Daniel van Strien PRO
davanstrien
AI & ML interests
Machine Learning Librarian
Recent Activity
published
a dataset
about 2 hours ago
davanstrien/smol-hub-tldr-summaries-dpo-reviews
updated
a dataset
about 2 hours ago
data-is-better-together/fineweb-c-progress
updated
a dataset
about 3 hours ago
davanstrien/smol-hub-tldr-summaries-dpo-reviews
Organizations
davanstrien's activity
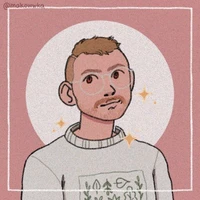
posted
an
update
1 day ago
Post
1306
Dataset descriptions for trending Hugging Face datasets? Powered by a Smol model
davanstrien/Smol-Hub-tldr
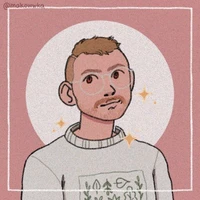
posted
an
update
3 days ago
Post
1690
How do you make 1M+ Hugging Face models & datasets more discoverable?
davanstrien/Smol-Hub-tldr!
I fine-tuned HuggingFaceTB/SmolLM2-360M to generate one-line summaries from a model or dataset README.
Its own self-description?
"A model for generating concise summaries of model & dataset cards from the Hugging Face Hub"
The goal? Make it easier to find the right models and datasets for your specific needs. It's already powering a semantic search for datasets Space.
It's still a WIP but thanks to @loubnabnl , @anton-l , @eliebak et al, for cooking such a nice base model for fine-tuning small, efficient models for specific domains and tasks. π
davanstrien/Smol-Hub-tldr!
I fine-tuned HuggingFaceTB/SmolLM2-360M to generate one-line summaries from a model or dataset README.
Its own self-description?
"A model for generating concise summaries of model & dataset cards from the Hugging Face Hub"
The goal? Make it easier to find the right models and datasets for your specific needs. It's already powering a semantic search for datasets Space.
It's still a WIP but thanks to @loubnabnl , @anton-l , @eliebak et al, for cooking such a nice base model for fine-tuning small, efficient models for specific domains and tasks. π
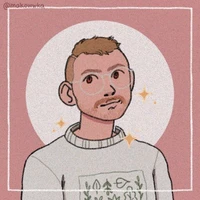
posted
an
update
4 days ago
Post
1223
Made some significant updates to my π€ semantic datasets search app. If you love falling into a wiki black hole, you might like this...
librarian-bots/huggingface-datasets-semantic-search
librarian-bots/huggingface-datasets-semantic-search
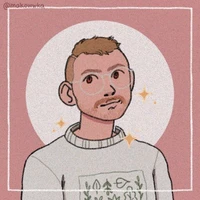
reacted to
Ihor's
post with π
17 days ago
Post
1414
π Reproducing DeepSeek R1 for Text-to-Graph Extraction
Iβve been working on replicating DeepSeek R1, focusing on zero-shot text-to-graph extractionβa challenging task where LMs extract entities and relations from text based on predefined types.
π§ Key Insight:
Language models struggle when constrained by entity/relation types. Supervised training alone isnβt enough, but reinforcement learning (RL), specifically Guided Reward Policy Optimization (GRPO), shows promise.
π‘ Why GRPO?
It trains the model to generate structured graphs, optimizing multiple reward functions (format, JSON validity, and extraction accuracy).
It allows the model to learn from both positive and hard negative examples dynamically.
RL can be fine-tuned to emphasize relation extraction improvements.
π Early Results:
Even with limited training, F1 scores consistently improved, and we saw clear benefits from RL-based optimization. More training = better performance!
π¬ Next Steps:
Weβre scaling up experiments with larger models and high-quality data. Stay tuned for updates! Meanwhile, check out one of our experimental models here:
Ihor/Text2Graph-R1-Qwen2.5-0.5b
π Learn more details from the blog post: https://medium.com/p/d8b648d9f419
Feel free to share your thoughts and ask questions!
