Spaces:
Running
on
Zero
Running
on
Zero
# ControlNet training example | |
[Adding Conditional Control to Text-to-Image Diffusion Models](https://arxiv.org/abs/2302.05543) by Lvmin Zhang and Maneesh Agrawala. | |
This example is based on the [training example in the original ControlNet repository](https://github.com/lllyasviel/ControlNet/blob/main/docs/train.md). It trains a ControlNet to fill circles using a [small synthetic dataset](https://huggingface.co/datasets/fusing/fill50k). | |
## Installing the dependencies | |
Before running the scripts, make sure to install the library's training dependencies: | |
**Important** | |
To make sure you can successfully run the latest versions of the example scripts, we highly recommend **installing from source** and keeping the install up to date as we update the example scripts frequently and install some example-specific requirements. To do this, execute the following steps in a new virtual environment: | |
```bash | |
git clone https://github.com/huggingface/diffusers | |
cd diffusers | |
pip install -e . | |
``` | |
Then cd in the example folder and run | |
```bash | |
pip install -r requirements.txt | |
``` | |
And initialize an [🤗Accelerate](https://github.com/huggingface/accelerate/) environment with: | |
```bash | |
accelerate config | |
``` | |
Or for a default accelerate configuration without answering questions about your environment | |
```bash | |
accelerate config default | |
``` | |
Or if your environment doesn't support an interactive shell e.g. a notebook | |
```python | |
from accelerate.utils import write_basic_config | |
write_basic_config() | |
``` | |
## Circle filling dataset | |
The original dataset is hosted in the [ControlNet repo](https://huggingface.co/lllyasviel/ControlNet/blob/main/training/fill50k.zip). We re-uploaded it to be compatible with `datasets` [here](https://huggingface.co/datasets/fusing/fill50k). Note that `datasets` handles dataloading within the training script. | |
Our training examples use [Stable Diffusion 1.5](https://huggingface.co/runwayml/stable-diffusion-v1-5) as the original set of ControlNet models were trained from it. However, ControlNet can be trained to augment any Stable Diffusion compatible model (such as [CompVis/stable-diffusion-v1-4](https://huggingface.co/CompVis/stable-diffusion-v1-4)) or [stabilityai/stable-diffusion-2-1](https://huggingface.co/stabilityai/stable-diffusion-2-1). | |
## Training | |
Our training examples use two test conditioning images. They can be downloaded by running | |
```sh | |
wget https://huggingface.co/datasets/huggingface/documentation-images/resolve/main/diffusers/controlnet_training/conditioning_image_1.png | |
wget https://huggingface.co/datasets/huggingface/documentation-images/resolve/main/diffusers/controlnet_training/conditioning_image_2.png | |
``` | |
```bash | |
export MODEL_DIR="runwayml/stable-diffusion-v1-5" | |
export OUTPUT_DIR="path to save model" | |
accelerate launch train_controlnet.py \ | |
--pretrained_model_name_or_path=$MODEL_DIR \ | |
--output_dir=$OUTPUT_DIR \ | |
--dataset_name=fusing/fill50k \ | |
--resolution=512 \ | |
--learning_rate=1e-5 \ | |
--validation_image "./conditioning_image_1.png" "./conditioning_image_2.png" \ | |
