{}
Kosmos-2: Grounding Multimodal Large Language Models to the World
(There is an on going effort to port Kosmos-2
directly into transformers
. This repository (remote code) might need some more bug fixes later, including breaking changes.)
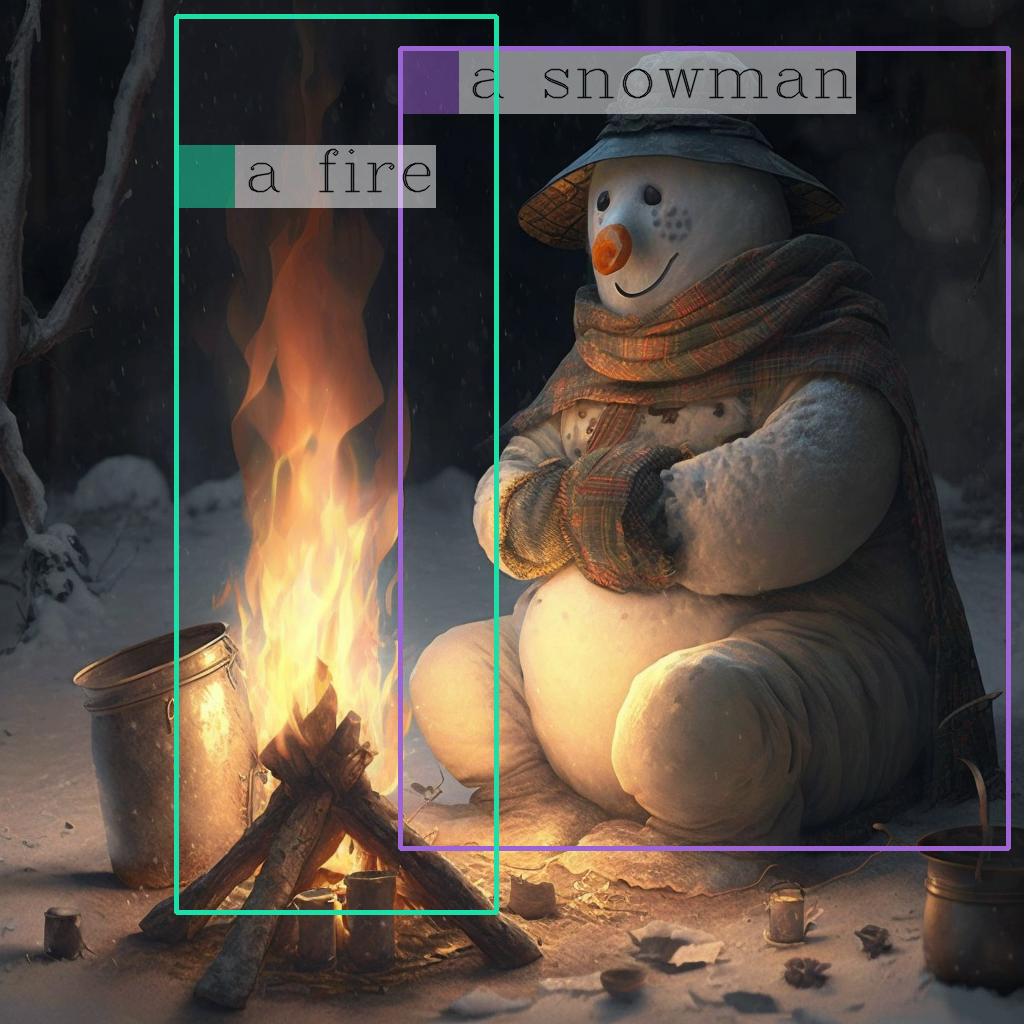
This Hub repository contains a HuggingFace's transformers
implementation of the original Kosmos-2 model from Microsoft.