Iβve been working on replicating DeepSeek R1, focusing on zero-shot text-to-graph extractionβa challenging task where LMs extract entities and relations from text based on predefined types.
π§ Key Insight:
Language models struggle when constrained by entity/relation types. Supervised training alone isnβt enough, but reinforcement learning (RL), specifically Guided Reward Policy Optimization (GRPO), shows promise.
π‘ Why GRPO?
It trains the model to generate structured graphs, optimizing multiple reward functions (format, JSON validity, and extraction accuracy).
It allows the model to learn from both positive and hard negative examples dynamically.
RL can be fine-tuned to emphasize relation extraction improvements.
π Early Results:
Even with limited training, F1 scores consistently improved, and we saw clear benefits from RL-based optimization. More training = better performance!
π¬ Next Steps:
Weβre scaling up experiments with larger models and high-quality data. Stay tuned for updates! Meanwhile, check out one of our experimental models here:
Ihor/Text2Graph-R1-Qwen2.5-0.5b
π Learn more details from the blog post: https://medium.com/p/d8b648d9f419
Feel free to share your thoughts and ask questions!
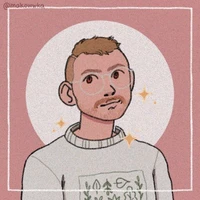
posted
an
update
19 days ago
Post
1805
Why choose between strong LLM reasoning and efficient models?
Use DeepSeek to generate high-quality training data, then distil that knowledge into ModernBERT answerdotai/ModernBERT-base for fast, efficient classification.
Blog post: https://danielvanstrien.xyz/posts/2025/deepseek/distil-deepseek-modernbert.html
Use DeepSeek to generate high-quality training data, then distil that knowledge into ModernBERT answerdotai/ModernBERT-base for fast, efficient classification.
Blog post: https://danielvanstrien.xyz/posts/2025/deepseek/distil-deepseek-modernbert.html
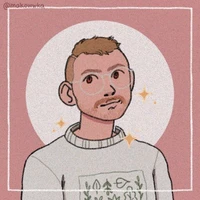
posted
an
update
20 days ago
Post
1886
Updated the ColPali Query Generator Space
davanstrien/ColPali-Query-Generator to use
Qwen/Qwen2.5-VL-7B-Instruct.
Given an input image, it generates several queries along with explanations to justify them. This approach can generate synthetic data for fine-tuning ColPali models.
Given an input image, it generates several queries along with explanations to justify them. This approach can generate synthetic data for fine-tuning ColPali models.
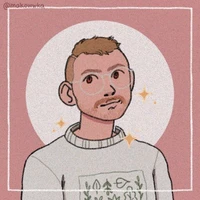
reacted to
fdaudens's
post with β€οΈ
21 days ago
Post
8449
Yes, DeepSeek R1's release is impressive. But the real story is what happened in just 7 days after:
- Original release: 8 models, 540K downloads. Just the beginning...
- The community turned those open-weight models into +550 NEW models on Hugging Face. Total downloads? 2.5Mβnearly 5X the originals.
The reason? DeepSeek models are open-weight, letting anyone build on top of them. Interesting to note that the community focused on quantized versions for better efficiency & accessibility. They want models that use less memory, run faster, and are more energy-efficient.
When you empower builders, innovation explodes. For everyone. π
The most popular community model? @bartowski 's DeepSeek-R1-Distill-Qwen-32B-GGUF version β 1M downloads alone.
- Original release: 8 models, 540K downloads. Just the beginning...
- The community turned those open-weight models into +550 NEW models on Hugging Face. Total downloads? 2.5Mβnearly 5X the originals.
The reason? DeepSeek models are open-weight, letting anyone build on top of them. Interesting to note that the community focused on quantized versions for better efficiency & accessibility. They want models that use less memory, run faster, and are more energy-efficient.
