Spaces:
Runtime error
Runtime error
# Use in huggingface transformers (Beta) | |
[**Colab Notebook**](https://colab.research.google.com/drive/1Ho81RBV8jysZ7e0FhsSCk_v938QeDuy3?usp=sharing) | |
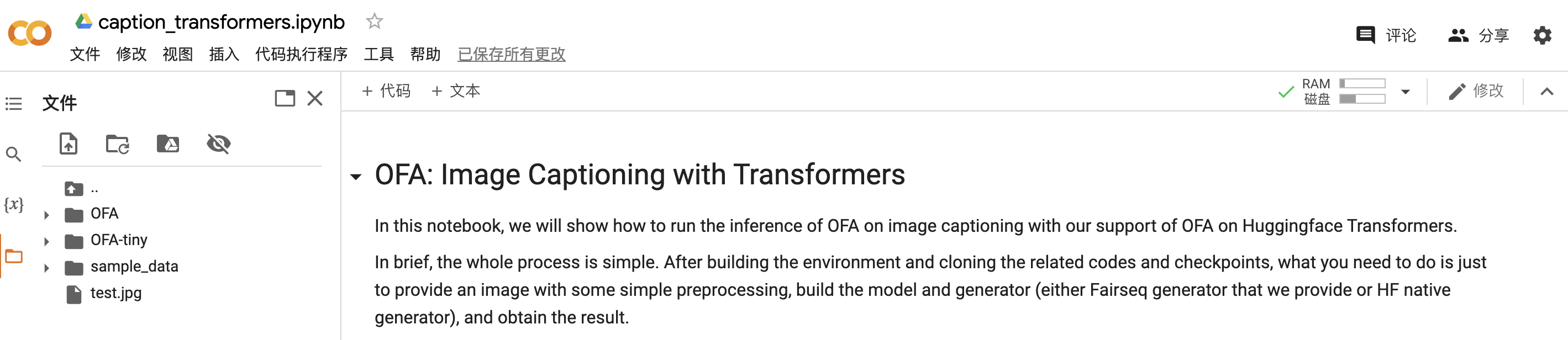 | |
We now support inference of OFA on the huggingface transformers. In the near future, we will provide the codes for training. | |
Model checkpoints are stored in our [huggingface models](https://huggingface.co/OFA-Sys). Specifically, 5 versions of the pretrained OFA models, namely OFA-tiny, OFA-medium, OFA-base, OFA-large, and OFA-huge have been already uploaded. For more information about the models, please refer to the Model Card on our [README](https://github.com/OFA-Sys/OFA). | |
Note that each directory includes 4 files, namely `config.json` which consists of model configuration, `vocab.json` and `merge.txt` for our OFA tokenizer, and lastly `pytorch_model.bin` which consists of model weights. There is no need to worry about the mismatch between Fairseq and transformers, since we have addressed the issue yet. | |
To use it in transformers, you can first refer to this notebook ([link](https://colab.research.google.com/drive/1Ho81RBV8jysZ7e0FhsSCk_v938QeDuy3?usp=sharing)). For more information, you can find codes in this branch https://github.com/OFA-Sys/OFA/tree/feature/add_transformers. | |
In the following, we introduce the details in our provided notebook and illustrate how to use OFA in Transformers. | |
First, install the transformers and download the models (take OFA-tiny as an example) as shown below. | |
``` | |
git clone --single-branch --branch feature/add_transformers https://github.com/OFA-Sys/OFA.git | |
pip install OFA/transformers/ | |
git clone https://huggingface.co/OFA-Sys/OFA-tiny | |
``` | |
Next, refer the path to OFA-tiny to `ckpt_dir`, and prepare an image for the testing example below. Also, ensure that you have pillow and torchvision in your environment. Check if there is the directory `generate` in your model directory `transformers/src/transformers/models/ofa` to ensure that you can use the sequence generator that we provide. | |
``` | |
>>> from PIL import Image | |
>>> from torchvision import transforms | |
>>> from transformers import OFATokenizer, OFAModel | |
>>> from transformers.models.ofa.generate import sequence_generator | |
>>> mean, std = [0.5, 0.5, 0.5], [0.5, 0.5, 0.5] | |
>>> resolution = 256 | |
>>> patch_resize_transform = transforms.Compose([ | |
lambda image: image.convert("RGB"), | |
transforms.Resize((resolution, resolution), interpolation=Image.BICUBIC), | |
transforms.ToTensor(), | |
transforms.Normalize(mean=mean, std=std) | |
]) | |
>>> tokenizer = OFATokenizer.from_pretrained(ckpt_dir) | |
>>> txt = " what does the image describe?" | |
>>> inputs = tokenizer([txt], return_tensors="pt").input_ids | |
>>> img = Image.open(path_to_image) | |
>>> patch_img = patch_resize_transform(img).unsqueeze(0) | |
>>> # using the generator of fairseq version | |
>>> model = OFAModel.from_pretrained(ckpt_dir, use_cache=True) | |
>>> generator = sequence_generator.SequenceGenerator( | |
tokenizer=tokenizer, | |
beam_size=5, | |
max_len_b=16, | |
min_len=0, | |
no_repeat_ngram_size=3, | |
) | |
>>> data = {} | |
>>> data["net_input"] = {"input_ids": inputs, 'patch_images': patch_img, 'patch_masks':torch.tensor([True])} | |
>>> gen_output = generator.generate([model], data) | |
>>> gen = [gen_output[i][0]["tokens"] for i in range(len(gen_output))] | |
>>> # using the generator of huggingface version | |
>>> model = OFAModel.from_pretrained(ckpt_dir, use_cache=False) | |
>>> gen = model.generate(inputs, patch_images=patch_img, num_beams=5, no_repeat_ngram_size=3) | |
>>> print(tokenizer.batch_decode(gen, skip_special_tokens=True)) | |
``` | |