image
imagewidth (px) 18
939
| item_ID
stringlengths 13
13
| query
stringclasses 1
value | title
stringlengths 1
1.78k
⌀ | position
int64 0
0
|
---|---|---|---|---|
id-0002216949 | None | Ransom | 0 |
|
id-0006128513 | None | Caravan to Vaccares | 0 |
|
id-0006338658 | None | Chronicles of Wasted Time: The Infernal Grove v. 2 | 0 |
|
id-0006483682 | None | Dragonshadow | 0 |
|
id-0006511252 | None | Flashman (The Flashman Papers, Book 1) (The Flashman Papers) | 0 |
|
id-0006530451 | None | The Black Box | 0 |
|
id-0007138024 | None | The Megalithic European: The 21st Century Traveller in Prehistoric Europe | 0 |
|
id-0007145578 | None | Naked Empire - The Sword Of Truth, Book 8 | 0 |
|
id-0007156073 | None | 30-Minute-a-Day Body Challenge | 0 |
|
id-0007172354 | None | Q Pootle 5 | 0 |
|
id-0007177429 | None | People of the Book | 0 |
|
id-000719479X | None | The Long March | 0 |
|
id-0007214324 | None | The Broken Souls | 0 |
|
id-0007262620 | None | For the Love of Julie: A nightmare come true. A mother's courage. A desperate fight for justice. | 0 |
|
id-0007263783 | None | Please Don't Make Me Go: The True Story of the Little Boy Who Couldn't Be Beaten | 0 |
|
id-0007289944 | None | Tattoo Sourcebook | 0 |
|
id-000729204X | None | ELSPETH HUXLEY: A Biography | 0 |
|
id-0007457138 | None | BIG NATE BOREDOM BUSTER 2 PB | 0 |
|
id-0007583540 | None | Collins Very First French Dictionary (Collins Primary Dictionaries) | 0 |
|
id-0008128006 | None | A MIND OF YOUR OWN THE TRUTH A | 0 |
|
id-000820571X | None | Greek Audio Course (Collins Easy Learning Audio Course) | 0 |
|
id-000820666X | None | A Postcard from Italy: The most uplifting and escapist romance for 2023 from the No.1 bestseller (Book 1) | 0 |
|
id-0008215995 | None | Bloodchild | 0 |
|
id-000824121X | None | The Times Fiendish Su Doku Book 11: 200 Challenging Su Doku Puzzles | 0 |
|
id-0008359202 | None | Emperors of the Deep: The Mysterious and Misunderstood World of the Shark | 0 |
|
id-0008495742 | None | The Guilty Couple: The completely nail-biting, unputdownable crime thriller from the international million-copy bestseller | 0 |
|
id-0008527229 | None | BOSH! on a Budget: From the bestselling vegan authors comes the latest healthy plant-based, meat-free cookbook with new deliciously simple recipes | 0 |
|
id-002035441X | None | Ordeal of the Union Vol.1: Fruits of Manifest Destiny 1847-1852 : A House Dividing 1852-1857 | 0 |
|
id-0020354436 | None | Ordeal of the Union Vol. 3: The Improvised War 1861-1862; War Becomes Revolution 1862-1863 | 0 |
|
id-0020625804 | None | Birds of North America: Western Region : A Quick Identification Guide for All Bird-Watchers (Macmillan Field Guides) | 0 |
|
id-0021150214 | None | McGraw-Hill My Math, Grade 2, Student Edition, Volume 1 (ELEMENTARY MATH CONNECTS) | 0 |
|
id-0023611367 | None | C for Scientists and Engineers | 0 |
|
id-0023795204 | None | Writing of Economics | 0 |
|
id-0023882204 | None | Writing Exercises: Building, Combining, and Revising | 0 |
|
id-0024035459 | None | Approaches to Early Childhood Education | 0 |
|