When you empower builders, innovation explodes. For everyone. π
The most popular community model? @bartowski 's DeepSeek-R1-Distill-Qwen-32B-GGUF version β 1M downloads alone.
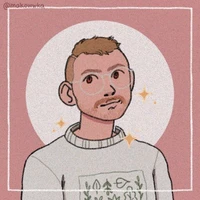
posted
an
update
21 days ago
Post
2017
π Big step for multilingual AI data!
The Hugging Face community has rated educational content in languages spoken by 1.6 billion people! New additions:
β’ Japanese
β’ Italian
β’ Old High German
Learn more and contribute: https://huggingface.co/blog/davanstrien/fineweb2-community
These ratings can help enhance training data for major world languages.
The Hugging Face community has rated educational content in languages spoken by 1.6 billion people! New additions:
β’ Japanese
β’ Italian
β’ Old High German
Learn more and contribute: https://huggingface.co/blog/davanstrien/fineweb2-community
These ratings can help enhance training data for major world languages.
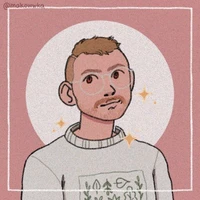
reacted to
tomaarsen's
post with π₯β€οΈ
about 1 month ago
Post
4602
ποΈ Today I'm introducing a method to train static embedding models that run 100x to 400x faster on CPU than common embedding models, while retaining 85%+ of the quality! Including 2 fully open models: training scripts, datasets, metrics.
We apply our recipe to train 2 Static Embedding models that we release today! We release:
2οΈβ£ an English Retrieval model and a general-purpose Multilingual similarity model (e.g. classification, clustering, etc.), both Apache 2.0
π§ my modern training strategy: ideation -> dataset choice -> implementation -> evaluation
π my training scripts, using the Sentence Transformers library
π my Weights & Biases reports with losses & metrics
π my list of 30 training and 13 evaluation datasets
The 2 Static Embedding models have the following properties:
ποΈ Extremely fast, e.g. 107500 sentences per second on a consumer CPU, compared to 270 for 'all-mpnet-base-v2' and 56 for 'gte-large-en-v1.5'
0οΈβ£ Zero active parameters: No Transformer blocks, no attention, not even a matrix multiplication. Super speed!
π No maximum sequence length! Embed texts at any length (note: longer texts may embed worse)
π Linear instead of exponential complexity: 2x longer text takes 2x longer, instead of 2.5x or more.
πͺ Matryoshka support: allow you to truncate embeddings with minimal performance loss (e.g. 4x smaller with a 0.56% perf. decrease for English Similarity tasks)
Check out the full blogpost if you'd like to 1) use these lightning-fast models or 2) learn how to train them with consumer-level hardware: https://huggingface.co/blog/static-embeddings
The blogpost contains a lengthy list of possible advancements; I'm very confident that our 2 models are only the tip of the iceberg, and we may be able to get even better performance.
Alternatively, check out the models:
* sentence-transformers/static-retrieval-mrl-en-v1
* sentence-transformers/static-similarity-mrl-multilingual-v1
We apply our recipe to train 2 Static Embedding models that we release today! We release:
2οΈβ£ an English Retrieval model and a general-purpose Multilingual similarity model (e.g. classification, clustering, etc.), both Apache 2.0
π§ my modern training strategy: ideation -> dataset choice -> implementation -> evaluation
π my training scripts, using the Sentence Transformers library
π my Weights & Biases reports with losses & metrics
π my list of 30 training and 13 evaluation datasets
The 2 Static Embedding models have the following properties:
ποΈ Extremely fast, e.g. 107500 sentences per second on a consumer CPU, compared to 270 for 'all-mpnet-base-v2' and 56 for 'gte-large-en-v1.5'
0οΈβ£ Zero active parameters: No Transformer blocks, no attention, not even a matrix multiplication. Super speed!
