Sebastian Gilits
sepal
AI & ML interests
Working on AI during day and night :P
Generative AI, NLP, TTI TTV, Audio
Recent Activity
reacted
to
nroggendorff's
post
with 😔
10 days ago
im so tired
liked
a model
15 days ago
fal/AuraFlow
liked
a model
17 days ago
FastVideo/FastHunyuan
Organizations
sepal's activity
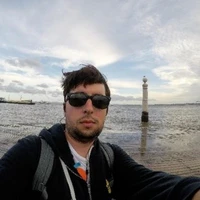
reacted to
nroggendorff's
post with 😔
10 days ago
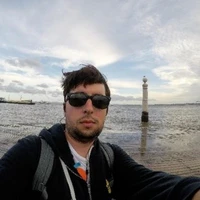
reacted to
Kseniase's
post with 🔥
19 days ago
Post
2797
TL;DR: The Story of Attention's Development by
@karpathy
Origin: First proposed in 2014 by @Dzmitry Bahdanau, @KyunghyunCho , and Yoshua Bengio in Neural Machine Translation by Jointly Learning to Align and Translate (1409.0473) . Inspired by cognitive processes and later renamed from "RNNSearch."
Key Idea: A data-dependent weighted average for pooling and communication, enabling flexible and powerful neural network connections.
Breakthrough: Bahdanau's "soft search" mechanism (softmax + weighted averaging) solved encoder-decoder bottlenecks in machine translation.
Transformer Revolution: Attention Is All You Need (1706.03762) (2017) by @ashishvaswanigoogle et al. simplified architectures by stacking attention layers, introducing multi-headed attention and positional encodings.
Legacy: Attention replaced RNNs, driving modern AI systems like ChatGPT. It emerged independently but was influenced by contemporaneous work like Alex Graves’s Neural Turing Machines (1410.5401) and Jason Weston’s Memory Networks (1410.3916) .
Attention to history: Jürgen Schmidhuber claims his 1992 Fast Weight Programmers anticipated modern attention mechanisms. While conceptually similar, the term “attention” was absent, and there’s no evidence it influenced Bahdanau, Cho, and Bengio’s 2014 work. Paying attention (!) to history might have brought us to genAI earlier – but credit for the breakthrough still goes to Montreal.
Referenced Papers:
Attention Origin: Neural Machine Translation by Jointly Learning to Align and Translate (1409.0473)
Transformers: Attention Is All You Need (1706.03762)
Alex Graves' Work: Neural Turing Machines (1410.5401), Generating Sequences With Recurrent Neural Networks (1308.0850)
Jason Weston @spermwhale 's Memory Networks (1410.3916)
Sequence to Sequence Learning with Neural Networks (1409.3215) by Ilya Sutskever ( @ilyasut ), Oriol Vinyals, Quoc V. Le
Who else deserves recognition in this groundbreaking narrative of innovation? Let’s ensure every contributor gets the credit they deserve. Leave a comment below 👇🏻🤗
Origin: First proposed in 2014 by @Dzmitry Bahdanau, @KyunghyunCho , and Yoshua Bengio in Neural Machine Translation by Jointly Learning to Align and Translate (1409.0473) . Inspired by cognitive processes and later renamed from "RNNSearch."
Key Idea: A data-dependent weighted average for pooling and communication, enabling flexible and powerful neural network connections.
Breakthrough: Bahdanau's "soft search" mechanism (softmax + weighted averaging) solved encoder-decoder bottlenecks in machine translation.
Transformer Revolution: Attention Is All You Need (1706.03762) (2017) by @ashishvaswanigoogle et al. simplified architectures by stacking attention layers, introducing multi-headed attention and positional encodings.
Legacy: Attention replaced RNNs, driving modern AI systems like ChatGPT. It emerged independently but was influenced by contemporaneous work like Alex Graves’s Neural Turing Machines (1410.5401) and Jason Weston’s Memory Networks (1410.3916) .
Attention to history: Jürgen Schmidhuber claims his 1992 Fast Weight Programmers anticipated modern attention mechanisms. While conceptually similar, the term “attention” was absent, and there’s no evidence it influenced Bahdanau, Cho, and Bengio’s 2014 work. Paying attention (!) to history might have brought us to genAI earlier – but credit for the breakthrough still goes to Montreal.
Referenced Papers:
Attention Origin: Neural Machine Translation by Jointly Learning to Align and Translate (1409.0473)
Transformers: Attention Is All You Need (1706.03762)
Alex Graves' Work: Neural Turing Machines (1410.5401), Generating Sequences With Recurrent Neural Networks (1308.0850)
Jason Weston @spermwhale 's Memory Networks (1410.3916)
Sequence to Sequence Learning with Neural Networks (1409.3215) by Ilya Sutskever ( @ilyasut ), Oriol Vinyals, Quoc V. Le
Who else deserves recognition in this groundbreaking narrative of innovation? Let’s ensure every contributor gets the credit they deserve. Leave a comment below 👇🏻🤗
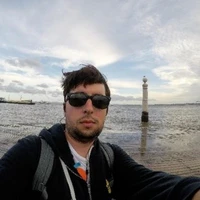
reacted to
FranckAbgrall's
post with 🔥
23 days ago
Post
1988
Hey!
✨ If you're using HF access tokens, we just released an overview of the permissions for fine-grained tokens by hovering over the badge on token settings page (org and user)
It will show the highest permission you've set for each entity 👀
✨ If you're using HF access tokens, we just released an overview of the permissions for fine-grained tokens by hovering over the badge on token settings page (org and user)
It will show the highest permission you've set for each entity 👀