--validation_prompt "red circle with blue background" "cyan circle with brown floral background" \ | |
--train_batch_size=4 | |
``` | |
This default configuration requires ~38GB VRAM. | |
By default, the training script logs outputs to tensorboard. Pass `--report_to wandb` to use weights and | |
biases. | |
Gradient accumulation with a smaller batch size can be used to reduce training requirements to ~20 GB VRAM. | |
```bash | |
export MODEL_DIR="runwayml/stable-diffusion-v1-5" | |
export OUTPUT_DIR="path to save model" | |
accelerate launch train_controlnet.py \ | |
--pretrained_model_name_or_path=$MODEL_DIR \ | |
--output_dir=$OUTPUT_DIR \ | |
--dataset_name=fusing/fill50k \ | |
--resolution=512 \ | |
--learning_rate=1e-5 \ | |
--validation_image "./conditioning_image_1.png" "./conditioning_image_2.png" \ | |
--validation_prompt "red circle with blue background" "cyan circle with brown floral background" \ | |
--train_batch_size=1 \ | |
--gradient_accumulation_steps=4 | |
``` | |
## Training with multiple GPUs | |
`accelerate` allows for seamless multi-GPU training. Follow the instructions [here](https://huggingface.co/docs/accelerate/basic_tutorials/launch) | |
for running distributed training with `accelerate`. Here is an example command: | |
```bash | |
export MODEL_DIR="runwayml/stable-diffusion-v1-5" | |
export OUTPUT_DIR="path to save model" | |
accelerate launch --mixed_precision="fp16" --multi_gpu train_controlnet.py \ | |
--pretrained_model_name_or_path=$MODEL_DIR \ | |
--output_dir=$OUTPUT_DIR \ | |
--dataset_name=fusing/fill50k \ | |
--resolution=512 \ | |
--learning_rate=1e-5 \ | |
--validation_image "./conditioning_image_1.png" "./conditioning_image_2.png" \ | |
--validation_prompt "red circle with blue background" "cyan circle with brown floral background" \ | |
--train_batch_size=4 \ | |
--mixed_precision="fp16" \ | |
--tracker_project_name="controlnet-demo" \ | |
--report_to=wandb | |
``` | |
## Example results | |
#### After 300 steps with batch size 8 | |
| | | | |
|-------------------|:-------------------------:| | |
| | red circle with blue background | | |
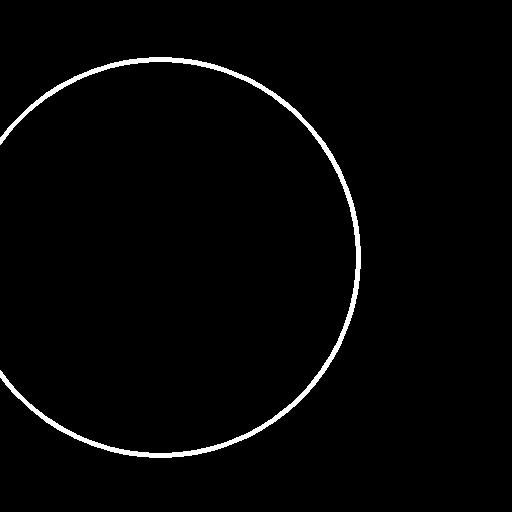 | 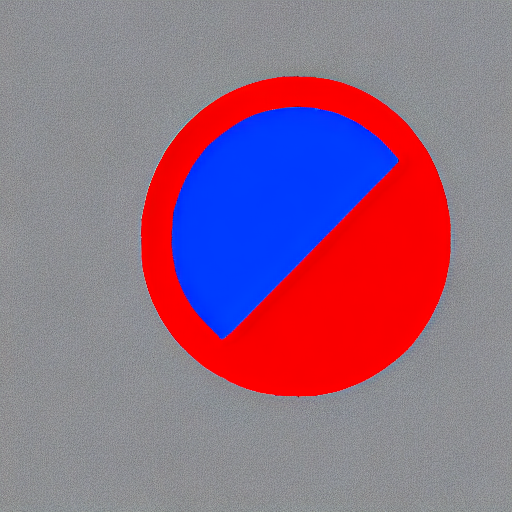 | | |
| | cyan circle with brown floral background | | |
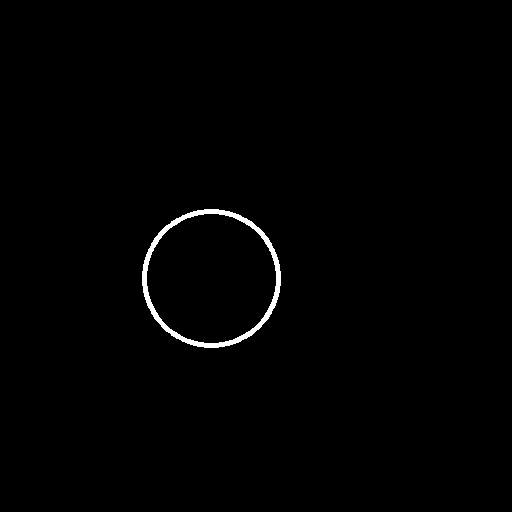 | 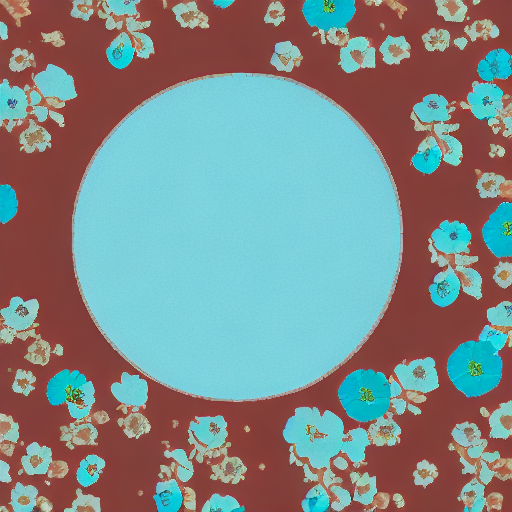 | | |
#### After 6000 steps with batch size 8: | |
| | | | |
|-------------------|:-------------------------:| | |
| | red circle with blue background | | |
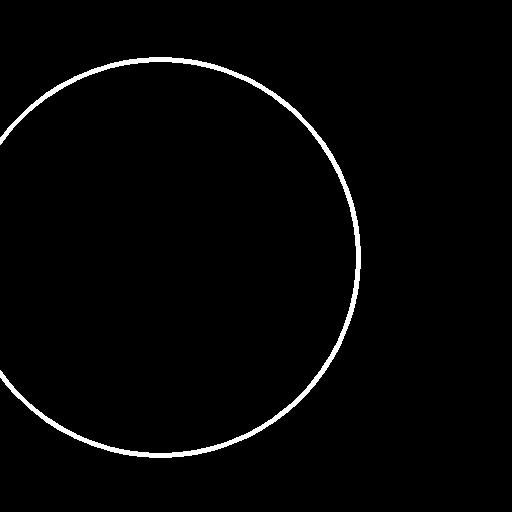 | 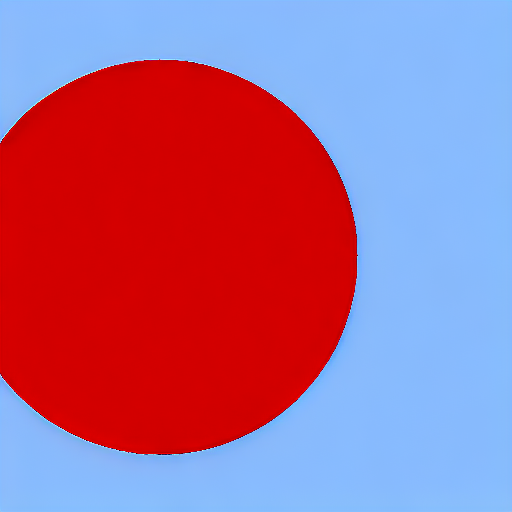 | | |
| | cyan circle with brown floral background | | |
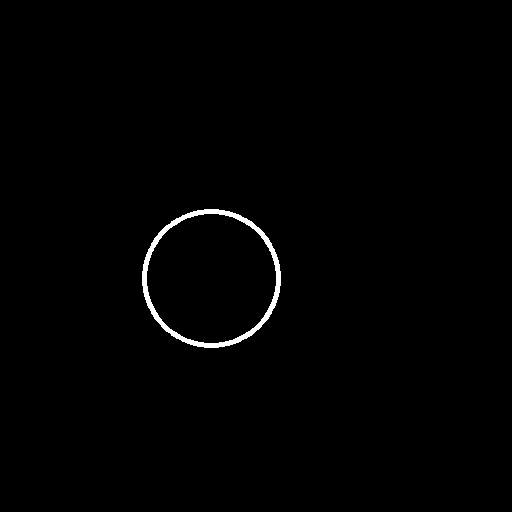 |  | | |
## Training on a 16 GB GPU | |
Optimizations: | |
- Gradient checkpointing | |
- bitsandbyte's 8-bit optimizer | |
[bitandbytes install instructions](https://github.com/TimDettmers/bitsandbytes#requirements--installation). | |
```bash | |
export MODEL_DIR="runwayml/stable-diffusion-v1-5" | |
export OUTPUT_DIR="path to save model" | |
accelerate launch train_controlnet.py \ | |
--pretrained_model_name_or_path=$MODEL_DIR \ | |
--output_dir=$OUTPUT_DIR \ | |
--dataset_name=fusing/fill50k \ | |
--resolution=512 \ | |
--learning_rate=1e-5 \ | |