id-0024174009 | None | Theory of Price | 0 |
|
id-0025074903 | None | Royal Service: My Twelve Years As Valet to Prince Charles | 0 |
|
id-0026123002 | None | Liquid Assets: How to Develop an Enjoyable and Profitable Wine Portfolio | 0 |
|
id-0028619897 | None | The Pro Football Encyclopedia: The Complete and Definitive Record of Professional Football | 0 |
|
id-0028720105 | None | Straight Life: The Story of Art Pepper | 0 |
|
id-0029183308 | None | Power and Influence | 0 |
|
id-0029268214 | None | Lives on the Boundary | 0 |
|
id-0030210291 | None | PKG:FINL MGMT 9E+STD SPDSHT APP DSK (Dryden Press Series in Finance) | 0 |
|
id-0030384427 | None | A Book of Short Stories 1 | 0 |
|
id-0030914566 | None | Soldier | 0 |
|
id-0046410120 | None | The art of vegetarian cookery | 0 |
|
id-0060000023 | None | Warriors: Into the Wild | 0 |
|
id-0060005556 | None | Last to Die (Jack Swyteck Novel) | 0 |
|
id-0060083700 | None | Rooms: Creating Luxurious, Livable Spaces (Design) | 0 |
|
id-0060083913 | None | How to Cook Revised Edition: An Easy and Imaginative Guide for the Beginner | 0 |
|
id-0060087919 | None | Shockproof Sydney Skate | 0 |
|
id-0060142774 | None | The Cuban Revolution | 0 |
|
id-0060147326 | None | No Chinese Stranger (1st Edition) | 0 |
|
id-0060154551 | None | A Christmas Treasury | 0 |
|
id-0060156783 | None | Make Way For Lucia: The Complete Lucia, Including Queen Lucia / Lucia in London / Miss Mapp / The Male Impersonator / Mapp and Lucia / The Worshipful Lucia / Trouble for Lucia | 0 |
|
id-0060199733 | None | Statecraft: Strategies for a Changing World | 0 |
|
id-0060236035 | None | The Rooster's Gift | 0 |
|
id-0060241020 | None | Sarah, Plain and Tall: A Newbery Award Winner (Sarah, Plain and Tall, 1) | 0 |
|
id-0060241039 | None | Back home | 0 |
|
id-0060263458 | None | Cowboys and Cattle Country (American Heritage Junior Library) | 0 |
|
id-006039157X | None | Think a Second Time | 0 |
|
id-0060439785 | None | Music: A Listener's Introduction | 0 |
|
id-0060502266 | None | A Game of Scandal | 0 |
|
id-0060538252 | None | She Comes First: The Thinking Man's Guide to Pleasuring a Woman | 0 |
|
id-0060560177 | None | Backstage Pass | 0 |
|
id-0060582723 | None | What's Right with Islam: A New Vision for Muslims and the West | 0 |
|
id-006058338X | None | The Berenstain Bears Play T-Ball (I Can Read Level 1) | 0 |
|
id-0060595353 | None | Homecourt Advantage | 0 |
|
id-0060621516 | None | The HarperCollins Concise Guide to World Religion: The A-to-Z Encyclopedia of All the Major Religious Traditions | 0 |
|
id-0060652578 | None | A man under orders: Lieutenant General William K. Harrison, Jr | 0 |
|
id-006075978X | None | Twins: A Novel | 0 |
|
id-006077620X | None | Sugar Rush | 0 |
|
id-0060783109 | None | The Dog Princess Fairy Tails | 0 |
|
id-006079335X | None | Prince Caspian (The Chronicles of Narnia) | 0 |
|
id-006082221X | None | Annette Vallon: A Novel of the French Revolution | 0 |
|
id-006082512X | None | The Big Book of Women Saints | 0 |
|
