Model Card for udever-bloom
udever-bloom-1b1
is finetuned from bigscience/bloom-1b1 via BitFit on MS MARCO Passage Ranking, SNLI and MultiNLI data.
It is a universal embedding model across tasks, natural and programming languages.
(From the technical view, udever
is merely with some minor improvements to sgpt-bloom
)
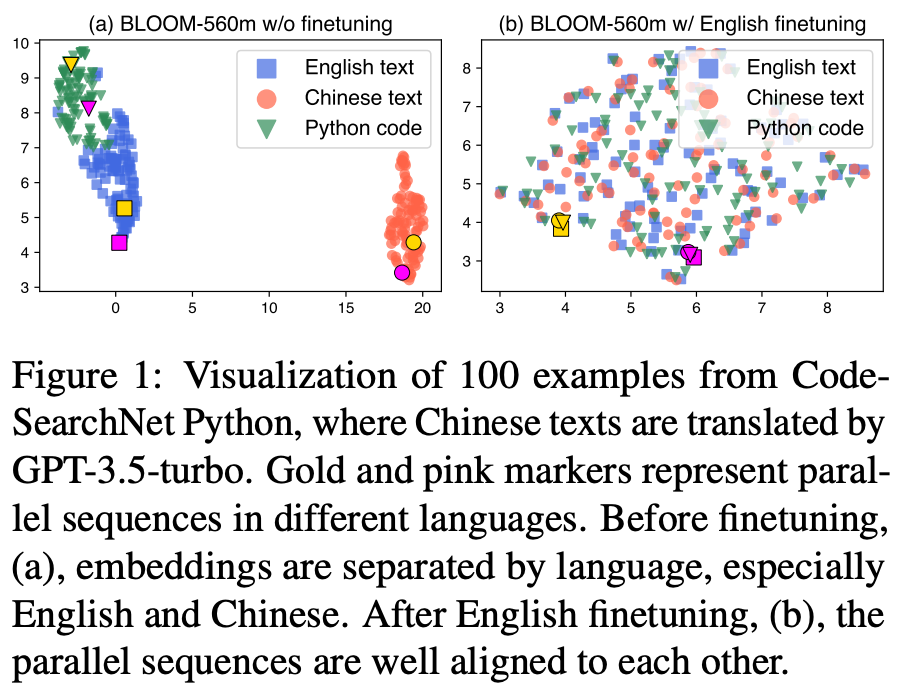
Model Details
Model Description
- Developed by: Alibaba Group
- Model type: Transformer-based Language Model (decoder-only)
- Language(s) (NLP): Multiple; see bloom training data
- Finetuned from model : bigscience/bloom-1b1
Model Sources
- Repository: github.com/izhx/uni-rep
- Paper : Language Models are Universal Embedders
- Training Date : 2023-06
How to Get Started with the Model
Use the code below to get started with the model.
import torch
from transformers import AutoTokenizer, BloomModel
tokenizer = AutoTokenizer.from_pretrained('izhx/udever-bloom-1b1')
model = BloomModel.from_pretrained('izhx/udever-bloom-1b1')
boq, eoq, bod, eod = '[BOQ]', '[EOQ]', '[BOD]', '[EOD]'
eoq_id, eod_id = tokenizer.convert_tokens_to_ids([eoq, eod])
if tokenizer.padding_side != 'left':
print('!!!', tokenizer.padding_side)
tokenizer.padding_side = 'left'
def encode(texts: list, is_query: bool = True, max_length=300):
bos = boq if is_query else bod
eos_id = eoq_id if is_query else eod_id
texts = [bos + t for t in texts]
encoding = tokenizer(
texts, truncation=True, max_length=max_length - 1, padding=True
)
for ids, mask in zip(encoding['input_ids'], encoding['attention_mask']):
ids.append(eos_id)
mask.append(1)
inputs = tokenizer.pad(encoding, return_tensors='pt')
with torch.inference_mode():
outputs = model(**inputs)
embeds = outputs.last_hidden_state[:, -1]
return embeds
encode(['I am Bert', 'You are Elmo'])
Training Details
Training Data
- MS MARCO Passage Ranking, retrieved by (https://github.com/UKPLab/sentence-transformers/blob/master/examples/training/ms_marco/train_bi-encoder_mnrl.py#L86)
- SNLI and MultiNLI (https://sbert.net/datasets/AllNLI.tsv.gz)
Training Procedure
Preprocessing
MS MARCO hard negatives provided by (https://github.com/UKPLab/sentence-transformers/blob/master/examples/training/ms_marco/train_bi-encoder_mnrl.py#L86). Negatives for SNLI and MultiNLI are randomly sampled.
