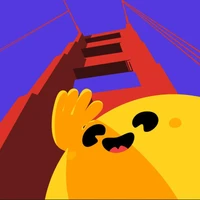
Open-Source AI Meetup
community
AI & ML interests
Open science and open source
Recent Activity
View all activity
SFEvent's activity
Post
425
🌐 The Stanford Institute for Human-Centered AI (https://aiindex.stanford.edu/vibrancy/) has released its 2024 Global AI Vibrancy Tool, a way to explore and compare AI progress across 36 countries.
📊 It measures progress across the 8 broad pillars of R&D, Responsible AI, Economy, Education, Diversity, Policy and Governance, Public Opinion and Infrastructure. (Each of these pillars have a number of Sub Indices)
📈 As a whole it is not surprising that the USA was at the top in terms of overall score as of 2023 (AI investment activity is a large part of the economic pillar for example and that is a large part of the overall USA ranking) but drilling in to more STRATEGIC Macro pillars like Education, Infrastructure or R&D reveal interesting growth patterns in Asia (particularly China) and Western Europe that I suspect the 2024 metrics will bear out.
🤖 Hopefully the 2024 Global Vibrancy ranking will break out AI and ML verticals like Computer Vision or NLP and or the AI Agent space as that may also from a global macro level give indications of what is to come globally for AI in 2025.
📊 It measures progress across the 8 broad pillars of R&D, Responsible AI, Economy, Education, Diversity, Policy and Governance, Public Opinion and Infrastructure. (Each of these pillars have a number of Sub Indices)
📈 As a whole it is not surprising that the USA was at the top in terms of overall score as of 2023 (AI investment activity is a large part of the economic pillar for example and that is a large part of the overall USA ranking) but drilling in to more STRATEGIC Macro pillars like Education, Infrastructure or R&D reveal interesting growth patterns in Asia (particularly China) and Western Europe that I suspect the 2024 metrics will bear out.
🤖 Hopefully the 2024 Global Vibrancy ranking will break out AI and ML verticals like Computer Vision or NLP and or the AI Agent space as that may also from a global macro level give indications of what is to come globally for AI in 2025.
Post
688
🤖💻 Function Calling is a key component of Agent workflows. To call functions, an LLM needs a way to interact with other systems and run code. This usually means connecting it to a runtime environment that can handle function calls, data, and security.
Per the Berkeley Function-Calling Leaderboard there are only 2 fully open source models (The other 2 in the top 20 that are not closed source have cc-by-nc-4.0 licenses) out of the top 20 models that currently have function calling built in as of 17 Nov 2024.
https://gorilla.cs.berkeley.edu/leaderboard.html
The 2 Open Source Models out of the top 20 that currently support function calling are:
meetkai/functionary-medium-v3.1
Team-ACE/ToolACE-8B
This is a both a huge disadvantage AND an opportunity for the Open Source community as Enterprises, Small Business, Government Agencies etc. quickly adopt Agents and Agent workflows over the next few months. Open Source will have a lot of catching up to do as Enterprises will be hesitant to switch from the closed source models that they may initially build their Agent workflows on in the next few months to an open source alternative later.
Hopefully more open source models will support function calling in the near future.
Per the Berkeley Function-Calling Leaderboard there are only 2 fully open source models (The other 2 in the top 20 that are not closed source have cc-by-nc-4.0 licenses) out of the top 20 models that currently have function calling built in as of 17 Nov 2024.
https://gorilla.cs.berkeley.edu/leaderboard.html
The 2 Open Source Models out of the top 20 that currently support function calling are:
meetkai/functionary-medium-v3.1
Team-ACE/ToolACE-8B
This is a both a huge disadvantage AND an opportunity for the Open Source community as Enterprises, Small Business, Government Agencies etc. quickly adopt Agents and Agent workflows over the next few months. Open Source will have a lot of catching up to do as Enterprises will be hesitant to switch from the closed source models that they may initially build their Agent workflows on in the next few months to an open source alternative later.