How to Get Started with the Model
Use the code below to get started with the model.
import requests
from PIL import Image
from transformers import AutoProcessor, AutoModelForVision2Seq
model = AutoModelForVision2Seq.from_pretrained("ydshieh/kosmos-2-patch14-224", trust_remote_code=True)
processor = AutoProcessor.from_pretrained("ydshieh/kosmos-2-patch14-224", trust_remote_code=True)
prompt = "<grounding>An image of"
url = "https://huggingface.co/ydshieh/kosmos-2-patch14-224/resolve/main/snowman.png"
image = Image.open(requests.get(url, stream=True).raw)
# The original Kosmos-2 demo saves the image first then reload it. For some images, this will give slightly different image input and change the generation outputs.
# Uncomment the following 2 lines if you want to match the original demo's outputs.
# (One example is the `two_dogs.jpg` from the demo)
# image.save("new_image.jpg")
# image = Image.open("new_image.jpg")
inputs = processor(text=prompt, images=image, return_tensors="pt")
generated_ids = model.generate(
pixel_values=inputs["pixel_values"],
input_ids=inputs["input_ids"][:, :-1],
attention_mask=inputs["attention_mask"][:, :-1],
img_features=None,
img_attn_mask=inputs["img_attn_mask"][:, :-1],
use_cache=True,
max_new_tokens=64,
)
generated_text = processor.batch_decode(generated_ids, skip_special_tokens=True)[0]
# Specify `cleanup_and_extract=False` in order to see the raw model generation.
processed_text = processor.post_process_generation(generated_text, cleanup_and_extract=False)
print(processed_text)
# `<grounding> An image of<phrase> a snowman</phrase><object><patch_index_0044><patch_index_0863></object> warming himself by<phrase> a fire</phrase><object><patch_index_0005><patch_index_0911></object>.`
# By default, the generated text is cleanup and the entities are extracted.
processed_text, entities = processor.post_process_generation(generated_text)
print(processed_text)
# `An image of a snowman warming himself by a fire.`
print(entities)
# `[('a snowman', (12, 21), [(0.390625, 0.046875, 0.984375, 0.828125)]), ('a fire', (41, 47), [(0.171875, 0.015625, 0.484375, 0.890625)])]`
Draw the bounding bboxes of the entities on the image
Once you have the entities
, you can use the following helper function to draw their bounding bboxes on the image:
import cv2
import numpy as np
import os
import requests
import torch
import torchvision.transforms as T
from PIL import Image
def is_overlapping(rect1, rect2):
x1, y1, x2, y2 = rect1
x3, y3, x4, y4 = rect2
return not (x2 < x3 or x1 > x4 or y2 < y3 or y1 > y4)
def draw_entity_boxes_on_image(image, entities, show=False, save_path=None):
"""_summary_
Args:
image (_type_): image or image path
collect_entity_location (_type_): _description_
"""
if isinstance(image, Image.Image):
image_h = image.height
image_w = image.width
image = np.array(image)[:, :, [2, 1, 0]]
elif isinstance(image, str):
if os.path.exists(image):
pil_img = Image.open(image).convert("RGB")
image = np.array(pil_img)[:, :, [2, 1, 0]]
image_h = pil_img.height
image_w = pil_img.width
else:
raise ValueError(f"invaild image path, {image}")
elif isinstance(image, torch.Tensor):
# pdb.set_trace()
image_tensor = image.cpu()
reverse_norm_mean = torch.tensor([0.48145466, 0.4578275, 0.40821073])[:, None, None]
reverse_norm_std = torch.tensor([0.26862954, 0.26130258, 0.27577711])[:, None, None]