π No maximum sequence length! Embed texts at any length (note: longer texts may embed worse)
π Linear instead of exponential complexity: 2x longer text takes 2x longer, instead of 2.5x or more.
πͺ Matryoshka support: allow you to truncate embeddings with minimal performance loss (e.g. 4x smaller with a 0.56% perf. decrease for English Similarity tasks)
Check out the full blogpost if you'd like to 1) use these lightning-fast models or 2) learn how to train them with consumer-level hardware: https://huggingface.co/blog/static-embeddings
The blogpost contains a lengthy list of possible advancements; I'm very confident that our 2 models are only the tip of the iceberg, and we may be able to get even better performance.
Alternatively, check out the models:
* sentence-transformers/static-retrieval-mrl-en-v1
* sentence-transformers/static-similarity-mrl-multilingual-v1
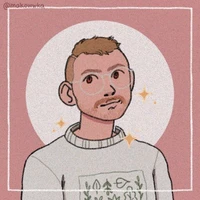
reacted to
AdinaY's
post with π₯
about 1 month ago
Post
3113
MiniMax, the company behind Hailuo_AI, has joined the open source community by releasing both models and demos of MiniMax-Text-01 & MiniMax-VL-01π₯
- Model
MiniMaxAI/MiniMax-VL-01
MiniMaxAI/MiniMax-Text-01
- Demo
MiniMaxAI/MiniMax-VL-01
MiniMaxAI/MiniMax-Text-01
β¨ MiniMax-text-01:
- 456B with 45.9B activated per token
- Combines Lightning Attention, Softmax Attention, and MoE for optimal performance
- Training context up to 1M tokens, inference handles 4M tokens
β¨ MiniMax-VL-01:
- ViT-MLP-LLM framework ( non-transformerπ)
- Handles image inputs from 336Γ336 to 2016Γ2016
- 694M image-caption pairs + 512B tokens processed across 4 stages
- Model
MiniMaxAI/MiniMax-VL-01
MiniMaxAI/MiniMax-Text-01
- Demo
MiniMaxAI/MiniMax-VL-01
MiniMaxAI/MiniMax-Text-01
β¨ MiniMax-text-01:
- 456B with 45.9B activated per token
- Combines Lightning Attention, Softmax Attention, and MoE for optimal performance
- Training context up to 1M tokens, inference handles 4M tokens
β¨ MiniMax-VL-01:
- ViT-MLP-LLM framework ( non-transformerπ)
- Handles image inputs from 336Γ336 to 2016Γ2016
- 694M image-caption pairs + 512B tokens processed across 4 stages
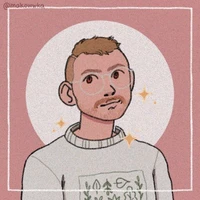
reacted to
AdinaY's
post with π₯
about 1 month ago
Post
3188
MiniCPM-o2.6 π₯ an end-side multimodal LLMs released by OpenBMB from the Chinese community
Model: openbmb/MiniCPM-o-2_6
β¨ Real-time English/Chinese conversation, emotion control and ASR/STT
β¨ Real-time video/audio understanding
β¨ Processes up to 1.8M pixels, leads OCRBench & supports 30+ languages
Model: openbmb/MiniCPM-o-2_6
β¨ Real-time English/Chinese conversation, emotion control and ASR/STT
β¨ Real-time video/audio understanding
β¨ Processes up to 1.8M pixels, leads OCRBench & supports 30+ languages
Post
3066
Introducing scandi-fine-web-cleaner
davanstrien/scandi-fine-web-cleaner, the first model trained on FineWeb-C community annotations!
FineWeb2 is a massive multilingual dataset for pre-training language models. Like any web-scale dataset, it contains low-quality content. How can we improve it?
Over the past months, an amazing community of 400+ annotators has been labelling content quality (using Argilla) across 23 languages through the FineWeb-C initiative.
Today, I'm happy to share the first classifier trained on this data.
