AI & ML interests
None defined yet.
Recent Activity
View all activity
openmmlab's activity
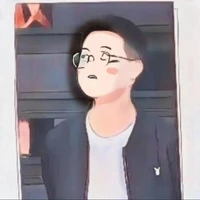
vansinĀ
authored
a
paper
4 months ago
Post
1735
With the open-weight release of CogVideoX-5B from THUDM, i.e. GLM team, the Video Generation Model (how about calling it VGM) field has officially became the next booming "LLM"
What does the landscape look like? What are other video generation models? This collection below is all your need.
xianbao/video-generation-models-66c350163c74f60f5c412af6
The above video is generated by @a-r-r-o-w with CogVideoX-5B, taken from a nice lookout for the field!
What does the landscape look like? What are other video generation models? This collection below is all your need.
xianbao/video-generation-models-66c350163c74f60f5c412af6
The above video is generated by @a-r-r-o-w with CogVideoX-5B, taken from a nice lookout for the field!
ly015Ā
authored
a
paper
6 months ago
xianbaoĀ
authored
a
paper
7 months ago
Post
1817
Why Apache 2.0 Matters for LLMs š¤
@01AI_Yi recently switched from a permissive & commercially friendly license, to Apache 2.0. And the community loved it! š
@JustinLin610 also had a poll on model license and the majority votes for Apache 2.0.
Why it is a Big Deal? ā¬ļø
š Legal Simplicity: Custom licenses need costly & time-consuming legal review. Apache 2.0 is well-known & easier for legal teams to handle.
š©āš» Developer-Friendly: Legal docs are a pain for devs! Apache 2.0 is well-known and tech-friendly, making it easier for non-native developers to understand the implications too.
š Easier Integration: Apache 2.0 is compatible with many other licenses, simplifying tasks like model merging with models of different licensing requirements.
š« No Permission Needed: Custom licenses often require explicit permission and additional documentation work of filling forms, creating barriers. Apache 2.0 removes this hurdle, letting devs focus on innovation.
There are a lot interesting discussions from
@JustinLin610 's poll: https://x.com/JustinLin610/status/1793559737482764375 which inspired this thread.
Any other thoughts? Let me know ^^
@01AI_Yi recently switched from a permissive & commercially friendly license, to Apache 2.0. And the community loved it! š
@JustinLin610 also had a poll on model license and the majority votes for Apache 2.0.
Why it is a Big Deal? ā¬ļø
š Legal Simplicity: Custom licenses need costly & time-consuming legal review. Apache 2.0 is well-known & easier for legal teams to handle.
š©āš» Developer-Friendly: Legal docs are a pain for devs! Apache 2.0 is well-known and tech-friendly, making it easier for non-native developers to understand the implications too.
š Easier Integration: Apache 2.0 is compatible with many other licenses, simplifying tasks like model merging with models of different licensing requirements.
š« No Permission Needed: Custom licenses often require explicit permission and additional documentation work of filling forms, creating barriers. Apache 2.0 removes this hurdle, letting devs focus on innovation.
There are a lot interesting discussions from
@JustinLin610 's poll: https://x.com/JustinLin610/status/1793559737482764375 which inspired this thread.
Any other thoughts? Let me know ^^
Post
1219
DeepSeekV2 is a big deal. Not only because its significant improvements to both key components of Transformer: the Attention layer and FFN layer.
It has also completed disrupted the Chines LLM market and forcing the competitors to drop the price to 1% of the original price.
---
There are two key components in Transformer architecture: the self-attention layer, which captures relationships between tokens in context, and the Feed-Forward Network (FFN) layer, which stores knowledge.
DeepSeek V2 introduces optimizations to both:
Attention layer normally uses KV Cache to reduce repetitive compute, but it consumes significant GPU RAM, limiting concurrent requests. DeepSeek V2 introduces Multi-head Latent Attention (MLA), which stores only a small latent representation, resulting in substantial RAM savings.
DeepSeek V2 utilizes 162 experts instead of the usual 8 as in Mixtral. This approach segments experts into finer granularity for higher specialization and more accurate knowledge acquisition. Activating only a small subset of experts for each token, leads to efficient processing.
It disrupted the market by dropping API prices to $0.14 per 1M tokens. This dramatic reduction forced competitors like GLM, Ernie, and QWen to follow suit, lowering their prices to 1% of their original offerings. Now, users can access these APIs at 1/35th the cost of ChatGPT-4o.
It has also completed disrupted the Chines LLM market and forcing the competitors to drop the price to 1% of the original price.
