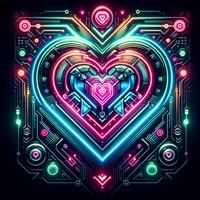
kinokokoro/cyberagent-mistral-nemo-webnovels
Text Generation
•
Updated
•
34
•
1
JA LLM & Data
gpt-3.5-turbo-0125
's JA performance, which is worth noting, and is tuned *exclusively* with the old shisa-v1 dataset (so it's chart position will be very short lived).from transformers import AutoTokenizer
tokenizer = AutoTokenizer.from_pretrained("augmxnt/shisa-7b-v1")
messages = [
{'role': 'user', 'content': 'This is the first user input.'},
{'role': 'assistant', 'content': 'This is the first assistant response.'},
{'role': 'user', 'content': 'This is the second user input.'},
]
print()
print('Chat Template:')
print(tokenizer.chat_template)
print()
print('---')
print()
print(tokenizer.apply_chat_template(messages, tokenize=False))