id-006083353X | None | The Loathsome Library: A Box of Unfortunate Events, Books 1-6 (The Bad Beginning; The Reptile Room; The Wide Window; The Miserable Mill; The Austere Academy; The Ersatz Elevator) | 0 |
|
id-0060846747 | None | Sex with the Queen: 900 Years of Vile Kings, Virile Lovers, and Passionate Politics (P.S.) | 0 |
|
id-0060846844 | None | Lost Girls and Love Hotels: A Novel | 0 |
|
id-0060851988 | None | Queen of Babble: A Novel | 0 |
|
id-006090691X | None | Self-exposures: A workbook in photographic self-portraiture | 0 |
|
id-0060910607 | None | Writing With a Word Processor | 0 |
|
id-0060924691 | None | Into The Garden: A Wedding Anthology: Poetry and Prose on Love and Marriage | 0 |
|
id-0060929103 | None | Absolute Beauty: Radiant Skin and Inner Harmony Through the Ancient Secrets of Ayurveda | 0 |
|
id-0060973293 | None | Market Wizards: Interviews with Top Traders | 0 |
|
id-0061004251 | None | Crazymaker: The Shocking True Story of Murder and Betrayal in an American Family | 0 |
|
id-0061053082 | None | Tapestries: An Anthology (Magic : The Gathering) | 0 |
|
id-0061080985 | None | Heart Sounds (Harper Monogram) | 0 |
|
id-006115430X | None | Universe of Stone: Chartres Cathedral and the Invention of the Gothic AKA Universe of Stone: A Biography of Chartres Cathedral | 0 |
|
id-0061189138 | None | El Ticket de Tu Vida | 0 |
|
id-0061251445 | None | Smithsonian Atlas of World Aviation | 0 |
|
id-0061256412 | None | Michael Tolliver Lives CD | 0 |
|
id-0061288845 | None | The Coffin Club (Vampire Kisses, Book 5) | 0 |
|
id-0061363936 | None | Run: A Novel | 0 |
|
id-0061379093 | None | Ana's Story: A Journey of Hope | 0 |
|
id-0061450650 | None | The Dress Doctor: Prescriptions for Style, From A to Z | 0 |
|
id-0061473723 | None | Zack's Alligator and the First Snow: A Winter and Holiday Book for Kids (I Can Read Level 2) | 0 |
|
id-0061478253 | None | Little Critter 12-Book Phonics Fun!: Includes 12 Mini-Books Featuring Short and Long Vowel Sounds (My First I Can Read) | 0 |
|
id-0061624810 | None | The Map of True Places: A Novel | 0 |
|
id-0061697214 | None | Broker, Trader, Lawyer, Spy: The Secret World of Corporate Espionage | 0 |
Marqo Ecommerce Embedding Models
In this work, we introduce the GoogleShopping-1m dataset for evaluation. This dataset comes with the release of our state-of-the-art embedding models for ecommerce products: Marqo-Ecommerce-B and Marqo-Ecommerce-L.
Released Content:
- Marqo-Ecommerce-B and Marqo-Ecommerce-L embedding models
- GoogleShopping-1m and AmazonProducts-3m for evaluation
- Evaluation Code
The benchmarking results show that the Marqo-Ecommerce models consistently outperformed all other models across various metrics. Specifically, marqo-ecommerce-L
achieved an average improvement of 17.6% in MRR and 20.5% in nDCG@10 when compared with the current best open source model, ViT-SO400M-14-SigLIP
across all three tasks in the marqo-ecommerce-hard
dataset. When compared with the best private model, Amazon-Titan-Multimodal
, we saw an average improvement of 38.9% in MRR and 45.1% in nDCG@10 across all three tasks, and 35.9% in Recall across the Text-to-Image tasks in the marqo-ecommerce-hard
dataset.
