modelId
stringlengths 5
122
| author
stringlengths 2
42
| last_modified
unknown | downloads
int64 0
157M
| likes
int64 0
6.51k
| library_name
stringclasses 339
values | tags
sequencelengths 1
4.05k
| pipeline_tag
stringclasses 51
values | createdAt
unknown | card
stringlengths 1
913k
|
---|---|---|---|---|---|---|---|---|---|
Jukaboo/Llama2_7B_chat_arithmetic_nocarry | Jukaboo | "2024-01-02T11:42:23Z" | 0 | 0 | null | [
"tensorboard",
"safetensors",
"trl",
"sft",
"generated_from_trainer",
"base_model:meta-llama/Llama-2-7b-chat-hf",
"base_model:finetune:meta-llama/Llama-2-7b-chat-hf",
"region:us"
] | null | "2024-01-02T11:16:47Z" | ---
base_model: meta-llama/Llama-2-7b-chat-hf
tags:
- trl
- sft
- generated_from_trainer
model-index:
- name: Llama2_7B_chat_arithmetic_nocarry
results: []
---
<!-- This model card has been generated automatically according to the information the Trainer had access to. You
should probably proofread and complete it, then remove this comment. -->
# Llama2_7B_chat_arithmetic_nocarry
This model is a fine-tuned version of [meta-llama/Llama-2-7b-chat-hf](https://huggingface.co/meta-llama/Llama-2-7b-chat-hf) on the None dataset.
It achieves the following results on the evaluation set:
- Loss: 1.1935
## Model description
More information needed
## Intended uses & limitations
More information needed
## Training and evaluation data
More information needed
## Training procedure
### Training hyperparameters
The following hyperparameters were used during training:
- learning_rate: 0.002
- train_batch_size: 4
- eval_batch_size: 8
- seed: 42
- gradient_accumulation_steps: 4
- total_train_batch_size: 16
- optimizer: Adam with betas=(0.9,0.999) and epsilon=1e-08
- lr_scheduler_type: cosine
- lr_scheduler_warmup_ratio: 0.05
- num_epochs: 1
- mixed_precision_training: Native AMP
### Training results
| Training Loss | Epoch | Step | Validation Loss |
|:-------------:|:-----:|:----:|:---------------:|
| 0.5437 | 0.2 | 94 | 1.6203 |
| 0.499 | 0.4 | 188 | 2.2858 |
| 0.6523 | 0.6 | 282 | 1.6741 |
| 0.7247 | 0.8 | 376 | 1.1935 |
### Framework versions
- Transformers 4.35.2
- Pytorch 2.1.0+cu121
- Datasets 2.16.1
- Tokenizers 0.15.0
|
Silvers-145/mistral_7b_ticket | Silvers-145 | "2024-01-02T11:19:07Z" | 0 | 0 | null | [
"region:us"
] | null | "2024-01-02T11:19:07Z" | Entry not found |
ocutaminofficial/Ocutamin-review | ocutaminofficial | "2024-01-02T11:22:21Z" | 0 | 0 | null | [
"region:us"
] | null | "2024-01-02T11:21:57Z" | <p><a href="https://ocutamin-review.company.site/"><strong>Ocutamin</strong> </a>asserts itself as the inaugural all-natural solution designed to enhance vision without the need for medications or risky surgical procedures. It addresses the underlying factors contributing to poor eyesight, aiming to rectify issues solely through the use of natural ingredients.</p>
<h2><a href="https://www.globalfitnessmart.com/get-ocutamin"><strong>{</strong><strong>Ocutamin- Official Website -- Order Now}</strong></a></h2>
<h2><strong>➡️● For Order Official Website - <a href="https://www.globalfitnessmart.com/get-ocutamin">https://www.globalfitnessmart.com/get-ocutamin</a></strong><br /><strong>➡️● Item Name: — {<a href="https://www.globalfitnessmart.com/get-ocutamin">Ocutamin</a>}</strong><br /><strong>➡️● Ingredients: — All Natural</strong><br /><strong>➡️● Incidental Effects: — NA</strong><br /><strong>➡️● Accessibility: — <a href="https://www.globalfitnessmart.com/get-ocutamin">Online</a></strong></h2>
<h2><a href="https://www.globalfitnessmart.com/get-ocutamin"><strong>✅HUGE DISCOUNT ! HURRY UP! ORDER NOW!✅</strong></a><br /><a href="https://www.globalfitnessmart.com/get-ocutamin"><strong>✅HUGE DISCOUNT ! HURRY UP! ORDER NOW!✅</strong></a><br /><a href="https://www.globalfitnessmart.com/get-ocutamin"><strong>✅HUGE DISCOUNT ! HURRY UP! ORDER NOW!✅</strong></a></h2>
<div class="separator" style="clear: both; text-align: center;"><a style="margin-left: 1em; margin-right: 1em;" href="https://www.globalfitnessmart.com/get-ocutamin"><img src="https://blogger.googleusercontent.com/img/b/R29vZ2xl/AVvXsEi6fMN6eP2w2aWzlD20lXNjeC9khIkKrqpeQGdQafo5uZky94zbc2L9jUOPchIC0GWot_dC6BcSSMY_r13ceOr78u9HCyXEgYPkfEOFr50D57NivRpa0FYKNtLmBU37gyqO6uZAiStjh5Vrch6qW_U3djTQcD5sKrUCWeNqvqdcdWXaOL6XIwVZ3vLITwko/w640-h342/Ocutamin.jpg" alt="" width="640" height="342" border="0" data-original-height="423" data-original-width="790" /></a></div>
<h2><strong>What is <a href="https://groups.google.com/g/ocutamin/c/Ryoz9Suf-RY">Ocutamin</a> Dietary Supplement?</strong></h2>
<p><a href="https://sites.google.com/view/ocutamin-review-usa/home"><strong>Ocutamin</strong></a> is a daily supplement claiming to improve and fortify eye health. The formula is doctor-formulated and contains various nutrients to address the root of poor sight. The supplement is easy to swallow and can provide quality results in days.</p>
<p>According to the <a href="https://colab.research.google.com/drive/1jB-UNGMX6zTmxUioGB_s0BLe2NYQXlAB"><strong>Ocutamin</strong></a> website, the supplement has eight science-approved ingredients to manage eye issues. It is purportedly a safe, affordable, and effective solution to worsening eye health. It can prevent users from undergoing expensive Laser Eye Surgery (LASIK) or using contact lenses for the rest of their lives.</p>
<p>A former eye specialist Dr. Dean Avant is the formulator of <a href="https://lookerstudio.google.com/u/0/reporting/56d93833-e5a4-45dc-a27e-cbd11c011e07/page/KkTmD"><strong>Ocutamin</strong></a>. He experienced failing sight despite his knowledge and expertise. With another researcher, he discovered certain nutrients, including lutein and quercetin, that nurture the eyes and restore sight quickly.Today, thousands have tried the <a href="https://gamma.app/docs/Ocutamin-Pressura-Work-To-Promote-1Vision-Support-Formula-Reviews-i0d33n9jfq7fwyq?mode=doc"><strong>Ocutamin</strong></a> supplement, supposedly restoring their vision. The supplement is ideal for adults of all ages.</p>
<h2 style="text-align: center;"><a href="https://www.globalfitnessmart.com/get-ocutamin"><strong>(EXCLUSIVE OFFER)Click Here : "Ocutamin USA"Official Website!</strong></a></h2>
<h2><strong>How Does <a href="https://forum.mmm.ucar.edu/threads/ocutamin-pressura-work-to-promote-ocutamin-1vision-support-formula-united-states-canada-does-it-really-work.15058/">Ocutamin</a> Work?</strong></h2>
<p><a href="https://ocutamin-official.clubeo.com/calendar/2024/01/04/ocutamin-work-to-promote-restores-eyesight-united-states-canada-does-it-really-work"><strong>Ocutamin</strong></a>'s creator points out that modern problems like excessive use of computers, laptops, mobile phones, and TV is the primary cause of eye problems. In addition, environmental toxins, UV rays, foods, and water can damage the eyes.</p>
<p><a href="https://ocutamin-official.clubeo.com/page/ocutamin-pressura-work-to-promote-1vision-support-formula-reviews-scientifically-formulated-supplement.html"><strong>Ocutamin</strong></a> formulator reasons that ancestors enjoyed laser-sharp sight despite their age. They needed unfailing sight to gather food and protect themselves from animals. How did they maintain quality sight? Below is how <a href="https://ocutamin-official.clubeo.com/page/ocutamin-reviews-updated-2024-do-not-buy-till-you-read-this-updated-2024-do-not-buy-till-you-read-this.html"><strong>Ocutamin</strong></a> can support and restore sight</p>
<p><strong>Nourish the Eyes</strong> – Due to poor dietary patterns; most Americans cannot get sufficient vision-improving nutrients. Many homes eat junk and processed foods that increase inflammation and toxins in the eyes. <a href="https://ocutamin-official.clubeo.com/"><strong>Ocutamin</strong></a> has eight active ingredients that nourish the different eye cells, improving their function. The supplement can fight eye malnourishment.</p>
<p><strong>Clear Toxins</strong> – The environment is full of toxins. Avoiding some of these contaminants is impossible because they are in the air, foods, medicine, and cleaning products. <a href="https://www.scoop.it/topic/ocutamin-by-ocutamin-official"><strong>Ocutamin</strong></a> maker lists organophosphate (OP) as the most dangerous toxin that can damage the eye cells. The supplement has nutrients that enhance the cleansing and detoxification process. It can aid the body in eliminating toxins, thus improving sight.</p>
<p><strong>Fight Optic Atrophy</strong> – <a href="https://www.scoop.it/topic/ocutamin-eye-health-care-new-2024-advanced-formula"><strong>Ocutamin</strong></a> creator claims that most people do not utilize the eyes as required leading to optic atrophy. Studies show that people using their eyes actively, indoors and outdoors, train the different cells to become powerful. The supplement may strengthen the different eye parts.</p>
<p><strong>Refine Blood Circulation –</strong> Impaired blood flow in the eye restricts nutrient and oxygen intake. <a href="https://ocutamin-1.jimdosite.com/"><strong>Ocutamin</strong></a> can strengthen the eye capillaries and arteries, thus advancing blood circulation. The maker claims it may restore crystal-clear sight and prevent eye cells from dying.</p>
<p><strong>Improve Cellular Health</strong> – Some <a href="https://ocutamin.bandcamp.com/album/ocutamin-reviews-updated-2024-do-not-buy-till-you-read-this"><strong>Ocutamin</strong></a> ingredients are designed to support cellular regeneration and revitalization. It works by repairing different cells and preventing cellular decay. Consequently, it may protect the eyes from macular degeneration, cataracts, and other age-related sight problems.</p>
<div class="separator" style="clear: both; text-align: center;"><a style="margin-left: 1em; margin-right: 1em;" href="https://www.globalfitnessmart.com/get-ocutamin"><img src="https://blogger.googleusercontent.com/img/b/R29vZ2xl/AVvXsEgcEzO1QezmEbPhvZ4YrTSrDfWt3DwsUHsZ9SYa75ohTNJeaGZAP6KYoahtktMiNKNopFti7eQn1cFQ_HmNVi0cIJVK9Pky0pLr2x9FRsPdR52PctwzZpBwBEwhE98fMosHgyRFO58iM-Zqb55rQwCr7tkQk0VMewVisuL3uRZSufQ-bmFtL2HSSN1oDioF/w640-h376/EYE.jpg" alt="" width="640" height="376" border="0" data-original-height="422" data-original-width="720" /></a></div>
<h2><strong>Benefits Of Using <a href="https://soundcloud.com/ocutaminofficial/ocutamin-usa-is-legit-2024-updated-report">Ocutamin</a>:</strong></h2>
<p>OCUTAMIN's distinctive formulation offers a range of benefits that contribute to improved eye health and enhanced vision. These advantages include:</p>
<p><strong>Support Against Digital Eye Strain</strong>: In today's digital age, prolonged screen exposure often leads to digital eye strain. OCUTAMIN's blend of nutrients is designed to alleviate discomfort and mitigate the effects of eye strain associated with screen use.</p>
<p><strong>Protection from Age-related Vision Decline</strong>: The potent antioxidants found in OCUTAMIN, such as lutein and zeaxanthin, serve as a defense against age-related vision decline, fostering long-term eye health.</p>
<p><strong>Enhanced Night Vision</strong>: Featuring bilberry extract as a key component, OCUTAMIN draws on traditional uses to enhance night vision, allowing for clearer visibility in low-light conditions.</p>
<p><strong>Overall Visual Clarity:</strong> By supplying essential nutrients crucial for optimal eye function, OCUTAMIN may contribute to improved visual clarity and focus. This support helps you navigate the world with increased confidence.</p>
<h2 style="text-align: center;"><a href="https://www.globalfitnessmart.com/get-ocutamin"><strong>SPECIAL PROMO[Limited Discount]: "Ocutamin USA"Official Website!</strong></a></h2>
<h1><strong><a href="https://ocutamin.hashnode.dev/ocutamin-usa-is-legit-2024-updated-report">Ocutamin</a> Ingredients</strong></h1>
<p><a href="https://followme.tribe.so/post/ocutamin---usa-is-legit-2024-updated-report-6593bc86f64295489d92b9f1"><strong>Ocutamin</strong></a> is rich in natural ingredients that have undergone extensive research to affirm their effectiveness in enhancing vision. The different ingredients are purportedly in approved dosages and quantities to give users rapid results. The maker boldly claims that you can experience an improvement in eye health within a few days. Below are some of the active ingredients and their role in boosting sight.</p>
<p><strong>Quercetin</strong></p>
<p><a href="https://medium.com/@ocutaminofficial/ocutamin-usa-is-legit-2024-updated-report-12098509e48f"><strong>Ocutamin</strong></a> argues that most eye problems emanate from high toxin levels. The environment contains various chemicals, including OP, linked to severe vision problems. Scholarly studies show that people exposed to organophosphate have sight defects, including retinal degeneration, optic nerve atrophy, blurred vision, astigmatism, myopia, and optic disc edema.</p>
<p>Peer-reviewed studies show that quercetin may improve the strength and functions of neurotransmitters inside the retina. Additionally, the nutrient may restore sight, prevent optic atrophy, and enhance overall cellular health.</p>
<p><strong>Bilberry Fruit</strong></p>
<p>There are various scientific proofs that bilberry can improve vision. Historical reports show that British Royal Air Force pilots consumed the inky blue fruit to enhance their night vision and combat their enemies.</p>
<p>Bilberry is rich in anti-inflammatory and antioxidant components. It can eliminate pollutants reducing vision health. It can nourish every ocular cell, thus boosting its functions. Bilberry fruit can relax the blood capillaries in the eyes, thus enhancing nutrient intake and waste removal.</p>
<p><strong>Lutein</strong></p>
<p><a href="https://bitbucket.org/ocutamin/ocutamin/issues/1/ocutamin-work-to-promote-restores-eyesight"><strong>Ocutamin</strong></a> contains lutein from Marigold flowers. The nutrient is a natural anti-inflammatory that can combat optic atrophy problems. Studies show it can aid in the removal of toxins. Similarly, it can protect the eyes from UV rays and harmful blue wavelength light.Lutein can strengthen the muscles in the optic nerve, thus boosting its function. It can also enhance communication between the eyes and brain, enhancing vision.</p>
<div class="separator" style="clear: both; text-align: center;"><a style="margin-left: 1em; margin-right: 1em;" href="https://www.globalfitnessmart.com/get-ocutamin"><img src="https://blogger.googleusercontent.com/img/b/R29vZ2xl/AVvXsEj4G_Cw6H6VeNUwebIX_XlaimE4cZDI8bSLKMmLE7_8H3QDken2VOdGMwClWRjRcRxEHQNtwxpozXaArWepk2rNTmoe8eu9oYkxM4mbVnP9WweTDbgUPmQIy-ix7EOFzk3Ovsf9irq2GP1b4Z6k2-LNRNnpkD8xWF8j-zyFv9Oz-mz24QxXJYczH_MdIwJt/w640-h444/price02.jpg" alt="" width="640" height="444" border="0" data-original-height="560" data-original-width="809" /></a></div>
<h2><strong><a href="https://followme.tribe.so/post/ocutamin-work-to-promote-restores-eyesight-united-states-canada-does-it-rea--6593bd0602d8d6065bff9e12">Ocutamin</a> Dosage and Side Effects</strong></h2>
<p><a href="https://medium.com/@ocutaminofficial/ocutamin-work-to-promote-restores-eyesight-united-states-canada-does-it-really-work-54c725b1b601"><strong>Ocutamin</strong></a> recommends using one capsule daily. Customers can use the supplement at any time of the day. However, users should stay within the suggested dosages.</p>
<p>Side Effects – <a href="https://bitbucket.org/ocutamin/ocutamin/issues/2/ocutamin-reviews-updated-2024-do-not-buy"><strong>Ocutamin</strong></a> is natural and manufactured using pure ingredients. The formulator claims it cannot give users any side effects. Still, the manufacturer recommends seeking medical authorization before using the supplement. Consumers who experience adverse side effects should seek medical help and stop the dosage.</p>
<p>Place your order today before stock runs out!</p>
<h2><strong>Pros</strong></h2>
<p><strong>Clear vision:</strong> As the distortion, blurriness, flashes, and floaters gradually lessen, the clarity of vision is no longer an issue.</p>
<p><strong>No surgery:</strong> If the damage can be repaired naturally, there is no need for surgery, which can save time and money.</p>
<p><strong>No glasses or lenses:</strong> After taking <a href="https://haitiliberte.com/advert/ocutamin-usa-is-legit-2024-updated-report/"><strong>Ocutamin</strong></a> for a while, the need for vision aids decreases.</p>
<p><strong>Protection from the sun:</strong> <a href="https://haitiliberte.com/advert/ocutamin-usa-is-legit-2024-updated-report/"><strong>Ocutamin</strong></a> components also assist to lessen light sensitivity and sun damage.</p>
<p><strong>Better vision and focus:</strong> The eyes can see clearly and with complete focus.</p>
<h2><strong>Cons</strong></h2>
<p><strong>Limited accessibility:</strong> this product may only be purchased online and is not offered by nearby vendors, pharmacies, or shops.</p>
