|
# Building the World's Best Open-Source Large Language Model: H2O.ai's Journey |
|
|
|
by Arno Candel, PhD, CTO H2O.ai, April 19 2023 |
|
|
|
At H2O.ai, we pride ourselves on developing world-class Machine Learning, Deep Learning, and AI platforms. We released H2O, the most widely used open-source distributed and scalable machine learning platform, before XGBoost, TensorFlow and PyTorch existed. H2O.ai is home to over 25 Kaggle grandmasters, including the current #1. In 2017, we used GPUs to create the world's best AutoML in H2O Driverless AI. We have witnessed first-hand how Large Language Models (LLMs) have taken over the world by storm. |
|
|
|
We are proud to announce that we are building h2oGPT, an LLM that not only excels in performance but is also fully open-source and commercially usable, providing a valuable resource for developers, researchers, and organizations worldwide. |
|
|
|
In this blog, we'll explore our journey in building h2oGPT in our effort to further democratize AI. |
|
|
|
## Why Open-Source LLMs? |
|
|
|
While LLMs like OpenAI's ChatGPT/GPT-4, Anthropic's Claude, Microsoft's Bing AI Chat, Google's Bard, and Cohere are powerful and effective, they have certain limitations compared to open-source LLMs: |
|
|
|
1. **Data Privacy and Security**: Using hosted LLMs requires sending data to external servers. This can raise concerns about data privacy, security, and compliance, especially for sensitive information or industries with strict regulations. |
|
2. **Dependency and Customization**: Hosted LLMs often limit the extent of customization and control, as users rely on the service provider's infrastructure and predefined models. Open-source LLMs allow users to tailor the models to their specific needs, deploy on their own infrastructure, and even modify the underlying code. |
|
3. **Cost and Scalability**: Hosted LLMs usually come with usage fees, which can increase significantly with large-scale applications. Open-source LLMs can be more cost-effective, as users can scale the models on their own infrastructure without incurring additional costs from the service provider. |
|
4. **Access and Availability**: Hosted LLMs may be subject to downtime or limited availability, affecting users' access to the models. Open-source LLMs can be deployed on-premises or on private clouds, ensuring uninterrupted access and reducing reliance on external providers. |