Hopefully more open source models will support function calling in the near future.
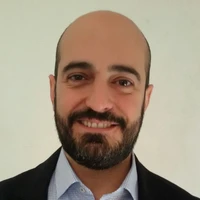
albertvillanova
posted
an
update
about 1 month ago
Post
1364
🚨 How green is your model? 🌱 Introducing a new feature in the Comparator tool: Environmental Impact for responsible #LLM research!
👉 open-llm-leaderboard/comparator
Now, you can not only compare models by performance, but also by their environmental footprint!
🌍 The Comparator calculates CO₂ emissions during evaluation and shows key model characteristics: evaluation score, number of parameters, architecture, precision, type... 🛠️
Make informed decisions about your model's impact on the planet and join the movement towards greener AI!
👉 open-llm-leaderboard/comparator
Now, you can not only compare models by performance, but also by their environmental footprint!
🌍 The Comparator calculates CO₂ emissions during evaluation and shows key model characteristics: evaluation score, number of parameters, architecture, precision, type... 🛠️
Make informed decisions about your model's impact on the planet and join the movement towards greener AI!
Post
1778
ever wondered how you can make an API call to a visual-question-answering model without sending an image url 👀
you can do that by converting your local image to base64 and sending it to the API.
recently I made some changes to my library "loadimg" that allows you to make converting images to base64 a breeze.
🔗 https://github.com/not-lain/loadimg
API request example 🛠️:
you can do that by converting your local image to base64 and sending it to the API.
recently I made some changes to my library "loadimg" that allows you to make converting images to base64 a breeze.
🔗 https://github.com/not-lain/loadimg
API request example 🛠️:
from loadimg import load_img
from huggingface_hub import InferenceClient
# or load a local image
my_b64_img = load_img(imgPath_url_pillow_or_numpy ,output_type="base64" )
client = InferenceClient(api_key="hf_xxxxxxxxxxxxxxxxxxxxxxxxxxxxxxxxx")
messages = [
{
"role": "user",
"content": [
{
"type": "text",
"text": "Describe this image in one sentence."
},
{
"type": "image_url",
"image_url": {
"url": my_b64_img # base64 allows using images without uploading them to the web
}
}
]
}
]
stream = client.chat.completions.create(
model="meta-llama/Llama-3.2-11B-Vision-Instruct",
messages=messages,
max_tokens=500,
stream=True
)
for chunk in stream:
print(chunk.choices[0].delta.content, end="")
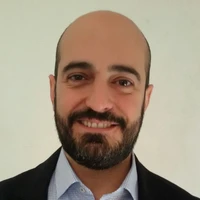
albertvillanova
posted
an
update
about 2 months ago
Post
1457
🚀 New feature of the Comparator of the 🤗 Open LLM Leaderboard: now compare models with their base versions & derivatives (finetunes, adapters, etc.). Perfect for tracking how adjustments affect performance & seeing innovations in action. Dive deeper into the leaderboard!
🛠️ Here's how to use it:
1. Select your model from the leaderboard.
2. Load its model tree.
3. Choose any base & derived models (adapters, finetunes, merges, quantizations) for comparison.
4. Press Load.
See side-by-side performance metrics instantly!
Ready to dive in? 🏆 Try the 🤗 Open LLM Leaderboard Comparator now! See how models stack up against their base versions and derivatives to understand fine-tuning and other adjustments. Easier model analysis for better insights! Check it out here: open-llm-leaderboard/comparator 🌐
🛠️ Here's how to use it:
1. Select your model from the leaderboard.
2. Load its model tree.
3. Choose any base & derived models (adapters, finetunes, merges, quantizations) for comparison.
4. Press Load.
See side-by-side performance metrics instantly!