<p><strong>Variable results:</strong> depending on how the body responds, results may vary across users and take many months.</p>
<p><strong>Not a medication:<a href="https://grabcad.com/library/ocutamin-eye-health-care-new-2024-advanced-formula-1"> Ocutamin</a></strong> is a dietary supplement that promotes eye health but is not a medication. It does not treat anything and cannot be used in place of medicine.</p>
<h2 style="text-align: center;"><strong><a href="https://www.globalfitnessmart.com/get-ocutamin">SPECIAL PROMO: Get Ocutamin at the Lowest Discounted Price Online</a></strong></h2>
<h2><strong>FAQs about <a href="https://the-dots.com/projects/ocutamin-pressura-work-to-promote-1vision-support-formula-reviews-scientifically-formulated-supplement-1007374">Ocutamin</a> Supplement</strong></h2>
<p><strong>Q: What causes poor sight?</strong></p>
<p>A: According to <a href="http://kaymakgames.com/forum/index.php?thread/40447-ocutamin-pressura-work-to-promote-1vision-support-formula-reviews-scientifically/"><strong>Ocutamin</strong></a>, too much screen time, low water intake, poor diet, sleep deficiency, and unhealthy lifestyle habits are the leading causes of eye problems.</p>
<p><strong>Q: Can I inherit eye problems?</strong></p>
<p>A: Some eye issues like hyperopia and myopia are genetically linked. However, experts claim you can prevent the development of these eye problems by maintaining a healthy diet and good eye hygiene.</p>
<p><strong>Q: Can <a href="https://the-dots.com/projects/ocutamin-usa-is-legit-2024-updated-report-1007373">Ocutamin</a> improve eyesight?</strong></p>
<p>A: <a href="http://kaymakgames.com/forum/index.php?thread/40445-ocutamin-usa-is-legit-2024-updated-report/"><strong>Ocutamin</strong></a> is not a quick fix to better vision. The manufacturer recommends using it consistently for extended periods to nourish the eyes and improve sight.</p>
<p><strong>Q: Does Ocutamin interact with other medications?</strong></p>
<p>A: The maker recommends seeking medical guidance before using the supplement.</p>
<p><strong>Q: Who can use the <a href="https://www.eventcreate.com/e/ocutamin">Ocutamin</a> supplement?</strong></p>
<p>A: <a href="https://huggingface.co/datasets/ocutaminofficial/ocutamin/blob/main/README.md"><strong>Ocutamin</strong></a> is marketed for anyone experiencing vision problems, including blurry eyes and poor sight.</p>
<p><strong>Q: Can children use <a href="https://www.c-sharpcorner.com/article/ocutamin-eye-health-care-new-2024-advanced-formula/">Ocutamin</a>?</strong></p>
<p>A: No, <a href="https://rapbeatsforum.com/viewtopic.php?t=73634"><strong>Ocutamin</strong></a> is only for adult men and women.</p>
<p><strong>Q: What ingredients are inside <a href="https://forum.teknofest.az/d/13429-ocutamin-eye-health-care-new-2024-advanced-formula">Ocutamin</a>?</strong></p>
<p>A: <a href="https://oqqur.tribe.so/post/ocutamin-eye-health-care-new-2024-advanced-formula-6593d44914a1fa006ec92032"><strong>Ocutamin</strong></a> has eight ingredients, including bilberry fruit extract, lutein, and quercetin.</p>
<p><strong>Q: How long should I use the <a href="https://www.c-sharpcorner.com/article/ocutamin-usa-is-legit-2024-updated-report/">Ocutamin</a> supplement?</strong></p>
<p>A: The manufacturer suggests using it for over three months.</p>
<p><strong>Q: Is <a href="https://forum.teknofest.az/d/13427-ocutamin-usa-is-legit-2024-updated-report">Ocutamin</a> addictive?</strong></p>
<p>A: <a href="https://oqqur.tribe.so/post/ocutamin---usa-is-legit-2024-updated-report-6593d309c9c8c3537bbf25d6"><strong>Ocutamin</strong></a> is supposedly free from stimulants and thus unlikely to cause addiction even with prolonged usage. However, the maker recommends taking a two-week break after every three months.</p>
<p><strong>Q: What if <a href="https://bookshop.org/wishlists/d80727f710a5264110b72e6fe411e2ae7958e123">Ocutamin</a> fails to work?</strong></p>
<p>A: <a href="https://leetcode.com/discuss/interview-question/4491223/Ocutamin1-Vision-Support-Formula"><strong>Ocutamin</strong></a> comes with a 60-day money-back guarantee. Customers can request a refund if they experience no improvement in their vision within the stipulated period.</p>
<div class="separator" style="clear: both; text-align: center;"><a style="margin-left: 1em; margin-right: 1em;" href="https://www.globalfitnessmart.com/get-ocutamin"><img src="https://blogger.googleusercontent.com/img/b/R29vZ2xl/AVvXsEizRoy7i90SUHcWn2w9-dLmxhFC8UunAXs4cG9tD45sHT-0rXnBLzyzQDwqULKLAKsDuQqy1020-FlXTJh38IYPQt8LOoyYisAB4iQhAA1Je-apBelAMW8si0PiYi8VPoTSejn5sdXRmQaqL2tncgi9AWYwLYVTLrpNHLSTd500oNRDl_cJqpTQERjBrCUw/w640-h483/Ocutamin04.jpg" alt="" width="640" height="483" border="0" data-original-height="424" data-original-width="562" /></a></div>
<h1><strong>Pricing</strong></h1>
<p><strong><a href="https://wandering.flarum.cloud/d/35305-ocutamin1-vision-support-formula">Ocutamin</a></strong> is only available through the official website. The manufacturer warns against buying from third parties. Customers can buy a one-month- six-month package depending on their budget. However, multiple buys come with free shipping and price reduction.</p>
<p><a href="https://bookshop.org/wishlists/7b030215c10d2bce3555aaa3b68625bc343bab23"><strong>Ocutamin</strong></a> is being sold currently at a discount offer. The pricing of <a href="https://community.thebatraanumerology.com/post/ocutamin-1-vision-support-formula-6593c213d4d0ed7307dad45c"><strong>Ocutamin</strong></a> is as follows:</p>
<ul>
<li><strong>Order one bottle of <a href="https://leetcode.com/discuss/interview-question/4491198/Ocutamin-USA-*IS-Legit*-2024-Updated-Report!">Ocutamin </a>and pay $69.00 and a small shipping fee. You save $30 off the regular retail price of $99.</strong></li>
<li><strong>Three-bottle bundle and pay $59.00 each (order total $177). You save $120 off the regular retail price of $297. There’s free US shipping included with your order.</strong></li>
<li><strong>A six-bottle bundle is $49.00 each (order total $294). You save $300 off the regular retail price of $594. There’s free US shipping included with your order.</strong></li>
</ul>
<h2><strong>Conclusion</strong></h2>
<p><a href="https://community.thebatraanumerology.com/post/ocutamin---usa-is-legit-2024-updated-report-6593c1648f5b2c0a5837c75d"><strong>Ocutamin</strong></a> is a dietary supplement that promotes the health of the macular, retina, and optic nerve. <a href="https://public.flourish.studio/visualisation/16316331/"><strong>Ocutamin</strong></a>'s makers also assert that it can enhance vision and lower the risk of age-related eye conditions. However, these statements are not backed by any scientific data. Ocutamin's long-term safety is also unknown because peer evaluations have not endorsed it. This supplement should not be taken by women who are pregnant, nursing, under 18, or who have a significant medical condition.</p>
<div class="separator" style="clear: both; text-align: center;"><a style="margin-left: 1em; margin-right: 1em;" href="https://www.globalfitnessmart.com/get-ocutamin"><img src="https://blogger.googleusercontent.com/img/b/R29vZ2xl/AVvXsEidqnej3Jsvo3-m6tBgDiGfpvcmY7IVp6MYf-iS1XKFgdTIC0dHaXGrtpSvcZUJcMQW-SjO793kZGPR8H9erSoH7AC_zi2m_NSIEr7RoRwXP46pS1Coe_V6ckKtmLsg7VKdBee1ntF35YgG8Ap1PII2lxA34rjDFq4F1a7drjMPMMzef8Xkkq3aL3ezoTEy/w640-h380/PRICE%2001.jpg" alt="" width="640" height="380" border="0" data-original-height="472" data-original-width="795" /></a></div>
<h2 style="text-align: center;"><strong><a href="https://www.globalfitnessmart.com/get-ocutamin">Exclive Details: *Ocutamin* Read More Details on Official Website USA!</a></strong></h2>
<h2># READ MORE</h2>
<p><a href="https://myhealthfitnessmart.blogspot.com/2024/01/ocutamin.html">https://myhealthfitnessmart.blogspot.com/2024/01/ocutamin.html</a></p>
<p><a href="https://ocutamin-review.company.site/">https://ocutamin-review.company.site/</a></p>
<p><a href="https://groups.google.com/g/ocutamin/c/Ryoz9Suf-RY">https://groups.google.com/g/ocutamin/c/Ryoz9Suf-RY</a></p>
<p><a href="https://sites.google.com/view/ocutamin-review-usa/home">https://sites.google.com/view/ocutamin-review-usa/home</a></p>
<p><a href="https://colab.research.google.com/drive/1jB-UNGMX6zTmxUioGB_s0BLe2NYQXlAB">https://colab.research.google.com/drive/1jB-UNGMX6zTmxUioGB_s0BLe2NYQXlAB</a></p>
<p><a href="https://lookerstudio.google.com/u/0/reporting/56d93833-e5a4-45dc-a27e-cbd11c011e07/page/KkTmD">https://lookerstudio.google.com/u/0/reporting/56d93833-e5a4-45dc-a27e-cbd11c011e07/page/KkTmD</a></p>
<p><a href="https://www.scoop.it/topic/ocutamin-by-ocutamin-official">https://www.scoop.it/topic/ocutamin-by-ocutamin-official</a></p>
<p><a href="https://ocutamin-official.clubeo.com/">https://ocutamin-official.clubeo.com/</a></p>
<p><a href="https://ocutamin-official.clubeo.com/page/ocutamin-reviews-updated-2024-do-not-buy-till-you-read-this-updated-2024-do-not-buy-till-you-read-this.html">https://ocutamin-official.clubeo.com/page/ocutamin-reviews-updated-2024-do-not-buy-till-you-read-this-updated-2024-do-not-buy-till-you-read-this.html</a></p>
<p><a href="https://ocutamin-official.clubeo.com/page/ocutamin-pressura-work-to-promote-1vision-support-formula-reviews-scientifically-formulated-supplement.html">https://ocutamin-official.clubeo.com/page/ocutamin-pressura-work-to-promote-1vision-support-formula-reviews-scientifically-formulated-supplement.html</a></p>
<p><a href="https://ocutamin-official.clubeo.com/calendar/2024/01/04/ocutamin-work-to-promote-restores-eyesight-united-states-canada-does-it-really-work">https://ocutamin-official.clubeo.com/calendar/2024/01/04/ocutamin-work-to-promote-restores-eyesight-united-states-canada-does-it-really-work</a></p>
<p><a href="https://forum.mmm.ucar.edu/threads/ocutamin-pressura-work-to-promote-ocutamin-1vision-support-formula-united-states-canada-does-it-really-work.15058/">https://forum.mmm.ucar.edu/threads/ocutamin-pressura-work-to-promote-ocutamin-1vision-support-formula-united-states-canada-does-it-really-work.15058/</a></p>
<p><a href="https://gamma.app/docs/Ocutamin-Pressura-Work-To-Promote-1Vision-Support-Formula-Reviews-i0d33n9jfq7fwyq?mode=doc">https://gamma.app/docs/Ocutamin-Pressura-Work-To-Promote-1Vision-Support-Formula-Reviews-i0d33n9jfq7fwyq?mode=doc</a></p>
<p><a href="https://soundcloud.com/ocutaminofficial/ocutamin-usa-is-legit-2024-updated-report">https://soundcloud.com/ocutaminofficial/ocutamin-usa-is-legit-2024-updated-report</a></p>
<p><a href="https://ocutamin.bandcamp.com/album/ocutamin-reviews-updated-2024-do-not-buy-till-you-read-this">https://ocutamin.bandcamp.com/album/ocutamin-reviews-updated-2024-do-not-buy-till-you-read-this</a></p>
<p><a href="https://ocutamin-1.jimdosite.com/">https://ocutamin-1.jimdosite.com/</a></p>
<p><a href="https://bitbucket.org/ocutamin/ocutamin/issues/2/ocutamin-reviews-updated-2024-do-not-buy">https://bitbucket.org/ocutamin/ocutamin/issues/2/ocutamin-reviews-updated-2024-do-not-buy</a></p>
<p><a href="https://medium.com/@ocutaminofficial/ocutamin-work-to-promote-restores-eyesight-united-states-canada-does-it-really-work-54c725b1b601">https://medium.com/@ocutaminofficial/ocutamin-work-to-promote-restores-eyesight-united-states-canada-does-it-really-work-54c725b1b601</a></p>
<p><a href="https://followme.tribe.so/post/ocutamin-work-to-promote-restores-eyesight-united-states-canada-does-it-rea--6593bd0602d8d6065bff9e12">https://followme.tribe.so/post/ocutamin-work-to-promote-restores-eyesight-united-states-canada-does-it-rea--6593bd0602d8d6065bff9e12</a></p>
<p><a href="https://public.flourish.studio/visualisation/16316331/">https://public.flourish.studio/visualisation/16316331/</a></p>
<p><a href="https://pdfhost.io/v/cf1sceR3l_Ocutamin_USA_IS_Legit_2024_Updated_Report">https://pdfhost.io/v/cf1sceR3l_Ocutamin_USA_IS_Legit_2024_Updated_Report</a></p>
<p><a href="https://community.thebatraanumerology.com/post/ocutamin---usa-is-legit-2024-updated-report-6593c1648f5b2c0a5837c75d">https://community.thebatraanumerology.com/post/ocutamin---usa-is-legit-2024-updated-report-6593c1648f5b2c0a5837c75d</a></p>
<p><a href="https://wandering.flarum.cloud/d/35304-ocutamin-usa-is-legit-2024-updated-report">https://wandering.flarum.cloud/d/35304-ocutamin-usa-is-legit-2024-updated-report</a></p>
<p><a href="https://leetcode.com/discuss/interview-question/4491198/Ocutamin-USA-*IS-Legit*-2024-Updated-Report!">https://leetcode.com/discuss/interview-question/4491198/Ocutamin-USA-*IS-Legit*-2024-Updated-Report!</a></p>
<p><a href="https://community.thebatraanumerology.com/post/ocutamin-1-vision-support-formula-6593c213d4d0ed7307dad45c">https://community.thebatraanumerology.com/post/ocutamin-1-vision-support-formula-6593c213d4d0ed7307dad45c</a></p>
<p><a href="https://wandering.flarum.cloud/d/35305-ocutamin1-vision-support-formula">https://wandering.flarum.cloud/d/35305-ocutamin1-vision-support-formula</a></p>
<p><a href="https://forum.teknofest.az/d/13427-ocutamin-usa-is-legit-2024-updated-report">https://forum.teknofest.az/d/13427-ocutamin-usa-is-legit-2024-updated-report</a></p>
<p><a href="https://www.c-sharpcorner.com/article/ocutamin-usa-is-legit-2024-updated-report/">https://www.c-sharpcorner.com/article/ocutamin-usa-is-legit-2024-updated-report/</a></p>
<p><a href="https://huggingface.co/datasets/ocutaminofficial/ocutamin/blob/main/README.md">https://huggingface.co/datasets/ocutaminofficial/ocutamin/blob/main/README.md</a></p>
<p><a href="https://huggingface.co/ocutaminofficial/ocutamin/blob/main/README.md">https://huggingface.co/ocutaminofficial/ocutamin/blob/main/README.md</a></p>
<p><a href="https://www.eventcreate.com/e/ocutamin">https://www.eventcreate.com/e/ocutamin</a></p>
<p><a href="http://kaymakgames.com/forum/index.php?thread/40447-ocutamin-pressura-work-to-promote-1vision-support-formula-reviews-scientifically/">http://kaymakgames.com/forum/index.php?thread/40447-ocutamin-pressura-work-to-promote-1vision-support-formula-reviews-scientifically/</a></p>
<p><a href="https://haitiliberte.com/advert/ocutamin-usa-is-legit-2024-updated-report/">https://haitiliberte.com/advert/ocutamin-usa-is-legit-2024-updated-report/</a></p>
<p><a href="https://grabcad.com/library/ocutamin-eye-health-care-new-2024-advanced-formula-1">https://grabcad.com/library/ocutamin-eye-health-care-new-2024-advanced-formula-1</a></p>
<p><a href="https://sketchfab.com/3d-models/ocutamin-usa-is-legit-2024-updated-report-4c2dd7484e7c405b8cbfb4b2c07b7793">https://sketchfab.com/3d-models/ocutamin-usa-is-legit-2024-updated-report-4c2dd7484e7c405b8cbfb4b2c07b7793</a></p>
<p> </p> |
buruzaemon/smithsonian_butterflies_subset | buruzaemon | "2024-01-02T12:32:08Z" | 0 | 0 | null | [
"tensorboard",
"region:us"
] | null | "2024-01-02T11:27:39Z" | Entry not found |
ParazaxComplex/ParazaxComplexSwitzerland | ParazaxComplex | "2024-01-02T11:30:21Z" | 0 | 0 | null | [
"region:us"
] | null | "2024-01-02T11:27:49Z" | <a href="https://www.boxdrug.com/ParaCompSwitz">Parazax Complex Kaufe jetzt!! Klicken Sie auf den Link unten für weitere Informationen und erhalten Sie jetzt 50 % Rabatt!! Beeil dich !!</a>
Offizielle Website: <a href="https://www.boxdrug.com/ParaCompSwitz">www.ParazaxComplex.com</a>
<p><a href="https://www.boxdrug.com/ParaCompSwitz"> <img src="https://blogger.googleusercontent.com/img/b/R29vZ2xl/AVvXsEg5gBAL6Bmx-9Qp2ZGkLBCb-pEhOpXQUNi5MFy3QEr4cr6k7fuy_soI2IEDw7NoxWOBmeKYkLPeWU19TneK6aRsZWk5J3hWBY-yZDIHaJ5RNnRFaFGLJzL2T0gY_ocwLsijfnHVo7vmcOJqh4DH5dCvNZcBmler7aGmS7DIzYQBSrm8W_0m9XeRsUzY1dU/w643-h354/Parazax%20Complex%20switzerland%201.png" alt="enter image description here"> </a></p>
Parazax Complex ist ausdrücklich für die Magengesundheit gedacht, die darauf abzielt, Ihr Magensystem zu verbessern und eine geringe gastrointestinale Bakterienzahl, Parasiten, Magenbeschwerden, Gewichtszunahme und andere damit verbundene Komplikationen einzuschließen. Parazax Complex Switzerland!
➢Produktname – Parazax Complex
➢Kategorie – Parasitenkapsel
➢Hauptvorteile – dieses Produkt fördert eine bessere Verdauungsfunktion
➢ Zusammensetzung – Natürliche organische Verbindung
➢ Nebenwirkungen – Nicht zutreffend
➢Endgültige Bewertung: – 4,8
➢ Verfügbarkeit – Online
➢Angebote und Rabatte; SPAREN SIE HEUTE! JETZT EINKAUFEN, UM SONDERANGEBOT zu kaufen!!!
Was ist Parazax Complex?
Parazax Complex ist ein einzigartiges, alltägliches magenbezogenes Präparat, das sorgfältig entwickelt wurde, um schädliche Mikroorganismen und andere Mikroorganismen gezielt zu bekämpfen und gleichzeitig das Magenmikrobiom zu stärken und die Vertrauenswürdigkeit der Magengrenze zu unterstützen. Dieses beispiellose Rezept wurde in Zusammenarbeit mit dem renommierten Experten für Magengesundheit entwickelt.