--validation_image "./conditioning_image_1.png" "./conditioning_image_2.png" \ | |
--validation_prompt "red circle with blue background" "cyan circle with brown floral background" \ | |
--train_batch_size=1 \ | |
--gradient_accumulation_steps=4 \ | |
--gradient_checkpointing \ | |
--use_8bit_adam | |
``` | |
## Training on a 12 GB GPU | |
Optimizations: | |
- Gradient checkpointing | |
- bitsandbyte's 8-bit optimizer | |
- xformers | |
- set grads to none | |
```bash | |
export MODEL_DIR="runwayml/stable-diffusion-v1-5" | |
export OUTPUT_DIR="path to save model" | |
accelerate launch train_controlnet.py \ | |
--pretrained_model_name_or_path=$MODEL_DIR \ | |
--output_dir=$OUTPUT_DIR \ | |
--dataset_name=fusing/fill50k \ | |
--resolution=512 \ | |
--learning_rate=1e-5 \ | |
--validation_image "./conditioning_image_1.png" "./conditioning_image_2.png" \ | |
--validation_prompt "red circle with blue background" "cyan circle with brown floral background" \ | |
--train_batch_size=1 \ | |
--gradient_accumulation_steps=4 \ | |
--gradient_checkpointing \ | |
--use_8bit_adam \ | |
--enable_xformers_memory_efficient_attention \ | |
--set_grads_to_none | |
``` | |
When using `enable_xformers_memory_efficient_attention`, please make sure to install `xformers` by `pip install xformers`. | |
## Training on an 8 GB GPU | |
We have not exhaustively tested DeepSpeed support for ControlNet. While the configuration does | |
save memory, we have not confirmed the configuration to train successfully. You will very likely | |
have to make changes to the config to have a successful training run. | |
Optimizations: | |
- Gradient checkpointing | |
- xformers | |
- set grads to none | |
- DeepSpeed stage 2 with parameter and optimizer offloading | |
- fp16 mixed precision | |
[DeepSpeed](https://www.deepspeed.ai/) can offload tensors from VRAM to either | |
CPU or NVME. This requires significantly more RAM (about 25 GB). | |
Use `accelerate config` to enable DeepSpeed stage 2. | |
The relevant parts of the resulting accelerate config file are | |
```yaml | |
compute_environment: LOCAL_MACHINE | |
deepspeed_config: | |
gradient_accumulation_steps: 4 | |
offload_optimizer_device: cpu | |
offload_param_device: cpu | |
zero3_init_flag: false | |
zero_stage: 2 | |
distributed_type: DEEPSPEED | |
``` | |
See [documentation](https://huggingface.co/docs/accelerate/usage_guides/deepspeed) for more DeepSpeed configuration options. | |
Changing the default Adam optimizer to DeepSpeed's Adam | |
`deepspeed.ops.adam.DeepSpeedCPUAdam` gives a substantial speedup but | |
it requires CUDA toolchain with the same version as pytorch. 8-bit optimizer | |
does not seem to be compatible with DeepSpeed at the moment. | |
```bash | |
export MODEL_DIR="runwayml/stable-diffusion-v1-5" | |
export OUTPUT_DIR="path to save model" | |
accelerate launch train_controlnet.py \ | |
--pretrained_model_name_or_path=$MODEL_DIR \ | |
--output_dir=$OUTPUT_DIR \ | |
--dataset_name=fusing/fill50k \ | |
--resolution=512 \ | |
--validation_image "./conditioning_image_1.png" "./conditioning_image_2.png" \ | |
--validation_prompt "red circle with blue background" "cyan circle with brown floral background" \ | |
--train_batch_size=1 \ | |