Ready to dive in? 🏆 Try the 🤗 Open LLM Leaderboard Comparator now! See how models stack up against their base versions and derivatives to understand fine-tuning and other adjustments. Easier model analysis for better insights! Check it out here: open-llm-leaderboard/comparator 🌐
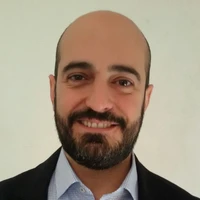
albertvillanova
posted
an
update
about 2 months ago
Post
3114
🚀 Exciting update! You can now compare multiple models side-by-side with the Hugging Face Open LLM Comparator! 📊
open-llm-leaderboard/comparator
Dive into multi-model evaluations, pinpoint the best model for your needs, and explore insights across top open LLMs all in one place. Ready to level up your model comparison game?
open-llm-leaderboard/comparator
Dive into multi-model evaluations, pinpoint the best model for your needs, and explore insights across top open LLMs all in one place. Ready to level up your model comparison game?
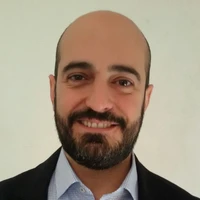
albertvillanova
posted
an
update
about 2 months ago
Post
1220
🚨 Instruct-tuning impacts models differently across families! Qwen2.5-72B-Instruct excels on IFEval but struggles with MATH-Hard, while Llama-3.1-70B-Instruct avoids MATH performance loss! Why? Can they follow the format in examples? 📊 Compare models:
open-llm-leaderboard/comparator
Post
2260
The Mystery Bot 🕵️♂️ saga I posted about from earlier this week has been solved...🤗
Cohere for AI has just announced its open source Aya Expanse multilingual model. The Initial release supports 23 languages with more on the way soon.🌌 🌍
You can also try Aya Expanse via SMS on your mobile phone using the global WhatsApp number or one of the initial set of country specific numbers listed below.⬇️
🌍WhatsApp - +14313028498
Germany - (+49) 1771786365
USA – +18332746219
United Kingdom — (+44) 7418373332
Canada – (+1) 2044107115
Netherlands – (+31) 97006520757
Brazil — (+55) 11950110169
Portugal – (+351) 923249773
Italy – (+39) 3399950813
Poland - (+48) 459050281
Cohere for AI has just announced its open source Aya Expanse multilingual model. The Initial release supports 23 languages with more on the way soon.🌌 🌍
You can also try Aya Expanse via SMS on your mobile phone using the global WhatsApp number or one of the initial set of country specific numbers listed below.⬇️
🌍WhatsApp - +14313028498
Germany - (+49) 1771786365
USA – +18332746219
United Kingdom — (+44) 7418373332
Canada – (+1) 2044107115
Netherlands – (+31) 97006520757
Brazil — (+55) 11950110169
Portugal – (+351) 923249773
Italy – (+39) 3399950813
Poland - (+48) 459050281
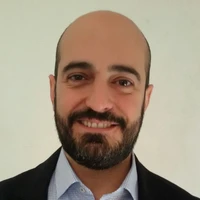
albertvillanova
posted
an
update
2 months ago
Post
1910
Finding the Best SmolLM for Your Project
Need an LLM assistant but unsure which hashtag#smolLM to run locally? With so many models available, how can you decide which one suits your needs best? 🤔
If the model you’re interested in is evaluated on the Hugging Face Open LLM Leaderboard, there’s an easy way to compare them: use the model Comparator tool: open-llm-leaderboard/comparator
Let’s walk through an example👇
Let’s compare two solid options:
- Qwen2.5-1.5B-Instruct from Alibaba Cloud Qwen (1.5B params)
- gemma-2-2b-it from Google (2.5B params)
For an assistant, you want a model that’s great at instruction following. So, how do these two models stack up on the IFEval task?
What about other evaluations?
Both models are close in performance on many other tasks, showing minimal differences. Surprisingly, the 1.5B Qwen model performs just as well as the 2.5B Gemma in many areas, even though it's smaller in size! 📊
This is a great example of how parameter size isn’t everything. With efficient design and training, a smaller model like Qwen2.5-1.5B can match or even surpass larger models in certain tasks.