<a href="https://www.boxdrug.com/ParaCompSwitz">Parazax Complex Kaufe jetzt!! Klicken Sie auf den Link unten für weitere Informationen und erhalten Sie jetzt 50 % Rabatt!! Beeil dich !!</a>
Offizielle Website: <a href="https://www.boxdrug.com/ParaCompSwitz">www.ParazaxComplex.com</a>
<p><a href="https://www.boxdrug.com/ParaCompSwitz"> <img src="https://blogger.googleusercontent.com/img/b/R29vZ2xl/AVvXsEg5gBAL6Bmx-9Qp2ZGkLBCb-pEhOpXQUNi5MFy3QEr4cr6k7fuy_soI2IEDw7NoxWOBmeKYkLPeWU19TneK6aRsZWk5J3hWBY-yZDIHaJ5RNnRFaFGLJzL2T0gY_ocwLsijfnHVo7vmcOJqh4DH5dCvNZcBmler7aGmS7DIzYQBSrm8W_0m9XeRsUzY1dU/w643-h354/Parazax%20Complex%20switzerland%201.png" alt="enter image description here"> </a></p>
|
behzadnet/Llama-2-7b-chat-hf-sharded-bf16-fine-tuned-adapters_humanMix_Seed112 | behzadnet | "2024-01-02T11:29:59Z" | 0 | 0 | peft | [
"peft",
"arxiv:1910.09700",
"base_model:Trelis/Llama-2-7b-chat-hf-sharded-bf16",
"base_model:adapter:Trelis/Llama-2-7b-chat-hf-sharded-bf16",
"region:us"
] | null | "2024-01-02T11:29:54Z" | ---
library_name: peft
base_model: Trelis/Llama-2-7b-chat-hf-sharded-bf16
---
# Model Card for Model ID
<!-- Provide a quick summary of what the model is/does. -->
## Model Details
### Model Description
<!-- Provide a longer summary of what this model is. -->
- **Developed by:** [More Information Needed]
- **Shared by [optional]:** [More Information Needed]
- **Model type:** [More Information Needed]
- **Language(s) (NLP):** [More Information Needed]
- **License:** [More Information Needed]
- **Finetuned from model [optional]:** [More Information Needed]
### Model Sources [optional]
<!-- Provide the basic links for the model. -->
- **Repository:** [More Information Needed]
- **Paper [optional]:** [More Information Needed]
- **Demo [optional]:** [More Information Needed]
## Uses
<!-- Address questions around how the model is intended to be used, including the foreseeable users of the model and those affected by the model. -->
### Direct Use
<!-- This section is for the model use without fine-tuning or plugging into a larger ecosystem/app. -->
[More Information Needed]
### Downstream Use [optional]
<!-- This section is for the model use when fine-tuned for a task, or when plugged into a larger ecosystem/app -->
[More Information Needed]
### Out-of-Scope Use
<!-- This section addresses misuse, malicious use, and uses that the model will not work well for. -->
[More Information Needed]
## Bias, Risks, and Limitations
<!-- This section is meant to convey both technical and sociotechnical limitations. -->
[More Information Needed]
### Recommendations
<!-- This section is meant to convey recommendations with respect to the bias, risk, and technical limitations. -->
Users (both direct and downstream) should be made aware of the risks, biases and limitations of the model. More information needed for further recommendations.
## How to Get Started with the Model
Use the code below to get started with the model.
[More Information Needed]
## Training Details
### Training Data
<!-- This should link to a Data Card, perhaps with a short stub of information on what the training data is all about as well as documentation related to data pre-processing or additional filtering. -->
[More Information Needed]
### Training Procedure
<!-- This relates heavily to the Technical Specifications. Content here should link to that section when it is relevant to the training procedure. -->
#### Preprocessing [optional]
[More Information Needed]
#### Training Hyperparameters
- **Training regime:** [More Information Needed] <!--fp32, fp16 mixed precision, bf16 mixed precision, bf16 non-mixed precision, fp16 non-mixed precision, fp8 mixed precision -->
#### Speeds, Sizes, Times [optional]
<!-- This section provides information about throughput, start/end time, checkpoint size if relevant, etc. -->
[More Information Needed]
## Evaluation
<!-- This section describes the evaluation protocols and provides the results. -->
### Testing Data, Factors & Metrics
#### Testing Data
<!-- This should link to a Data Card if possible. -->
[More Information Needed]
#### Factors
<!-- These are the things the evaluation is disaggregating by, e.g., subpopulations or domains. -->
[More Information Needed]
#### Metrics
<!-- These are the evaluation metrics being used, ideally with a description of why. -->
[More Information Needed]
### Results
[More Information Needed]
#### Summary
## Model Examination [optional]
<!-- Relevant interpretability work for the model goes here -->
[More Information Needed]
## Environmental Impact
<!-- Total emissions (in grams of CO2eq) and additional considerations, such as electricity usage, go here. Edit the suggested text below accordingly -->
Carbon emissions can be estimated using the [Machine Learning Impact calculator](https://mlco2.github.io/impact#compute) presented in [Lacoste et al. (2019)](https://arxiv.org/abs/1910.09700).
- **Hardware Type:** [More Information Needed]
- **Hours used:** [More Information Needed]
- **Cloud Provider:** [More Information Needed]
- **Compute Region:** [More Information Needed]
- **Carbon Emitted:** [More Information Needed]
## Technical Specifications [optional]
### Model Architecture and Objective
[More Information Needed]
### Compute Infrastructure
[More Information Needed]
#### Hardware
[More Information Needed]
#### Software
[More Information Needed]
## Citation [optional]
<!-- If there is a paper or blog post introducing the model, the APA and Bibtex information for that should go in this section. -->
**BibTeX:**
[More Information Needed]
**APA:**
[More Information Needed]
## Glossary [optional]
<!-- If relevant, include terms and calculations in this section that can help readers understand the model or model card. -->
[More Information Needed]
## More Information [optional]
[More Information Needed]
## Model Card Authors [optional]
[More Information Needed]
## Model Card Contact
[More Information Needed]
## Training procedure
The following `bitsandbytes` quantization config was used during training:
- load_in_8bit: False
- load_in_4bit: True
- llm_int8_threshold: 6.0
- llm_int8_skip_modules: None
- llm_int8_enable_fp32_cpu_offload: False
- llm_int8_has_fp16_weight: False
- bnb_4bit_quant_type: nf4
- bnb_4bit_use_double_quant: True
- bnb_4bit_compute_dtype: bfloat16
### Framework versions
- PEFT 0.7.0.dev0
## Training procedure
The following `bitsandbytes` quantization config was used during training:
- load_in_8bit: False
- load_in_4bit: True
- llm_int8_threshold: 6.0
- llm_int8_skip_modules: None
- llm_int8_enable_fp32_cpu_offload: False
- llm_int8_has_fp16_weight: False
- bnb_4bit_quant_type: nf4
- bnb_4bit_use_double_quant: True
- bnb_4bit_compute_dtype: bfloat16
### Framework versions
- PEFT 0.7.0.dev0
|
behzadnet/Llama-2-7b-chat-hf-sharded-bf16-fine-tuned_humanMix_Seed112 | behzadnet | "2024-01-02T11:30:08Z" | 0 | 0 | peft | [
"peft",
"arxiv:1910.09700",
"base_model:Trelis/Llama-2-7b-chat-hf-sharded-bf16",
"base_model:adapter:Trelis/Llama-2-7b-chat-hf-sharded-bf16",
"region:us"
] | null | "2024-01-02T11:30:05Z" | ---
library_name: peft
base_model: Trelis/Llama-2-7b-chat-hf-sharded-bf16
---
# Model Card for Model ID
<!-- Provide a quick summary of what the model is/does. -->
## Model Details
### Model Description
<!-- Provide a longer summary of what this model is. -->
- **Developed by:** [More Information Needed]
- **Shared by [optional]:** [More Information Needed]
- **Model type:** [More Information Needed]
- **Language(s) (NLP):** [More Information Needed]
- **License:** [More Information Needed]
- **Finetuned from model [optional]:** [More Information Needed]
### Model Sources [optional]
<!-- Provide the basic links for the model. -->
- **Repository:** [More Information Needed]
- **Paper [optional]:** [More Information Needed]
- **Demo [optional]:** [More Information Needed]
## Uses
<!-- Address questions around how the model is intended to be used, including the foreseeable users of the model and those affected by the model. -->
### Direct Use
<!-- This section is for the model use without fine-tuning or plugging into a larger ecosystem/app. -->
[More Information Needed]
### Downstream Use [optional]
<!-- This section is for the model use when fine-tuned for a task, or when plugged into a larger ecosystem/app -->
[More Information Needed]
### Out-of-Scope Use
<!-- This section addresses misuse, malicious use, and uses that the model will not work well for. -->
[More Information Needed]
## Bias, Risks, and Limitations
<!-- This section is meant to convey both technical and sociotechnical limitations. -->
[More Information Needed]
### Recommendations
<!-- This section is meant to convey recommendations with respect to the bias, risk, and technical limitations. -->
Users (both direct and downstream) should be made aware of the risks, biases and limitations of the model. More information needed for further recommendations.
## How to Get Started with the Model
Use the code below to get started with the model.
[More Information Needed]
## Training Details
### Training Data
<!-- This should link to a Data Card, perhaps with a short stub of information on what the training data is all about as well as documentation related to data pre-processing or additional filtering. -->
[More Information Needed]
### Training Procedure
<!-- This relates heavily to the Technical Specifications. Content here should link to that section when it is relevant to the training procedure. -->
#### Preprocessing [optional]
[More Information Needed]
#### Training Hyperparameters
- **Training regime:** [More Information Needed] <!--fp32, fp16 mixed precision, bf16 mixed precision, bf16 non-mixed precision, fp16 non-mixed precision, fp8 mixed precision -->
#### Speeds, Sizes, Times [optional]
<!-- This section provides information about throughput, start/end time, checkpoint size if relevant, etc. -->
[More Information Needed]
## Evaluation
<!-- This section describes the evaluation protocols and provides the results. -->
### Testing Data, Factors & Metrics
#### Testing Data
<!-- This should link to a Data Card if possible. -->
[More Information Needed]
#### Factors
<!-- These are the things the evaluation is disaggregating by, e.g., subpopulations or domains. -->
[More Information Needed]
#### Metrics
<!-- These are the evaluation metrics being used, ideally with a description of why. -->
[More Information Needed]
### Results
[More Information Needed]
#### Summary
## Model Examination [optional]
<!-- Relevant interpretability work for the model goes here -->
[More Information Needed]
## Environmental Impact
<!-- Total emissions (in grams of CO2eq) and additional considerations, such as electricity usage, go here. Edit the suggested text below accordingly -->
Carbon emissions can be estimated using the [Machine Learning Impact calculator](https://mlco2.github.io/impact#compute) presented in [Lacoste et al. (2019)](https://arxiv.org/abs/1910.09700).
- **Hardware Type:** [More Information Needed]
- **Hours used:** [More Information Needed]
- **Cloud Provider:** [More Information Needed]
- **Compute Region:** [More Information Needed]
- **Carbon Emitted:** [More Information Needed]
## Technical Specifications [optional]
### Model Architecture and Objective
[More Information Needed]
### Compute Infrastructure
[More Information Needed]
#### Hardware
[More Information Needed]
#### Software
[More Information Needed]
## Citation [optional]
<!-- If there is a paper or blog post introducing the model, the APA and Bibtex information for that should go in this section. -->
**BibTeX:**
[More Information Needed]
**APA:**
[More Information Needed]
## Glossary [optional]
<!-- If relevant, include terms and calculations in this section that can help readers understand the model or model card. -->
[More Information Needed]
## More Information [optional]
[More Information Needed]
## Model Card Authors [optional]
[More Information Needed]
## Model Card Contact
[More Information Needed]
## Training procedure
The following `bitsandbytes` quantization config was used during training:
- load_in_8bit: False
- load_in_4bit: True
- llm_int8_threshold: 6.0
- llm_int8_skip_modules: None
- llm_int8_enable_fp32_cpu_offload: False
- llm_int8_has_fp16_weight: False
- bnb_4bit_quant_type: nf4
- bnb_4bit_use_double_quant: True
- bnb_4bit_compute_dtype: bfloat16
### Framework versions
- PEFT 0.7.0.dev0
|
anshu1357/qa | anshu1357 | "2024-01-02T11:39:37Z" | 0 | 0 | null | [
"region:us"
] | null | "2024-01-02T11:31:43Z" | language:
- en
license: llama2
tags:
- facebook
- meta
- pytorch
- llama
- llama-2
model_name: Llama 2 7B Chat
arxiv: 2307.09288
base_model: meta-llama/Llama-2-7b-chat-hf
inference: false
model_creator: Meta Llama 2
model_type: llama
pipeline_tag: text-generation
prompt_template: >
[INST] <<SYS>>
You are a helpful, respectful and honest assistant. Always answer as helpfully
as possible, while being safe. Your answers should not include any harmful,
unethical, racist, sexist, toxic, dangerous, or illegal content. Please ensure
that your responses are socially unbiased and positive in nature. If a
question does not make any sense, or is not factually coherent, explain why
instead of answering something not correct. If you don't know the answer to a
question, please don't share false information.
<</SYS>>
{prompt}[/INST]
quantized_by: Anshu
|
PikaMiju/Reinforce-Pixelcopter-PLE-v0 | PikaMiju | "2024-01-02T11:36:57Z" | 0 | 0 | null | [
"Pixelcopter-PLE-v0",
"reinforce",
"reinforcement-learning",
"custom-implementation",
"deep-rl-class",
"model-index",
"region:us"
] | reinforcement-learning | "2024-01-02T11:36:54Z" | ---
tags:
- Pixelcopter-PLE-v0
- reinforce
- reinforcement-learning
- custom-implementation
- deep-rl-class
model-index:
- name: Reinforce-Pixelcopter-PLE-v0
results:
- task:
type: reinforcement-learning
name: reinforcement-learning
dataset:
name: Pixelcopter-PLE-v0
type: Pixelcopter-PLE-v0
metrics:
- type: mean_reward
value: 18.30 +/- 14.56
name: mean_reward
verified: false
---
# **Reinforce** Agent playing **Pixelcopter-PLE-v0**
This is a trained model of a **Reinforce** agent playing **Pixelcopter-PLE-v0** .
To learn to use this model and train yours check Unit 4 of the Deep Reinforcement Learning Course: https://huggingface.co/deep-rl-course/unit4/introduction
|
derek-thomas/speecht5_tts-finetuned_voxpopuli_hr | derek-thomas | "2024-01-02T11:37:35Z" | 0 | 0 | null | [
"region:us"
] | null | "2024-01-02T11:37:35Z" | Entry not found |
Rafaelfr87/dqn-SpaceInvadersNoFrameskip-v4 | Rafaelfr87 | "2024-01-02T11:40:52Z" | 0 | 0 | stable-baselines3 | [
"stable-baselines3",
"SpaceInvadersNoFrameskip-v4",
"deep-reinforcement-learning",
"reinforcement-learning",
"model-index",
"region:us"
] | reinforcement-learning | "2024-01-02T11:40:19Z" | ---
library_name: stable-baselines3
tags:
- SpaceInvadersNoFrameskip-v4
- deep-reinforcement-learning
- reinforcement-learning
- stable-baselines3
model-index:
- name: DQN
results:
- task:
type: reinforcement-learning
name: reinforcement-learning
dataset:
name: SpaceInvadersNoFrameskip-v4
type: SpaceInvadersNoFrameskip-v4
metrics:
- type: mean_reward
value: 581.50 +/- 152.10
name: mean_reward
verified: false
---
# **DQN** Agent playing **SpaceInvadersNoFrameskip-v4**
This is a trained model of a **DQN** agent playing **SpaceInvadersNoFrameskip-v4**
using the [stable-baselines3 library](https://github.com/DLR-RM/stable-baselines3)
and the [RL Zoo](https://github.com/DLR-RM/rl-baselines3-zoo).
The RL Zoo is a training framework for Stable Baselines3
reinforcement learning agents,
with hyperparameter optimization and pre-trained agents included.
## Usage (with SB3 RL Zoo)
RL Zoo: https://github.com/DLR-RM/rl-baselines3-zoo<br/>
SB3: https://github.com/DLR-RM/stable-baselines3<br/>
SB3 Contrib: https://github.com/Stable-Baselines-Team/stable-baselines3-contrib
Install the RL Zoo (with SB3 and SB3-Contrib):
```bash
pip install rl_zoo3
```
```
# Download model and save it into the logs/ folder
python -m rl_zoo3.load_from_hub --algo dqn --env SpaceInvadersNoFrameskip-v4 -orga Rafaelfr87 -f logs/
python -m rl_zoo3.enjoy --algo dqn --env SpaceInvadersNoFrameskip-v4 -f logs/
```
If you installed the RL Zoo3 via pip (`pip install rl_zoo3`), from anywhere you can do:
```
python -m rl_zoo3.load_from_hub --algo dqn --env SpaceInvadersNoFrameskip-v4 -orga Rafaelfr87 -f logs/
python -m rl_zoo3.enjoy --algo dqn --env SpaceInvadersNoFrameskip-v4 -f logs/
```
## Training (with the RL Zoo)
```
python -m rl_zoo3.train --algo dqn --env SpaceInvadersNoFrameskip-v4 -f logs/
# Upload the model and generate video (when possible)
python -m rl_zoo3.push_to_hub --algo dqn --env SpaceInvadersNoFrameskip-v4 -f logs/ -orga Rafaelfr87
```
## Hyperparameters
```python
OrderedDict([('batch_size', 32),
('buffer_size', 100000),
('env_wrapper',
['stable_baselines3.common.atari_wrappers.AtariWrapper']),
('exploration_final_eps', 0.01),
('exploration_fraction', 0.1),
('frame_stack', 4),
('gradient_steps', 1),
('learning_rate', 0.0001),
('learning_starts', 100000),
('n_timesteps', 1000000.0),
('optimize_memory_usage', False),
('policy', 'CnnPolicy'),
('target_update_interval', 1000),
('train_freq', 4),
('normalize', False)])
```
# Environment Arguments
```python
{'render_mode': 'rgb_array'}
```
|
Mikulej/CartPole | Mikulej | "2024-01-02T11:56:50Z" | 0 | 0 | null | [
"CartPole-v1",
"reinforce",
"reinforcement-learning",
"custom-implementation",
"deep-rl-class",
"model-index",
"region:us"
] | reinforcement-learning | "2024-01-02T11:56:41Z" | ---
tags:
- CartPole-v1
- reinforce
- reinforcement-learning
- custom-implementation
- deep-rl-class
model-index:
- name: CartPole
results:
- task:
type: reinforcement-learning
name: reinforcement-learning
dataset:
name: CartPole-v1
type: CartPole-v1
metrics:
- type: mean_reward
value: 500.00 +/- 0.00
name: mean_reward
verified: false
---
# **Reinforce** Agent playing **CartPole-v1**
This is a trained model of a **Reinforce** agent playing **CartPole-v1** .