image_tensor = image_tensor * reverse_norm_std + reverse_norm_mean
pil_img = T.ToPILImage()(image_tensor)
image_h = pil_img.height
image_w = pil_img.width
image = np.array(pil_img)[:, :, [2, 1, 0]]
else:
raise ValueError(f"invaild image format, {type(image)} for {image}")
if len(entities) == 0:
return image
new_image = image.copy()
previous_bboxes = []
# size of text
text_size = 1
# thickness of text
text_line = 1 # int(max(1 * min(image_h, image_w) / 512, 1))
box_line = 3
(c_width, text_height), _ = cv2.getTextSize("F", cv2.FONT_HERSHEY_COMPLEX, text_size, text_line)
base_height = int(text_height * 0.675)
text_offset_original = text_height - base_height
text_spaces = 3
for entity_name, (start, end), bboxes in entities:
for (x1_norm, y1_norm, x2_norm, y2_norm) in bboxes:
orig_x1, orig_y1, orig_x2, orig_y2 = int(x1_norm * image_w), int(y1_norm * image_h), int(x2_norm * image_w), int(y2_norm * image_h)
# draw bbox
# random color
color = tuple(np.random.randint(0, 255, size=3).tolist())
new_image = cv2.rectangle(new_image, (orig_x1, orig_y1), (orig_x2, orig_y2), color, box_line)
l_o, r_o = box_line // 2 + box_line % 2, box_line // 2 + box_line % 2 + 1
x1 = orig_x1 - l_o
y1 = orig_y1 - l_o
if y1 < text_height + text_offset_original + 2 * text_spaces:
y1 = orig_y1 + r_o + text_height + text_offset_original + 2 * text_spaces
x1 = orig_x1 + r_o
# add text background
(text_width, text_height), _ = cv2.getTextSize(f" {entity_name}", cv2.FONT_HERSHEY_COMPLEX, text_size, text_line)
text_bg_x1, text_bg_y1, text_bg_x2, text_bg_y2 = x1, y1 - (text_height + text_offset_original + 2 * text_spaces), x1 + text_width, y1
for prev_bbox in previous_bboxes:
while is_overlapping((text_bg_x1, text_bg_y1, text_bg_x2, text_bg_y2), prev_bbox):
text_bg_y1 += (text_height + text_offset_original + 2 * text_spaces)
text_bg_y2 += (text_height + text_offset_original + 2 * text_spaces)
y1 += (text_height + text_offset_original + 2 * text_spaces)
if text_bg_y2 >= image_h:
text_bg_y1 = max(0, image_h - (text_height + text_offset_original + 2 * text_spaces))
text_bg_y2 = image_h
y1 = image_h
break
alpha = 0.5
for i in range(text_bg_y1, text_bg_y2):
for j in range(text_bg_x1, text_bg_x2):
if i < image_h and j < image_w:
if j < text_bg_x1 + 1.35 * c_width:
# original color
bg_color = color
else:
# white
bg_color = [255, 255, 255]
new_image[i, j] = (alpha * new_image[i, j] + (1 - alpha) * np.array(bg_color)).astype(np.uint8)
cv2.putText(
new_image, f" {entity_name}", (x1, y1 - text_offset_original - 1 * text_spaces), cv2.FONT_HERSHEY_COMPLEX, text_size, (0, 0, 0), text_line, cv2.LINE_AA
)
# previous_locations.append((x1, y1))
previous_bboxes.append((text_bg_x1, text_bg_y1, text_bg_x2, text_bg_y2))
pil_image = Image.fromarray(new_image[:, :, [2, 1, 0]])
if save_path:
pil_image.save(save_path)
if show:
pil_image.show()
return new_image
# (The same image from the previous code example)
url = "https://huggingface.co/ydshieh/kosmos-2-patch14-224/resolve/main/snowman.jpg"
image = Image.open(requests.get(url, stream=True).raw)
# From the previous code example
entities = [('a snowman', (12, 21), [(0.390625, 0.046875, 0.984375, 0.828125)]), ('a fire', (41, 47), [(0.171875, 0.015625, 0.484375, 0.890625)])]
# Draw the bounding bboxes
draw_entity_boxes_on_image(image, entities, show=True)
Here is the annotated image:
Tasks
This model is capable of performing different tasks through changing the prompts.
