|
--- |
|
library_name: pytorch |
|
license: agpl-3.0 |
|
pipeline_tag: image-segmentation |
|
tags: |
|
- android |
|
|
|
--- |
|
|
|
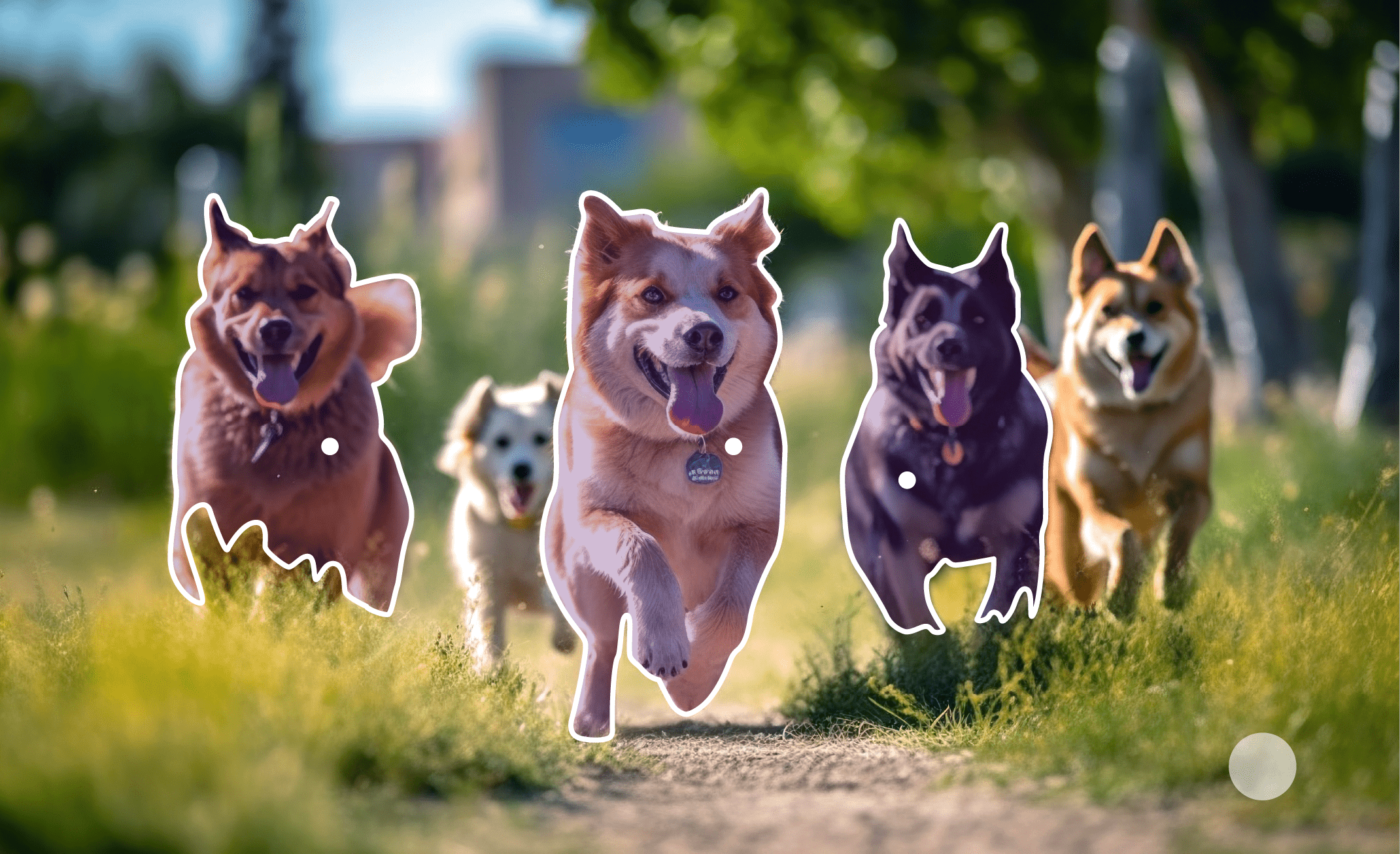 |
|
|
|
# FastSam-S: Optimized for Mobile Deployment |
|
## Generate high quality segmentation mask on device |
|
|
|
The Fast Segment Anything Model (FastSAM) is a novel, real-time CNN-based solution for the Segment Anything task. This task is designed to segment any object within an image based on various possible user interaction prompts. The model performs competitively despite significantly reduced computation, making it a practical choice for a variety of vision tasks. |
|
|
|
This model is an implementation of FastSam-S found [here]({source_repo}). |
|
This repository provides scripts to run FastSam-S on Qualcomm® devices. |
|
More details on model performance across various devices, can be found |
|
[here](https://aihub.qualcomm.com/models/fastsam_s). |
|
|
|
|
|
### Model Details |
|
|
|
- **Model Type:** Semantic segmentation |
|
- **Model Stats:** |
|
- Model checkpoint: fastsam-s.pt |
|
- Inference latency: RealTime |
|
- Input resolution: 640x640 |
|
- Number of parameters: 11.8M |
|
- Model size: 45.1 MB |
|
|
|
| Model | Device | Chipset | Target Runtime | Inference Time (ms) | Peak Memory Range (MB) | Precision | Primary Compute Unit | Target Model |
|
|---|---|---|---|---|---|---|---|---| |
|
| FastSam-S | Samsung Galaxy S23 | Snapdragon® 8 Gen 2 | QNN | 8.064 ms | 4 - 18 MB | FP16 | NPU | [FastSam-S.so](https://huggingface.co/qualcomm/FastSam-S/blob/main/FastSam-S.so) | |
|
| FastSam-S | Samsung Galaxy S23 | Snapdragon® 8 Gen 2 | ONNX | 9.58 ms | 4 - 25 MB | FP16 | NPU | [FastSam-S.onnx](https://huggingface.co/qualcomm/FastSam-S/blob/main/FastSam-S.onnx) | |
|
| FastSam-S | Samsung Galaxy S24 | Snapdragon® 8 Gen 3 | QNN | 6.96 ms | 5 - 38 MB | FP16 | NPU | [FastSam-S.so](https://huggingface.co/qualcomm/FastSam-S/blob/main/FastSam-S.so) | |
|
| FastSam-S | Samsung Galaxy S24 | Snapdragon® 8 Gen 3 | ONNX | 7.273 ms | 1 - 81 MB | FP16 | NPU | [FastSam-S.onnx](https://huggingface.co/qualcomm/FastSam-S/blob/main/FastSam-S.onnx) | |
|
| FastSam-S | QCS8550 (Proxy) | QCS8550 Proxy | QNN | 7.38 ms | 5 - 10 MB | FP16 | NPU | Use Export Script | |
|
| FastSam-S | SA8255 (Proxy) | SA8255P Proxy | QNN | 7.689 ms | 5 - 10 MB | FP16 | NPU | Use Export Script | |
|
| FastSam-S | SA8775 (Proxy) | SA8775P Proxy | QNN | 7.719 ms | 5 - 9 MB | FP16 | NPU | Use Export Script | |
|
| FastSam-S | SA8650 (Proxy) | SA8650P Proxy | QNN | 7.618 ms | 5 - 8 MB | FP16 | NPU | Use Export Script | |
|
| FastSam-S | QCS8450 (Proxy) | QCS8450 Proxy | QNN | 13.749 ms | 5 - 42 MB | FP16 | NPU | Use Export Script | |
|
| FastSam-S | Snapdragon 8 Elite QRD | Snapdragon® 8 Elite | QNN | 5.49 ms | 5 - 36 MB | FP16 | NPU | Use Export Script | |
|
| FastSam-S | Snapdragon 8 Elite QRD | Snapdragon® 8 Elite | ONNX | 5.354 ms | 16 - 62 MB | FP16 | NPU | [FastSam-S.onnx](https://huggingface.co/qualcomm/FastSam-S/blob/main/FastSam-S.onnx) | |
|
| FastSam-S | Snapdragon X Elite CRD | Snapdragon® X Elite | QNN | 8.317 ms | 5 - 5 MB | FP16 | NPU | Use Export Script | |