Looking for other comparisons? Drop your model suggestions below! 👇
Need an LLM assistant but unsure which hashtag#smolLM to run locally? With so many models available, how can you decide which one suits your needs best? 🤔
If the model you’re interested in is evaluated on the Hugging Face Open LLM Leaderboard, there’s an easy way to compare them: use the model Comparator tool: open-llm-leaderboard/comparator
Let’s walk through an example👇
Let’s compare two solid options:
- Qwen2.5-1.5B-Instruct from Alibaba Cloud Qwen (1.5B params)
- gemma-2-2b-it from Google (2.5B params)
For an assistant, you want a model that’s great at instruction following. So, how do these two models stack up on the IFEval task?
What about other evaluations?
Both models are close in performance on many other tasks, showing minimal differences. Surprisingly, the 1.5B Qwen model performs just as well as the 2.5B Gemma in many areas, even though it's smaller in size! 📊
This is a great example of how parameter size isn’t everything. With efficient design and training, a smaller model like Qwen2.5-1.5B can match or even surpass larger models in certain tasks.
Looking for other comparisons? Drop your model suggestions below! 👇
Post
2510
Spent the weekend testing out some prompts with 🕵️♂️Mystery Bot🕵️♂️ on my mobile... exciting things are coming soon for the following languages:
🌐Arabic, Chinese, Czech, Dutch, English French, German, Greek, Hebrew, Hindi, Indonesian, Italian, Japanese, Korean, Persian, Polish, Portuguese, Romanian, Russian, Spanish, Turkish, Ukrainian, and Vietnamese!🌐
🌐Arabic, Chinese, Czech, Dutch, English French, German, Greek, Hebrew, Hindi, Indonesian, Italian, Japanese, Korean, Persian, Polish, Portuguese, Romanian, Russian, Spanish, Turkish, Ukrainian, and Vietnamese!🌐
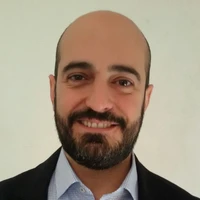
albertvillanova
posted
an
update
2 months ago
Post
1946
🚨 We’ve just released a new tool to compare the performance of models in the 🤗 Open LLM Leaderboard: the Comparator 🎉
open-llm-leaderboard/comparator
Want to see how two different versions of LLaMA stack up? Let’s walk through a step-by-step comparison of LLaMA-3.1 and LLaMA-3.2. 🦙🧵👇
1/ Load the Models' Results
- Go to the 🤗 Open LLM Leaderboard Comparator: open-llm-leaderboard/comparator
- Search for "LLaMA-3.1" and "LLaMA-3.2" in the model dropdowns.
- Press the Load button. Ready to dive into the results!
2/ Compare Metric Results in the Results Tab 📊
- Head over to the Results tab.
- Here, you’ll see the performance metrics for each model, beautifully color-coded using a gradient to highlight performance differences: greener is better! 🌟
- Want to focus on a specific task? Use the Task filter to hone in on comparisons for tasks like BBH or MMLU-Pro.
3/ Check Config Alignment in the Configs Tab ⚙️
- To ensure you’re comparing apples to apples, head to the Configs tab.
- Review both models’ evaluation configurations, such as metrics, datasets, prompts, few-shot configs...
- If something looks off, it’s good to know before drawing conclusions! ✅
4/ Compare Predictions by Sample in the Details Tab 🔍
- Curious about how each model responds to specific inputs? The Details tab is your go-to!
- Select a Task (e.g., MuSR) and then a Subtask (e.g., Murder Mystery) and then press the Load Details button.
- Check out the side-by-side predictions and dive into the nuances of each model’s outputs.
5/ With this tool, it’s never been easier to explore how small changes between model versions affect performance on a wide range of tasks. Whether you’re a researcher or enthusiast, you can instantly visualize improvements and dive into detailed comparisons.
