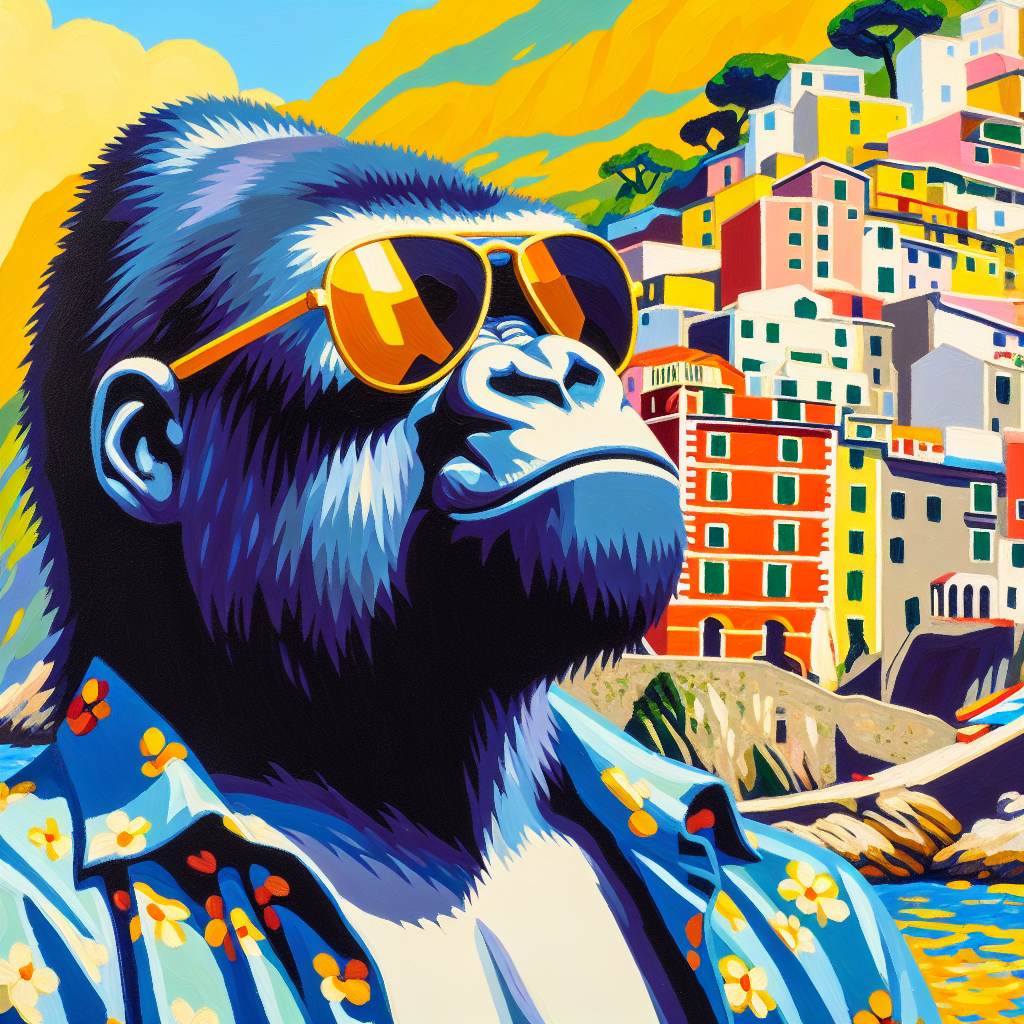
Introduction
Zefiro functioncalling extends Large Language Model(LLM) Chat Completion feature to formulate executable APIs call given Italian based natural language instructions and API context. With OpenFunctions v2,
we now support:
- Relevance detection - when chatting, chat. When asked for function, returns a function
- REST - native REST support
Model description
- Model type: A 7B parameter GPT-like model fine-tuned on a mix of publicly available, synthetic datasets.
- Language(s) (NLP): Primarily Italian
- License: Apache 2
- Finetuned from model: gorilla-llm
- Developed by: zefiro.ai
- Sponsored by: Seeweb
Models Available
Model | Functionality |
---|---|
zefiro-funcioncalling-v0.3-alpha | Given a function, and user intent, returns properly formatted json with the right arguments |
All of our models are hosted on our Huggingface mii-community org: zefiro-functioncalling-v0.3-alpha.
Training
Zefiro functioncalling alpha is a 7B parameter model, and is fine tuned version of gorilla-llm that is built on top of the deepseek coder LLM.