|
| FastSam-S | Snapdragon X Elite CRD | Snapdragon® X Elite | ONNX | 9.903 ms | 21 - 21 MB | FP16 | NPU | [FastSam-S.onnx](https://huggingface.co/qualcomm/FastSam-S/blob/main/FastSam-S.onnx) | |
|
|
|
|
|
|
|
|
|
## Installation |
|
|
|
This model can be installed as a Python package via pip. |
|
|
|
```bash |
|
pip install "qai-hub-models[fastsam_s]" |
|
``` |
|
|
|
|
|
|
|
## Configure Qualcomm® AI Hub to run this model on a cloud-hosted device |
|
|
|
Sign-in to [Qualcomm® AI Hub](https://app.aihub.qualcomm.com/) with your |
|
Qualcomm® ID. Once signed in navigate to `Account -> Settings -> API Token`. |
|
|
|
With this API token, you can configure your client to run models on the cloud |
|
hosted devices. |
|
```bash |
|
qai-hub configure --api_token API_TOKEN |
|
``` |
|
Navigate to [docs](https://app.aihub.qualcomm.com/docs/) for more information. |
|
|
|
|
|
|
|
## Demo off target |
|
|
|
The package contains a simple end-to-end demo that downloads pre-trained |
|
weights and runs this model on a sample input. |
|
|
|
```bash |
|
python -m qai_hub_models.models.fastsam_s.demo |
|
``` |
|
|
|
The above demo runs a reference implementation of pre-processing, model |
|
inference, and post processing. |
|
|
|
**NOTE**: If you want running in a Jupyter Notebook or Google Colab like |
|
environment, please add the following to your cell (instead of the above). |
|
``` |
|
%run -m qai_hub_models.models.fastsam_s.demo |
|
``` |
|
|
|
|
|
### Run model on a cloud-hosted device |
|
|
|
In addition to the demo, you can also run the model on a cloud-hosted Qualcomm® |
|
device. This script does the following: |
|
* Performance check on-device on a cloud-hosted device |
|
* Downloads compiled assets that can be deployed on-device for Android. |
|
* Accuracy check between PyTorch and on-device outputs. |
|
|
|
```bash |
|
python -m qai_hub_models.models.fastsam_s.export |
|
``` |
|
``` |
|
Profiling Results |
|
------------------------------------------------------------ |
|
FastSam-S |
|
Device : Samsung Galaxy S23 (13) |
|
Runtime : QNN |
|
Estimated inference time (ms) : 8.1 |
|
Estimated peak memory usage (MB): [4, 18] |
|
Total # Ops : 286 |
|
Compute Unit(s) : NPU (286 ops) |
|
``` |
|
|
|
|
|
## How does this work? |
|
|
|
This [export script](https://aihub.qualcomm.com/models/fastsam_s/qai_hub_models/models/FastSam-S/export.py) |
|
leverages [Qualcomm® AI Hub](https://aihub.qualcomm.com/) to optimize, validate, and deploy this model |
|
on-device. Lets go through each step below in detail: |
|
|
|
Step 1: **Compile model for on-device deployment** |
|
|
|
To compile a PyTorch model for on-device deployment, we first trace the model |
|
in memory using the `jit.trace` and then call the `submit_compile_job` API. |
|
|
|
```python |
|
import torch |
|
|
|
import qai_hub as hub |
|
from qai_hub_models.models.fastsam_s import |
|
|
|
# Load the model |
|
|
|
# Device |
|
device = hub.Device("Samsung Galaxy S23") |
|
|
|
|
|
``` |
|
|
|
|
|
Step 2: **Performance profiling on cloud-hosted device** |
|
|
|
