A newer version of the Gradio SDK is available:
5.9.1
Leveraging Content-based Features from Multiple Acoustic Models for Singing Voice Conversion
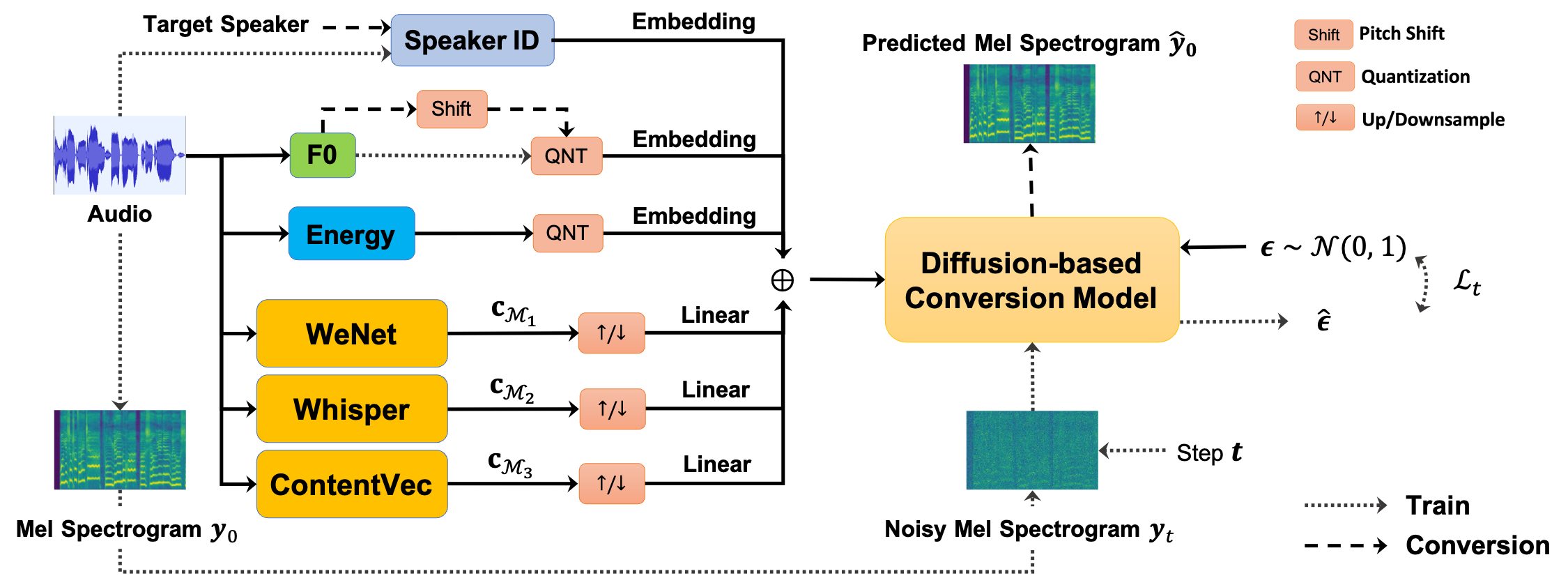
This is the official implementation of the paper "Leveraging Diverse Semantic-based Audio Pretrained Models for Singing Voice Conversion" (2024 IEEE Spoken Language Technology Workshop). Specially,
- The muptile content features are from Whipser and ContentVec.
- The acoustic model is based on Bidirectional Non-Causal Dilated CNN (called
DiffWaveNetSVC
in Amphion), which is similar to WaveNet, DiffWave, and DiffSVC. - The vocoder is BigVGAN architecture and we fine-tuned it in over 120 hours singing voice data.
A Little Taste Before Getting Started
Before you delve into the code, we suggest exploring the interactive DEMO we've provided for a comprehensive overview. There are several ways you can engage with it:
Online DEMO
Run Local Gradio DEMO
Run with the Extended Colab
You can check out this repo to run it with Colab. Thanks to @camenduru and the community for their support!
