license: llama2
inference: false
pipeline_tag: text-generation
tags:
- not-for-all-audiences
language:
- en
GGML's of Pygmalion Vicuna 1.1 7B
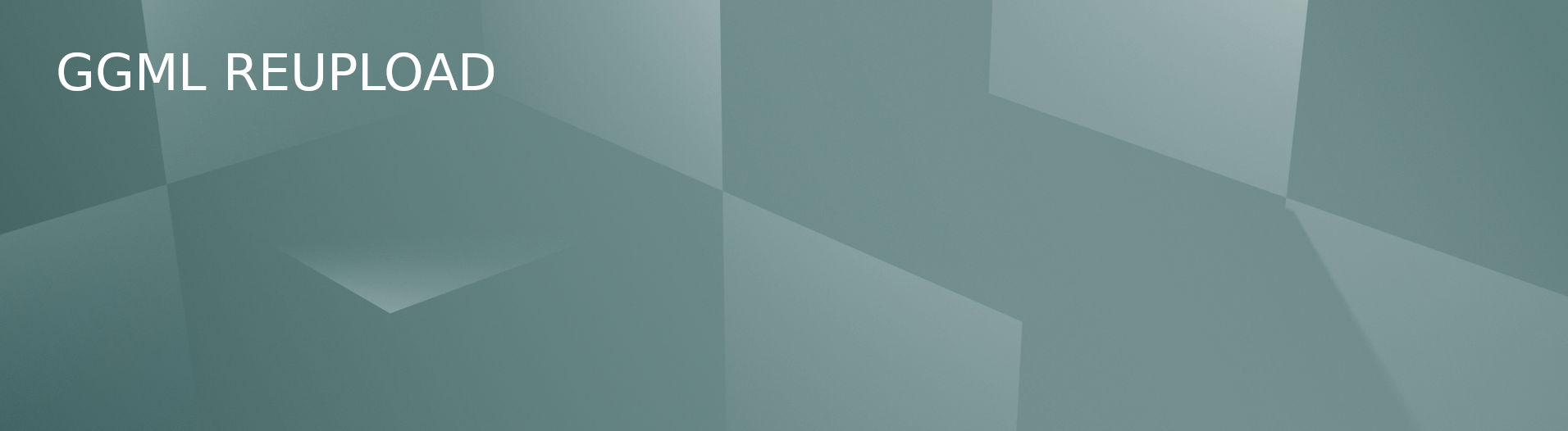
a GGML re-upload by Shadowsword
https://huggingface.co/TehVenom/Pygmalion-Vicuna-1.1-7b
ggmlv3 from TheBloke's make-ggml.py commit to huggingface repo
example$ python3 ./make-ggml.py --model /home/inpw/Pygmalion-1.1-7b --outname Pygmalion-Vicuna-1.1-7b --outdir /home/inpw/Pygmalion-Vicuna-1.1-7b --keep_fp16 --quants ...
Includes USE_POLICY.md
making sure to comply with license agreements / legalities.
Provided GGML Quants
Quant Method | Use Case |
---|---|
Q2_K | New k-quant method. Uses GGML_TYPE_Q4_K for the attention.vw and feed_forward.w2 tensors, GGML_TYPE_Q2_K for the other tensors. |
Q3_K_S | New k-quant method. Uses GGML_TYPE_Q3_K for all tensors |
Q3_K_M | New k-quant method. Uses GGML_TYPE_Q4_K for the attention.wv, attention.wo, and feed_forward.w2 tensors, else GGML_TYPE_Q3_K |
Q3_K_L | New k-quant method. Uses GGML_TYPE_Q5_K for the attention.wv, attention.wo, and feed_forward.w2 tensors, else GGML_TYPE_Q3_K |
Q4_0 | Original quant method, 4-bit. |
Q4_1 | Original quant method, 4-bit. Higher accuracy than q4_0 but not as high as q5_0. However has quicker inference than q5 models. |
Q4_K_S | New k-quant method. Uses GGML_TYPE_Q4_K for all tensors |
Q4_K_M | New k-quant method. Uses GGML_TYPE_Q6_K for half of the attention.wv and feed_forward.w2 tensors, else GGML_TYPE_Q4_K |
Q5_0 | Original quant method, 5-bit. Higher accuracy, higher resource usage and slower inference. |
Q5_1 | Original quant method, 5-bit. Even higher accuracy, resource usage and slower inference. |
Q5_K_S | New k-quant method. Uses GGML_TYPE_Q5_K for all tensors |
Q5_K_M | New k-quant method. Uses GGML_TYPE_Q6_K for half of the attention.wv and feed_forward.w2 tensors, else GGML_TYPE_Q5_K |
Q6_K | New k-quant method. Uses GGML_TYPE_Q8_K for all tensors - 6-bit quantization |
fp16 | Compiled Safetensors, can be used to quantize |
Thanks to TheBloke for the information on quant use cases.
RAM/VRAM | Parameters | GPU Offload (2K ctx, Q4_0, 6GB RTX 2060) |
---|---|---|
4GB | 3B | |
8GB | 7B | 32 |
16GB | 13B | 18 |
32GB | 30B | 8 |
64GB | 65B |
Original Card:
Pygmalion Vicuna 1.1 7B
The LLaMA based Pygmalion-7b model:
https://huggingface.co/PygmalionAI/pygmalion-7b
Merged alongside lmsys's Vicuna v1.1 deltas:
https://huggingface.co/lmsys/vicuna-13b-delta-v1.1
This merge was done using an weighted average merge strategy, and the end result is a model composed of:
Pygmalion-7b [60%] + LLaMA Vicuna v1.1 [40%]
This was done under request, but the end result is intended to lean heavily towards Pygmalion's chatting + RP tendencies, and to inherit some of Vicuna's Assistant / Instruct / Helpful properties.
Due to the influence of Pygmalion, this model will very likely generate content that is considered NSFW.
The specific prompting is unknown, but try Pygmalion's prompt styles first, then a mix of the two to see what brings most interesting results.