release de-tokenizer adaptation training code.
## Introduction
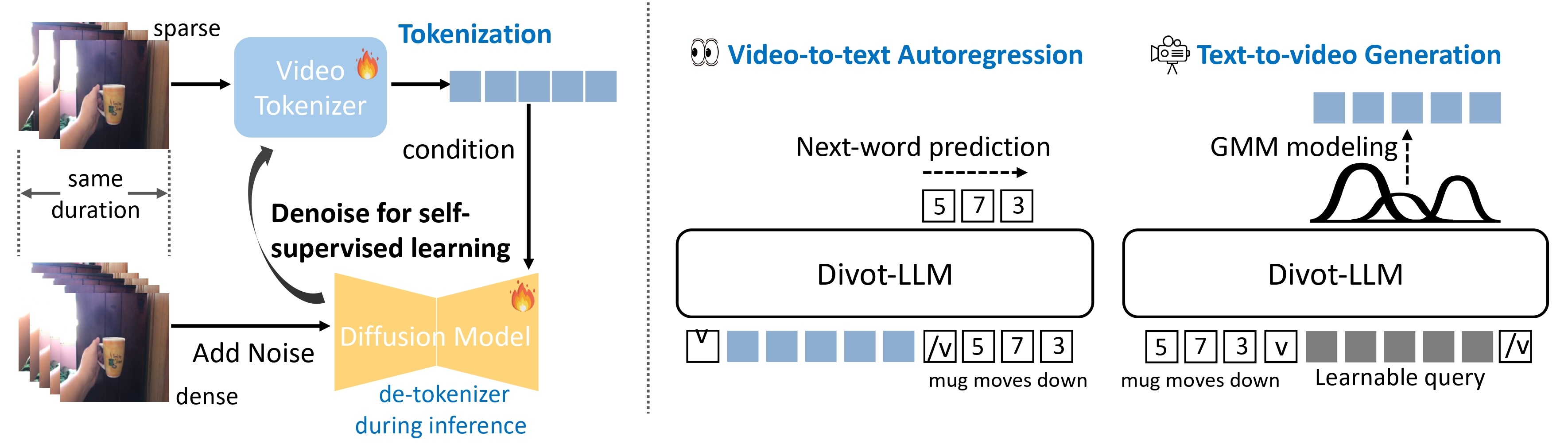
We utilize the diffusion procedure to learn **a video tokenizer** in a self-supervised manner for unified comprehension and
generation, where the spatiotemporal representations serve as the
condition of a diffusion model to de-noise video clips. Additionally,
the proxy diffusion model functions as a **de-tokenizer** to decode
realistic video clips from the video representations.
After training the the Divot tokenizer, video features from the Divot tokenizer are fed into the LLM to perform next-word prediction for video comprehension, while learnable queries are input into the LLM to model the distributions of Divot features using **a Gaussian Mixture Model (GMM)** for video generation. During inference,
video features are sampled from the predicted GMM distribution to
decode videos using the de-tokenizer.
## Usage
### Dependencies
- Python >= 3.8 (Recommend to use [Anaconda](https://www.anaconda.com/download/#linux))
- [PyTorch >=2.1.0](https://pytorch.org/)
- NVIDIA GPU + [CUDA](https://developer.nvidia.com/cuda-downloads)
### Installation
Clone the repo and install dependent packages
```bash
git clone https://github.com/TencentARC/Divot.git
cd Divot
pip install -r requirements.txt
```
### Model Weights
We release the pretrained tokenizer and de-tokenizer, pre-trained and instruction-tuned Divot-LLM. Please download the checkpoints and save them under the folder `./pretrained`. For example, `./pretrained/Divot_tokenizer_detokenizer`.
You also need to download [Mistral-7B-Instruct-v0.1](https://huggingface.co/mistralai/Mistral-7B-Instruct-v0.1) and [CLIP-ViT-H-14-laion2B-s32B-b79K](https://huggingface.co/laion/CLIP-ViT-H-14-laion2B-s32B-b79K), and save them under the folder `./pretrained`.
### Inference
#### Video Reconstruction with Divot
```bash
python3 src/tools/eval_Divot_video_recon.py
```
#### Video Comprehension with Divot-LLM
```bash
python3 src/tools/eval_Divot_video_comp.py
```
#### Video Generation with Divot-LLM
```bash
python3 src/tools/eval_Divot_video_gen.py
```
### Training
#### Pre-training
1. Download the checkpoints of pre-trained [Mistral-7B-Instruct-v0.1](https://huggingface.co/mistralai/Mistral-7B-Instruct-v0.1) and [CLIP-ViT-H-14-laion2B-s32B-b79K](https://huggingface.co/laion/CLIP-ViT-H-14-laion2B-s32B-b79K) , and save them under the folder `./pretrained`.
2. Prepare the training data in the format of webdataset.
3. Run the following script.
```bash
sh scripts/train_Divot_pretrain_comp_gen.sh
```
#### Instruction-tuning
1. Download the checkpoints of pre-trained Divot tokenizer and Divot-LLM in [Divot](https://huggingface.co/TencentARC/Divot/), and save them under the folder `./pretrained`.
2. Prepare the instruction data in the format of webdataset (for generation) and jsonl (for comprehension, where each line stores a dictionary used to specify the video_path, question, and answer).
3. Run the following script.
```bash
### For video comprehension
sh scripts/train_Divot_sft_comp.sh
### For video generation
sh scripts/train_Divot_sft_gen.sh
```
#### Inference with your own model
1. Obtain "pytorch_model.bin" with the following script.
```bash
cd train_output/sft_comp/checkpoint-xxxx
python3 zero_to_fp32.py . pytorch_model.bin
```
2. Merge your trained lora with the original LLM model using the following script.
```bash
python3 src/tools/merge_agent_lora_weight.py
```
3. Load your merged model in "mistral7b_merged_xxx" and and corresponding "agent" path, For example,
```bash
llm_cfg_path = 'configs/clm_models/mistral7b_merged_sft_comp.yaml'
agent_cfg_path = 'configs/clm_models/agent_7b_in64_out64_video_gmm_sft_comp.yaml'
```
## License
`Divot` is licensed under the Apache License Version 2.0 for academic purpose only except for the third-party components listed in [License](https://huggingface.co/TencentARC/Divot/blob/main/License.txt).
## Citation
If you find the work helpful, please consider citing:
```bash
@article{ge2024divot,
title={Divot: Diffusion Powers Video Tokenizer for Comprehension and Generation},
author={Ge, Yuying and Li, Yizhuo and Ge, Yixiao and Shan, Ying},
journal={arXiv preprint arXiv:2412.04432},
year={2024}
}
```
## Acknowledge
Our code for Divot tokenizer and de-tokenizer is built upon [DynamiCrafter](https://github.com/Doubiiu/DynamiCrafter). Thanks for their excellent work!
|