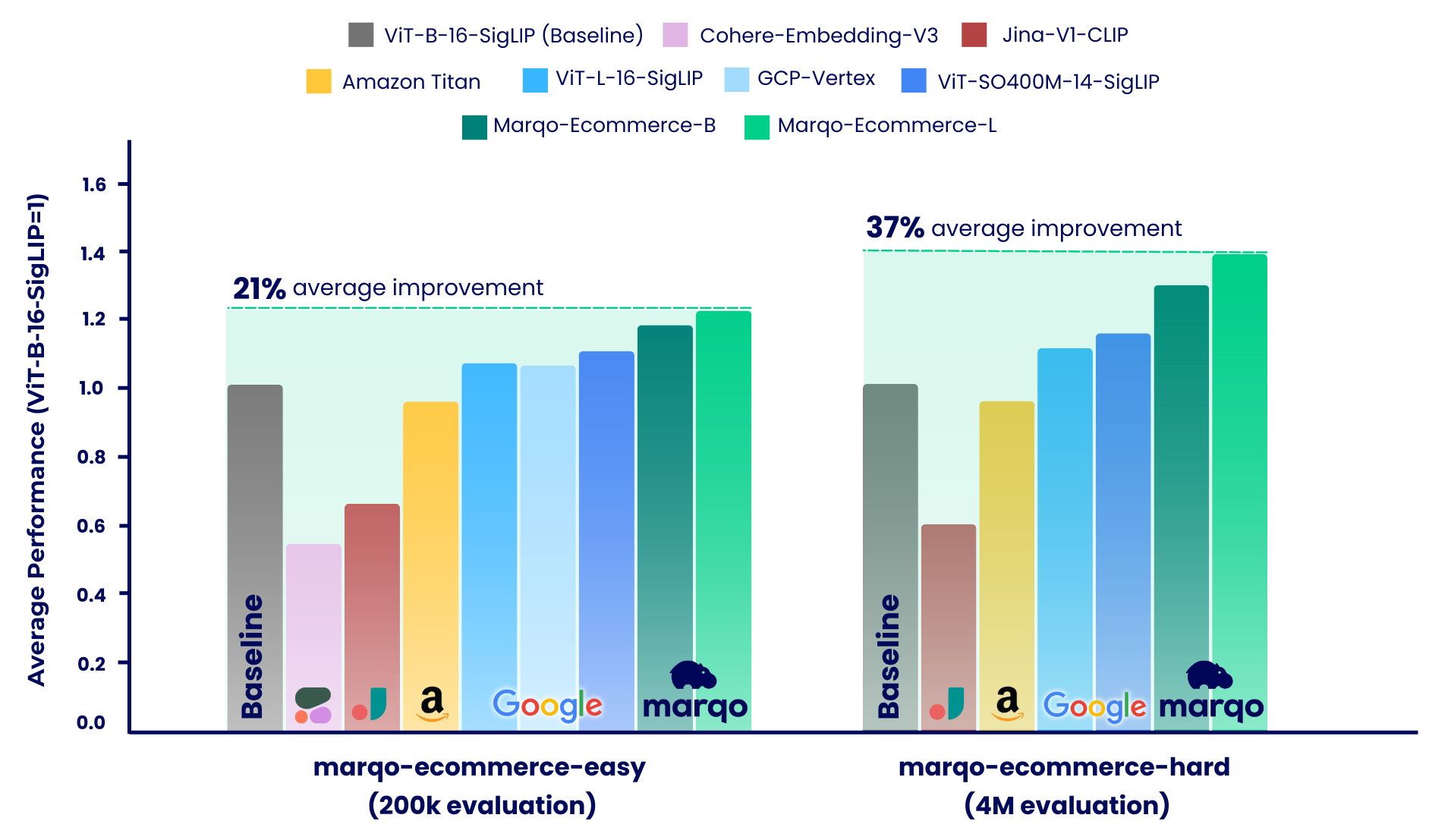
More benchmarking results can be found below.
Models
Embedding Model | #Params (m) | Dimension | HuggingFace | Download .pt |
---|---|---|---|---|
Marqo-Ecommerce-B | 203 | 768 | Marqo/marqo-ecommerce-embeddings-B | link |
Marqo-Ecommerce-L | 652 | 1024 | Marqo/marqo-ecommerce-embeddings-L | link |
Load from HuggingFace with transformers
To load the models in Transformers, see below. The models are hosted on Hugging Face and loaded using Transformers.