First, let's define a function to run a prompt.
import requests
from PIL import Image
from transformers import AutoProcessor, AutoModelForVision2Seq
model = AutoModelForVision2Seq.from_pretrained("ydshieh/kosmos-2-patch14-224", trust_remote_code=True)
processor = AutoProcessor.from_pretrained("ydshieh/kosmos-2-patch14-224", trust_remote_code=True)
url = "https://huggingface.co/ydshieh/kosmos-2-patch14-224/resolve/main/snowman.png"
image = Image.open(requests.get(url, stream=True).raw)
def run_example(prompt):
inputs = processor(text=prompt, images=image, return_tensors="pt")
generated_ids = model.generate(
pixel_values=inputs["pixel_values"],
input_ids=inputs["input_ids"][:, :-1],
attention_mask=inputs["attention_mask"][:, :-1],
img_features=None,
img_attn_mask=inputs["img_attn_mask"][:, :-1],
use_cache=True,
max_new_tokens=64,
)
generated_text = processor.batch_decode(generated_ids, skip_special_tokens=True)[0]
_processed_text = processor.post_process_generation(generated_text, cleanup_and_extract=False)
processed_text, entities = processor.post_process_generation(generated_text)
print(processed_text)
print(entities)
print(_processed_text)
Here are the tasks Kosmos-2
could perform:
Multimodal Grounding
• Phrase Grounding
prompt = "<grounding><phrase> a snowman</phrase>"
run_example(prompt)
# a snowman is warming himself by the fire
# [('a snowman', (0, 9), [(0.390625, 0.046875, 0.984375, 0.828125)]), ('the fire', (32, 40), [(0.203125, 0.015625, 0.453125, 0.859375)])]
# <grounding><phrase> a snowman</phrase><object><patch_index_0044><patch_index_0863></object> is warming himself by<phrase> the fire</phrase><object><patch_index_0006><patch_index_0878></object>
• Referring Expression Comprehension
prompt = "<grounding><phrase> a snowman next to a fire</phrase>"
run_example(prompt)
# a snowman next to a fire
# [('a snowman next to a fire', (0, 24), [(0.390625, 0.046875, 0.984375, 0.828125)])]
# <grounding><phrase> a snowman next to a fire</phrase><object><patch_index_0044><patch_index_0863></object>
Multimodal Referring
• Referring expression generation
prompt = "<grounding><phrase> It</phrase><object><patch_index_0044><patch_index_0863></object> is"
run_example(prompt)
# It is snowman in a hat and scarf
# [('It', (0, 2), [(0.390625, 0.046875, 0.984375, 0.828125)])]
# <grounding><phrase> It</phrase><object><patch_index_0044><patch_index_0863></object> is snowman in a hat and scarf
Perception-Language Tasks
• Grounded VQA
prompt = "<grounding> Question: What is special about this image? Answer:"
run_example(prompt)
# Question: What is special about this image? Answer: The image features a snowman sitting by a campfire in the snow.
# [('a snowman', (71, 80), [(0.390625, 0.046875, 0.984375, 0.828125)]), ('a campfire', (92, 102), [(0.109375, 0.640625, 0.546875, 0.984375)])]
# <grounding> Question: What is special about this image? Answer: The image features<phrase> a snowman</phrase><object><patch_index_0044><patch_index_0863></object> sitting by<phrase> a campfire</phrase><object><patch_index_0643><patch_index_1009></object> in the snow.
• Grounded VQA with multimodal referring via bounding boxes
prompt = "<grounding> Question: Where is<phrase> the fire</phrase><object><patch_index_0005><patch_index_0911></object> next to? Answer:"
run_example(prompt)
# Question: Where is the fire next to? Answer: Near the snowman.
# [('the fire', (19, 27), [(0.171875, 0.015625, 0.484375, 0.890625)]), ('the snowman', (50, 61), [(0.390625, 0.046875, 0.984375, 0.828125)])]
# <grounding> Question: Where is<phrase> the fire</phrase><object><patch_index_0005><patch_index_0911></object> next to? Answer: Near<phrase> the snowman</phrase><object><patch_index_0044><patch_index_0863></object>.
Grounded Image captioning
• Brief
prompt = "<grounding> An image of"
run_example(prompt)
# An image of a snowman warming himself by a campfire.
# [('a snowman', (12, 21), [(0.390625, 0.046875, 0.984375, 0.828125)]), ('a campfire', (41, 51), [(0.109375, 0.640625, 0.546875, 0.984375)])]
# <grounding> An image of<phrase> a snowman</phrase><object><patch_index_0044><patch_index_0863></object> warming himself by<phrase> a campfire</phrase><object><patch_index_0643><patch_index_1009></object>.