π What we've built:
- A lightweight classifier that efficiently removes low-quality content
- 90%+ precision demonstrated on Danish & Swedish
- Can process the 43M+ documents in Danish FineWeb2 with minimal compute
π Why this matters: The approach can be reproduced for any of the 23 languages in FineWeb-C ( data-is-better-together/fineweb-c). We can improve training data quality at scale without massive compute resources by starting with community annotations and training small, efficient classifiers.
Want to build a classifier for your language? Check out the full blog post with code examples and implementation details: https://danielvanstrien.xyz/posts/2025/FineWeb-c/scandinavian-content-filtering-fineweb.html
FineWeb2 is a massive multilingual dataset for pre-training language models. Like any web-scale dataset, it contains low-quality content. How can we improve it?
Over the past months, an amazing community of 400+ annotators has been labelling content quality (using Argilla) across 23 languages through the FineWeb-C initiative.
Today, I'm happy to share the first classifier trained on this data.
π What we've built:
- A lightweight classifier that efficiently removes low-quality content
- 90%+ precision demonstrated on Danish & Swedish
- Can process the 43M+ documents in Danish FineWeb2 with minimal compute
π Why this matters: The approach can be reproduced for any of the 23 languages in FineWeb-C ( data-is-better-together/fineweb-c). We can improve training data quality at scale without massive compute resources by starting with community annotations and training small, efficient classifiers.
Want to build a classifier for your language? Check out the full blog post with code examples and implementation details: https://danielvanstrien.xyz/posts/2025/FineWeb-c/scandinavian-content-filtering-fineweb.html
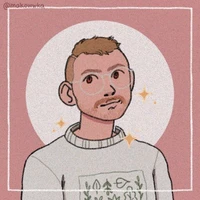
replied to
their
post
about 1 month ago
Model wouldn't be possible without @Lauler @AntonVic @ohallstrom @bjarlestam @menbom @Ekgren @apsod for Swedish and @rasgaard @JakobBlaa @saattrupdan @FrLars21 @markhougaard @KennethEnevoldsen @Apasalic @tqvist @cnila @Soeren-B @KristianL @mathiasn1 @ITK-dev @jannikskytt @AndreasLH @perlausten @sorenmulli @organicoder for Danish!
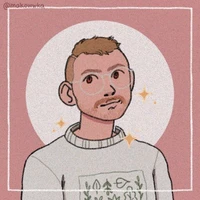
posted
an
update
about 1 month ago
Post
3066
Introducing scandi-fine-web-cleaner
davanstrien/scandi-fine-web-cleaner, the first model trained on FineWeb-C community annotations!
FineWeb2 is a massive multilingual dataset for pre-training language models. Like any web-scale dataset, it contains low-quality content. How can we improve it?
Over the past months, an amazing community of 400+ annotators has been labelling content quality (using Argilla) across 23 languages through the FineWeb-C initiative.
Today, I'm happy to share the first classifier trained on this data.
π What we've built:
- A lightweight classifier that efficiently removes low-quality content
- 90%+ precision demonstrated on Danish & Swedish
- Can process the 43M+ documents in Danish FineWeb2 with minimal compute
π Why this matters: The approach can be reproduced for any of the 23 languages in FineWeb-C ( data-is-better-together/fineweb-c). We can improve training data quality at scale without massive compute resources by starting with community annotations and training small, efficient classifiers.
Want to build a classifier for your language? Check out the full blog post with code examples and implementation details: https://danielvanstrien.xyz/posts/2025/FineWeb-c/scandinavian-content-filtering-fineweb.html
FineWeb2 is a massive multilingual dataset for pre-training language models. Like any web-scale dataset, it contains low-quality content. How can we improve it?
Over the past months, an amazing community of 400+ annotators has been labelling content quality (using Argilla) across 23 languages through the FineWeb-C initiative.
Today, I'm happy to share the first classifier trained on this data.