from transformers import AutoModel, AutoProcessor
import torch
from PIL import Image
import requests
model_name= 'Marqo/marqo-ecommerce-embeddings-L'
# model_name = 'Marqo/marqo-ecommerce-embeddings-B'
model = AutoModel.from_pretrained(model_name, trust_remote_code=True)
processor = AutoProcessor.from_pretrained(model_name, trust_remote_code=True)
img = Image.open(requests.get('https://raw.githubusercontent.com/marqo-ai/marqo-ecommerce-embeddings/refs/heads/main/images/dining-chairs.png', stream=True).raw).convert("RGB")
image = [img]
text = ["dining chairs", "a laptop", "toothbrushes"]
processed = processor(text=text, images=image, padding='max_length', return_tensors="pt")
processor.image_processor.do_rescale = False
with torch.no_grad():
image_features = model.get_image_features(processed['pixel_values'], normalize=True)
text_features = model.get_text_features(processed['input_ids'], normalize=True)
text_probs = (100 * image_features @ text_features.T).softmax(dim=-1)
print(text_probs)
# [1.0000e+00, 8.3131e-12, 5.2173e-12]
Load from HuggingFace with OpenCLIP
To load the models in OpenCLIP, see below. The models are hosted on Hugging Face and loaded using OpenCLIP. You can also find this code inside run_models.py
.
pip install open_clip_torch
from PIL import Image
import open_clip
import requests
import torch
# Specify model from Hugging Face Hub
model_name = 'hf-hub:Marqo/marqo-ecommerce-embeddings-L'
# model_name = 'hf-hub:Marqo/marqo-ecommerce-embeddings-B'
model, preprocess_train, preprocess_val = open_clip.create_model_and_transforms(model_name)
tokenizer = open_clip.get_tokenizer(model_name)
# Preprocess the image and tokenize text inputs
# Load an example image from a URL
img = Image.open(requests.get('https://raw.githubusercontent.com/marqo-ai/marqo-ecommerce-embeddings/refs/heads/main/images/dining-chairs.png', stream=True).raw)
image = preprocess_val(img).unsqueeze(0)
text = tokenizer(["dining chairs", "a laptop", "toothbrushes"])
# Perform inference
with torch.no_grad(), torch.cuda.amp.autocast():
image_features = model.encode_image(image, normalize=True)
text_features = model.encode_text(text, normalize=True)
# Calculate similarity probabilities
text_probs = (100.0 * image_features @ text_features.T).softmax(dim=-1)
# Display the label probabilities
print("Label probs:", text_probs)
# [1.0000e+00, 8.3131e-12, 5.2173e-12]
Evaluation
Generalised Contrastiove Learning (GCL) is used for the evaluation. The following code can also be found in scripts
.
git clone https://github.com/marqo-ai/GCL
Install the packages required by GCL.
1. GoogleShopping-Text2Image Retrieval.
cd ./GCL
MODEL=hf-hub:Marqo/marqo-ecommerce-B
outdir=/MarqoModels/GE/marqo-ecommerce-B/gs-title2image
hfdataset=Marqo/google-shopping-general-eval
python evals/eval_hf_datasets_v1.py \
--model_name $MODEL \
--hf-dataset $hfdataset \
--output-dir $outdir \
--batch-size 1024 \
--num_workers 8 \
--left-key "['title']" \
--right-key "['image']" \
--img-or-txt "[['txt'], ['img']]" \
--left-weight "[1]" \
--right-weight "[1]" \
--run-queries-cpu \
--top-q 4000 \
--doc-id-key item_ID \
--context-length "[[64], [0]]"
2. GoogleShopping-Category2Image Retrieval.
cd ./GCL
MODEL=hf-hub:Marqo/marqo-ecommerce-B
outdir=/MarqoModels/GE/marqo-ecommerce-B/gs-cat2image
hfdataset=Marqo/google-shopping-general-eval
python evals/eval_hf_datasets_v1.py \
--model_name $MODEL \
--hf-dataset $hfdataset \
--output-dir $outdir \
--batch-size 1024 \
--num_workers 8 \
--left-key "['query']" \
--right-key "['image']" \
--img-or-txt "[['txt'], ['img']]" \
--left-weight "[1]" \
--right-weight "[1]" \
--run-queries-cpu \
--top-q 4000 \
--doc-id-key item_ID \
--context-length "[[64], [0]]"