|
|
|
Overall, open-source LLMs offer greater flexibility, control, and cost-effectiveness, while addressing data privacy and security concerns. They foster a competitive landscape in the AI industry and empower users to innovate and customize models to suit their specific needs. |
|
|
|
## The H2O.ai LLM Ecosystem |
|
|
|
Our open-source LLM ecosystem currently includes the following components: |
|
|
|
1. **Code, data, and models**: Fully permissive, commercially usable [code](https://github.com/h2oai/h2ogpt), curated fine-tuning [data](https://huggingface.co/h2oai), and fine-tuned [models](https://huggingface.co/h2oai) ranging from 7 to 20 billion parameters. |
|
2. **State-of-the-art fine-tuning**: We provide code for highly efficient fine-tuning, including targeted data preparation, prompt engineering, and computational optimizations to fine-tune LLMs with up to 20 billion parameters (even larger models expected soon) in hours on commodity hardware or enterprise servers. Techniques like low-rank approximations (LoRA) and data compression allow computational savings of several orders of magnitude. |
|
3. **Chatbot**: We provide code to run a multi-tenant chatbot on GPU servers, with an easily shareable end-point and a Python client API, allowing you to evaluate and compare the performance of fine-tuned LLMs. |
|
4. **H2O LLM Studio**: Our no-code LLM fine-tuning framework created by the world's top Kaggle grandmasters makes it even easier to fine-tune and evaluate LLMs. |
|
|
|
Everything we release is based on fully permissive data and models, with all code open-sourced, enabling broader access for businesses and commercial products without legal concerns, thus expanding access to cutting-edge AI while adhering to licensing requirements. |
|
|
|
## Roadmap and Future Plans |
|
|
|
We have an ambitious roadmap for our LLM ecosystem, including: |
|
|
|
1. Integration with downstream applications and low/no-code platforms (H2O Document AI, H2O LLM Studio, etc.) |
|
2. Improved validation and benchmarking frameworks of LLMs |
|
3. Complementing our chatbot with search and other APIs (LangChain, etc.) |
|