Usage Overview
To train a DiffWaveNetSVC
model, there are four stages in total:
- Data preparation
- Features extraction
- Training
- Inference/conversion
NOTE: You need to run every command of this recipe in the
Amphion
root path:
cd Amphion
1. Data Preparation
Dataset Download
By default, we utilize the five datasets for training: M4Singer, Opencpop, OpenSinger, SVCC, and VCTK. How to download them is detailed here.
Configuration
Specify the dataset paths in exp_config.json
. Note that you can change the dataset
list to use your preferred datasets.
"dataset": [
"m4singer",
"opencpop",
"opensinger",
"svcc",
"vctk"
],
"dataset_path": {
// TODO: Fill in your dataset path
"m4singer": "[M4Singer dataset path]",
"opencpop": "[Opencpop dataset path]",
"opensinger": "[OpenSinger dataset path]",
"svcc": "[SVCC dataset path]",
"vctk": "[VCTK dataset path]"
},
Custom Dataset
We support custom dataset, see here for the file structure to follow.
After constructing proper file structure, specify your dataset name in dataset
and its path in dataset_path
, also add its name in use_custom_dataset
:
"dataset": [
"[Exisiting Dataset Name]",
//...
"[Your Custom Dataset Name]"
],
"dataset_path": {
"[Exisiting Dataset Name]": "[Exisiting Dataset Path]",
//...
"[Your Custom Dataset Name]": "[Your Custom Dataset Path]"
},
"use_custom_dataset": [
"[Your Custom Dataset Name]"
],
NOTE: Custom dataset name does not have to be the same as the folder name. But it needs to satisfy these rules:
- It can not be the same as the exisiting dataset name.
- It can not contain any space or underline(
_
).- It must be a valid folder name for operating system.
Some examples of valid custom dataset names are
mydataset
,myDataset
,my-dataset
,mydataset1
,my-dataset-1
, etc.
2. Features Extraction
Content-based Pretrained Models Download
By default, we utilize the Whisper and ContentVec to extract content features. How to download them is detailed here.
Configuration
Specify the dataset path and the output path for saving the processed data and the training model in exp_config.json
:
// TODO: Fill in the output log path. The default value is "Amphion/ckpts/svc"
"log_dir": "ckpts/svc",
"preprocess": {
// TODO: Fill in the output data path. The default value is "Amphion/data"
"processed_dir": "data",
...
},
Run
Run the run.sh
as the preproces stage (set --stage 1
).
sh egs/svc/MultipleContentsSVC/run.sh --stage 1
NOTE: The
CUDA_VISIBLE_DEVICES
is set as"0"
in default. You can change it when runningrun.sh
by specifying such as--gpu "1"
.
3. Training
Configuration
We provide the default hyparameters in the exp_config.json
. They can work on single NVIDIA-24g GPU. You can adjust them based on you GPU machines.
"train": {
"batch_size": 32,
...
"adamw": {
"lr": 2.0e-4
},
...
}
Train From Scratch
Run the run.sh
as the training stage (set --stage 2
). Specify a experimental name to run the following command. The tensorboard logs and checkpoints will be saved in Amphion/ckpts/svc/[YourExptName]
.
sh egs/svc/MultipleContentsSVC/run.sh --stage 2 --name [YourExptName]
Train From Existing Source
We support training from existing source for various purposes. You can resume training the model from a checkpoint or fine-tune a model from another checkpoint.