After compiling models from step 1. Models can be profiled model on-device using the |
|
`target_model`. Note that this scripts runs the model on a device automatically |
|
provisioned in the cloud. Once the job is submitted, you can navigate to a |
|
provided job URL to view a variety of on-device performance metrics. |
|
```python |
|
profile_job = hub.submit_profile_job( |
|
model=target_model, |
|
device=device, |
|
) |
|
|
|
``` |
|
|
|
Step 3: **Verify on-device accuracy** |
|
|
|
To verify the accuracy of the model on-device, you can run on-device inference |
|
on sample input data on the same cloud hosted device. |
|
```python |
|
input_data = torch_model.sample_inputs() |
|
inference_job = hub.submit_inference_job( |
|
model=target_model, |
|
device=device, |
|
inputs=input_data, |
|
) |
|
on_device_output = inference_job.download_output_data() |
|
|
|
``` |
|
With the output of the model, you can compute like PSNR, relative errors or |
|
spot check the output with expected output. |
|
|
|
**Note**: This on-device profiling and inference requires access to Qualcomm® |
|
AI Hub. [Sign up for access](https://myaccount.qualcomm.com/signup). |
|
|
|
|
|
|
|
## Run demo on a cloud-hosted device |
|
|
|
You can also run the demo on-device. |
|
|
|
```bash |
|
python -m qai_hub_models.models.fastsam_s.demo --on-device |
|
``` |
|
|
|
**NOTE**: If you want running in a Jupyter Notebook or Google Colab like |
|
environment, please add the following to your cell (instead of the above). |
|
``` |
|
%run -m qai_hub_models.models.fastsam_s.demo -- --on-device |
|
``` |
|
|
|
|
|
## Deploying compiled model to Android |
|
|
|
|
|
The models can be deployed using multiple runtimes: |
|
- TensorFlow Lite (`.tflite` export): [This |
|
tutorial](https://www.tensorflow.org/lite/android/quickstart) provides a |
|
guide to deploy the .tflite model in an Android application. |
|
|
|
|
|
- QNN (`.so` export ): This [sample |
|
app](https://docs.qualcomm.com/bundle/publicresource/topics/80-63442-50/sample_app.html) |
|
provides instructions on how to use the `.so` shared library in an Android application. |
|
|
|
|
|
## View on Qualcomm® AI Hub |
|
Get more details on FastSam-S's performance across various devices [here](https://aihub.qualcomm.com/models/fastsam_s). |
|
Explore all available models on [Qualcomm® AI Hub](https://aihub.qualcomm.com/) |
|
|
|
|
|
## License |
|
* The license for the original implementation of FastSam-S can be found [here](https://github.com/CASIA-IVA-Lab/FastSAM/blob/main/LICENSE). |
|
* The license for the compiled assets for on-device deployment can be found [here](https://github.com/CASIA-IVA-Lab/FastSAM/blob/main/LICENSE) |
|
|
|
|
|
|
|
## References |
|
* [Fast Segment Anything](https://arxiv.org/abs/2306.12156) |
|
* [Source Model Implementation](https://github.com/CASIA-IVA-Lab/FastSAM) |
|
|
|
|
|
|
|
## Community |
|
* Join [our AI Hub Slack community](https://aihub.qualcomm.com/community/slack) to collaborate, post questions and learn more about on-device AI. |
|
* For questions or feedback please [reach out to us](mailto:ai-hub-support@qti.qualcomm.com). |
|
|
|
|
|
|