3. AmazonProducts-Category2Image Retrieval.
cd ./GCL
MODEL=hf-hub:Marqo/marqo-ecommerce-B
outdir=/MarqoModels/GE/marqo-ecommerce-B/ap-title2image
hfdataset=Marqo/amazon-products-eval
python evals/eval_hf_datasets_v1.py \
--model_name $MODEL \
--hf-dataset $hfdataset \
--output-dir $outdir \
--batch-size 1024 \
--num_workers 8 \
--left-key "['title']" \
--right-key "['image']" \
--img-or-txt "[['txt'], ['img']]" \
--left-weight "[1]" \
--right-weight "[1]" \
--run-queries-cpu \
--top-q 4000 \
--doc-id-key item_ID \
--context-length "[[64], [0]]"
Detailed Performance
Our benchmarking process was divided into two distinct regimes, each using different datasets of ecommerce product listings: marqo-ecommerce-hard and marqo-ecommerce-easy. Both datasets contained product images and text and only differed in size. The "easy" dataset is approximately 10-30 times smaller (200k vs 4M products), and designed to accommodate rate-limited models, specifically Cohere-Embeddings-v3 and GCP-Vertex (with limits of 0.66 rps and 2 rps respectively). The "hard" dataset represents the true challenge, since it contains four million ecommerce product listings and is more representative of real-world ecommerce search scenarios.
Within both these scenarios, the models were benchmarked against three different tasks:
- Google Shopping Text-to-Image
- Google Shopping Category-to-Image
- Amazon Products Text-to-Image
Marqo-Ecommerce-Hard
Marqo-Ecommerce-Hard looks into the comprehensive evaluation conducted using the full 4 million dataset, highlighting the robust performance of our models in a real-world context.
GoogleShopping-Text2Image Retrieval.
Embedding Model | mAP | R@10 | MRR | nDCG@10 |
---|---|---|---|---|
Marqo-Ecommerce-L | 0.682 | 0.878 | 0.683 | 0.726 |
Marqo-Ecommerce-B | 0.623 | 0.832 | 0.624 | 0.668 |
ViT-SO400M-14-SigLip | 0.573 | 0.763 | 0.574 | 0.613 |
ViT-L-16-SigLip | 0.540 | 0.722 | 0.540 | 0.577 |
ViT-B-16-SigLip | 0.476 | 0.660 | 0.477 | 0.513 |
Amazon-Titan-MultiModal | 0.475 | 0.648 | 0.475 | 0.509 |
Jina-V1-CLIP | 0.285 | 0.402 | 0.285 | 0.306 |
GoogleShopping-Category2Image Retrieval.
Embedding Model | mAP | P@10 | MRR | nDCG@10 |
---|---|---|---|---|
Marqo-Ecommerce-L | 0.463 | 0.652 | 0.822 | 0.666 |
Marqo-Ecommerce-B | 0.423 | 0.629 | 0.810 | 0.644 |
ViT-SO400M-14-SigLip | 0.352 | 0.516 | 0.707 | 0.529 |
ViT-L-16-SigLip | 0.324 | 0.497 | 0.687 | 0.509 |
ViT-B-16-SigLip | 0.277 | 0.458 | 0.660 | 0.473 |
Amazon-Titan-MultiModal | 0.246 | 0.429 | 0.642 | 0.446 |
Jina-V1-CLIP | 0.123 | 0.275 | 0.504 | 0.294 |
AmazonProducts-Text2Image Retrieval.