To learn to use this model and train yours check Unit 4 of the Deep Reinforcement Learning Course: https://huggingface.co/deep-rl-course/unit4/introduction
|
Zangs3011/gpt2_137m_DolphinCoder | Zangs3011 | "2024-01-02T11:58:27Z" | 0 | 0 | peft | [
"peft",
"region:us"
] | null | "2024-01-02T11:58:23Z" | ---
library_name: peft
---
## Training procedure
### Framework versions
- PEFT 0.5.0
|
tranhuonglan/diffuse-pose-sample | tranhuonglan | "2024-01-02T13:48:45Z" | 0 | 0 | null | [
"tensorboard",
"region:us"
] | null | "2024-01-02T12:03:00Z" | Entry not found |
Zienab/wav2vec | Zienab | "2024-01-07T07:31:19Z" | 0 | 0 | adapter-transformers | [
"adapter-transformers",
"code",
"ar",
"dataset:mozilla-foundation/common_voice_16_0",
"license:apache-2.0",
"region:us"
] | null | "2024-01-02T12:06:45Z" | ---
license: apache-2.0
datasets:
- mozilla-foundation/common_voice_16_0
language:
- ar
metrics:
- accuracy
library_name: adapter-transformers
tags:
- code
--- |
Ayush3690/distilbert-base-uncased-finetuned-squad | Ayush3690 | "2024-01-02T12:18:56Z" | 0 | 0 | null | [
"region:us"
] | null | "2024-01-02T12:18:56Z" | Entry not found |
sdfmndshgf/civi2 | sdfmndshgf | "2024-01-02T12:21:28Z" | 0 | 0 | null | [
"license:openrail",
"region:us"
] | null | "2024-01-02T12:21:02Z" | ---
license: openrail
---
|
ParazaxComplex/EasyFlexKenya | ParazaxComplex | "2024-01-02T12:27:35Z" | 0 | 0 | null | [
"region:us"
] | null | "2024-01-02T12:26:15Z" | <a href="https://www.nutritionsee.com/EasFleKen">Easy Flex Nunua sasa!! Bofya kiungo hapa chini kwa maelezo zaidi na upate punguzo la 50% sasa !! Harakisha !!</a>
Tovuti rasmi: <a href="https://www.nutritionsee.com/EasFleKen">www.EasyFlex.com</a>
<p><a href="https://www.nutritionsee.com/EasFleKen"> <img src="https://blogger.googleusercontent.com/img/b/R29vZ2xl/AVvXsEilOZiCnMM2fsRFHNaZuNipsNWml3UUiLhnCYQIsmlxBmXgtCO0PNbDorKpmeHdbHMzlgBeGg7ubDOS9EuKeXi0xums0O-o92Lh0-G9UWEYSUut9kFmBTDZ-Jssczk3bRNSFnlOqU1jr_n3NsESd4K-ZOnQu3LNyHBfKlawCDgyAkxaY2ncBX-al_qXROM/w659-h447/Easy%20Flex%20kenya.png" alt="enter image description here"> </a></p>
Easy Flex ni viambato vya asili vinavyofanya kazi ya kupunguza maumivu ya viungo huku pia ikiwasaidia watumiaji kurejesha gegedu iliyoharibika katika miili yao. Soma zaidi. Easy Flex Kenya
➢Jina la Bidhaa - Easy Flex
➢Kategoria -Afya ya Pamoja
➢Manufaa Muhimu — Kukupa faraja ya pamoja zaidi na ufanye maisha yako kuwa ya furaha na amilifu zaidi.
➢ Muundo - Mchanganyiko Asilia wa Kikaboni
➢ Madhara—NA
➢Ukadiriaji wa Mwisho: - 4.8
➢ Upatikanaji — Mtandaoni
➢Ofa na Punguzo; HIFADHI LEO! NUNUA SASA ILI ununue OFA MAALUM!!!
Easy Flex ni nini?
Easy Flex ni fomula mpya ya kimapinduzi ambayo husaidia kushughulikia masuala ya msingi ambayo husababisha maumivu ya muda mrefu ya viungo. Vipengele Easy Flex hupunguza ugumu wa viungo, maumivu katika mabega na magoti, na uvimbe kwenye viungo. Watu walitumia formula hii kuondoa sumu na maumivu katika mwili. Kutuliza maumivu na kuongezeka kwa utoaji wa virutubisho ni faida mbili tu kati ya nyingi za kuchukua bidhaa hii.
https://www.nutritionsee.com/EasFleKen
https://sites.google.com/view/easy-flex-kenya/home
https://healthtoned.blogspot.com/2024/01/vidonge-easy-flex-vya-kutuliza-maumivu.html
https://medium.com/@healthytalk24x7/easy-flex-kenya-b3c8c6281173
https://medium.com/@healthytalk24x7/vidonge-easy-flex-vya-kutuliza-maumivu-nunua-katika-kenya-soma-maoni-2024-817ed2a634c3
https://www.weddingwire.com/website/easy-flex-and-kenya
https://groups.google.com/g/snshine/c/KAYQ1E0nqhM
https://infogram.com/easy-flex-kenya-1h0n25y5v7p3z6p?live
|
Dieickson/Tecnologia | Dieickson | "2024-01-09T17:00:47Z" | 0 | 0 | null | [
"region:us"
] | null | "2024-01-02T12:28:48Z" | tecnologia
robôs
bunker
|
Anudip2003/my-pet-dog-asb-updated | Anudip2003 | "2024-01-02T12:42:44Z" | 0 | 0 | diffusers | [
"diffusers",
"safetensors",
"NxtWave-GenAI-Webinar",
"text-to-image",
"stable-diffusion",
"license:creativeml-openrail-m",
"autotrain_compatible",
"endpoints_compatible",
"diffusers:StableDiffusionPipeline",
"region:us"
] | text-to-image | "2024-01-02T12:30:59Z" | ---
license: creativeml-openrail-m
tags:
- NxtWave-GenAI-Webinar
- text-to-image
- stable-diffusion
---
### My-Pet-Dog-asb-updated Dreambooth model trained by Anudip2003 following the "Build your own Gen AI model" session by NxtWave.
Project Submission Code: 272008
Sample pictures of this concept:



|
Mikulej/Pixelcopter-PLE-v0 | Mikulej | "2024-01-02T12:33:53Z" | 0 | 0 | null | [
"Pixelcopter-PLE-v0",
"reinforce",
"reinforcement-learning",
"custom-implementation",
"deep-rl-class",
"model-index",
"region:us"
] | reinforcement-learning | "2024-01-02T12:33:16Z" | ---
tags:
- Pixelcopter-PLE-v0
- reinforce
- reinforcement-learning
- custom-implementation
- deep-rl-class
model-index:
- name: Pixelcopter-PLE-v0
results:
- task:
type: reinforcement-learning
name: reinforcement-learning
dataset:
name: Pixelcopter-PLE-v0
type: Pixelcopter-PLE-v0
metrics:
- type: mean_reward
value: 13.70 +/- 8.54
name: mean_reward
verified: false
---
# **Reinforce** Agent playing **Pixelcopter-PLE-v0**
This is a trained model of a **Reinforce** agent playing **Pixelcopter-PLE-v0** .
To learn to use this model and train yours check Unit 4 of the Deep Reinforcement Learning Course: https://huggingface.co/deep-rl-course/unit4/introduction
|
shidowake/test-240102-mistral-lora-adaptor | shidowake | "2024-01-02T12:49:20Z" | 0 | 0 | null | [
"tensorboard",
"safetensors",
"region:us"
] | null | "2024-01-02T12:33:33Z" | Entry not found |
VoidZeroe/llama6.0-model | VoidZeroe | "2024-01-02T12:43:54Z" | 0 | 0 | peft | [
"peft",
"region:us"
] | null | "2024-01-02T12:43:12Z" | ---
library_name: peft
---
## Training procedure
The following `bitsandbytes` quantization config was used during training:
- load_in_8bit: False
- load_in_4bit: True
- llm_int8_threshold: 6.0
- llm_int8_skip_modules: None
- llm_int8_enable_fp32_cpu_offload: False
- llm_int8_has_fp16_weight: False
- bnb_4bit_quant_type: nf4
- bnb_4bit_use_double_quant: False
- bnb_4bit_compute_dtype: float16
### Framework versions
- PEFT 0.4.0
|
ctrltokyo/mistral-finetune-gaban-samsay | ctrltokyo | "2024-01-02T13:24:32Z" | 0 | 0 | peft | [
"peft",
"safetensors",
"arxiv:1910.09700",
"base_model:mistralai/Mistral-7B-v0.1",
"base_model:adapter:mistralai/Mistral-7B-v0.1",
"region:us"
] | null | "2024-01-02T12:47:14Z" | ---
library_name: peft
base_model: mistralai/Mistral-7B-v0.1
---
# Model Card for Model ID
<!-- Provide a quick summary of what the model is/does. -->
## Model Details
### Model Description
<!-- Provide a longer summary of what this model is. -->
- **Developed by:** [More Information Needed]
- **Funded by [optional]:** [More Information Needed]
- **Shared by [optional]:** [More Information Needed]
- **Model type:** [More Information Needed]
- **Language(s) (NLP):** [More Information Needed]
- **License:** [More Information Needed]
- **Finetuned from model [optional]:** [More Information Needed]
### Model Sources [optional]
<!-- Provide the basic links for the model. -->
- **Repository:** [More Information Needed]
- **Paper [optional]:** [More Information Needed]
- **Demo [optional]:** [More Information Needed]
## Uses
<!-- Address questions around how the model is intended to be used, including the foreseeable users of the model and those affected by the model. -->
### Direct Use
```
import torch
from transformers import AutoTokenizer, AutoModelForCausalLM, BitsAndBytesConfig
base_model_id = "mistralai/Mistral-7B-v0.1"
bnb_config = BitsAndBytesConfig(
load_in_4bit=True,
bnb_4bit_use_double_quant=True,
bnb_4bit_quant_type="nf4",
bnb_4bit_compute_dtype=torch.bfloat16
)
base_model = AutoModelForCausalLM.from_pretrained(
base_model_id, # Mistral, same as before
quantization_config=bnb_config, # Same quantization config as before
device_map="auto",
trust_remote_code=True,
use_auth_token=True
)
eval_tokenizer = AutoTokenizer.from_pretrained(base_model_id, add_bos_token=True, trust_remote_code=True)
from peft import PeftModel, PeftConfig
from transformers import AutoModelForCausalLM
ft_model = PeftModel.from_pretrained(base_model, "ctrltokyo/mistral-finetune-gaban-samsay")
# Inference
eval_prompt = """The following is a script for an episode of Kitchen Nightmares:
[Gordon] Goddamn it, this restaurant is in the toilet!
"""
model_input = eval_tokenizer(eval_prompt, return_tensors="pt").to("cuda")
ft_model.eval()
with torch.no_grad():
print(eval_tokenizer.decode(ft_model.generate(**model_input, max_new_tokens=150, repetition_penalty=1.5)[0], skip_special_tokens=True))
```
### Downstream Use [optional]
<!-- This section is for the model use when fine-tuned for a task, or when plugged into a larger ecosystem/app -->
[More Information Needed]
### Out-of-Scope Use
<!-- This section addresses misuse, malicious use, and uses that the model will not work well for. -->
[More Information Needed]
## Bias, Risks, and Limitations
<!-- This section is meant to convey both technical and sociotechnical limitations. -->
[More Information Needed]
### Recommendations
<!-- This section is meant to convey recommendations with respect to the bias, risk, and technical limitations. -->
Users (both direct and downstream) should be made aware of the risks, biases and limitations of the model. More information needed for further recommendations.
## How to Get Started with the Model
Use the code below to get started with the model.
[More Information Needed]
## Training Details
### Training Data
<!-- This should link to a Dataset Card, perhaps with a short stub of information on what the training data is all about as well as documentation related to data pre-processing or additional filtering. -->
[More Information Needed]
### Training Procedure
<!-- This relates heavily to the Technical Specifications. Content here should link to that section when it is relevant to the training procedure. -->
#### Preprocessing [optional]
[More Information Needed]
#### Training Hyperparameters
- **Training regime:** [More Information Needed] <!--fp32, fp16 mixed precision, bf16 mixed precision, bf16 non-mixed precision, fp16 non-mixed precision, fp8 mixed precision -->
#### Speeds, Sizes, Times [optional]
<!-- This section provides information about throughput, start/end time, checkpoint size if relevant, etc. -->
[More Information Needed]
## Evaluation
<!-- This section describes the evaluation protocols and provides the results. -->
### Testing Data, Factors & Metrics
#### Testing Data
<!-- This should link to a Dataset Card if possible. -->
[More Information Needed]
#### Factors
<!-- These are the things the evaluation is disaggregating by, e.g., subpopulations or domains. -->
[More Information Needed]
#### Metrics
<!-- These are the evaluation metrics being used, ideally with a description of why. -->
[More Information Needed]
### Results
[More Information Needed]
#### Summary
## Model Examination [optional]
<!-- Relevant interpretability work for the model goes here -->
[More Information Needed]
## Environmental Impact
<!-- Total emissions (in grams of CO2eq) and additional considerations, such as electricity usage, go here. Edit the suggested text below accordingly -->
Carbon emissions can be estimated using the [Machine Learning Impact calculator](https://mlco2.github.io/impact#compute) presented in [Lacoste et al. (2019)](https://arxiv.org/abs/1910.09700).
- **Hardware Type:** [More Information Needed]
- **Hours used:** [More Information Needed]
- **Cloud Provider:** [More Information Needed]
- **Compute Region:** [More Information Needed]
- **Carbon Emitted:** [More Information Needed]
## Technical Specifications [optional]
### Model Architecture and Objective
[More Information Needed]
### Compute Infrastructure
[More Information Needed]
#### Hardware
[More Information Needed]
#### Software
[More Information Needed]
## Citation [optional]
<!-- If there is a paper or blog post introducing the model, the APA and Bibtex information for that should go in this section. -->
**BibTeX:**
[More Information Needed]
**APA:**
[More Information Needed]
## Glossary [optional]
<!-- If relevant, include terms and calculations in this section that can help readers understand the model or model card. -->
[More Information Needed]
## More Information [optional]
[More Information Needed]
## Model Card Authors [optional]
[More Information Needed]
## Model Card Contact
[More Information Needed]
### Framework versions
- PEFT 0.7.2.dev0 |
Mrljq/Clip_mine | Mrljq | "2024-01-02T13:57:54Z" | 0 | 0 | null | [
"region:us"
] | null | "2024-01-02T12:48:19Z" | Entry not found |
Vissy/RemoteBFBSpanish | Vissy | "2024-01-02T13:09:06Z" | 0 | 0 | null | [
"license:openrail",
"region:us"
] | null | "2024-01-02T13:00:54Z" | ---
license: openrail
---
|
bartowski/OpenCAI-7B-exl2 | bartowski | "2024-01-02T14:42:39Z" | 0 | 0 | null | [
"art",
"not-for-all-audiences",
"text-generation",
"en",
"dataset:Norquinal/OpenCAI",
"license:cc-by-nc-4.0",
"region:us"
] | text-generation | "2024-01-02T13:03:18Z" | ---
license: cc-by-nc-4.0
datasets: Norquinal/OpenCAI
language: en
tags:
- art
- not-for-all-audiences
quantized_by: bartowski
pipeline_tag: text-generation
---
## Exllama v2 Quantizations of OpenCAI-7B
Using <a href="https://github.com/turboderp/exllamav2/releases/tag/v0.0.11">turboderp's ExLlamaV2 v0.0.11</a> for quantization.
Each branch contains an individual bits per weight, with the main one containing only the meaurement.json for further conversions.
Conversion was done using the default calibration dataset.
Default arguments used except when the bits per weight is above 6.0, at that point the lm_head layer is quantized at 8 bits per weight instead of the default 6.
Original model: https://huggingface.co/Norquinal/OpenCAI-7B
<a href="https://huggingface.co/bartowski/OpenCAI-7B-exl2/tree/3_5">3.5 bits per weight</a>
<a href="https://huggingface.co/bartowski/OpenCAI-7B-exl2/tree/4_0">4.0 bits per weight</a>
<a href="https://huggingface.co/bartowski/OpenCAI-7B-exl2/tree/5_0">5.0 bits per weight</a>
<a href="https://huggingface.co/bartowski/OpenCAI-7B-exl2/tree/6_5">6.5 bits per weight</a>
<a href="https://huggingface.co/bartowski/OpenCAI-7B-exl2/tree/8_0">8.0 bits per weight</a>
## Download instructions
With git:
```shell
git clone --single-branch --branch 4_0 https://huggingface.co/bartowski/OpenCAI-7B-exl2
```
With huggingface hub (credit to TheBloke for instructions):
```shell
pip3 install huggingface-hub
```
To download the `main` (only useful if you only care about measurement.json) branch to a folder called `OpenCAI-7B-exl2`:
```shell
mkdir OpenCAI-7B-exl2
huggingface-cli download bartowski/OpenCAI-7B-exl2 --local-dir OpenCAI-7B-exl2 --local-dir-use-symlinks False
```
To download from a different branch, add the `--revision` parameter:
```shell
mkdir OpenCAI-7B-exl2
huggingface-cli download bartowski/OpenCAI-7B-exl2 --revision 4_0 --local-dir OpenCAI-7B-exl2 --local-dir-use-symlinks False
```
|
elliotthwangmsa/KimLan_mistral-0.5b-40k | elliotthwangmsa | "2024-01-03T03:22:10Z" | 0 | 0 | null | [
"safetensors",
"region:us"
] | null | "2024-01-02T13:06:02Z" | Entry not found |
DataVare/OLM-TO-PST-CONVERTER | DataVare | "2024-01-02T13:07:54Z" | 0 | 0 | null | [
"region:us"
] | null | "2024-01-02T13:07:32Z" | The greatest tool for converting Mac OLM files to PST for Windows Outlook is our DataVare OLM to PST Converter. It has several advanced features that increase its technicality or user appeal. Emails, contacts, calendars, tasks, notes, diaries, and other entire data must all be exported from Mac OLM files to PST. Throughout the conversion process, it keeps the data structure and email attributes intact. Any user, technical or not, can use this application without any prior expertise because of its very simple and intuitive user interface. It supports all versions of Windows and MS OS.
For the convenience of our consumers, we also provide a free demo pack where they may convert 25 OLM files to PST files and see how they function.
Visit Here:- https://www.datavare.com/software/olm-to-pst-converter.html |
bbillapati/wav2vec2-base-finetuned-gtzan | bbillapati | "2024-01-03T08:03:28Z" | 0 | 0 | transformers | [
"transformers",
"tensorboard",
"safetensors",
"wav2vec2",
"audio-classification",
"endpoints_compatible",
"region:us"
] | audio-classification | "2024-01-02T13:08:17Z" | Entry not found |
LoicSteve/q-FrozenLake-v1-4x4-noSlippery | LoicSteve | "2024-01-02T13:11:01Z" | 0 | 0 | null | [
"FrozenLake-v1-4x4-no_slippery",
"q-learning",
"reinforcement-learning",
"custom-implementation",
"model-index",
"region:us"
] | reinforcement-learning | "2024-01-02T13:10:59Z" | ---
tags:
- FrozenLake-v1-4x4-no_slippery
- q-learning
- reinforcement-learning
- custom-implementation
model-index:
- name: q-FrozenLake-v1-4x4-noSlippery
results:
- task:
type: reinforcement-learning
name: reinforcement-learning
dataset:
name: FrozenLake-v1-4x4-no_slippery
type: FrozenLake-v1-4x4-no_slippery
metrics:
- type: mean_reward
value: 1.00 +/- 0.00
name: mean_reward
verified: false
---
# **Q-Learning** Agent playing1 **FrozenLake-v1**
This is a trained model of a **Q-Learning** agent playing **FrozenLake-v1** .