Training Hyperparameters
- Training regime: tf32, BitFit
- Batch size: 1024
- Epochs: 3
- Optimizer: AdamW
- Learning rate: 1e-4
- Scheduler: constant with warmup.
- Warmup: 0.25 epoch
Evaluation
Table 1: Massive Text Embedding Benchmark MTEB
MTEB | Avg. | Class. | Clust. | PairClass. | Rerank. | Retr. | STS | Summ. |
---|---|---|---|---|---|---|---|---|
#Datasets ➡️ | 56 | 12 | 11 | 3 | 4 | 15 | 10 | 1 |
bge-large-en-v1.5 | 64.23 | 75.97 | 46.08 | 87.12 | 60.03 | 54.29 | 83.11 | 31.61 |
bge-base-en-v1.5 | 63.55 | 75.53 | 45.77 | 86.55 | 58.86 | 53.25 | 82.4 | 31.07 |
gte-large | 63.13 | 73.33 | 46.84 | 85 | 59.13 | 52.22 | 83.35 | 31.66 |
gte-base | 62.39 | 73.01 | 46.2 | 84.57 | 58.61 | 51.14 | 82.3 | 31.17 |
e5-large-v2 | 62.25 | 75.24 | 44.49 | 86.03 | 56.61 | 50.56 | 82.05 | 30.19 |
instructor-xl | 61.79 | 73.12 | 44.74 | 86.62 | 57.29 | 49.26 | 83.06 | 32.32 |
instructor-large | 61.59 | 73.86 | 45.29 | 85.89 | 57.54 | 47.57 | 83.15 | 31.84 |
e5-base-v2 | 61.5 | 73.84 | 43.8 | 85.73 | 55.91 | 50.29 | 81.05 | 30.28 |
e5-large | 61.42 | 73.14 | 43.33 | 85.94 | 56.53 | 49.99 | 82.06 | 30.97 |
text-embedding-ada-002 (OpenAI API) | 60.99 | 70.93 | 45.9 | 84.89 | 56.32 | 49.25 | 80.97 | 30.8 |
e5-base | 60.44 | 72.63 | 42.11 | 85.09 | 55.7 | 48.75 | 80.96 | 31.01 |
SGPT-5.8B-msmarco | 58.93 | 68.13 | 40.34 | 82 | 56.56 | 50.25 | 78.1 | 31.46 |
sgpt-bloom-7b1-msmarco | 57.59 | 66.19 | 38.93 | 81.9 | 55.65 | 48.22 | 77.74 | 33.6 |
Udever-bloom-560m | 55.80 | 68.04 | 36.89 | 81.05 | 52.60 | 41.19 | 79.93 | 32.06 |
Udever-bloom-1b1 | 58.28 | 70.18 | 39.11 | 83.11 | 54.28 | 45.27 | 81.52 | 31.10 |
Udever-bloom-3b | 59.86 | 71.91 | 40.74 | 84.06 | 54.90 | 47.67 | 82.37 | 30.62 |
Udever-bloom-7b1 | 60.63 | 72.13 | 40.81 | 85.40 | 55.91 | 49.34 | 83.01 | 30.97 |
Table 2: CodeSearchNet
CodeSearchNet | Go | Ruby | Python | Java | JS | PHP | Avg. |
---|---|---|---|---|---|---|---|
CodeBERT | 69.3 | 70.6 | 84.0 | 86.8 | 74.8 | 70.6 | 76.0 |
GraphCodeBERT | 84.1 | 73.2 | 87.9 | 75.7 | 71.1 | 72.5 | 77.4 |
cpt-code S | 97.7 | 86.3 | 99.8 | 94.0 | 86.0 | 96.7 | 93.4 |
cpt-code M | 97.5 | 85.5 | 99.9 | 94.4 | 86.5 | 97.2 | 93.5 |
sgpt-bloom-7b1-msmarco | 76.79 | 69.25 | 95.68 | 77.93 | 70.35 | 73.45 | 77.24 |
Udever-bloom-560m | 75.38 | 66.67 | 96.23 | 78.99 | 69.39 | 73.69 | 76.73 |
Udever-bloom-1b1 | 78.76 | 72.85 | 97.67 | 82.77 | 74.38 | 78.97 | 80.90 |
Udever-bloom-3b | 80.63 | 75.40 | 98.02 | 83.88 | 76.18 | 79.67 | 82.29 |