Setting --resume true
, the training will resume from the latest checkpoint by default. For example, if you want to resume training from the latest checkpoint in Amphion/ckpts/svc/[YourExptName]/checkpoint
, run:
sh egs/svc/MultipleContentsSVC/run.sh --stage 2 --name [YourExptName] \
--resume true
You can choose a specific checkpoint for retraining by --resume_from_ckpt_path
argument. For example, if you want to fine-tune from the checkpoint Amphion/ckpts/svc/[YourExptName]/checkpoint/[SpecificCheckpoint]
, run:
sh egs/svc/MultipleContentsSVC/run.sh --stage 2 --name [YourExptName] \
--resume true
--resume_from_ckpt_path "Amphion/ckpts/svc/[YourExptName]/checkpoint/[SpecificCheckpoint]" \
If you want to fine-tune from another checkpoint, just use --resume_type
and set it to "finetune"
. For example, If you want to fine-tune from the checkpoint Amphion/ckpts/svc/[AnotherExperiment]/checkpoint/[SpecificCheckpoint]
, run:
sh egs/svc/MultipleContentsSVC/run.sh --stage 2 --name [YourExptName] \
--resume true
--resume_from_ckpt_path "Amphion/ckpts/svc/[AnotherExperiment]/checkpoint/[SpecificCheckpoint]" \
--resume_type "finetune"
NOTE: The
--resume_type
is set as"resume"
in default. It's not necessary to specify it when resuming training.The difference between
"resume"
and"finetune"
is that the"finetune"
will only load the pretrained model weights from the checkpoint, while the"resume"
will load all the training states (including optimizer, scheduler, etc.) from the checkpoint.
Here are some example scenarios to better understand how to use these arguments:
Scenario | --resume |
--resume_from_ckpt_path |
--resume_type |
---|---|---|---|
You want to train from scratch | no | no | no |
The machine breaks down during training and you want to resume training from the latest checkpoint | true |
no | no |
You find the latest model is overfitting and you want to re-train from the checkpoint before | true |
SpecificCheckpoint Path |
no |
You want to fine-tune a model from another checkpoint | true |
SpecificCheckpoint Path |
"finetune" |
NOTE: The
CUDA_VISIBLE_DEVICES
is set as"0"
in default. You can change it when runningrun.sh
by specifying such as--gpu "0,1,2,3"
.
4. Inference/Conversion
Pretrained Vocoder Download
We fine-tune the official BigVGAN pretrained model with over 120 hours singing voice data. The benifits of fine-tuning has been investigated in our paper (see this demo page). The final pretrained singing voice vocoder is released here (called Amphion Singing BigVGAN
).
Run
For inference/conversion, you need to specify the following configurations when running run.sh
:
Parameters | Description | Example |
---|---|---|
--infer_expt_dir |
The experimental directory which contains checkpoint |
Amphion/ckpts/svc/[YourExptName] |
--infer_output_dir |
The output directory to save inferred audios. | Amphion/ckpts/svc/[YourExptName]/result |
--infer_source_file or --infer_source_audio_dir |
The inference source (can be a json file or a dir). | The infer_source_file could be Amphion/data/[YourDataset]/test.json , and the infer_source_audio_dir is a folder which includes several audio files (*.wav, *.mp3 or *.flac). |
--infer_target_speaker |
The target speaker you want to convert into. You can refer to Amphion/ckpts/svc/[YourExptName]/singers.json to choose a trained speaker. |
For opencpop dataset, the speaker name would be opencpop_female1 . |
--infer_key_shift |
How many semitones you want to transpose. | "autoshfit" (by default), 3 , -3 , etc. |
For example, if you want to make opencpop_female1
sing the songs in the [Your Audios Folder]
, just run:
sh egs/svc/MultipleContentsSVC/run.sh --stage 3 --gpu "0" \
--infer_expt_dir ckpts/svc/[YourExptName] \
--infer_output_dir ckpts/svc/[YourExptName]/result \
--infer_source_audio_dir [Your Audios Folder] \
--infer_target_speaker "opencpop_female1" \
--infer_key_shift "autoshift"
Citations
@inproceedings{zhang2024leveraging,
author={Zhang, Xueyao and Fang, Zihao and Gu, Yicheng and Chen, Haopeng and Zou, Lexiao and Zhang, Junan and Xue, Liumeng and Wu, Zhizheng},
title={Leveraging Diverse Semantic-based Audio Pretrained Models for Singing Voice Conversion},
booktitle={{IEEE} Spoken Language Technology Workshop, {SLT} 2024},
year={2024}
}