## Usage
```python
model = load_from_hub(repo_id="LoicSteve/q-FrozenLake-v1-4x4-noSlippery", filename="q-learning.pkl")
# Don't forget to check if you need to add additional attributes (is_slippery=False etc)
env = gym.make(model["env_id"])
```
|
LoicSteve/Taxi-v3 | LoicSteve | "2024-01-02T13:12:18Z" | 0 | 0 | null | [
"region:us"
] | null | "2024-01-02T13:12:18Z" | Entry not found |
LoicSteve/q-Taxi-v3 | LoicSteve | "2024-01-02T15:24:49Z" | 0 | 0 | null | [
"Taxi-v3",
"q-learning",
"reinforcement-learning",
"custom-implementation",
"model-index",
"region:us"
] | reinforcement-learning | "2024-01-02T13:16:13Z" | ---
tags:
- Taxi-v3
- q-learning
- reinforcement-learning
- custom-implementation
model-index:
- name: q-Taxi-v3
results:
- task:
type: reinforcement-learning
name: reinforcement-learning
dataset:
name: Taxi-v3
type: Taxi-v3
metrics:
- type: mean_reward
value: 7.56 +/- 2.71
name: mean_reward
verified: false
---
# **Q-Learning** Agent playing1 **Taxi-v3**
This is a trained model of a **Q-Learning** agent playing **Taxi-v3** .
## Usage
```python
model = load_from_hub(repo_id="LoicSteve/q-Taxi-v3", filename="q-learning.pkl")
# Don't forget to check if you need to add additional attributes (is_slippery=False etc)
env = gym.make(model["env_id"])
```
|
myshell-ai/OpenVoice | myshell-ai | "2024-04-24T13:59:44Z" | 0 | 388 | null | [
"audio",
"text-to-speech",
"instant-voice-cloning",
"en",
"zh",
"license:mit",
"region:us"
] | text-to-speech | "2024-01-02T13:16:15Z" | ---
license: mit
tags:
- audio
- text-to-speech
- instant-voice-cloning
language:
- en
- zh
inference: false
---
# OpenVoice
OpenVoice, a versatile instant voice cloning approach that requires only a short audio clip from the reference speaker to replicate their voice and generate speech in multiple languages. OpenVoice enables granular control over voice styles, including emotion, accent, rhythm, pauses, and intonation, in addition to replicating the tone color of the reference speaker. OpenVoice also achieves zero-shot cross-lingual voice cloning for languages not included in the massive-speaker training set.
<video controls autoplay src="https://cdn-uploads.huggingface.co/production/uploads/641de0213239b631552713e4/uCHTHD9OUotgOflqDu3QK.mp4"></video>
### Features
- **Accurate Tone Color Cloning.** OpenVoice can accurately clone the reference tone color and generate speech in multiple languages and accents.
- **Flexible Voice Style Control.** OpenVoice enables granular control over voice styles, such as emotion and accent, as well as other style parameters including rhythm, pauses, and intonation.
- **Zero-shot Cross-lingual Voice Cloning.** Neither of the language of the generated speech nor the language of the reference speech needs to be presented in the massive-speaker multi-lingual training dataset.
### How to Use
Please see [usage](https://github.com/myshell-ai/OpenVoice/blob/main/docs/USAGE.md) for detailed instructions.
### Links
- [Github](https://github.com/myshell-ai/OpenVoice)
- [HFDemo](https://huggingface.co/spaces/myshell-ai/OpenVoice)
- [Discord](https://discord.gg/myshell)
|
LoicSteve/q-Taxi-v33 | LoicSteve | "2024-01-02T13:16:47Z" | 0 | 0 | null | [
"region:us"
] | null | "2024-01-02T13:16:47Z" | Entry not found |
LoicSteve/q-Taxi-v3-v3 | LoicSteve | "2024-01-02T13:17:11Z" | 0 | 0 | null | [
"region:us"
] | null | "2024-01-02T13:17:11Z" | Entry not found |
taku-yoshioka/test | taku-yoshioka | "2024-01-02T13:18:15Z" | 0 | 0 | transformers | [
"transformers",
"pytorch",
"safetensors",
"trl",
"ppo",
"reinforcement-learning",
"license:apache-2.0",
"endpoints_compatible",
"region:us"
] | reinforcement-learning | "2024-01-02T13:18:12Z" | ---
license: apache-2.0
tags:
- trl
- ppo
- transformers
- reinforcement-learning
---
# TRL Model
This is a [TRL language model](https://github.com/huggingface/trl) that has been fine-tuned with reinforcement learning to
guide the model outputs according to a value, function, or human feedback. The model can be used for text generation.
## Usage
To use this model for inference, first install the TRL library:
```bash
python -m pip install trl
```
You can then generate text as follows:
```python
from transformers import pipeline
generator = pipeline("text-generation", model="taku-yoshioka//tmp/tmp1_n5iu5l/taku-yoshioka/test")
outputs = generator("Hello, my llama is cute")
```
If you want to use the model for training or to obtain the outputs from the value head, load the model as follows:
```python
from transformers import AutoTokenizer
from trl import AutoModelForCausalLMWithValueHead
tokenizer = AutoTokenizer.from_pretrained("taku-yoshioka//tmp/tmp1_n5iu5l/taku-yoshioka/test")
model = AutoModelForCausalLMWithValueHead.from_pretrained("taku-yoshioka//tmp/tmp1_n5iu5l/taku-yoshioka/test")
inputs = tokenizer("Hello, my llama is cute", return_tensors="pt")
outputs = model(**inputs, labels=inputs["input_ids"])
```
|
louisbrulenaudet/docutron | louisbrulenaudet | "2024-01-02T13:32:54Z" | 0 | 1 | null | [
"faster_rcnn_R_50_FPN",
"legal",
"CNN",
"droit français",
"tax",
"droit fiscal",
"document",
"feature-extraction",
"license:apache-2.0",
"region:us"
] | feature-extraction | "2024-01-02T13:21:08Z" | ---
license: apache-2.0
librairy_name: Detectron2
pipeline_tag: feature-extraction
tags:
- faster_rcnn_R_50_FPN
- legal
- CNN
- droit français
- tax
- droit fiscal
- document
pretty_name: Docutron, detection and segmentation analysis for legal data extraction over documents
---
# Docutron : detection and segmentation analysis for legal data extraction over documents
Docutron is a tool designed to facilitate the extraction of relevant information from legal documents, enabling professionals to create datasets for fine-tuning language models (LLM) for specific legal domains.
Legal professionals often deal with vast amounts of text data in various formats, including legal documents, contracts, regulations, and case law. Extracting structured information from these documents is a time-consuming and error-prone task. Docutron simplifies this process by using state-of-the-art computer vision and natural language processing techniques to automate the extraction of key information from legal documents.
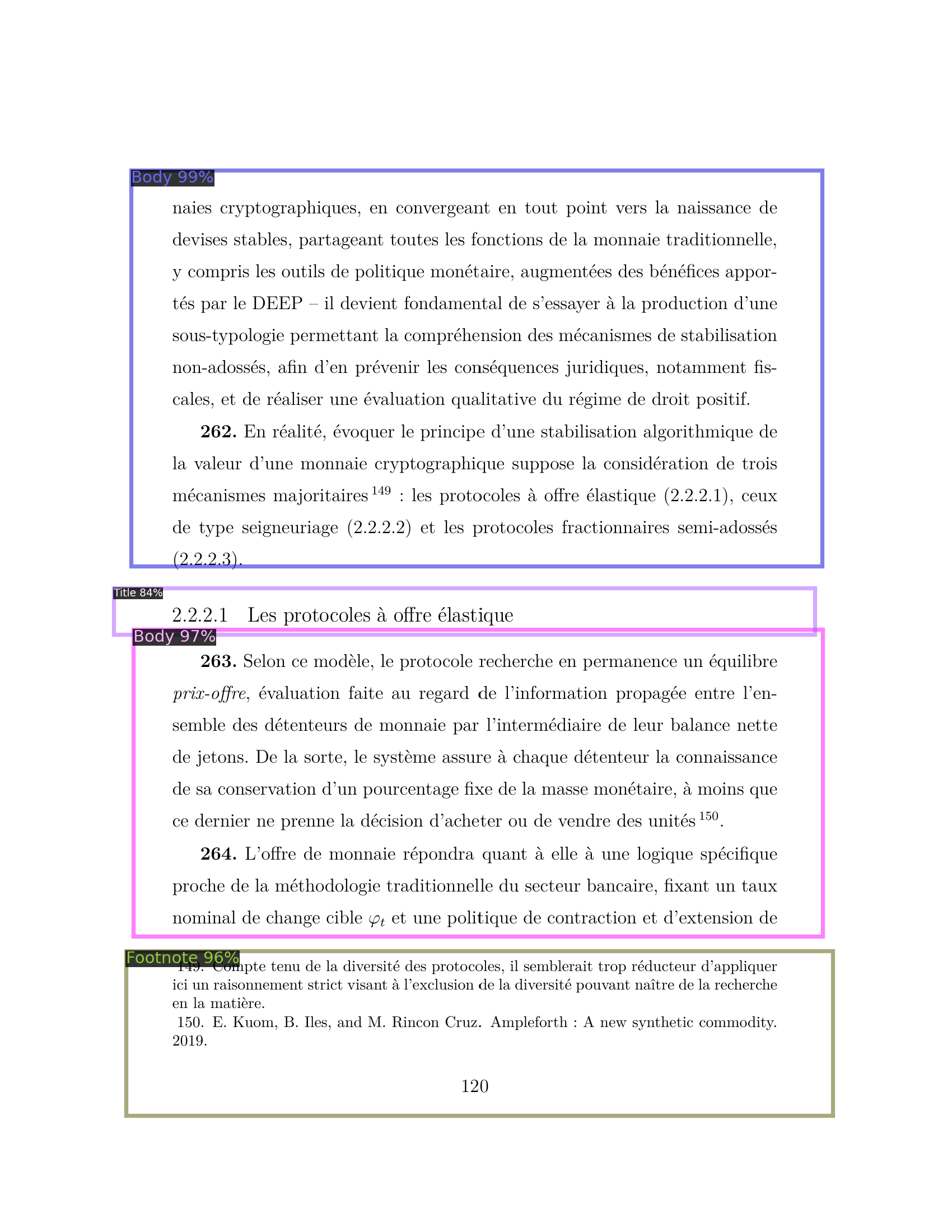
Whether you are delving into contract analysis, legal document summarization, or any other legal task that demands meticulous data extraction, Docutron stands ready to be your reliable technical companion, simplifying complex legal workflows and opening doors to new possibilities in legal research and analysis.
## Citing this project
If you use this code in your research, please use the following BibTeX entry.
```BibTeX
@misc{louisbrulenaudet2023,
author = {Louis Brulé Naudet},
title = {Docutron Toolkit: detection and segmentation analysis for legal data extraction over documents},
howpublished = {\url{https://github.com/louisbrulenaudet/docutron}},
year = {2023}
}
```
## Feedback
If you have any feedback, please reach out at [louisbrulenaudet@icloud.com](mailto:louisbrulenaudet@icloud.com). |
MEgMOONT/my_awesome_qa_model | MEgMOONT | "2024-01-02T13:22:08Z" | 0 | 0 | null | [
"region:us"
] | null | "2024-01-02T13:22:08Z" | Entry not found |
charoori/llm4movies | charoori | "2024-01-02T16:24:33Z" | 0 | 0 | null | [
"safetensors",
"region:us"
] | null | "2024-01-02T13:29:40Z" | Entry not found |
TeeA/roberta-base-pokemon | TeeA | "2024-01-02T14:09:51Z" | 0 | 0 | transformers | [
"transformers",
"safetensors",
"RobertaClassifier",
"endpoints_compatible",
"region:us"
] | null | "2024-01-02T13:36:04Z" | Entry not found |
zhangxiongwei1996/path-to-save-model_1 | zhangxiongwei1996 | "2024-01-02T13:38:17Z" | 0 | 0 | null | [
"region:us"
] | null | "2024-01-02T13:38:17Z" | Entry not found |
idontgoddamn/AsagaoHanae | idontgoddamn | "2024-01-02T13:40:07Z" | 0 | 0 | null | [
"region:us"
] | null | "2024-01-02T13:39:50Z" | Entry not found |
kollis/rl_course_vizdoom_health_gathering_supreme | kollis | "2024-01-02T13:42:08Z" | 0 | 0 | sample-factory | [
"sample-factory",
"tensorboard",
"deep-reinforcement-learning",
"reinforcement-learning",
"model-index",
"region:us"
] | reinforcement-learning | "2024-01-02T13:42:02Z" | ---
library_name: sample-factory
tags:
- deep-reinforcement-learning
- reinforcement-learning
- sample-factory
model-index:
- name: APPO
results:
- task:
type: reinforcement-learning
name: reinforcement-learning
dataset:
name: doom_health_gathering_supreme
type: doom_health_gathering_supreme
metrics:
- type: mean_reward
value: 8.50 +/- 2.09
name: mean_reward
verified: false
---
A(n) **APPO** model trained on the **doom_health_gathering_supreme** environment.
This model was trained using Sample-Factory 2.0: https://github.com/alex-petrenko/sample-factory.
Documentation for how to use Sample-Factory can be found at https://www.samplefactory.dev/
## Downloading the model
After installing Sample-Factory, download the model with:
```
python -m sample_factory.huggingface.load_from_hub -r kollis/rl_course_vizdoom_health_gathering_supreme
```
## Using the model
To run the model after download, use the `enjoy` script corresponding to this environment:
```
python -m .usr.local.lib.python3.10.dist-packages.colab_kernel_launcher --algo=APPO --env=doom_health_gathering_supreme --train_dir=./train_dir --experiment=rl_course_vizdoom_health_gathering_supreme
```
You can also upload models to the Hugging Face Hub using the same script with the `--push_to_hub` flag.
See https://www.samplefactory.dev/10-huggingface/huggingface/ for more details
## Training with this model
To continue training with this model, use the `train` script corresponding to this environment:
```
python -m .usr.local.lib.python3.10.dist-packages.colab_kernel_launcher --algo=APPO --env=doom_health_gathering_supreme --train_dir=./train_dir --experiment=rl_course_vizdoom_health_gathering_supreme --restart_behavior=resume --train_for_env_steps=10000000000
```
Note, you may have to adjust `--train_for_env_steps` to a suitably high number as the experiment will resume at the number of steps it concluded at.
|
csujeong/Mistral-7B-Finetuning-Stock | csujeong | "2024-01-02T13:50:00Z" | 0 | 0 | peft | [
"peft",
"tensorboard",
"safetensors",
"trl",
"sft",
"generated_from_trainer",
"base_model:mistralai/Mistral-7B-v0.1",
"base_model:adapter:mistralai/Mistral-7B-v0.1",
"license:apache-2.0",
"region:us"
] | null | "2024-01-02T13:43:04Z" | ---
license: apache-2.0
library_name: peft
tags:
- trl
- sft
- generated_from_trainer
base_model: mistralai/Mistral-7B-v0.1
model-index:
- name: Mistral-7B-Finetuning-Stock
results: []
---
<!-- This model card has been generated automatically according to the information the Trainer had access to. You
should probably proofread and complete it, then remove this comment. -->
# Mistral-7B-Finetuning-Stock
This model is a fine-tuned version of [mistralai/Mistral-7B-v0.1](https://huggingface.co/mistralai/Mistral-7B-v0.1) on an unknown dataset.
## Model description
More information needed
## Intended uses & limitations
More information needed
## Training and evaluation data
More information needed
## Training procedure
### Training hyperparameters
The following hyperparameters were used during training:
- learning_rate: 0.0002
- train_batch_size: 2
- eval_batch_size: 8
- seed: 42
- gradient_accumulation_steps: 2
- total_train_batch_size: 4
- optimizer: Adam with betas=(0.9,0.999) and epsilon=1e-08
- lr_scheduler_type: cosine
- lr_scheduler_warmup_ratio: 0.03
- training_steps: 60
### Training results
### Framework versions
- PEFT 0.7.2.dev0
- Transformers 4.37.0.dev0
- Pytorch 2.1.2+cu121
- Datasets 2.16.1
- Tokenizers 0.15.0 |
lucapacini67/prova | lucapacini67 | "2024-01-02T13:44:27Z" | 0 | 0 | null | [
"region:us"
] | null | "2024-01-02T13:44:27Z" | Entry not found |
aumy/q-FrozenLake-v1-4x4-noSlippery | aumy | "2024-01-02T13:50:49Z" | 0 | 0 | null | [
"FrozenLake-v1-4x4-no_slippery",
"q-learning",
"reinforcement-learning",
"custom-implementation",
"model-index",
"region:us"
] | reinforcement-learning | "2024-01-02T13:50:47Z" | ---
tags:
- FrozenLake-v1-4x4-no_slippery
- q-learning
- reinforcement-learning
- custom-implementation
model-index:
- name: q-FrozenLake-v1-4x4-noSlippery
results:
- task:
type: reinforcement-learning
name: reinforcement-learning
dataset:
name: FrozenLake-v1-4x4-no_slippery
type: FrozenLake-v1-4x4-no_slippery
metrics:
- type: mean_reward
value: 1.00 +/- 0.00
name: mean_reward
verified: false
---
# **Q-Learning** Agent playing1 **FrozenLake-v1**
This is a trained model of a **Q-Learning** agent playing **FrozenLake-v1** .
## Usage
```python
model = load_from_hub(repo_id="aumy/q-FrozenLake-v1-4x4-noSlippery", filename="q-learning.pkl")
# Don't forget to check if you need to add additional attributes (is_slippery=False etc)
env = gym.make(model["env_id"])
```
|
aumy/Taxi-v3 | aumy | "2024-01-02T13:52:02Z" | 0 | 0 | null | [
"Taxi-v3",
"q-learning",
"reinforcement-learning",
"custom-implementation",
"model-index",
"region:us"
] | reinforcement-learning | "2024-01-02T13:52:00Z" | ---
tags:
- Taxi-v3
- q-learning
- reinforcement-learning
- custom-implementation
model-index:
- name: Taxi-v3
results:
- task:
type: reinforcement-learning
name: reinforcement-learning
dataset:
name: Taxi-v3
type: Taxi-v3
metrics:
- type: mean_reward
value: 7.52 +/- 2.73
name: mean_reward
verified: false
---
# **Q-Learning** Agent playing1 **Taxi-v3**
This is a trained model of a **Q-Learning** agent playing **Taxi-v3** .