Embedding Model | mAP | R@10 | MRR | nDCG@10 |
---|---|---|---|---|
Marqo-Ecommerce-L | 0.658 | 0.854 | 0.663 | 0.703 |
Marqo-Ecommerce-B | 0.592 | 0.795 | 0.597 | 0.637 |
ViT-SO400M-14-SigLip | 0.560 | 0.742 | 0.564 | 0.599 |
ViT-L-16-SigLip | 0.544 | 0.715 | 0.548 | 0.580 |
ViT-B-16-SigLip | 0.480 | 0.650 | 0.484 | 0.515 |
Amazon-Titan-MultiModal | 0.456 | 0.627 | 0.457 | 0.491 |
Jina-V1-CLIP | 0.265 | 0.378 | 0.266 | 0.285 |
Marqo-Ecommerce-Easy
This dataset is about 10-30 times smaller than the Marqo-Ecommerce-Hard, and designed to accommodate rate-limited models, specifically Cohere-Embeddings-v3 and GCP-Vertex.
GoogleShopping-Text2Image Retrieval.
Embedding Model | mAP | R@10 | MRR | nDCG@10 |
---|---|---|---|---|
Marqo-Ecommerce-L | 0.879 | 0.971 | 0.879 | 0.901 |
Marqo-Ecommerce-B | 0.842 | 0.961 | 0.842 | 0.871 |
ViT-SO400M-14-SigLip | 0.792 | 0.935 | 0.792 | 0.825 |
GCP-Vertex | 0.740 | 0.910 | 0.740 | 0.779 |
ViT-L-16-SigLip | 0.754 | 0.907 | 0.754 | 0.789 |
ViT-B-16-SigLip | 0.701 | 0.870 | 0.701 | 0.739 |
Amazon-Titan-MultiModal | 0.694 | 0.868 | 0.693 | 0.733 |
Jina-V1-CLIP | 0.480 | 0.638 | 0.480 | 0.511 |
Cohere-embedding-v3 | 0.358 | 0.515 | 0.358 | 0.389 |
GoogleShopping-Category2Image Retrieval.
Embedding Model | mAP | P@10 | MRR | nDCG@10 |
---|---|---|---|---|
Marqo-Ecommerce-L | 0.515 | 0.358 | 0.764 | 0.590 |
Marqo-Ecommerce-B | 0.479 | 0.336 | 0.744 | 0.558 |
ViT-SO400M-14-SigLip | 0.423 | 0.302 | 0.644 | 0.487 |
GCP-Vertex | 0.417 | 0.298 | 0.636 | 0.481 |
ViT-L-16-SigLip | 0.392 | 0.281 | 0.627 | 0.458 |
ViT-B-16-SigLip | 0.347 | 0.252 | 0.594 | 0.414 |
Amazon-Titan-MultiModal | 0.308 | 0.231 | 0.558 | 0.377 |
Jina-V1-CLIP | 0.175 | 0.122 | 0.369 | 0.229 |
Cohere-embedding-v3 | 0.136 | 0.110 | 0.315 | 0.178 |
AmazonProducts-Text2Image Retrieval.
Embedding Model | mAP | R@10 | MRR | nDCG@10 |
---|---|---|---|---|
Marqo-Ecommerce-L | 0.92 | 0.978 | 0.928 | 0.940 |
Marqo-Ecommerce-B | 0.897 | 0.967 | 0.897 | 0.914 |
ViT-SO400M-14-SigLip | 0.860 | 0.954 | 0.860 | 0.882 |
ViT-L-16-SigLip | 0.842 | 0.940 | 0.842 | 0.865 |
GCP-Vertex | 0.808 | 0.933 | 0.808 | 0.837 |
ViT-B-16-SigLip | 0.797 | 0.917 | 0.797 | 0.825 |
Amazon-Titan-MultiModal | 0.762 | 0.889 | 0.763 | 0.791 |
Jina-V1-CLIP | 0.530 | 0.699 | 0.530 | 0.565 |
Cohere-embedding-v3 | 0.433 | 0.597 | 0.433 | 0.465 |
Citation
@software{zhu2024marqoecommembed_2024,
author = {Tianyu Zhu and and Jesse Clark},
month = oct,
title = {{Marqo Ecommerce Embeddings - Foundation Model for Product Embeddings}},
url = {https://github.com/marqo-ai/marqo-ecommerce-embeddings/},
version = {1.0.0},
year = {2024}
}
- Downloads last month
- 50