Udever-bloom-7b1 | 79.37 | 76.59 | 98.38 | 84.68 | 77.49 | 80.03 | 82.76 |
Table 3: Chinese multi-domain retrieval Multi-cpr
E-commerce | Entertainment video | Medical | ||||||
---|---|---|---|---|---|---|---|---|
Model | Train | Backbone | MRR@10 | Recall@1k | MRR@10 | Recall@1k | MRR@10 | Recall@1k |
BM25 | - | - | 0.225 | 0.815 | 0.225 | 0.780 | 0.187 | 0.482 |
Doc2Query | - | - | 0.239 | 0.826 | 0.238 | 0.794 | 0.210 | 0.505 |
DPR-1 | In-Domain | BERT | 0.270 | 0.921 | 0.254 | 0.934 | 0.327 | 0.747 |
DPR-2 | In-Domain | BERT-CT | 0.289 | 0.926 | 0.263 | 0.935 | 0.339 | 0.769 |
text-embedding-ada-002 | General | GPT | 0.183 | 0.825 | 0.159 | 0.786 | 0.245 | 0.593 |
sgpt-bloom-7b1-msmarco | General | BLOOM | 0.242 | 0.840 | 0.227 | 0.829 | 0.311 | 0.675 |
Udever-bloom-560m | General | BLOOM | 0.156 | 0.802 | 0.149 | 0.749 | 0.245 | 0.571 |
Udever-bloom-1b1 | General | BLOOM | 0.244 | 0.863 | 0.208 | 0.815 | 0.241 | 0.557 |
Udever-bloom-3b | General | BLOOM | 0.267 | 0.871 | 0.228 | 0.836 | 0.288 | 0.619 |
Udever-bloom-7b1 | General | BLOOM | 0.296 | 0.889 | 0.267 | 0.907 | 0.343 | 0.705 |
More results refer to paper section 3.
Technical Specifications
Model Architecture and Objective
- Model: bigscience/bloom-1b1.
- Objective: Constrastive loss with hard negatives (refer to paper section 2.2).
Compute Infrastructure
- Nvidia A100 SXM4 80GB.
- torch 2.0.0, transformers 4.29.2.
Citation
BibTeX:
@article{zhang2023language,
title={Language Models are Universal Embedders},
author={Zhang, Xin and Li, Zehan and Zhang, Yanzhao and Long, Dingkun and Xie, Pengjun and Zhang, Meishan and Zhang, Min},
journal={arXiv preprint arXiv:2310.08232},
year={2023}
}
- Downloads last month
- 7,165
Spaces using izhx/udever-bloom-1b1 3
Evaluation results
- cos_sim_pearson on MTEB AFQMCvalidation set self-reported27.900
- cos_sim_spearman on MTEB AFQMCvalidation set self-reported27.981
- euclidean_pearson on MTEB AFQMCvalidation set self-reported27.412
- euclidean_spearman on MTEB AFQMCvalidation set self-reported27.608
- manhattan_pearson on MTEB AFQMCvalidation set self-reported27.494
- manhattan_spearman on MTEB AFQMCvalidation set self-reported27.715
- cos_sim_pearson on MTEB ATECtest set self-reported35.153
- cos_sim_spearman on MTEB ATECtest set self-reported35.864
- euclidean_pearson on MTEB ATECtest set self-reported37.657
- euclidean_spearman on MTEB ATECtest set self-reported35.501