🚀 Try the 🤗 Open LLM Leaderboard Comparator now and take your model evaluations to the next level!
open-llm-leaderboard/comparator
Want to see how two different versions of LLaMA stack up? Let’s walk through a step-by-step comparison of LLaMA-3.1 and LLaMA-3.2. 🦙🧵👇
1/ Load the Models' Results
- Go to the 🤗 Open LLM Leaderboard Comparator: open-llm-leaderboard/comparator
- Search for "LLaMA-3.1" and "LLaMA-3.2" in the model dropdowns.
- Press the Load button. Ready to dive into the results!
2/ Compare Metric Results in the Results Tab 📊
- Head over to the Results tab.
- Here, you’ll see the performance metrics for each model, beautifully color-coded using a gradient to highlight performance differences: greener is better! 🌟
- Want to focus on a specific task? Use the Task filter to hone in on comparisons for tasks like BBH or MMLU-Pro.
3/ Check Config Alignment in the Configs Tab ⚙️
- To ensure you’re comparing apples to apples, head to the Configs tab.
- Review both models’ evaluation configurations, such as metrics, datasets, prompts, few-shot configs...
- If something looks off, it’s good to know before drawing conclusions! ✅
4/ Compare Predictions by Sample in the Details Tab 🔍
- Curious about how each model responds to specific inputs? The Details tab is your go-to!
- Select a Task (e.g., MuSR) and then a Subtask (e.g., Murder Mystery) and then press the Load Details button.
- Check out the side-by-side predictions and dive into the nuances of each model’s outputs.
5/ With this tool, it’s never been easier to explore how small changes between model versions affect performance on a wide range of tasks. Whether you’re a researcher or enthusiast, you can instantly visualize improvements and dive into detailed comparisons.
🚀 Try the 🤗 Open LLM Leaderboard Comparator now and take your model evaluations to the next level!
MaximumEntropy
authored
a
paper
2 months ago
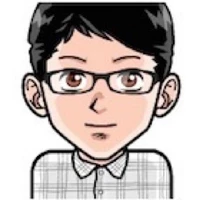
bwang0911
authored
4
papers
3 months ago
Leveraging Passage Embeddings for Efficient Listwise Reranking with Large Language Models
Paper
•
2406.14848
•
Published
•
3
Late Chunking: Contextual Chunk Embeddings Using Long-Context Embedding Models
Paper
•
2409.04701
•
Published
jina-embeddings-v3: Multilingual Embeddings With Task LoRA
Paper
•
2409.10173
•
Published
•
28
Jina-ColBERT-v2: A General-Purpose Multilingual Late Interaction Retriever
Paper
•
2408.16672
•
Published
•
7
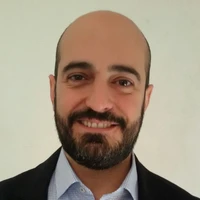
albertvillanova
posted
an
update
3 months ago
Post
1524
Check out the new Structured #Wikipedia dataset by Wikimedia Enterprise: abstract, infobox, structured sections, main image,...
Currently in early beta (English & French). Explore it and give feedback: wikimedia/structured-wikipedia
More info: https://enterprise.wikimedia.com/blog/hugging-face-dataset/
@sdelbecque @resquito-wmf
Currently in early beta (English & French). Explore it and give feedback: wikimedia/structured-wikipedia
More info: https://enterprise.wikimedia.com/blog/hugging-face-dataset/
@sdelbecque @resquito-wmf
Post
1385
📢 2024 CVPR Videos Are Now Available! 🎥
CVPR conference keynotes, panels, posters, workshops, and other content are now available.
⬇️
https://cvpr.thecvf.com/Conferences/2024/Videos
CVPR conference keynotes, panels, posters, workshops, and other content are now available.
⬇️
https://cvpr.thecvf.com/Conferences/2024/Videos
Post
2347
💡Andrew Ng recently gave a strong defense of Open Source AI models and the need to slow down legislative efforts in the US and the EU to restrict innovation in Open Source AI at Stanford GSB.
🎥See video below
https://youtu.be/yzUdmwlh1sQ?si=bZc690p8iubolXm_
🎥See video below
https://youtu.be/yzUdmwlh1sQ?si=bZc690p8iubolXm_