• Detailed
prompt = "<grounding> Describe this image in detail:"
run_example(prompt)
# Describe this image in detail: The image features a snowman sitting by a campfire in the snow. He is wearing a hat, scarf, and gloves, with a pot nearby and a cup
# [('a campfire', (71, 81), [(0.171875, 0.015625, 0.484375, 0.984375)]), ('a hat', (109, 114), [(0.515625, 0.046875, 0.828125, 0.234375)]), ('scarf', (116, 121), [(0.515625, 0.234375, 0.890625, 0.578125)]), ('gloves', (127, 133), [(0.515625, 0.390625, 0.640625, 0.515625)]), ('a pot', (140, 145), [(0.078125, 0.609375, 0.265625, 0.859375)])]
# <grounding> Describe this image in detail: The image features a snowman sitting by<phrase> a campfire</phrase><object><patch_index_0005><patch_index_1007></object> in the snow. He is wearing<phrase> a hat</phrase><object><patch_index_0048><patch_index_0250></object>,<phrase> scarf</phrase><object><patch_index_0240><patch_index_0604></object>, and<phrase> gloves</phrase><object><patch_index_0400><patch_index_0532></object>, with<phrase> a pot</phrase><object><patch_index_0610><patch_index_0872></object> nearby and<phrase> a cup</phrase><object>
Running the Flask Server
flask_kosmos2.py shows the implementation of a Flask server for the model. It allowes the model to be approached as a REST API.
After starting the server. You can send a POST request to http://localhost:8005/process_prompt
with the following form data:
prompt
: For example<grounding> an image of
image
: The image file as binary data
This in turn will produce a reply with the following JSON format:
message
: The Kosmos-2 generated textentities
: The extracted entities
An easy way to test this is through an application like Postman. Make sure the image field is set to File
.
from PIL import Image
from transformers import AutoProcessor, AutoModelForVision2Seq
from flask import Flask, request, jsonify
import json
app = Flask(__name__)
model = AutoModelForVision2Seq.from_pretrained("ydshieh/kosmos-2-patch14-224", trust_remote_code=True)
processor = AutoProcessor.from_pretrained("ydshieh/kosmos-2-patch14-224", trust_remote_code=True)
@app.route('/process_prompt', methods=['POST'])
def process_prompt():
try:
# Get the uploaded image data from the POST request
uploaded_file = request.files['image']
prompt = request.form.get('prompt')
image = Image.open(uploaded_file.stream)
print(image.size)
inputs = processor(text=prompt, images=image, return_tensors="pt")
generated_ids = model.generate(
pixel_values=inputs["pixel_values"],
input_ids=inputs["input_ids"][:, :-1],
attention_mask=inputs["attention_mask"][:, :-1],
img_features=None,
img_attn_mask=inputs["img_attn_mask"][:, :-1],
use_cache=True,
max_new_tokens=64,
)
generated_text = processor.batch_decode(generated_ids, skip_special_tokens=True)[0]
# By default, the generated text is cleanup and the entities are extracted.
processed_text, entities = processor.post_process_generation(generated_text)
parsed_entities = entities_to_json(entities)
print(generated_text)
print(processed_text)
return jsonify({"message": processed_text, 'entities': parsed_entities})
except Exception as e:
return jsonify({"error": str(e)})
def entities_to_json(entities):
result = []
for e in entities:
label = e[0]
box_coords = e[1]
box_size = e[2][0]
entity_result = {
"label": label,
"boundingBoxPosition": {"x": box_coords[0], "y": box_coords[1]},
"boundingBox": {"x_min": box_size[0], "y_min": box_size[1], "x_max": box_size[2], "y_max": box_size[3]}
}
print(entity_result)
result.append(entity_result)
return result
if __name__ == '__main__':
app.run(host='localhost', port=8005)