4. Contribute to large-scale data cleaning efforts (Open Assistant, Stability AI, RedPajama, etc.) |
|
5. High-performance distributed training of larger models on trillion tokens |
|
6. High-performance scalable on-premises hosting for high-throughput endpoints |
|
7. Improvements in code completion, reasoning, mathematics, factual correctness, hallucinations, and reducing repetitions |
|
|
|
## Getting Started with H2O.ai's LLMs |
|
|
|
You can [Chat with h2oGPT](https://gpt.h2o.ai/) right now! |
|
|
|
https://user-images.githubusercontent.com/6147661/232924684-6c0e2dfb-2f24-4098-848a-c3e4396f29f6.mov |
|
|
|
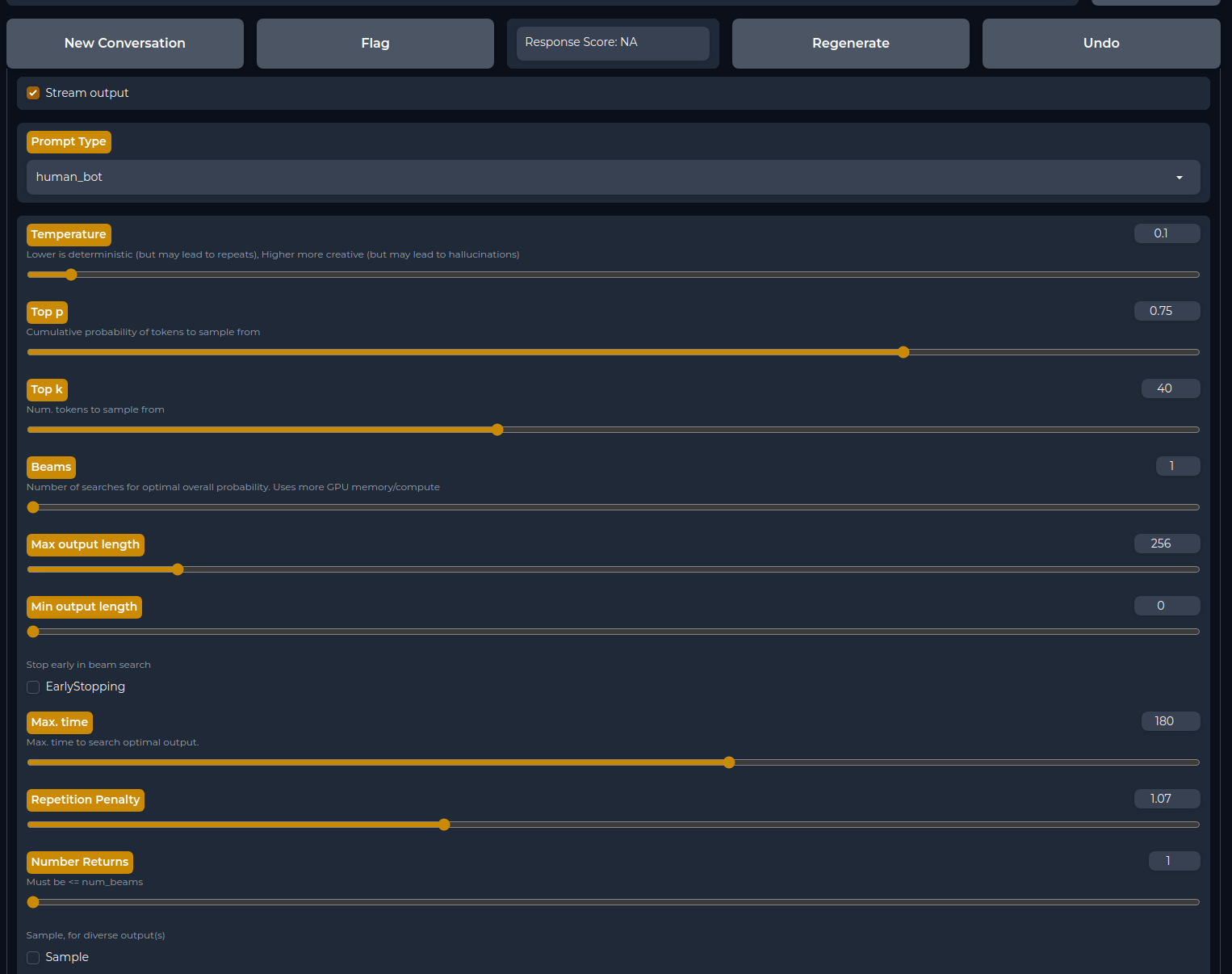 |
|
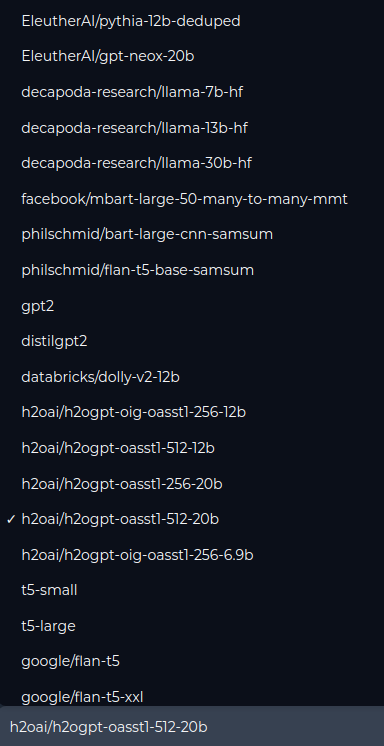 |
|
|
|
To start using our LLM as a developer, follow the steps below: |
|
|
|
1. Clone the repository: `git clone https://github.com/h2oai/h2ogpt.git` |
|
2. Change to the repository directory: `cd h2ogpt` |
|
3. Install the requirements: `pip install -r requirements.txt` |
|
4. Run the chatbot: `python generate.py --base_model=h2oai/h2ogpt-oig-oasst1-256-6_9b` |
|
5. Open your browser at `http://0.0.0.0:7860` or the public live URL printed by the server. |
|
|
|
For more information, visit [h2oGPT GitHub page](https://github.com/h2oai/h2ogpt), [H2O.ai's Hugging Face page](https://huggingface.co/h2oai) and [H2O LLM Studio GitHub page](https://github.com/h2oai/h2o-llmstudio). |
|
|
|
Join us on this exciting journey as we continue to improve and expand the capabilities of our open-source LLM ecosystem! |
|
|
|
## Acknowledgements |
|
|
|
We appreciate the work by many open-source contributors, especially: |
|
|
|
* [H2O.ai makers](https://h2o.ai/company/team/) |
|
* [Alpaca-LoRA](https://github.com/tloen/alpaca-lora/) |
|
* [LoRA](https://github.com/microsoft/LoRA/) |
|
* [Stanford Alpaca](https://github.com/tatsu-lab/stanford_alpaca/) |
|
* [Hugging Face](https://huggingface.co/) |
|
* [OpenAssistant](https://open-assistant.io/) |
|
* [EleutherAI](https://www.eleuther.ai/) |
|
* [LAION](https://laion.ai/blog/oig-dataset/) |
|
* [BigScience](https://github.com/bigscience-workshop/bigscience/) |
|
* [LLaMa](https://github.com/facebookresearch/llama/) |
|
* [StableLM](https://github.com/Stability-AI/StableLM/) |
|
* [Vicuna](https://github.com/lm-sys/FastChat/) |
|
|