Example Usage (Local)
- OpenFunctions is compatible with OpenAI Functions
!pip install openai==0.28.1, transformers
- Load the model
from transformers import AutoModelForCausalLM, AutoTokenizer
model_id = "mii-community/zefiro-functioncalling-v0.3-alpha"
model = AutoModelForCausalLM.from_pretrained(model_id)
model.to('cuda')
tokenizer = AutoTokenizer.from_pretrained(model_id)
- Prepare your data with a system prompt and an array of json openapi compatible: only the description key should be in Italian all the json in english a part all description keys.
json_arr = [{"name": "order_dinner", "description": "Ordina una cena al ristorante", "parameters": {"type": "object", "properties": {"restaurant_name": {"type": "string", "description": "il nome del ristorante", "enum" : ['Bufalo Bill','Pazzas']}}, "required": ["restaurant_name"]}},
{"name": "get_weather", "description": "Ottieni le previsioni del tempo meteorologica", "parameters": {"type": "object", "properties": {"location": {"type": "string", "description": "Il nome del luogo "}}, "required": ["location"]}},
{"name": "create_product", "description": "Crea un prodotto da vendere", "parameters": {"type": "object", "properties": {"product_name": {"type": "string", "description": "Il nome del prodotto "}, "size": {"type": "string", "description": "la taglia del prodotto"}, "price": {"type": "integer", "description": "Il prezzo del prodotto "}}, "required": ["product_name", "size", "price"]}},
{"name": "get_news", "description": "Dammi le ultime notizie", "parameters": {"type": "object", "properties": {"argument": {"type": "string", "description": "L'argomento su cui fare la ricerca"}}, "required": ["argument"]}},
]
json_string = ' '.join([json.dumps(json_obj) for json_obj in json_arr])
system_prompt = 'Tu sei un assistenze utile che ha accesso alle seguenti funzioni. Usa le funzioni solo se necessario - \n ' + json_string + ' \n '
print(system_prompt)
test_message = [{'role' : 'system' , 'content' : system_prompt2},
{'role' : 'user' ,'content' : 'Crea un prodotto di nome AIR size L price 100'}]
- Call the model
def generate_text():
prompt = tokenizer.apply_chat_template(test_message, tokenize=False)
model_inputs = tokenizer([prompt], return_tensors="pt").to("cuda")
generated_ids = model.generate(**model_inputs, max_new_tokens=1024)
return tokenizer.batch_decode(generated_ids, skip_special_tokens=True)[0]
text_response = generate_text()
- Parse the response
FN_CALL_DELIMITER = "<<functioncall>>"
def strip_function_calls(content: str) -> list[str]:
"""
Split the content by the function call delimiter and remove empty strings
"""
return [element.replace('\n', '') for element in content.split(FN_CALL_DELIMITER)[1:] if element ]
functions_string = strip_function_calls(text_response)
# Output: [' {"name": "create_product", "arguments": \'{"product_name": "AIR", "size": "L", "price": 100}\'}']
- Create an object representation of the string
# if functions_string contains a function string create a json cleaning
# multiple functions not supported yet
if functions_string:
obj_to_call = json.loads(functions_string[0].replace('\'', ''))
else:
print('nothing to do or return a normal chat response')
# Output: {'name': 'create_product', 'arguments': {'product_name': 'AIR', 'size': 'L', 'price': 100}}
- Prepare data to be OpenAI compatible
def obj_to_func(obj):
arguments_keys = obj['arguments'].keys()
params = []
for key in arguments_keys:
param = f'{key}=\"{obj["arguments"][key]}\"'
params.append(param)
func_params = ','.join(params)
print(f'{obj["name"]}({func_params})')
return f'{obj["name"]}({func_params})'
func_str = obj_to_func(obj_to_call)
openai_response = {
"index": 0,
"message": {
"role": "assistant",
"content": func_str,
"function_call": [
obj_to_call
]
},
"finish_reason": "stop"
}
'''
Output OpenAI compatible Dictionary
{'index': 0,
'message': {
'role': 'assistant',
'content': 'create_product(product_name="AIR",size="L",price="100")',
'function_call': [{'name': 'create_product', 'arguments': {'product_name': 'AIR', 'size': 'L', 'price': 100}}]
},
'finish_reason': 'stop'
}
'''
JSON to be OpenAI compatible.
Limitation
The model has some bug and some unexpected behaviour for example the more json you pass the less accurate it become filling the json output but the interesting thing is that those are pattern that i did not consider in the data. It will be enough to improove the cases in the data to fix the bugs. Stay tuned for a better version soon.
License
Zefiro-functioncalling is distributed under the Apache 2.0 license as the base model Gorilla-LLM v0.2. This software incorporates elements from the Deepseek model. Consequently, the licensing of Gorilla OpenFunctions v2 adheres to the Apache 2.0 license, with additional terms as outlined in Appendix A of the Deepseek license.
Contributing
Please email us your comments, criticism, and questions. More information about the project can be found at https://zefiro.ai
Citation
This work is based on Gorilla an open source effort from UC Berkeley and we welcome contributors.
- Downloads last month
- 4