## Usage
```python
model = load_from_hub(repo_id="aumy/Taxi-v3", filename="q-learning.pkl")
# Don't forget to check if you need to add additional attributes (is_slippery=False etc)
env = gym.make(model["env_id"])
```
|
noodlelife/hanzo | noodlelife | "2024-01-02T14:01:03Z" | 0 | 0 | null | [
"region:us"
] | null | "2024-01-02T13:54:26Z" | Entry not found |
idontgoddamn/ChiakiNanami | idontgoddamn | "2024-01-02T14:14:04Z" | 0 | 0 | null | [
"region:us"
] | null | "2024-01-02T14:13:37Z" | Entry not found |
Diconic/HanJisung | Diconic | "2024-01-02T14:15:30Z" | 0 | 0 | null | [
"license:openrail",
"region:us"
] | null | "2024-01-02T14:14:06Z" | ---
license: openrail
---
|
Thananan/thai-nutrichat | Thananan | "2024-01-02T14:16:07Z" | 0 | 0 | peft | [
"peft",
"tensorboard",
"arxiv:1910.09700",
"base_model:openthaigpt/openthaigpt-1.0.0-beta-7b-chat-ckpt-hf",
"base_model:adapter:openthaigpt/openthaigpt-1.0.0-beta-7b-chat-ckpt-hf",
"region:us"
] | null | "2024-01-02T14:14:08Z" | ---
library_name: peft
base_model: openthaigpt/openthaigpt-1.0.0-beta-7b-chat-ckpt-hf
---
# Model Card for Model ID
<!-- Provide a quick summary of what the model is/does. -->
## Model Details
### Model Description
<!-- Provide a longer summary of what this model is. -->
- **Developed by:** [More Information Needed]
- **Funded by [optional]:** [More Information Needed]
- **Shared by [optional]:** [More Information Needed]
- **Model type:** [More Information Needed]
- **Language(s) (NLP):** [More Information Needed]
- **License:** [More Information Needed]
- **Finetuned from model [optional]:** [More Information Needed]
### Model Sources [optional]
<!-- Provide the basic links for the model. -->
- **Repository:** [More Information Needed]
- **Paper [optional]:** [More Information Needed]
- **Demo [optional]:** [More Information Needed]
## Uses
<!-- Address questions around how the model is intended to be used, including the foreseeable users of the model and those affected by the model. -->
### Direct Use
<!-- This section is for the model use without fine-tuning or plugging into a larger ecosystem/app. -->
[More Information Needed]
### Downstream Use [optional]
<!-- This section is for the model use when fine-tuned for a task, or when plugged into a larger ecosystem/app -->
[More Information Needed]
### Out-of-Scope Use
<!-- This section addresses misuse, malicious use, and uses that the model will not work well for. -->
[More Information Needed]
## Bias, Risks, and Limitations
<!-- This section is meant to convey both technical and sociotechnical limitations. -->
[More Information Needed]
### Recommendations
<!-- This section is meant to convey recommendations with respect to the bias, risk, and technical limitations. -->
Users (both direct and downstream) should be made aware of the risks, biases and limitations of the model. More information needed for further recommendations.
## How to Get Started with the Model
Use the code below to get started with the model.
[More Information Needed]
## Training Details
### Training Data
<!-- This should link to a Dataset Card, perhaps with a short stub of information on what the training data is all about as well as documentation related to data pre-processing or additional filtering. -->
[More Information Needed]
### Training Procedure
<!-- This relates heavily to the Technical Specifications. Content here should link to that section when it is relevant to the training procedure. -->
#### Preprocessing [optional]
[More Information Needed]
#### Training Hyperparameters
- **Training regime:** [More Information Needed] <!--fp32, fp16 mixed precision, bf16 mixed precision, bf16 non-mixed precision, fp16 non-mixed precision, fp8 mixed precision -->
#### Speeds, Sizes, Times [optional]
<!-- This section provides information about throughput, start/end time, checkpoint size if relevant, etc. -->
[More Information Needed]
## Evaluation
<!-- This section describes the evaluation protocols and provides the results. -->
### Testing Data, Factors & Metrics
#### Testing Data
<!-- This should link to a Dataset Card if possible. -->
[More Information Needed]
#### Factors
<!-- These are the things the evaluation is disaggregating by, e.g., subpopulations or domains. -->
[More Information Needed]
#### Metrics
<!-- These are the evaluation metrics being used, ideally with a description of why. -->
[More Information Needed]
### Results
[More Information Needed]
#### Summary
## Model Examination [optional]
<!-- Relevant interpretability work for the model goes here -->
[More Information Needed]
## Environmental Impact
<!-- Total emissions (in grams of CO2eq) and additional considerations, such as electricity usage, go here. Edit the suggested text below accordingly -->
Carbon emissions can be estimated using the [Machine Learning Impact calculator](https://mlco2.github.io/impact#compute) presented in [Lacoste et al. (2019)](https://arxiv.org/abs/1910.09700).
- **Hardware Type:** [More Information Needed]
- **Hours used:** [More Information Needed]
- **Cloud Provider:** [More Information Needed]
- **Compute Region:** [More Information Needed]
- **Carbon Emitted:** [More Information Needed]
## Technical Specifications [optional]
### Model Architecture and Objective
[More Information Needed]
### Compute Infrastructure
[More Information Needed]
#### Hardware
[More Information Needed]
#### Software
[More Information Needed]
## Citation [optional]
<!-- If there is a paper or blog post introducing the model, the APA and Bibtex information for that should go in this section. -->
**BibTeX:**
[More Information Needed]
**APA:**
[More Information Needed]
## Glossary [optional]
<!-- If relevant, include terms and calculations in this section that can help readers understand the model or model card. -->
[More Information Needed]
## More Information [optional]
[More Information Needed]
## Model Card Authors [optional]
[More Information Needed]
## Model Card Contact
[More Information Needed]
## Training procedure
The following `bitsandbytes` quantization config was used during training:
- load_in_8bit: True
- llm_int8_threshold: 6.0
- llm_int8_skip_modules: None
- llm_int8_enable_fp32_cpu_offload: False
### Framework versions
- PEFT 0.6.2
|
RegalHyperus/BBEU-BBRWCJapaneseFancastRVCModels | RegalHyperus | "2024-10-03T08:53:01Z" | 0 | 2 | null | [
"license:openrail",
"region:us"
] | null | "2024-01-02T14:16:30Z" | ---
license: openrail
---
# English
Beyblade Burst Expanded Universe/Beyblade Burst: Real-World Chronicles voice models, except they were trained on the Japanese voice fancast.
Why?
"Hello. I'm the \[English\] voice actor for Kirara \[from Genshin Impact\] and I'm reaching out to you regarding the \[RVC voice\] models you made for Kirara \[from Genshin Impact\] and \[Nico Natsumiya from the Beyblade Burst Expanded Universe\]. I do not consent to having my voice used for AI so I am requesting that you take \[the RVC voice models of me\] down. Thank you." - Julia Gu, fancasted English voice of Nico Natsumiya
## End-User License Agreement
* You are responsible for whatever you make with any of my models.
* Please credit me if used. Thank you very much!
* No commercial usage. As a fanfiction writer, fancaster and AI hobbyist, I am not affiliated with any party involved in the SAG-AFTRA strikes and thus hold no responsibility for any jobs lost to corporate greed.
* If you wish to have a model of you taken down, contact me via X (formerly Twitter) like Julia Gu did way back in December 2023.
## Characters featured:
Aiger Akabane (CV: Tomohiro Ohmachi)
Aitor Talavera (CV: Ryohei Kimura)
Akira Takane (CV: Daiki Yamashita)
Alexia Gavira (CV: Kio Fukamachi)
Bel Daikokuten (CV: Tetsuya Kakihara)
Caiden Valdez (CV: Nobuhiko Okamoto)
Caleb Elm (CV: Soma Saito)
Dante Koryu (CV: Hiro Shimono)
Drew Lee (CV: Natsuki Hanae)
Eric Valentine (CV: Yuuma Uchida)
Free De La Hoya (CV: Yoshitaka Yamaya)
Ilya Mao (CV: Risa Taneda)
Hikaru Hizashi (CV: Yuki Kaji)
Hyuga Hizashi (CV: Gakuto Kajiwara)
Kai Tran (CV: KENN)
Koharu Tsukiyuki (CV: Reina Ueda)
Lia Tanikawa (CV: Manaka Iwami)
Logan Martin (CV: Shohei Komatsu)
Nahuel Cabrera (CV: Mark Ishii)
Nico Natsumiya (CV: Yui Nakajima)
Prithi Srinivasan (CV: Yui Horie)
Pritika Pathania (CV: Azumi Asakura)
Serena Bartlett (CV: Tomori Kusunoki)
Shizue Suzaki (CV: Tomoyo Kurosawa)
Shu Kurenai (CV: Junya Enoki)
Sierra Keagan (CV: Miyu Tomita)
Svetlana Sidorova (CV: Aya Endou)
Trixie Ansari (CV: Rie Takahashi)
Valt Aoi (CV: Daisuke Sakaguchi)
Xinran Zhao (CV: Mai Nakahara)
## Coming Soon:
Alex Cain (CV: Chihiro Suzuki)
Connor Cheng (CV: Ryota Osaka)
Isaiah Lance (CV: Shoya Chiba)
Lain Valhalla (CV: Wataru Hatano)
Preston Riley (CV: Gen Satou)
Ren Godai (CV: Saori Hayami)
For some characters you can just use other models from [MiscellaneousRVCModels](https://huggingface.co/RegalHyperus/MiscellaneousRVCModels/tree/main). The list is as follows:
David Tang / Hyperus18 / RegalHyperus (Me) (CV: Myself) - RegalHyperus
# 日本語
ベイブレードバースト エクスパンデッド ユニバース/ベイブレードバースト リアルワールド クロニクルズの声優モデル。ただし、私の日本のファンキャスティングで声を当てたキャラクターでトレーニングされています
注: 「Hyperus18」と「RegalHyperus」は同一人物です
## エンドユーザー ライセンス契約
* 私のモデルを使って作ったものはすべてあなたの責任です。
* 使用する場合、私にクレジットを付けてください。どうもありがとうございます!
* 商用利用は禁止です。ファンキャスターであり AI 愛好家である私は、声優を AI に置き換えることを検討している大企業とは提携していません。したがって、これらの企業が実際に声優を AI に置き換えた場合、ナレーションの仕事が失われても私は責任を負いません。
* モデルを削除したい場合は、2023年12月にジュリア・グーがしたように、X(旧Twitter)で私に連絡してください。
## 登場キャラクター:
赤刃アイガ (CV: 大町知広)
アイトール・タラヴェラ (CV: 木村良平)
アレクシア・ガビラ (CV: 深町季生)
鷹嶺亮 (CV: 山下大輝)
大黒天バベル (CV: 柿原徹也)
カイデン・バルデス (CV: 岡本信彦)
ケイレブ・エルム (CV: 斉藤壮馬)
虹龍ドラム (CV: 下野紘)
ドリュー・リー (CV: 花江夏樹)
エリック・バレンタイン (CV: 内田雄馬)
フリー・デ・ラ・ホーヤ(CV: 山谷祥生)
イリヤ・マオ(CV: 種田梨沙)
朝日ヒカル (CV: 梶裕貴)
朝日ヒュウガ (CV: 梶原岳人)
カイ・トラン (CV: KENN)
月雪小春 (CV: 上田麗奈)
谷川リア (CV: 石見舞菜香)
ローガン・マーティン (CV: 小松昌平)
ナウエル・カブレラ (CV: 石井マーク)
夏宮にこ (CV: 中島優衣)
プリティ・スリニヴァサン (CV: 堀江由衣)
プリティカ・パサニア (CV: 浅倉杏美)
セレナ・バートレット (CV: 楠木ともり)
洲崎静江 (CV: 黒沢ともよ)
紅シュウ (CV: 榎木淳弥)
シエラ・キーガン (CV: 富田美憂)
スヴェトラーナ・シドロヴァ (CV: 遠藤綾)
トリクシー・アンサリ (CV: 高橋李依)
蒼井バルト (CV: 阪口大助)
趙欣然 (CV: 中原麻衣)
## 近日公開:
アレックス・ケイン (CV: 鈴木千尋)
程舒皓 (CV: 逢坂良太)
アイザイア・ランス (CV: 千葉翔也)
レーン・ヴァルハラ (CV: 羽多野渉)
プレストン・ライリー (CV: 佐藤元)
五代廉(CV:早見沙織)
一部のキャラクターについては、[MiscellaneousRVCModels](https://huggingface.co/RegalHyperus/MiscellaneousRVCModels/tree/main) の他のモデルを使用できます。リストは次のとおりです:
David Tang / Hyperus18 / RegalHyperus (俺) (CV: 俺自身) - RegalHyperus |
s3nh/Walmart-the-bag-WordWoven-13B-GGUF | s3nh | "2024-01-02T14:17:10Z" | 0 | 0 | transformers | [
"transformers",
"text-generation",
"zh",
"en",
"license:openrail",
"endpoints_compatible",
"region:us"
] | text-generation | "2024-01-02T14:17:09Z" |
---
license: openrail
pipeline_tag: text-generation
library_name: transformers
language:
- zh
- en
---
## Original model card
Buy me a coffee if you like this project ;)
<a href="https://www.buymeacoffee.com/s3nh"><img src="https://www.buymeacoffee.com/assets/img/guidelines/download-assets-sm-1.svg" alt=""></a>
#### Description
GGUF Format model files for [This project](https://huggingface.co/Walmart-the-bag/WordWoven-13B).
### GGUF Specs
GGUF is a format based on the existing GGJT, but makes a few changes to the format to make it more extensible and easier to use. The following features are desired:
Single-file deployment: they can be easily distributed and loaded, and do not require any external files for additional information.
Extensible: new features can be added to GGML-based executors/new information can be added to GGUF models without breaking compatibility with existing models.
mmap compatibility: models can be loaded using mmap for fast loading and saving.
Easy to use: models can be easily loaded and saved using a small amount of code, with no need for external libraries, regardless of the language used.
Full information: all information needed to load a model is contained in the model file, and no additional information needs to be provided by the user.
The key difference between GGJT and GGUF is the use of a key-value structure for the hyperparameters (now referred to as metadata), rather than a list of untyped values.
This allows for new metadata to be added without breaking compatibility with existing models, and to annotate the model with additional information that may be useful for
inference or for identifying the model.
### Perplexity params
Model Measure Q2_K Q3_K_S Q3_K_M Q3_K_L Q4_0 Q4_1 Q4_K_S Q4_K_M Q5_0 Q5_1 Q5_K_S Q5_K_M Q6_K Q8_0 F16
7B perplexity 6.7764 6.4571 6.1503 6.0869 6.1565 6.0912 6.0215 5.9601 5.9862 5.9481 5.9419 5.9208 5.9110 5.9070 5.9066
13B perplexity 5.8545 5.6033 5.4498 5.4063 5.3860 5.3608 5.3404 5.3002 5.2856 5.2706 5.2785 5.2638 5.2568 5.2548 5.2543
### inference
TODO
# Original model card
|
UnionXX24/sentiment_analysis_app | UnionXX24 | "2024-01-02T14:21:28Z" | 0 | 0 | transformers | [
"transformers",
"tensorboard",
"safetensors",
"license:apache-2.0",
"endpoints_compatible",
"region:us"
] | null | "2024-01-02T14:17:56Z" | ---
license: apache-2.0
---
|
agusroccohd/elaspirantev2 | agusroccohd | "2024-01-02T14:24:50Z" | 0 | 0 | null | [
"region:us"
] | null | "2024-01-02T14:19:01Z" | Entry not found |
odunola/extra_yoruba_data | odunola | "2024-01-02T14:23:29Z" | 0 | 0 | null | [
"region:us"
] | null | "2024-01-02T14:23:28Z" | Entry not found |
Rahuldabra/Rahul | Rahuldabra | "2024-01-02T14:24:00Z" | 0 | 0 | null | [
"license:apache-2.0",
"region:us"
] | null | "2024-01-02T14:24:00Z" | ---
license: apache-2.0
---
|
anna-social-wonder/pokemon-lora | anna-social-wonder | "2024-01-02T14:29:15Z" | 0 | 0 | null | [
"region:us"
] | null | "2024-01-02T14:29:15Z" | Entry not found |
Kovid63/whisper-japan-fine | Kovid63 | "2024-01-02T14:31:55Z" | 0 | 0 | null | [
"region:us"
] | null | "2024-01-02T14:31:55Z" | Entry not found |
sergej23/caccio | sergej23 | "2024-01-02T14:32:28Z" | 0 | 0 | null | [
"region:us"
] | null | "2024-01-02T14:32:28Z" | Entry not found |
litfeng/out_dog | litfeng | "2024-01-02T14:36:07Z" | 0 | 0 | null | [
"region:us"
] | null | "2024-01-02T14:36:07Z" | Entry not found |
ziyuyuyuyu1/ACG-class-cond-ckpt | ziyuyuyuyu1 | "2024-01-02T14:37:01Z" | 0 | 0 | null | [
"region:us"
] | null | "2024-01-02T14:37:01Z" | Entry not found |
yhavinga/dutch-llama-tokenizer | yhavinga | "2024-01-04T11:52:30Z" | 0 | 1 | null | [
"license:apache-2.0",
"region:us"
] | null | "2024-01-02T14:39:35Z" | ---
license: apache-2.0
---
# Dutch-Llama Tokenizer
## Overview
The Dutch-Llama Tokenizer is a versatile tokenizer trained to handle a variety of languages and formats, including Dutch, English, Python code, Markdown, and general text. It's based on a dataset consisting of diverse sources, which ensures its capability to tokenize a wide range of text inputs effectively.
## Dataset Composition
The tokenizer was trained on a comprehensive dataset, including:
- MC4 Dutch and English texts (195M)
- English and Dutch Wikipedia (278M and 356M, respectively)
- Dutch and English book datasets (211M and 355M, respectively)
- Dutch news articles (256M)
- CodeParrot GitHub Python code (158M)
- CodeSearchNet Python code (126M)
- Markdown files with math markup (5.8M)
- Arxiv scientific papers (169M)
## Tokenizer Settings
The tokenizer was trained using the `spm_train` command with the following settings:
- Model Type: Byte Pair Encoding (BPE)
- Vocab Size: 32,000
- Character Coverage: 100%
- Support for splitting digits and whitespace-only pieces
- Optimized for large corpus training
- Byte Fallback and language acceptance for Dutch (nl) and English (en)
- Special tokens and IDs for unknown, beginning of sentence, end of sentence, padding, and custom user-defined symbols
## Installation
To use the Dutch-Llama Tokenizer, ensure you have Python 3.10.12 or later installed. Then, install the Transformers library from Hugging Face:
```shell
pip install transformers
```
## Usage
First, import the `AutoTokenizer` from the Transformers library and load the Dutch-Llama Tokenizer:
```python
from transformers import AutoTokenizer
tokenizer = AutoTokenizer.from_pretrained("yhavinga/dutch-llama-tokenizer")
```
To tokenize text, use the `tokenizer.tokenize` method. For converting tokens to IDs and decoding them back to text, use `tokenizer.convert_tokens_to_ids` and `tokenizer.decode` respectively:
```python
# Example text
text = "Steenvliegen of oevervliegen[2] (Plecoptera) 华为发布Mate60手机"
# Tokenization and decoding
tokens = tokenizer.tokenize(text)
token_ids = tokenizer.convert_tokens_to_ids(tokens)
decoded_text = tokenizer.decode(token_ids)
print(decoded_text)
```
## Dutch Tokenizer Arena
Compare the effectiveness of this tokenizer on different inputs at the Hugging Face Space: [Dutch Tokenizer Arena](https://huggingface.co/spaces/yhavinga/dutch-tokenizer-arena).