π What we've built:
- A lightweight classifier that efficiently removes low-quality content
- 90%+ precision demonstrated on Danish & Swedish
- Can process the 43M+ documents in Danish FineWeb2 with minimal compute
π Why this matters: The approach can be reproduced for any of the 23 languages in FineWeb-C ( data-is-better-together/fineweb-c). We can improve training data quality at scale without massive compute resources by starting with community annotations and training small, efficient classifiers.
Want to build a classifier for your language? Check out the full blog post with code examples and implementation details: https://danielvanstrien.xyz/posts/2025/FineWeb-c/scandinavian-content-filtering-fineweb.html
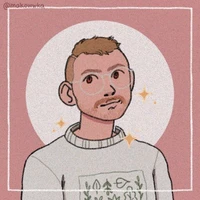
posted
an
update
about 1 month ago
Post
2251
The
data-is-better-together/fineweb-c dataset is growing!
This week a few more languages have got 1,000 annotations for the educational quality of data from HuggingFaceFW/fineweb-2.
Why should you care?
The quality of pre-training data can have a big impact on the performance of downstream language models trained on that data ( HuggingFaceFW/blogpost-fineweb-v1).
Being able to filter by educational quality is on way of improving the quality of the data you use for training an LLM. Very importantly this approach can also reduce the amount of data needed for pertaining.
Why not use an LLM?
LLMs can be used to annotate educational quality for a subset of data. This data can then be used to train a smaller encoder only model to label the full dataset. However, this may not work well for languages outside of english. This is where fineweb-c (community) comes in.
The community is annotating the educational quality of fineweb2 data. Currently 114 languages have some annotations. These annotations will enable a number of things:
- Evaluate whether an LLM can label the educational quality for texts in that language well
- Directly be used for training quality classifiers
- Help discover other rules and huerisitcs for refining fineweb2 further for different languages.
This week the following languages where done:
Swedish thanks to: @Lauler @AntonVic @ohallstrom @bjarlestam @menbom @Ekgren @apsod
Ukrainian thanks to: @hannayukhymenko @robinhad @realPivo @RabotiahovDmytro @reciprocate
Assamese thanks to: @moyoor97 @Arpanjyoti @nawaf-helmi123 @pahigogoi1 @aelhence @kishorekashyap
Want to learn more: https://huggingface.co/blog/davanstrien/fineweb2-community
Contribute yourself here: data-is-better-together/fineweb-c
This week a few more languages have got 1,000 annotations for the educational quality of data from HuggingFaceFW/fineweb-2.
Why should you care?
The quality of pre-training data can have a big impact on the performance of downstream language models trained on that data ( HuggingFaceFW/blogpost-fineweb-v1).
Being able to filter by educational quality is on way of improving the quality of the data you use for training an LLM. Very importantly this approach can also reduce the amount of data needed for pertaining.
Why not use an LLM?
LLMs can be used to annotate educational quality for a subset of data. This data can then be used to train a smaller encoder only model to label the full dataset. However, this may not work well for languages outside of english. This is where fineweb-c (community) comes in.
The community is annotating the educational quality of fineweb2 data. Currently 114 languages have some annotations. These annotations will enable a number of things:
- Evaluate whether an LLM can label the educational quality for texts in that language well
- Directly be used for training quality classifiers
- Help discover other rules and huerisitcs for refining fineweb2 further for different languages.
This week the following languages where done:
Swedish thanks to: @Lauler @AntonVic @ohallstrom @bjarlestam @menbom @Ekgren @apsod
Ukrainian thanks to: @hannayukhymenko @robinhad @realPivo @RabotiahovDmytro @reciprocate
Assamese thanks to: @moyoor97 @Arpanjyoti @nawaf-helmi123 @pahigogoi1 @aelhence @kishorekashyap
Want to learn more: https://huggingface.co/blog/davanstrien/fineweb2-community
Contribute yourself here: data-is-better-together/fineweb-c
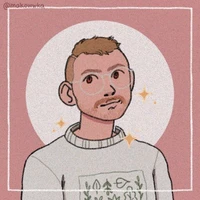
reacted to
albertvillanova's
post with π
about 1 month ago
Post
2065
Discover all the improvements in the new version of Lighteval: https://huggingface.co/docs/lighteval/
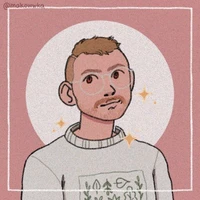
replied to
their
post
about 2 months ago
There are some already in the Argilla instance!