---
There are two key components in Transformer architecture: the self-attention layer, which captures relationships between tokens in context, and the Feed-Forward Network (FFN) layer, which stores knowledge.
DeepSeek V2 introduces optimizations to both:
Attention layer normally uses KV Cache to reduce repetitive compute, but it consumes significant GPU RAM, limiting concurrent requests. DeepSeek V2 introduces Multi-head Latent Attention (MLA), which stores only a small latent representation, resulting in substantial RAM savings.
DeepSeek V2 utilizes 162 experts instead of the usual 8 as in Mixtral. This approach segments experts into finer granularity for higher specialization and more accurate knowledge acquisition. Activating only a small subset of experts for each token, leads to efficient processing.
It disrupted the market by dropping API prices to $0.14 per 1M tokens. This dramatic reduction forced competitors like GLM, Ernie, and QWen to follow suit, lowering their prices to 1% of their original offerings. Now, users can access these APIs at 1/35th the cost of ChatGPT-4o.
Post
1861
So hard to keep up with pace!!! Lots of new Chinese fine-tunes are being released on HF
So I asked my agent to create a collection
xianbao/llama3-zh-662ba8503bdfe51948a28403
code: https://colab.research.google.com/drive/1ap6fP-VytZE367Nqk26DeQqgQkYaf-cD#scrollTo=eljRbYb4c92M
Would be nice to run then regularly. Any thoughts / suggestions on where to host this cron job?
So I asked my agent to create a collection
xianbao/llama3-zh-662ba8503bdfe51948a28403
code: https://colab.research.google.com/drive/1ap6fP-VytZE367Nqk26DeQqgQkYaf-cD#scrollTo=eljRbYb4c92M
Would be nice to run then regularly. Any thoughts / suggestions on where to host this cron job?
Post
Welcome Bunny! A family of lightweight but powerful multimodal models from BAAI
With detailed work on dataset curation, the Bunny-3B model built upon SigLIP and Phi-2 achieves performance on par with 13B models.
Model: BAAI/bunny-phi-2-siglip-lora
With detailed work on dataset curation, the Bunny-3B model built upon SigLIP and Phi-2 achieves performance on par with 13B models.
Model: BAAI/bunny-phi-2-siglip-lora
Post
There appears to be a huge misunderstanding regarding the licensing requirements for open sourced Chinese speaking speaking LLMs on
@huggingface
I initially shared this misconception too, but after conducting some research, I came up with the list below.
Veryimpressive!
@huggingface
I initially shared this misconception too, but after conducting some research, I came up with the list below.
Veryimpressive!
ly015Ā
authored
a
paper
11 months ago
ly015Ā
authored
a
paper
12 months ago
nielsrĀ
updated
6
models
over 1 year ago
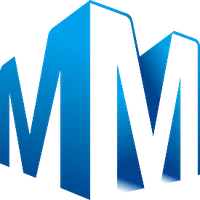
openmmlab/upernet-convnext-base
Image Segmentation
ā¢
Updated
ā¢
545
ā¢
1
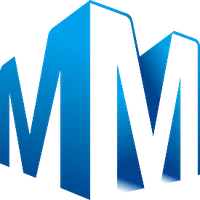
openmmlab/upernet-swin-small
Image Segmentation
ā¢
Updated
ā¢
212
ā¢
5
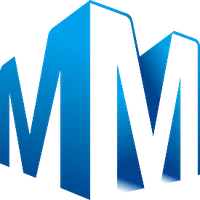
openmmlab/upernet-convnext-xlarge
Image Segmentation
ā¢
Updated
ā¢
441
ā¢
2
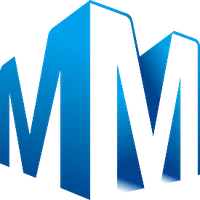
openmmlab/upernet-swin-base
Image Segmentation
ā¢
Updated
ā¢
255
ā¢
1
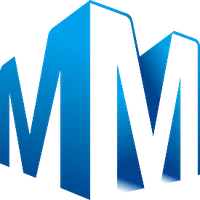
openmmlab/upernet-swin-large
Image Segmentation
ā¢
Updated
ā¢
5.96k
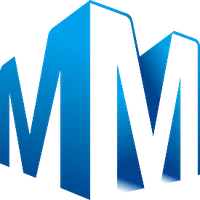
openmmlab/upernet-convnext-tiny
Image Segmentation
ā¢
Updated
ā¢
1.48k
ā¢
3