--gradient_accumulation_steps=4 \ | |
--gradient_checkpointing \ | |
--enable_xformers_memory_efficient_attention \ | |
--set_grads_to_none \ | |
--mixed_precision fp16 | |
``` | |
## Performing inference with the trained ControlNet | |
The trained model can be run the same as the original ControlNet pipeline with the newly trained ControlNet. | |
Set `base_model_path` and `controlnet_path` to the values `--pretrained_model_name_or_path` and | |
`--output_dir` were respectively set to in the training script. | |
```py | |
from diffusers import StableDiffusionControlNetPipeline, ControlNetModel, UniPCMultistepScheduler | |
from diffusers.utils import load_image | |
import torch | |
base_model_path = "path to model" | |
controlnet_path = "path to controlnet" | |
controlnet = ControlNetModel.from_pretrained(controlnet_path, torch_dtype=torch.float16) | |
pipe = StableDiffusionControlNetPipeline.from_pretrained( | |
base_model_path, controlnet=controlnet, torch_dtype=torch.float16 | |
) | |
# speed up diffusion process with faster scheduler and memory optimization | |
pipe.scheduler = UniPCMultistepScheduler.from_config(pipe.scheduler.config) | |
# remove following line if xformers is not installed or when using Torch 2.0. | |
pipe.enable_xformers_memory_efficient_attention() | |
# memory optimization. | |
pipe.enable_model_cpu_offload() | |
control_image = load_image("./conditioning_image_1.png") | |
prompt = "pale golden rod circle with old lace background" | |
# generate image | |
generator = torch.manual_seed(0) | |
image = pipe( | |
prompt, num_inference_steps=20, generator=generator, image=control_image | |
).images[0] | |
image.save("./output.png") | |
``` | |
## Training with Flax/JAX | |
For faster training on TPUs and GPUs you can leverage the flax training example. Follow the instructions above to get the model and dataset before running the script. | |
### Running on Google Cloud TPU | |
See below for commands to set up a TPU VM(`--accelerator-type v4-8`). For more details about how to set up and use TPUs, refer to [Cloud docs for single VM setup](https://cloud.google.com/tpu/docs/run-calculation-jax). | |
First create a single TPUv4-8 VM and connect to it: | |
``` | |
ZONE=us-central2-b | |
TPU_TYPE=v4-8 | |
VM_NAME=hg_flax | |
gcloud alpha compute tpus tpu-vm create $VM_NAME \ | |
--zone $ZONE \ | |
--accelerator-type $TPU_TYPE \ | |
--version tpu-vm-v4-base | |
gcloud alpha compute tpus tpu-vm ssh $VM_NAME --zone $ZONE -- \ | |
``` | |
When connected install JAX `0.4.5`: | |
```sh | |
pip install "jax[tpu]==0.4.5" -f https://storage.googleapis.com/jax-releases/libtpu_releases.html | |
``` | |
To verify that JAX was correctly installed, you can run the following command: | |
```py | |
import jax | |
jax.device_count() | |
``` | |
This should display the number of TPU cores, which should be 4 on a TPUv4-8 VM. | |
Then install Diffusers and the library's training dependencies: | |
```bash | |
git clone https://github.com/huggingface/diffusers | |
cd diffusers | |
pip install . | |
``` | |
Then cd in the example folder and run | |
```bash | |
pip install -U -r requirements_flax.txt | |
``` | |
If you want to use Weights and Biases logging, you should also install `wandb` now | |