## Comparison with Other Tokenizers
The following table shows the number of tokens produced by the Dutch-Llama Tokenizer, the Mistral Tokenizer, the GroNLP GPT-2 Dutch Tokenizer, and the UL2 Dutch Tokenizer on a variety of inputs.
| Input Type | Dutch LLama (32k) | Mistral (32k) | GroNLP GPT-2 Dutch (40k) | UL2 Dutch (32k) |
|--------------|-------------------|---------------|--------------------------|-------------------|
| Dutch news | 440 | 658 | 408 | 410 |
| English news | 414 | 404 | 565 | 402 |
| Code python | 566 | 582 | 767 | 639 (no newlines) |
| LaTeX math | 491 | 497 | 717 | 666 (no newlines) |
| **Total** | 1911 | 2141 | 2457 | 2117 |
🇳🇱 🇧🇪🐍📐
|
AdeWT/P1G5-Set-1-Ade-Wil | AdeWT | "2024-01-02T14:42:56Z" | 0 | 0 | null | [
"region:us"
] | null | "2024-01-02T14:42:14Z" | Entry not found |
casonir/prova | casonir | "2024-01-02T14:46:48Z" | 0 | 0 | null | [
"region:us"
] | null | "2024-01-02T14:46:48Z" | Entry not found |
Enes01/Text_to_voice | Enes01 | "2024-01-02T14:49:04Z" | 0 | 0 | null | [
"region:us"
] | null | "2024-01-02T14:49:04Z" | Entry not found |
odunola/whisper_yoruba_distilled | odunola | "2024-01-02T15:19:48Z" | 0 | 0 | transformers | [
"transformers",
"safetensors",
"whisper",
"automatic-speech-recognition",
"endpoints_compatible",
"region:us"
] | automatic-speech-recognition | "2024-01-02T14:53:58Z" | Entry not found |
aarongrainer/q-FrozenLake-v1-4x4-noSlippery | aarongrainer | "2024-01-02T14:55:03Z" | 0 | 0 | null | [
"FrozenLake-v1-4x4-no_slippery",
"q-learning",
"reinforcement-learning",
"custom-implementation",
"model-index",
"region:us"
] | reinforcement-learning | "2024-01-02T14:55:00Z" | ---
tags:
- FrozenLake-v1-4x4-no_slippery
- q-learning
- reinforcement-learning
- custom-implementation
model-index:
- name: q-FrozenLake-v1-4x4-noSlippery
results:
- task:
type: reinforcement-learning
name: reinforcement-learning
dataset:
name: FrozenLake-v1-4x4-no_slippery
type: FrozenLake-v1-4x4-no_slippery
metrics:
- type: mean_reward
value: 1.00 +/- 0.00
name: mean_reward
verified: false
---
# **Q-Learning** Agent playing1 **FrozenLake-v1**
This is a trained model of a **Q-Learning** agent playing **FrozenLake-v1** .
## Usage
model = load_from_hub(repo_id="aarongrainer/q-FrozenLake-v1-4x4-noSlippery", filename="q-learning.pkl")
# Don't forget to check if you need to add additional attributes (is_slippery=False etc)
env = gym.make(model["env_id"])
|
rolo9/xlm-roberta-base-finetuned-squad-es | rolo9 | "2024-01-02T14:55:45Z" | 0 | 0 | null | [
"region:us"
] | null | "2024-01-02T14:55:45Z" | Entry not found |
vwxyzjn/EleutherAI_pythia-410m-deduped__ppo__tldr | vwxyzjn | "2024-01-02T14:56:48Z" | 0 | 0 | null | [
"region:us"
] | null | "2024-01-02T14:56:48Z" | Entry not found |
maptun/maptunwoman | maptun | "2024-01-02T14:57:04Z" | 0 | 0 | null | [
"region:us"
] | null | "2024-01-02T14:57:04Z" | Entry not found |
bartowski/Nous-Hermes-2-SOLAR-10.7B-exl2 | bartowski | "2024-03-02T23:49:06Z" | 0 | 4 | null | [
"SOLAR",
"instruct",
"finetune",
"chatml",
"gpt4",
"synthetic data",
"distillation",
"text-generation",
"en",
"base_model:upstage/SOLAR-10.7B-v1.0",
"base_model:finetune:upstage/SOLAR-10.7B-v1.0",
"license:apache-2.0",
"region:us"
] | text-generation | "2024-01-02T14:59:19Z" | ---
base_model: upstage/SOLAR-10.7B-v1.0
tags:
- SOLAR
- instruct
- finetune
- chatml
- gpt4
- synthetic data
- distillation
model-index:
- name: Nous-Hermes-2-SOLAR-10.7B
results: []
license: apache-2.0
language:
- en
quantized_by: bartowski
pipeline_tag: text-generation
---
## Exllama v2 Quantizations of Nous-Hermes-2-SOLAR-10.7B
Using <a href="https://github.com/turboderp/exllamav2/releases/tag/v0.0.11">turboderp's ExLlamaV2 v0.0.11</a> for quantization.
<b>The "main" branch only contains the measurement.json, download one of the other branches for the model (see below)</b>
Each branch contains an individual bits per weight, with the main one containing only the meaurement.json for further conversions.
Original model: https://huggingface.co/NousResearch/Nous-Hermes-2-SOLAR-10.7B
| Branch | Bits | lm_head bits | VRAM (4k) | VRAM (16k) | VRAM (32k) | Description |
| ----- | ---- | ------- | ------ | ------ | ------ | ------------ |
| [8_0](https://huggingface.co/bartowski/Nous-Hermes-2-SOLAR-10.7B-exl2/tree/8_0) | 8.0 | 8.0 | 11.9 GB | 13.3 GB | 15.3 GB | Maximum quality that ExLlamaV2 can produce, near unquantized performance. |
| [6_5](https://huggingface.co/bartowski/Nous-Hermes-2-SOLAR-10.7B-exl2/tree/6_5) | 6.5 | 8.0 | 10.3 GB | 11.7 GB | 13.7 GB | Very similar to 8.0, good tradeoff of size vs performance, **recommended**. |
| [5_0](https://huggingface.co/bartowski/Nous-Hermes-2-SOLAR-10.7B-exl2/tree/5_0) | 5.0 | 6.0 | 8.3 GB | 9.7 GB | 11.7 GB | Slightly lower quality vs 6.5, but usable on 8GB cards. |
| [4_25](https://huggingface.co/bartowski/Nous-Hermes-2-SOLAR-10.7B-exl2/tree/4_25) | 4.25 | 6.0 | 7.4 GB | 8.6 GB | 10.6 GB | GPTQ equivalent bits per weight, slightly higher quality. |
| [3_5](https://huggingface.co/bartowski/Nous-Hermes-2-SOLAR-10.7B-exl2/tree/3_5) | 3.5 | 6.0 | 6.4 GB | 7.8 GB | 9.8 GB | Lower quality, only use if you have to. |
## Download instructions
With git:
```shell
git clone --single-branch --branch 4_0 https://huggingface.co/bartowski/Nous-Hermes-2-SOLAR-10.7B-exl2
```
With huggingface hub (credit to TheBloke for instructions):
```shell
pip3 install huggingface-hub
```
To download the `main` (only useful if you only care about measurement.json) branch to a folder called `Nous-Hermes-2-SOLAR-10.7B-exl2`:
```shell
mkdir Nous-Hermes-2-SOLAR-10.7B-exl2
huggingface-cli download bartowski/Nous-Hermes-2-SOLAR-10.7B-exl2 --local-dir Nous-Hermes-2-SOLAR-10.7B-exl2 --local-dir-use-symlinks False
```
To download from a different branch, add the `--revision` parameter:
```shell
mkdir Nous-Hermes-2-SOLAR-10.7B-exl2
huggingface-cli download bartowski/Nous-Hermes-2-SOLAR-10.7B-exl2 --revision 4_0 --local-dir Nous-Hermes-2-SOLAR-10.7B-exl2 --local-dir-use-symlinks False
```
|
AltLuv/pokemon-base-line | AltLuv | "2024-01-03T14:52:28Z" | 0 | 0 | diffusers | [
"diffusers",
"safetensors",
"diffusers:UTTIPipeline",
"region:us"
] | null | "2024-01-02T15:00:29Z" | Entry not found |
aarongrainer/q-taxi-v3 | aarongrainer | "2024-01-02T15:07:25Z" | 0 | 0 | null | [
"Taxi-v3",
"q-learning",
"reinforcement-learning",
"custom-implementation",
"model-index",
"region:us"
] | reinforcement-learning | "2024-01-02T15:07:23Z" | ---
tags:
- Taxi-v3
- q-learning
- reinforcement-learning
- custom-implementation
model-index:
- name: q-taxi-v3
results:
- task:
type: reinforcement-learning
name: reinforcement-learning
dataset:
name: Taxi-v3
type: Taxi-v3
metrics:
- type: mean_reward
value: 7.52 +/- 2.73
name: mean_reward
verified: false
---
# **Q-Learning** Agent playing1 **Taxi-v3**
This is a trained model of a **Q-Learning** agent playing **Taxi-v3** .
## Usage
model = load_from_hub(repo_id="aarongrainer/q-taxi-v3", filename="q-learning.pkl")
# Don't forget to check if you need to add additional attributes (is_slippery=False etc)
env = gym.make(model["env_id"])
|
behzadnet/Llama-2-7b-chat-hf-sharded-bf16-fine-tuned-adapters_humanMix_Seed113 | behzadnet | "2024-01-02T15:07:56Z" | 0 | 0 | peft | [
"peft",
"arxiv:1910.09700",
"base_model:Trelis/Llama-2-7b-chat-hf-sharded-bf16",
"base_model:adapter:Trelis/Llama-2-7b-chat-hf-sharded-bf16",
"region:us"
] | null | "2024-01-02T15:07:48Z" | ---
library_name: peft
base_model: Trelis/Llama-2-7b-chat-hf-sharded-bf16
---
# Model Card for Model ID
<!-- Provide a quick summary of what the model is/does. -->
## Model Details
### Model Description
<!-- Provide a longer summary of what this model is. -->
- **Developed by:** [More Information Needed]
- **Shared by [optional]:** [More Information Needed]
- **Model type:** [More Information Needed]
- **Language(s) (NLP):** [More Information Needed]
- **License:** [More Information Needed]
- **Finetuned from model [optional]:** [More Information Needed]
### Model Sources [optional]
<!-- Provide the basic links for the model. -->
- **Repository:** [More Information Needed]
- **Paper [optional]:** [More Information Needed]
- **Demo [optional]:** [More Information Needed]
## Uses
<!-- Address questions around how the model is intended to be used, including the foreseeable users of the model and those affected by the model. -->
### Direct Use
<!-- This section is for the model use without fine-tuning or plugging into a larger ecosystem/app. -->
[More Information Needed]
### Downstream Use [optional]
<!-- This section is for the model use when fine-tuned for a task, or when plugged into a larger ecosystem/app -->
[More Information Needed]
### Out-of-Scope Use
<!-- This section addresses misuse, malicious use, and uses that the model will not work well for. -->
[More Information Needed]
## Bias, Risks, and Limitations
<!-- This section is meant to convey both technical and sociotechnical limitations. -->
[More Information Needed]
### Recommendations
<!-- This section is meant to convey recommendations with respect to the bias, risk, and technical limitations. -->
Users (both direct and downstream) should be made aware of the risks, biases and limitations of the model. More information needed for further recommendations.
## How to Get Started with the Model
Use the code below to get started with the model.
[More Information Needed]
## Training Details
### Training Data
<!-- This should link to a Data Card, perhaps with a short stub of information on what the training data is all about as well as documentation related to data pre-processing or additional filtering. -->
[More Information Needed]
### Training Procedure
<!-- This relates heavily to the Technical Specifications. Content here should link to that section when it is relevant to the training procedure. -->
#### Preprocessing [optional]
[More Information Needed]
#### Training Hyperparameters
- **Training regime:** [More Information Needed] <!--fp32, fp16 mixed precision, bf16 mixed precision, bf16 non-mixed precision, fp16 non-mixed precision, fp8 mixed precision -->
#### Speeds, Sizes, Times [optional]
<!-- This section provides information about throughput, start/end time, checkpoint size if relevant, etc. -->
[More Information Needed]
## Evaluation
<!-- This section describes the evaluation protocols and provides the results. -->
### Testing Data, Factors & Metrics
#### Testing Data
<!-- This should link to a Data Card if possible. -->
[More Information Needed]
#### Factors
<!-- These are the things the evaluation is disaggregating by, e.g., subpopulations or domains. -->
[More Information Needed]
#### Metrics
<!-- These are the evaluation metrics being used, ideally with a description of why. -->
[More Information Needed]
### Results
[More Information Needed]
#### Summary
## Model Examination [optional]
<!-- Relevant interpretability work for the model goes here -->
[More Information Needed]
## Environmental Impact
<!-- Total emissions (in grams of CO2eq) and additional considerations, such as electricity usage, go here. Edit the suggested text below accordingly -->
Carbon emissions can be estimated using the [Machine Learning Impact calculator](https://mlco2.github.io/impact#compute) presented in [Lacoste et al. (2019)](https://arxiv.org/abs/1910.09700).
- **Hardware Type:** [More Information Needed]
- **Hours used:** [More Information Needed]
- **Cloud Provider:** [More Information Needed]
- **Compute Region:** [More Information Needed]
- **Carbon Emitted:** [More Information Needed]
## Technical Specifications [optional]
### Model Architecture and Objective
[More Information Needed]
### Compute Infrastructure
[More Information Needed]
#### Hardware
[More Information Needed]
#### Software
[More Information Needed]
## Citation [optional]
<!-- If there is a paper or blog post introducing the model, the APA and Bibtex information for that should go in this section. -->
**BibTeX:**
[More Information Needed]
**APA:**
[More Information Needed]
## Glossary [optional]
<!-- If relevant, include terms and calculations in this section that can help readers understand the model or model card. -->
[More Information Needed]
## More Information [optional]
[More Information Needed]
## Model Card Authors [optional]
[More Information Needed]
## Model Card Contact
[More Information Needed]
## Training procedure
The following `bitsandbytes` quantization config was used during training:
- load_in_8bit: False
- load_in_4bit: True
- llm_int8_threshold: 6.0
- llm_int8_skip_modules: None
- llm_int8_enable_fp32_cpu_offload: False
- llm_int8_has_fp16_weight: False
- bnb_4bit_quant_type: nf4
- bnb_4bit_use_double_quant: True
- bnb_4bit_compute_dtype: bfloat16
### Framework versions
- PEFT 0.7.0.dev0
## Training procedure
The following `bitsandbytes` quantization config was used during training:
- load_in_8bit: False
- load_in_4bit: True
- llm_int8_threshold: 6.0
- llm_int8_skip_modules: None
- llm_int8_enable_fp32_cpu_offload: False
- llm_int8_has_fp16_weight: False
- bnb_4bit_quant_type: nf4
- bnb_4bit_use_double_quant: True
- bnb_4bit_compute_dtype: bfloat16
### Framework versions
- PEFT 0.7.0.dev0
|
behzadnet/Llama-2-7b-chat-hf-sharded-bf16-fine-tuned_humanMix_Seed113 | behzadnet | "2024-01-02T15:08:05Z" | 0 | 0 | peft | [
"peft",
"arxiv:1910.09700",
"base_model:Trelis/Llama-2-7b-chat-hf-sharded-bf16",
"base_model:adapter:Trelis/Llama-2-7b-chat-hf-sharded-bf16",
"region:us"
] | null | "2024-01-02T15:08:02Z" | ---
library_name: peft
base_model: Trelis/Llama-2-7b-chat-hf-sharded-bf16
---
# Model Card for Model ID
<!-- Provide a quick summary of what the model is/does. -->
## Model Details
### Model Description
<!-- Provide a longer summary of what this model is. -->
- **Developed by:** [More Information Needed]
- **Shared by [optional]:** [More Information Needed]
- **Model type:** [More Information Needed]
- **Language(s) (NLP):** [More Information Needed]
- **License:** [More Information Needed]
- **Finetuned from model [optional]:** [More Information Needed]
### Model Sources [optional]
<!-- Provide the basic links for the model. -->
- **Repository:** [More Information Needed]
- **Paper [optional]:** [More Information Needed]
- **Demo [optional]:** [More Information Needed]
## Uses
<!-- Address questions around how the model is intended to be used, including the foreseeable users of the model and those affected by the model. -->
### Direct Use
<!-- This section is for the model use without fine-tuning or plugging into a larger ecosystem/app. -->
[More Information Needed]
### Downstream Use [optional]
<!-- This section is for the model use when fine-tuned for a task, or when plugged into a larger ecosystem/app -->
[More Information Needed]
### Out-of-Scope Use
<!-- This section addresses misuse, malicious use, and uses that the model will not work well for. -->
[More Information Needed]
## Bias, Risks, and Limitations
<!-- This section is meant to convey both technical and sociotechnical limitations. -->
[More Information Needed]
### Recommendations
<!-- This section is meant to convey recommendations with respect to the bias, risk, and technical limitations. -->
Users (both direct and downstream) should be made aware of the risks, biases and limitations of the model. More information needed for further recommendations.
## How to Get Started with the Model
Use the code below to get started with the model.
[More Information Needed]
## Training Details
### Training Data
<!-- This should link to a Data Card, perhaps with a short stub of information on what the training data is all about as well as documentation related to data pre-processing or additional filtering. -->
[More Information Needed]
### Training Procedure
<!-- This relates heavily to the Technical Specifications. Content here should link to that section when it is relevant to the training procedure. -->
#### Preprocessing [optional]
[More Information Needed]
#### Training Hyperparameters
- **Training regime:** [More Information Needed] <!--fp32, fp16 mixed precision, bf16 mixed precision, bf16 non-mixed precision, fp16 non-mixed precision, fp8 mixed precision -->
#### Speeds, Sizes, Times [optional]
<!-- This section provides information about throughput, start/end time, checkpoint size if relevant, etc. -->
[More Information Needed]
## Evaluation
<!-- This section describes the evaluation protocols and provides the results. -->
### Testing Data, Factors & Metrics
#### Testing Data
<!-- This should link to a Data Card if possible. -->
[More Information Needed]
#### Factors
<!-- These are the things the evaluation is disaggregating by, e.g., subpopulations or domains. -->
[More Information Needed]
#### Metrics
<!-- These are the evaluation metrics being used, ideally with a description of why. -->
[More Information Needed]
### Results
[More Information Needed]
#### Summary
## Model Examination [optional]
<!-- Relevant interpretability work for the model goes here -->
[More Information Needed]
## Environmental Impact
<!-- Total emissions (in grams of CO2eq) and additional considerations, such as electricity usage, go here. Edit the suggested text below accordingly -->
Carbon emissions can be estimated using the [Machine Learning Impact calculator](https://mlco2.github.io/impact#compute) presented in [Lacoste et al. (2019)](https://arxiv.org/abs/1910.09700).