You can also join the discussions here: https://huggingface.co/spaces/HuggingFaceFW/discussion :)
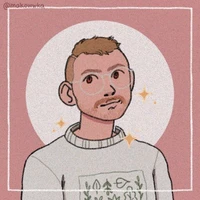
replied to
their
post
about 2 months ago
Thanks to the hard work of @ivykopal , the first 1,000 annotations for Slovak have been completed! Make sure to give Ivan a follow :)
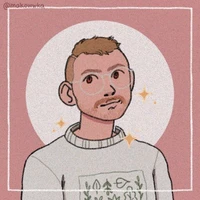
reacted to
nicolay-r's
post with β€οΈ
about 2 months ago
Post
2136
π’ Deligted to share the most recent milestone on quick deployment of Named Entity Recognition (NER) in Gen-AI powered systems.
Releasing the bulk-ner 0.25.0 which represent a tiny framework that would save you time for deploing NER with any model.
π Why is this important? In the era of GenAI the handling out textual output might be challenging. Instead, recognizing named-entities via domain-oriented systems for your donwstream LLM would be preferable option.
π¦: https://pypi.org/project/bulk-ner/0.25.0/
π: https://github.com/nicolay-r/bulk-ner
I noticed that the direct adaptaion of the LM for NER would result in spending signifcant amount of time on formatting your texts according to the NER-model needs.
In particular:
1. Processing CONLL format with B-I-O tags from model outputs
2. Input trimming: long input content might not be completely fitted
To cope with these problems, in version 0.25.0 I made a huge steps forward by providing:
β π Python API support: see screenshot below for a quick deployment (see screenshot below πΈ)
β πͺΆ No-string: dependencies are now clear, so it is purely Python implementation for API calls.
β π Simplified output formatting: we use lists to represent texts with inner lists that refer to annotated objects (see screenshot below πΈ)
π We have a colab for a quick start here (or screenshot for bash / Python API πΈ)
https://colab.research.google.com/github/nicolay-r/ner-service/blob/main/NER_annotation_service.ipynb
π The code for pipeline deployment is taken from the AREkit project:
https://github.com/nicolay-r/AREkit
Releasing the bulk-ner 0.25.0 which represent a tiny framework that would save you time for deploing NER with any model.
π Why is this important? In the era of GenAI the handling out textual output might be challenging. Instead, recognizing named-entities via domain-oriented systems for your donwstream LLM would be preferable option.
π¦: https://pypi.org/project/bulk-ner/0.25.0/
π: https://github.com/nicolay-r/bulk-ner
I noticed that the direct adaptaion of the LM for NER would result in spending signifcant amount of time on formatting your texts according to the NER-model needs.
In particular:
1. Processing CONLL format with B-I-O tags from model outputs
2. Input trimming: long input content might not be completely fitted
To cope with these problems, in version 0.25.0 I made a huge steps forward by providing:
β π Python API support: see screenshot below for a quick deployment (see screenshot below πΈ)
β πͺΆ No-string: dependencies are now clear, so it is purely Python implementation for API calls.
β π Simplified output formatting: we use lists to represent texts with inner lists that refer to annotated objects (see screenshot below πΈ)
π We have a colab for a quick start here (or screenshot for bash / Python API πΈ)
https://colab.research.google.com/github/nicolay-r/ner-service/blob/main/NER_annotation_service.ipynb
π The code for pipeline deployment is taken from the AREkit project:
https://github.com/nicolay-r/AREkit