```bash | |
pip install wandb | |
``` | |
Now let's downloading two conditioning images that we will use to run validation during the training in order to track our progress | |
```sh | |
wget https://huggingface.co/datasets/huggingface/documentation-images/resolve/main/diffusers/controlnet_training/conditioning_image_1.png | |
wget https://huggingface.co/datasets/huggingface/documentation-images/resolve/main/diffusers/controlnet_training/conditioning_image_2.png | |
``` | |
We encourage you to store or share your model with the community. To use huggingface hub, please login to your Hugging Face account, or ([create one](https://huggingface.co/docs/diffusers/main/en/training/hf.co/join) if you don’t have one already): | |
```sh | |
huggingface-cli login | |
``` | |
Make sure you have the `MODEL_DIR`,`OUTPUT_DIR` and `HUB_MODEL_ID` environment variables set. The `OUTPUT_DIR` and `HUB_MODEL_ID` variables specify where to save the model to on the Hub: | |
```bash | |
export MODEL_DIR="runwayml/stable-diffusion-v1-5" | |
export OUTPUT_DIR="runs/fill-circle-{timestamp}" | |
export HUB_MODEL_ID="controlnet-fill-circle" | |
``` | |
And finally start the training | |
```bash | |
python3 train_controlnet_flax.py \ | |
--pretrained_model_name_or_path=$MODEL_DIR \ | |
--output_dir=$OUTPUT_DIR \ | |
--dataset_name=fusing/fill50k \ | |
--resolution=512 \ | |
--learning_rate=1e-5 \ | |
--validation_image "./conditioning_image_1.png" "./conditioning_image_2.png" \ | |
--validation_prompt "red circle with blue background" "cyan circle with brown floral background" \ | |
--validation_steps=1000 \ | |
--train_batch_size=2 \ | |
--revision="non-ema" \ | |
--from_pt \ | |
--report_to="wandb" \ | |
--tracker_project_name=$HUB_MODEL_ID \ | |
--num_train_epochs=11 \ | |
--push_to_hub \ | |
--hub_model_id=$HUB_MODEL_ID | |
``` | |
Since we passed the `--push_to_hub` flag, it will automatically create a model repo under your huggingface account based on `$HUB_MODEL_ID`. By the end of training, the final checkpoint will be automatically stored on the hub. You can find an example model repo [here](https://huggingface.co/YiYiXu/fill-circle-controlnet). | |
Our training script also provides limited support for streaming large datasets from the Hugging Face Hub. In order to enable streaming, one must also set `--max_train_samples`. Here is an example command (from [this blog article](https://huggingface.co/blog/train-your-controlnet)): | |
```bash | |
export MODEL_DIR="runwayml/stable-diffusion-v1-5" | |
export OUTPUT_DIR="runs/uncanny-faces-{timestamp}" | |
export HUB_MODEL_ID="controlnet-uncanny-faces" | |
python3 train_controlnet_flax.py \ | |
--pretrained_model_name_or_path=$MODEL_DIR \ | |
--output_dir=$OUTPUT_DIR \ | |
--dataset_name=multimodalart/facesyntheticsspigacaptioned \ | |
--streaming \ | |
--conditioning_image_column=spiga_seg \ | |
--image_column=image \ | |
--caption_column=image_caption \ | |
--resolution=512 \ | |
--max_train_samples 100000 \ | |
--learning_rate=1e-5 \ | |
--train_batch_size=1 \ | |
--revision="flax" \ | |
--report_to="wandb" \ | |
--tracker_project_name=$HUB_MODEL_ID | |
``` | |