- **Hardware Type:** [More Information Needed]
- **Hours used:** [More Information Needed]
- **Cloud Provider:** [More Information Needed]
- **Compute Region:** [More Information Needed]
- **Carbon Emitted:** [More Information Needed]
## Technical Specifications [optional]
### Model Architecture and Objective
[More Information Needed]
### Compute Infrastructure
[More Information Needed]
#### Hardware
[More Information Needed]
#### Software
[More Information Needed]
## Citation [optional]
<!-- If there is a paper or blog post introducing the model, the APA and Bibtex information for that should go in this section. -->
**BibTeX:**
[More Information Needed]
**APA:**
[More Information Needed]
## Glossary [optional]
<!-- If relevant, include terms and calculations in this section that can help readers understand the model or model card. -->
[More Information Needed]
## More Information [optional]
[More Information Needed]
## Model Card Authors [optional]
[More Information Needed]
## Model Card Contact
[More Information Needed]
## Training procedure
The following `bitsandbytes` quantization config was used during training:
- load_in_8bit: False
- load_in_4bit: True
- llm_int8_threshold: 6.0
- llm_int8_skip_modules: None
- llm_int8_enable_fp32_cpu_offload: False
- llm_int8_has_fp16_weight: False
- bnb_4bit_quant_type: nf4
- bnb_4bit_use_double_quant: True
- bnb_4bit_compute_dtype: bfloat16
### Framework versions
- PEFT 0.7.0.dev0
|
iamsubrata/Llama-2-7b-chat-hf-sharded-bf16-fine-tuned-adapters | iamsubrata | "2024-01-02T18:39:22Z" | 0 | 0 | peft | [
"peft",
"arxiv:1910.09700",
"base_model:Trelis/Llama-2-7b-chat-hf-sharded-bf16",
"base_model:adapter:Trelis/Llama-2-7b-chat-hf-sharded-bf16",
"region:us"
] | null | "2024-01-02T15:12:29Z" | ---
library_name: peft
base_model: Trelis/Llama-2-7b-chat-hf-sharded-bf16
---
# Model Card for Model ID
<!-- Provide a quick summary of what the model is/does. -->
## Model Details
### Model Description
<!-- Provide a longer summary of what this model is. -->
- **Developed by:** [More Information Needed]
- **Funded by [optional]:** [More Information Needed]
- **Shared by [optional]:** [More Information Needed]
- **Model type:** [More Information Needed]
- **Language(s) (NLP):** [More Information Needed]
- **License:** [More Information Needed]
- **Finetuned from model [optional]:** [More Information Needed]
### Model Sources [optional]
<!-- Provide the basic links for the model. -->
- **Repository:** [More Information Needed]
- **Paper [optional]:** [More Information Needed]
- **Demo [optional]:** [More Information Needed]
## Uses
<!-- Address questions around how the model is intended to be used, including the foreseeable users of the model and those affected by the model. -->
### Direct Use
<!-- This section is for the model use without fine-tuning or plugging into a larger ecosystem/app. -->
[More Information Needed]
### Downstream Use [optional]
<!-- This section is for the model use when fine-tuned for a task, or when plugged into a larger ecosystem/app -->
[More Information Needed]
### Out-of-Scope Use
<!-- This section addresses misuse, malicious use, and uses that the model will not work well for. -->
[More Information Needed]
## Bias, Risks, and Limitations
<!-- This section is meant to convey both technical and sociotechnical limitations. -->
[More Information Needed]
### Recommendations
<!-- This section is meant to convey recommendations with respect to the bias, risk, and technical limitations. -->
Users (both direct and downstream) should be made aware of the risks, biases and limitations of the model. More information needed for further recommendations.
## How to Get Started with the Model
Use the code below to get started with the model.
[More Information Needed]
## Training Details
### Training Data
<!-- This should link to a Dataset Card, perhaps with a short stub of information on what the training data is all about as well as documentation related to data pre-processing or additional filtering. -->
[More Information Needed]
### Training Procedure
<!-- This relates heavily to the Technical Specifications. Content here should link to that section when it is relevant to the training procedure. -->
#### Preprocessing [optional]
[More Information Needed]
#### Training Hyperparameters
- **Training regime:** [More Information Needed] <!--fp32, fp16 mixed precision, bf16 mixed precision, bf16 non-mixed precision, fp16 non-mixed precision, fp8 mixed precision -->
#### Speeds, Sizes, Times [optional]
<!-- This section provides information about throughput, start/end time, checkpoint size if relevant, etc. -->
[More Information Needed]
## Evaluation
<!-- This section describes the evaluation protocols and provides the results. -->
### Testing Data, Factors & Metrics
#### Testing Data
<!-- This should link to a Dataset Card if possible. -->
[More Information Needed]
#### Factors
<!-- These are the things the evaluation is disaggregating by, e.g., subpopulations or domains. -->
[More Information Needed]
#### Metrics
<!-- These are the evaluation metrics being used, ideally with a description of why. -->
[More Information Needed]
### Results
[More Information Needed]
#### Summary
## Model Examination [optional]
<!-- Relevant interpretability work for the model goes here -->
[More Information Needed]
## Environmental Impact
<!-- Total emissions (in grams of CO2eq) and additional considerations, such as electricity usage, go here. Edit the suggested text below accordingly -->
Carbon emissions can be estimated using the [Machine Learning Impact calculator](https://mlco2.github.io/impact#compute) presented in [Lacoste et al. (2019)](https://arxiv.org/abs/1910.09700).
- **Hardware Type:** [More Information Needed]
- **Hours used:** [More Information Needed]
- **Cloud Provider:** [More Information Needed]
- **Compute Region:** [More Information Needed]
- **Carbon Emitted:** [More Information Needed]
## Technical Specifications [optional]
### Model Architecture and Objective
[More Information Needed]
### Compute Infrastructure
[More Information Needed]
#### Hardware
[More Information Needed]
#### Software
[More Information Needed]
## Citation [optional]
<!-- If there is a paper or blog post introducing the model, the APA and Bibtex information for that should go in this section. -->
**BibTeX:**
[More Information Needed]
**APA:**
[More Information Needed]
## Glossary [optional]
<!-- If relevant, include terms and calculations in this section that can help readers understand the model or model card. -->
[More Information Needed]
## More Information [optional]
[More Information Needed]
## Model Card Authors [optional]
[More Information Needed]
## Model Card Contact
[More Information Needed]
### Framework versions
- PEFT 0.7.2.dev0 |
odunola/yoruba_whisper_new | odunola | "2024-01-02T15:13:44Z" | 0 | 0 | transformers | [
"transformers",
"pytorch",
"tensorboard",
"whisper",
"automatic-speech-recognition",
"generated_from_trainer",
"base_model:openai/whisper-tiny",
"base_model:finetune:openai/whisper-tiny",
"license:apache-2.0",
"endpoints_compatible",
"region:us"
] | automatic-speech-recognition | "2024-01-02T15:13:12Z" | ---
license: apache-2.0
base_model: openai/whisper-tiny
tags:
- generated_from_trainer
metrics:
- wer
model-index:
- name: yoruba_whisper
results: []
---
<!-- This model card has been generated automatically according to the information the Trainer had access to. You
should probably proofread and complete it, then remove this comment. -->
# yoruba_whisper
This model is a fine-tuned version of [openai/whisper-tiny](https://huggingface.co/openai/whisper-tiny) on an unknown dataset.
It achieves the following results on the evaluation set:
- Loss: 0.7232
- Wer: 75.4654
## Model description
More information needed
## Intended uses & limitations
More information needed
## Training and evaluation data
More information needed
## Training procedure
### Training hyperparameters
The following hyperparameters were used during training:
- learning_rate: 1e-05
- train_batch_size: 16
- eval_batch_size: 16
- seed: 42
- optimizer: Adam with betas=(0.9,0.999) and epsilon=1e-08
- lr_scheduler_type: linear
- lr_scheduler_warmup_steps: 500
- training_steps: 3000
- mixed_precision_training: Native AMP
### Training results
| Training Loss | Epoch | Step | Validation Loss | Wer |
|:-------------:|:-----:|:----:|:---------------:|:-------:|
| 0.8696 | 1.85 | 1000 | 0.8730 | 79.4692 |
| 0.6117 | 3.7 | 2000 | 0.7474 | 75.6604 |
| 0.5195 | 5.56 | 3000 | 0.7232 | 75.4654 |
### Framework versions
- Transformers 4.36.2
- Pytorch 2.1.0+cu118
- Datasets 2.16.1
- Tokenizers 0.15.0
|
rayzox57/SA2B_RVC | rayzox57 | "2024-01-02T15:16:03Z" | 0 | 0 | null | [
"license:openrail",
"region:us"
] | null | "2024-01-02T15:15:20Z" | ---
license: openrail
---
|
StatsGary/mistral-7b-brian-clough-ft | StatsGary | "2024-01-02T15:18:08Z" | 0 | 0 | null | [
"safetensors",
"en",
"license:mit",
"region:us"
] | null | "2024-01-02T15:16:08Z" | ---
license: mit
language:
- en
--- |
rbrgAlou/Reinforce-CartPole-v1 | rbrgAlou | "2024-01-02T15:19:50Z" | 0 | 0 | null | [
"CartPole-v1",
"reinforce",
"reinforcement-learning",
"custom-implementation",
"deep-rl-class",
"model-index",
"region:us"
] | reinforcement-learning | "2024-01-02T15:19:41Z" | ---
tags:
- CartPole-v1
- reinforce
- reinforcement-learning
- custom-implementation
- deep-rl-class
model-index:
- name: Reinforce-CartPole-v1
results:
- task:
type: reinforcement-learning
name: reinforcement-learning
dataset:
name: CartPole-v1
type: CartPole-v1
metrics:
- type: mean_reward
value: 500.00 +/- 0.00
name: mean_reward
verified: false
---
# **Reinforce** Agent playing **CartPole-v1**
This is a trained model of a **Reinforce** agent playing **CartPole-v1** .
To learn to use this model and train yours check Unit 4 of the Deep Reinforcement Learning Course: https://huggingface.co/deep-rl-course/unit4/introduction
|
leeloli/cha-eunwoo-by-leelo | leeloli | "2024-01-02T15:22:58Z" | 0 | 0 | null | [
"license:openrail",
"region:us"
] | null | "2024-01-02T15:22:09Z" | ---
license: openrail
---
|
StephDeBayonne/distilbert-base-uncased.finetuned-emotion | StephDeBayonne | "2024-01-02T15:25:04Z" | 0 | 0 | null | [
"region:us"
] | null | "2024-01-02T15:25:03Z" | Entry not found |
nguyenduc513/AI | nguyenduc513 | "2024-01-02T15:26:50Z" | 0 | 0 | null | [
"license:bigcode-openrail-m",
"region:us"
] | null | "2024-01-02T15:26:50Z" | ---
license: bigcode-openrail-m
---
|
Katinan/happy-tt | Katinan | "2024-01-02T15:27:09Z" | 0 | 0 | null | [
"region:us"
] | null | "2024-01-02T15:27:08Z" | Entry not found |
yiyic/t5_me5_base_mtg_es_5m_32_inverter | yiyic | "2024-01-02T15:27:52Z" | 0 | 0 | transformers | [
"transformers",
"safetensors",
"endpoints_compatible",
"region:us"
] | null | "2024-01-02T15:27:28Z" | Entry not found |
amoshughugface/my_awesome_billsum_model | amoshughugface | "2024-01-02T15:27:30Z" | 0 | 0 | null | [
"region:us"
] | null | "2024-01-02T15:27:30Z" | Entry not found |
amoshughugface/T5_text_sum | amoshughugface | "2024-01-02T15:28:42Z" | 0 | 0 | null | [
"region:us"
] | null | "2024-01-02T15:28:42Z" | Entry not found |
yiyic/t5_me5_base_mtg_fr_5m_32_inverter | yiyic | "2024-01-02T15:31:49Z" | 0 | 0 | transformers | [
"transformers",
"safetensors",
"endpoints_compatible",
"region:us"
] | null | "2024-01-02T15:31:18Z" | Entry not found |
yiyic/t5_me5_base_mtg_de_5m_32_inverter | yiyic | "2024-01-02T15:34:02Z" | 0 | 0 | transformers | [
"transformers",
"safetensors",
"endpoints_compatible",
"region:us"
] | null | "2024-01-02T15:33:37Z" | Entry not found |
Priyanshu007/local-lion | Priyanshu007 | "2024-01-02T15:37:34Z" | 0 | 0 | null | [
"safetensors",
"NxtWave-GenAI-Webinar",
"text-to-image",
"stable-diffusion",
"license:creativeml-openrail-m",
"region:us"
] | text-to-image | "2024-01-02T15:34:06Z" | ---
license: creativeml-openrail-m
tags:
- NxtWave-GenAI-Webinar
- text-to-image
- stable-diffusion
---
### local-lion Dreambooth model trained by Priyanshu007 following the "Build your own Gen AI model" session by NxtWave.
Project Submission Code: 23547
Sample pictures of this concept:
















|
ehsanhallo/results | ehsanhallo | "2024-07-03T11:41:45Z" | 0 | 0 | peft | [
"peft",
"tensorboard",
"safetensors",
"generated_from_trainer",
"base_model:togethercomputer/RedPajama-INCITE-Chat-3B-v1",
"base_model:adapter:togethercomputer/RedPajama-INCITE-Chat-3B-v1",
"license:apache-2.0",
"region:us"
] | null | "2024-01-02T15:40:12Z" | ---
license: apache-2.0
library_name: peft
tags:
- generated_from_trainer
base_model: togethercomputer/RedPajama-INCITE-Chat-3B-v1
model-index:
- name: results
results: []
---
<!-- This model card has been generated automatically according to the information the Trainer had access to. You
should probably proofread and complete it, then remove this comment. -->
# results
This model is a fine-tuned version of [togethercomputer/RedPajama-INCITE-Chat-3B-v1](https://huggingface.co/togethercomputer/RedPajama-INCITE-Chat-3B-v1) on an unknown dataset.
## Model description
More information needed
## Intended uses & limitations
More information needed
## Training and evaluation data
More information needed
## Training procedure
### Training hyperparameters
The following hyperparameters were used during training:
- learning_rate: 5e-05
- train_batch_size: 1
- eval_batch_size: 8
- seed: 42
- gradient_accumulation_steps: 4
- total_train_batch_size: 4
- optimizer: Adam with betas=(0.9,0.999) and epsilon=1e-08
- lr_scheduler_type: linear
- lr_scheduler_warmup_steps: 50
- training_steps: 250
- mixed_precision_training: Native AMP
### Training results
### Framework versions
- PEFT 0.11.1
- Transformers 4.41.2
- Pytorch 2.1.2
- Datasets 2.19.2
- Tokenizers 0.19.1 |
yiyic/t5_me5_base_mtg_de_5m_64_inverter | yiyic | "2024-01-02T15:41:38Z" | 0 | 0 | transformers | [
"transformers",
"safetensors",
"endpoints_compatible",
"region:us"
] | null | "2024-01-02T15:41:09Z" | Entry not found |
yiyic/t5_me5_base_mtg_fr_5m_64_inverter | yiyic | "2024-01-02T15:45:00Z" | 0 | 0 | transformers | [
"transformers",
"safetensors",
"endpoints_compatible",
"region:us"
] | null | "2024-01-02T15:44:29Z" | Entry not found |
yiyic/t5_me5_base_mtg_en_5m_64_inverter | yiyic | "2024-01-02T15:47:58Z" | 0 | 0 | transformers | [
"transformers",
"safetensors",
"endpoints_compatible",
"region:us"
] | null | "2024-01-02T15:47:28Z" | Entry not found |
vvrules00/falcon7binstruct_mentalhealthmodel_oct23 | vvrules00 | "2024-01-03T09:24:49Z" | 0 | 0 | peft | [
"peft",
"tensorboard",
"safetensors",
"trl",
"sft",
"generated_from_trainer",
"base_model:vilsonrodrigues/falcon-7b-instruct-sharded",
"base_model:adapter:vilsonrodrigues/falcon-7b-instruct-sharded",
"license:apache-2.0",
"region:us"
] | null | "2024-01-02T15:48:48Z" | ---
license: apache-2.0
library_name: peft
tags:
- trl
- sft
- generated_from_trainer
base_model: vilsonrodrigues/falcon-7b-instruct-sharded
model-index:
- name: falcon7binstruct_mentalhealthmodel_oct23
results: []
---
<!-- This model card has been generated automatically according to the information the Trainer had access to. You
should probably proofread and complete it, then remove this comment. -->
# falcon7binstruct_mentalhealthmodel_oct23
This model is a fine-tuned version of [vilsonrodrigues/falcon-7b-instruct-sharded](https://huggingface.co/vilsonrodrigues/falcon-7b-instruct-sharded) on an unknown dataset.
## Model description
More information needed
## Intended uses & limitations
More information needed
## Training and evaluation data
More information needed
## Training procedure
### Training hyperparameters
The following hyperparameters were used during training:
- learning_rate: 0.0002
- train_batch_size: 16
- eval_batch_size: 8
- seed: 42
- gradient_accumulation_steps: 4
- total_train_batch_size: 64
- optimizer: Adam with betas=(0.9,0.999) and epsilon=1e-08
- lr_scheduler_type: cosine
- lr_scheduler_warmup_ratio: 0.03
- training_steps: 180
- mixed_precision_training: Native AMP
### Training results
### Framework versions
- PEFT 0.7.2.dev0
- Transformers 4.36.2
- Pytorch 2.1.0+cu121
- Datasets 2.16.1
- Tokenizers 0.15.0 |
yiyic/t5_me5_base_mtg_es_5m_64_inverter | yiyic | "2024-01-02T15:50:13Z" | 0 | 0 | transformers | [
"transformers",
"safetensors",
"endpoints_compatible",
"region:us"
] | null | "2024-01-02T15:49:45Z" | Entry not found |
rbrgAlou/Reinforce-Pixelcopter-PLE-v0 | rbrgAlou | "2024-01-02T17:30:58Z" | 0 | 0 | null | [
"Pixelcopter-PLE-v0",
"reinforce",
"reinforcement-learning",
"custom-implementation",
"deep-rl-class",
"model-index",
"region:us"
] | reinforcement-learning | "2024-01-02T15:50:22Z" | ---
tags:
- Pixelcopter-PLE-v0
- reinforce
- reinforcement-learning
- custom-implementation
- deep-rl-class
model-index:
- name: Reinforce-Pixelcopter-PLE-v0
results:
- task:
type: reinforcement-learning
name: reinforcement-learning
dataset:
name: Pixelcopter-PLE-v0
type: Pixelcopter-PLE-v0
metrics:
- type: mean_reward
value: 38.70 +/- 26.18
name: mean_reward
verified: false
---
# **Reinforce** Agent playing **Pixelcopter-PLE-v0**
This is a trained model of a **Reinforce** agent playing **Pixelcopter-PLE-v0** .
To learn to use this model and train yours check Unit 4 of the Deep Reinforcement Learning Course: https://huggingface.co/deep-rl-course/unit4/introduction
|
maldred/q-FrozenLake-v1-4x4-noSlippery | maldred | "2024-01-02T15:50:28Z" | 0 | 0 | null | [
"FrozenLake-v1-4x4-no_slippery",
"q-learning",
"reinforcement-learning",
"custom-implementation",
"model-index",
"region:us"
] | reinforcement-learning | "2024-01-02T15:50:26Z" | ---
tags:
- FrozenLake-v1-4x4-no_slippery
- q-learning
- reinforcement-learning
- custom-implementation
model-index:
- name: q-FrozenLake-v1-4x4-noSlippery
results:
- task:
type: reinforcement-learning
name: reinforcement-learning
dataset:
name: FrozenLake-v1-4x4-no_slippery
type: FrozenLake-v1-4x4-no_slippery
metrics:
- type: mean_reward
value: 1.00 +/- 0.00
name: mean_reward
verified: false
---
# **Q-Learning** Agent playing1 **FrozenLake-v1**
This is a trained model of a **Q-Learning** agent playing **FrozenLake-v1** .
## Usage
```python
model = load_from_hub(repo_id="maldred/q-FrozenLake-v1-4x4-noSlippery", filename="q-learning.pkl")
# Don't forget to check if you need to add additional attributes (is_slippery=False etc)
env = gym.make(model["env_id"])
```
|