Note, however, that the performance of the TPUs might get bottlenecked as streaming with `datasets` is not optimized for images. For ensuring maximum throughput, we encourage you to explore the following options: | |
* [Webdataset](https://webdataset.github.io/webdataset/) | |
* [TorchData](https://github.com/pytorch/data) | |
* [TensorFlow Datasets](https://www.tensorflow.org/datasets/tfless_tfds) | |
When work with a larger dataset, you may need to run training process for a long time and it’s useful to save regular checkpoints during the process. You can use the following argument to enable intermediate checkpointing: | |
```bash | |
--checkpointing_steps=500 | |
``` | |
This will save the trained model in subfolders of your output_dir. Subfolder names is the number of steps performed so far; for example: a checkpoint saved after 500 training steps would be saved in a subfolder named 500 | |
You can then start your training from this saved checkpoint with | |
```bash | |
--controlnet_model_name_or_path="./control_out/500" | |
``` | |
We support training with the Min-SNR weighting strategy proposed in [Efficient Diffusion Training via Min-SNR Weighting Strategy](https://arxiv.org/abs/2303.09556) which helps to achieve faster convergence by rebalancing the loss. To use it, one needs to set the `--snr_gamma` argument. The recommended value when using it is `5.0`. | |
We also support gradient accumulation - it is a technique that lets you use a bigger batch size than your machine would normally be able to fit into memory. You can use `gradient_accumulation_steps` argument to set gradient accumulation steps. The ControlNet author recommends using gradient accumulation to achieve better convergence. Read more [here](https://github.com/lllyasviel/ControlNet/blob/main/docs/train.md#more-consideration-sudden-converge-phenomenon-and-gradient-accumulation). | |
You can **profile your code** with: | |
```bash | |
--profile_steps==5 | |
``` | |
Refer to the [JAX documentation on profiling](https://jax.readthedocs.io/en/latest/profiling.html). To inspect the profile trace, you'll have to install and start Tensorboard with the profile plugin: | |
```bash | |
pip install tensorflow tensorboard-plugin-profile | |
tensorboard --logdir runs/fill-circle-100steps-20230411_165612/ | |
``` | |
The profile can then be inspected at http://localhost:6006/#profile | |
Sometimes you'll get version conflicts (error messages like `Duplicate plugins for name projector`), which means that you have to uninstall and reinstall all versions of Tensorflow/Tensorboard (e.g. with `pip uninstall tensorflow tf-nightly tensorboard tb-nightly tensorboard-plugin-profile && pip install tf-nightly tbp-nightly tensorboard-plugin-profile`). | |
Note that the debugging functionality of the Tensorboard `profile` plugin is still under active development. Not all views are fully functional, and for example the `trace_viewer` cuts off events after 1M (which can result in all your device traces getting lost if you for example profile the compilation step by accident). | |
## Support for Stable Diffusion XL | |
We provide a training script for training a ControlNet with [Stable Diffusion XL](https://huggingface.co/papers/2307.01952). Please refer to [README_sdxl.md](./README_sdxl.md) for more details. | |